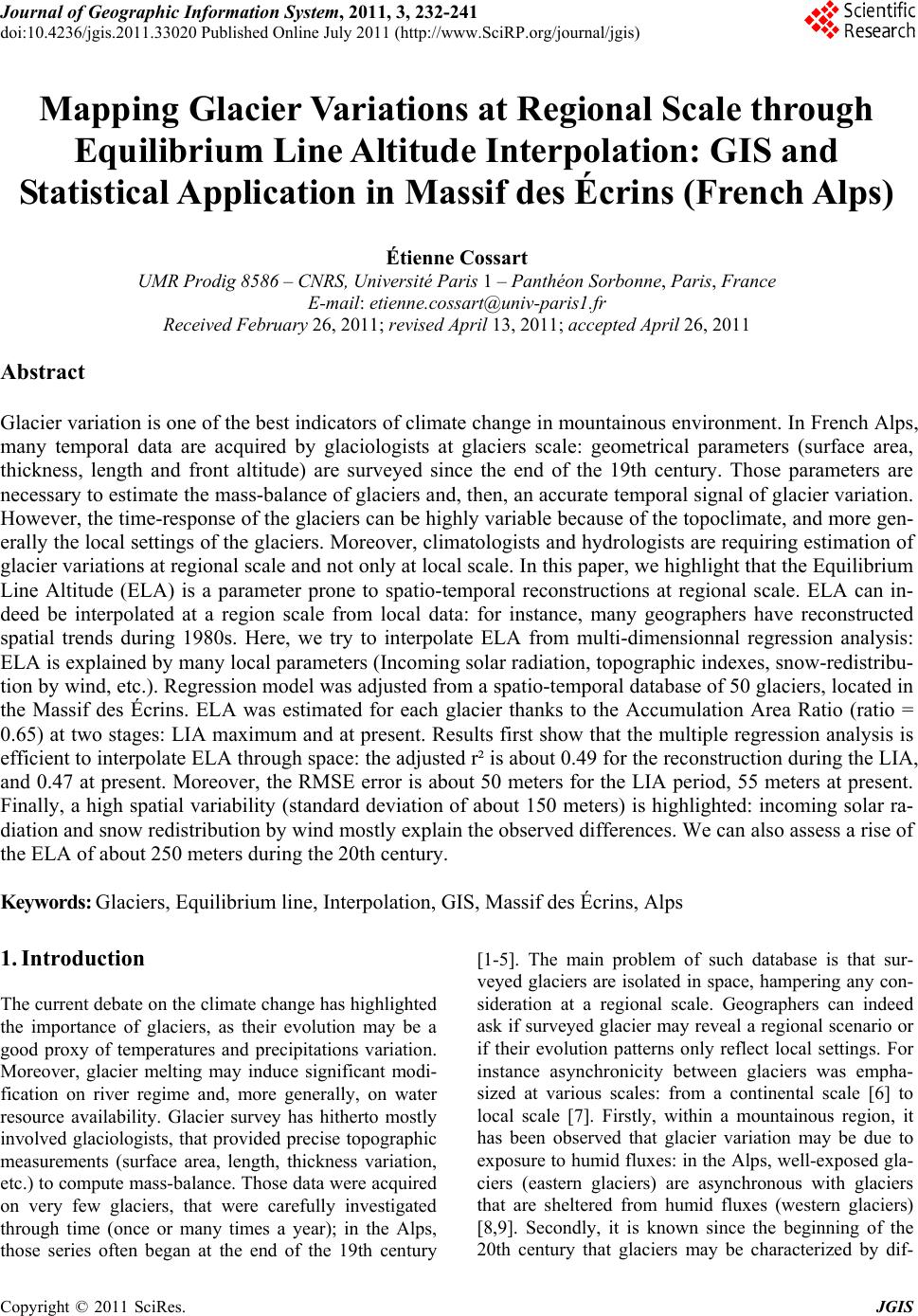 Journal of Geographic Information System, 2011, 3, 232-241 doi:10.4236/jgis.2011.33020 Published Online July 2011 (http://www.SciRP.org/journal/jgis) Copyright © 2011 SciRes. JGIS Mapping Glacier Variations at Regional Sca le through Equilibrium Line Altitude Interpolation: GIS and Statistical Application in Massif des Écrins (French Alps) Étienne Cossart UMR Prodig 8586 – CNRS, Université Paris 1 – Panthéon Sorbonne, Paris, France E-mail: etienne.cossart@univ-paris1.fr Received February 26, 201 1; revised April 13, 2011; accepted April 26, 2011 Abstract Glacier variation is one of the best indicators of climate change in mountainous environment. In French Alps, many temporal data are acquired by glaciologists at glaciers scale: geometrical parameters (surface area, thickness, length and front altitude) are surveyed since the end of the 19th century. Those parameters are necessary to estimate the mass-balance of glaciers and, then, an accurate temporal signal of glacier variation. However, the time-response of the glaciers can be highly variable because of the topoclimate, and more gen- erally the local settings of the glaciers. Moreover, climatologists and hydrologists are requiring estimation of glacier variations at regional scale and not only at local scale. In this paper, we highlight that the Equilibrium Line Altitude (ELA) is a parameter prone to spatio-temporal reconstructions at regional scale. ELA can in- deed be interpolated at a region scale from local data: for instance, many geographers have reconstructed spatial trends during 1980s. Here, we try to interpolate ELA from multi-dimensionnal regression analysis: ELA is explained by many local parameters (Incoming solar radiation, topographic indexes, snow-redistribu- tion by wind, etc.). Regression model was adjusted from a spatio-temporal database of 50 glaciers, located in the Massif des Écrins. ELA was estimated for each glacier thanks to the Accumulation Area Ratio (ratio = 0.65) at two stages: LIA maximum and at present. Results first show that the multiple regression analysis is efficient to interpolate ELA through space: the adjusted r² is about 0.49 for the reconstruction during the LIA, and 0.47 at present. Moreover, the RMSE error is about 50 meters for the LIA period, 55 meters at present. Finally, a high spatial variability (standard deviation of about 150 meters) is highlighted: incoming solar ra- diation and snow redistribution by wind mostly explain the observed differences. We can also assess a rise of the ELA of about 250 meters during the 20th century. Keywords: Glaciers, Equilibrium line, Interpolation, GIS, Massif des Écrins, Alps 1. Introduction The current debate on the climate change has highlighted the importance of glaciers, as their evolution may be a good proxy of temperatures and precipitations variation. Moreover, glacier melting may induce significant modi- fication on river regime and, more generally, on water resource availability. Glacier survey has hitherto mostly involved glaciologists, that provided precise topographic measurements (surface area, length, thickness variation, etc.) to compute mass-balance. Those data were acquired on very few glaciers, that were carefully investigated through time (once or many times a year); in the Alps, those series often began at the end of the 19th century [1-5]. The main problem of such database is that sur- veyed glaciers are isolated in space, hampering any con- sideration at a regional scale. Geographers can indeed ask if surveyed glacier may reveal a regional scenario or if their evolution patterns only reflect local settings. For instance asynchronicity between glaciers was empha- sized at various scales: from a continental scale [6] to local scale [7]. Firstly, within a mountainous region, it has been observed that glacier variation may be due to exposure to humid fluxes: in the Alps, well-exposed gla- ciers (eastern glaciers) are asynchronous with glaciers that are sheltered from humid fluxes (western glaciers) [8,9]. Secondly, it is known since the beginning of the 20th century that glaciers may be characterized by dif-
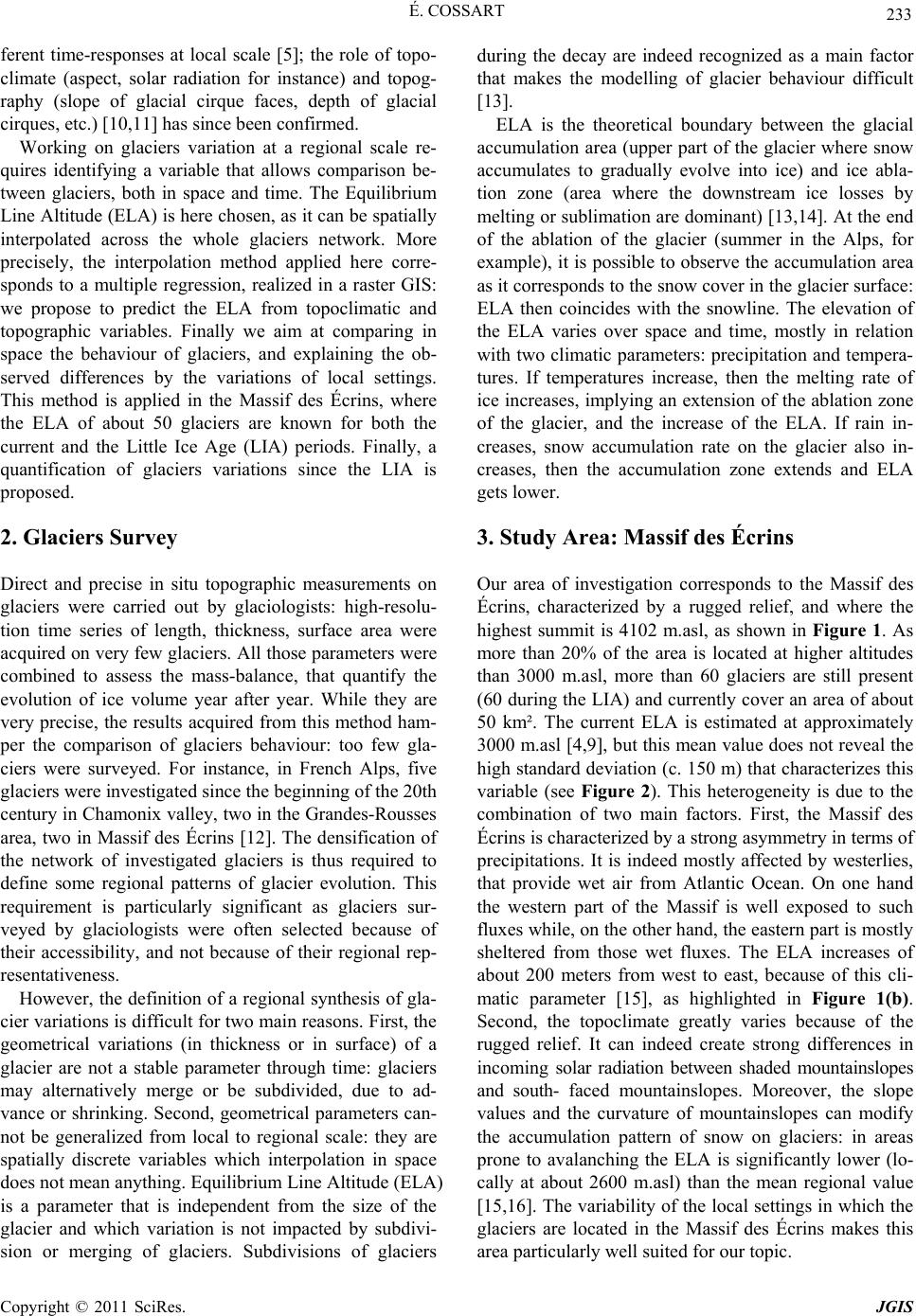 É. COSSART 233 ferent time-responses at local scale [5]; the role of topo- climate (aspect, solar radiation for instance) and topog- raphy (slope of glacial cirque faces, depth of glacial cirques, etc.) [10,11] has since been confirmed. Working on glaciers variation at a regional scale re- quires identifying a variable that allows comparison be- tween glaciers, both in space and time. The Equilibrium Line Altitude (ELA) is here chosen, as it can be spatially interpolated across the whole glaciers network. More precisely, the interpolation method applied here corre- sponds to a multiple regression, realized in a raster GIS: we propose to predict the ELA from topoclimatic and topographic variables. Finally we aim at comparing in space the behaviour of glaciers, and explaining the ob- served differences by the variations of local settings. This method is applied in the Massif des Écrins, where the ELA of about 50 glaciers are known for both the current and the Little Ice Age (LIA) periods. Finally, a quantification of glaciers variations since the LIA is proposed. 2. Glaciers Survey Direct and precise in situ topographic measurements on glaciers were carried out by glaciologists: high-resolu- tion time series of length, thickness, surface area were acquired on very few glaciers. All those parameters were combined to assess the mass-balance, that quantify the evolution of ice volume year after year. While they are very precise, the results acquired from this method ham- per the comparison of glaciers behaviour: too few gla- ciers were surveyed. For instance, in French Alps, five glaciers were investigated since the beginning of the 20th century in Chamonix valley, two in the Grandes-Rousses area, two in Massif des Écrins [12]. The densification of the network of investigated glaciers is thus required to define some regional patterns of glacier evolution. This requirement is particularly significant as glaciers sur- veyed by glaciologists were often selected because of their accessibility, and not because of their regional rep- resentativeness. However, the definition of a regional synthesis of gla- cier variations is difficult for two main reasons. First, the geometrical variations (in thickness or in surface) of a glacier are not a stable parameter through time: glaciers may alternatively merge or be subdivided, due to ad- vance or shrinking. Second, geometrical parameters can- not be generalized from local to regional scale: they are spatially discrete variables which interpolation in space does not mean anything. Equilibrium Line Altitude (ELA) is a parameter that is independent from the size of the glacier and which variation is not impacted by subdivi- sion or merging of glaciers. Subdivisions of glaciers during the decay are indeed recognized as a main factor that makes the modelling of glacier behaviour difficult [13]. ELA is the theoretical boundary between the glacial accumulation area (upper part of the glacier where snow accumulates to gradually evolve into ice) and ice abla- tion zone (area where the downstream ice losses by melting or sublimation are dominant) [13,14]. At the end of the ablation of the glacier (summer in the Alps, for example), it is possible to observe the accumulation area as it corresponds to the snow cover in the glacier surface: ELA then coincides with the snowline. The elevation of the ELA varies over space and time, mostly in relation with two climatic parameters: precipitation and tempera- tures. If temperatures increase, then the melting rate of ice increases, implying an extension of the ablation zone of the glacier, and the increase of the ELA. If rain in- creases, snow accumulation rate on the glacier also in- creases, then the accumulation zone extends and ELA gets lower. 3. Study Area: Massif des Écrin s Our area of investigation corresponds to the Massif des Écrins, characterized by a rugged relief, and where the highest summit is 4102 m.asl, as shown in Figure 1. As more than 20% of the area is located at higher altitudes than 3000 m.asl, more than 60 glaciers are still present (60 during the LIA) and currently cover an area of about 50 km². The current ELA is estimated at approximately 3000 m.asl [4,9], but this mean value does not reveal the high standard deviation (c. 150 m) that characterizes this variable (see Figure 2). This heterogeneity is due to the combination of two main factors. First, the Massif des Écrins is characterized by a strong asymmetry in terms of precipitations. It is indeed mostly affected by westerlies, that provide wet air from Atlantic Ocean. On one hand the western part of the Massif is well exposed to such fluxes while, on the other hand , the eastern part is mostly sheltered from those wet fluxes. The ELA increases of about 200 meters from west to east, because of this cli- matic parameter [15], as highlighted in Figure 1(b). Second, the topoclimate greatly varies because of the rugged relief. It can indeed create strong differences in incoming solar radiation between shaded mountainslopes and south- faced mountainslopes. Moreover, the slope values and the curvature of mountainslopes can modify the accumulation pattern of snow on glaciers: in areas prone to avalanching the ELA is significantly lower (lo- cally at about 2600 m.asl) than the mean regional value [15,16]. The variability of the local setting s in which the glaciers are located in the Massif des Écrins makes this area particularly well suited for our topic. Copyright © 2011 SciRes. JGIS
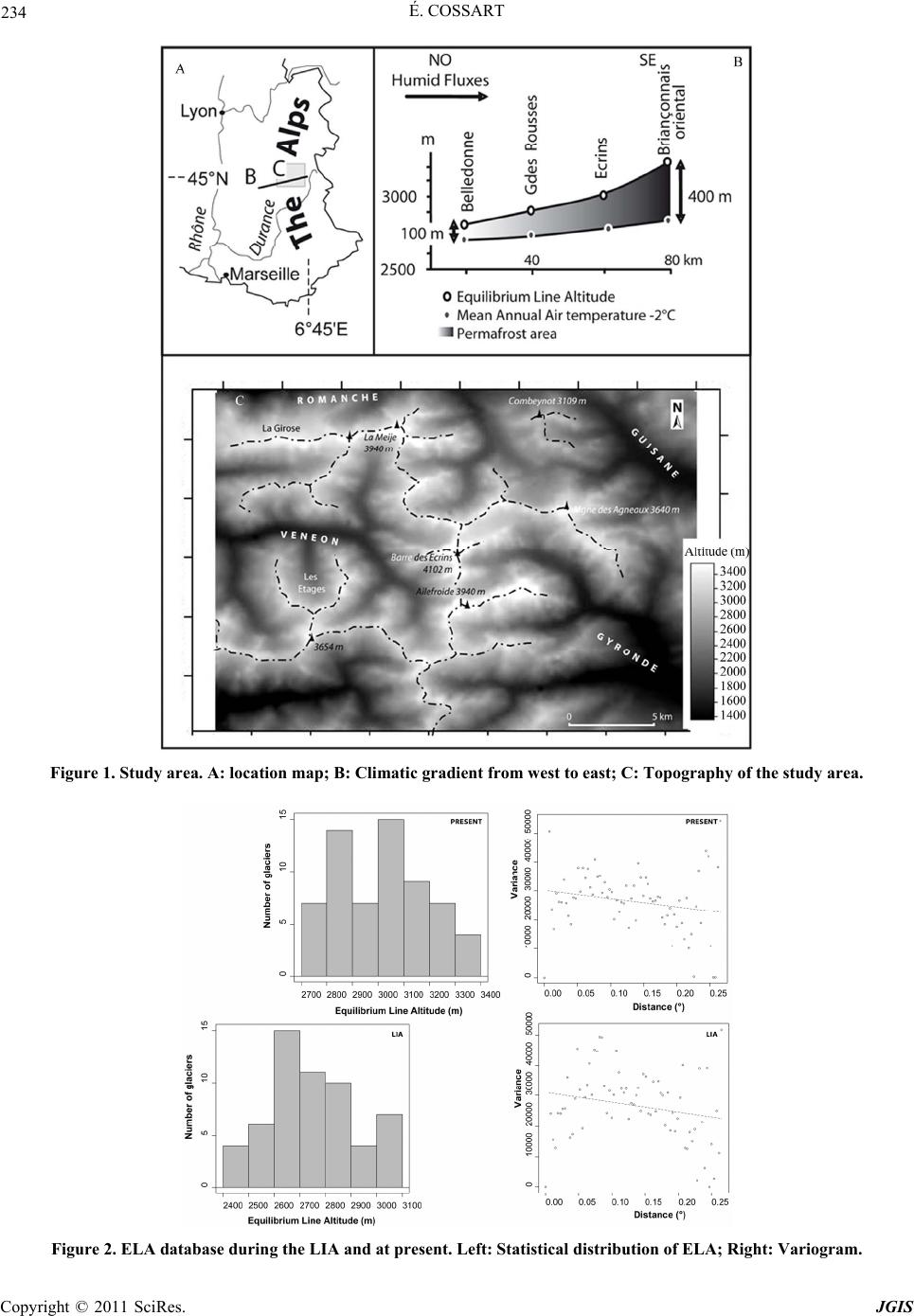 É. COSSART Copyright © 2011 SciRes. JGIS 234 Figure 1. Study area. A: location map; B: Climatic gradient from west to east; C: Topography of the study area. Figure 2. ELA database during the LIA and at prese nt. Left: Statistical distribution of ELA; Right: Variogram.
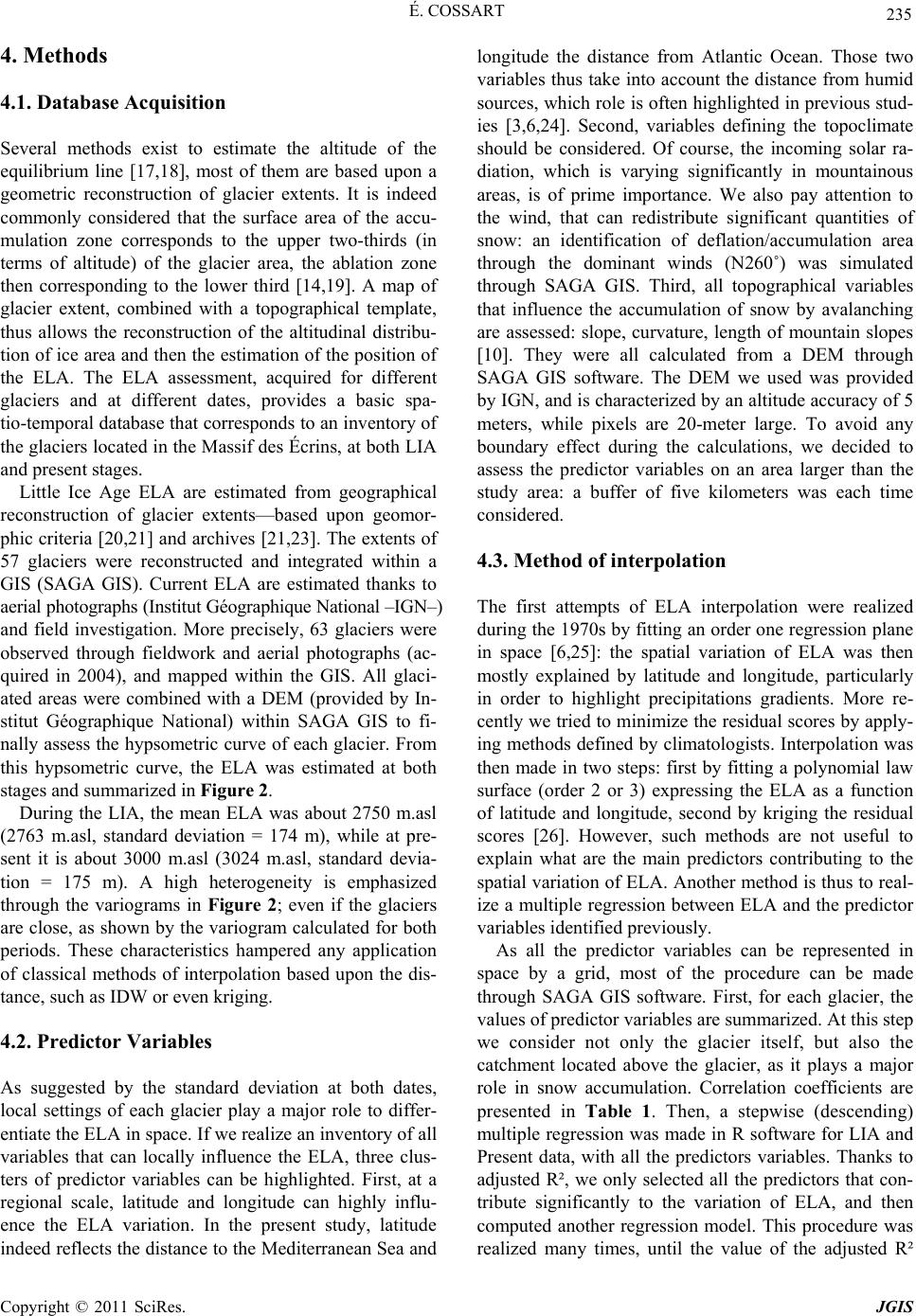 É. COSSART Copyright © 2011 SciRes. JGIS 235 4. Methods 4.1. Database Acquisition Several methods exist to estimate the altitude of the equilibrium line [17,18], most of them are based upon a geometric reconstruction of glacier extents. It is indeed commonly considered that the surface area of the accu- mulation zone corresponds to the upper two-thirds (in terms of altitude) of the glacier area, the ablation zone then corresponding to the lower third [14,19]. A map of glacier extent, combined with a topographical template, thus allows the reconstruction of the altitudinal distribu- tion of ice area and then the estimation of the position of the ELA. The ELA assessment, acquired for different glaciers and at different dates, provides a basic spa- tio-temporal database that corresponds to an inventory of the glaciers located in the Massif des Écrins, at both LIA and present stages. Little Ice Age ELA are estimated from geographical reconstruction of glacier extents—based upon geomor- phic criteria [20,21] and archives [21,23]. The extents of 57 glaciers were reconstructed and integrated within a GIS (SAGA GIS). Current ELA are estimated thanks to aerial photographs (Institut Géographique National –IGN–) and field investigation. More precisely, 63 glaciers were observed through fieldwork and aerial photographs (ac- quired in 2004), and mapped within the GIS. All glaci- ated areas were combined with a DEM (provided by In- stitut Géographique National) within SAGA GIS to fi- nally assess the hypsometric curve of each glacier. From this hypsometric curve, the ELA was estimated at both stages and summarized in Figure 2. During the LIA, the mean ELA was about 2750 m.asl (2763 m.asl, standard deviation = 174 m), while at pre- sent it is about 3000 m.asl (3024 m.asl, standard devia- tion = 175 m). A high heterogeneity is emphasized through the variograms in Figure 2; even if the glaciers are close, as shown by the variogram calculated for both periods. These characteristics hampered any application of classical methods of interpolation based upon the dis- tance, such as IDW or even kriging. 4.2. Predictor Variables As suggested by the standard deviation at both dates, local settings of each glacier play a major role to differ- entiate the ELA in space. If we realize an inventory of all variables that can locally influence the ELA, three clus- ters of predictor variables can be highlighted. First, at a regional scale, latitude and longitude can highly influ- ence the ELA variation. In the present study, latitude indeed reflects the distance to the Mediterranean Sea and longitude the distance from Atlantic Ocean. Those two variables thus take into account the distance from humid sources, which role is of ten highlighted in previous stud- ies [3,6,24]. Second, variables defining the topoclimate should be considered. Of course, the incoming solar ra- diation, which is varying significantly in mountainous areas, is of prime importance. We also pay attention to the wind, that can redistribute significant quantities of snow: an identification of deflation/accumulation area through the dominant winds (N260˚) was simulated through SAGA GIS. Third, all topographical variables that influence the accumulation of snow by avalanching are assessed: slope, curvature, length of mountain slopes [10]. They were all calculated from a DEM through SAGA GIS software. The DEM we used was provided by IGN, and is characterized by an altitude accuracy of 5 meters, while pixels are 20-meter large. To avoid any boundary effect during the calculations, we decided to assess the predictor variables on an area larger than the study area: a buffer of five kilometers was each time considered. 4.3. Method of interpolation The first attempts of ELA interpolation were realized during the 1970s by fitting an order one regression plane in space [6,25]: the spatial variation of ELA was then mostly explained by latitude and longitude, particularly in order to highlight precipitations gradients. More re- cently we tried to minimize the residual scores b y apply- ing methods defined by climatologists. Interpolation was then made in two steps: first by fitting a polynomial law surface (order 2 or 3) expressing the ELA as a function of latitude and longitude, second by kriging the residual scores [26]. However, such methods are not useful to explain what are the main predictors contributing to the spatial variation of ELA. Another method is thus to real- ize a multiple regression between ELA and the predictor variables identified previously. As all the predictor variables can be represented in space by a grid, most of the procedure can be made through SAGA GIS software. First, for each glacier, the values of pr ed ictor variables are summarized. At this step we consider not only the glacier itself, but also the catchment located above the glacier, as it plays a major role in snow accumulation. Correlation coefficients are presented in Table 1. Then, a stepwise (descending) multiple regression was made in R software for LIA and Present data, with all the predictors variables. Thanks to adjusted R², we only selected all the predictors that con- tribute significantly to the variation of ELA, and then computed another regression model. This procedure was realized many times, until the value of the adjusted R²
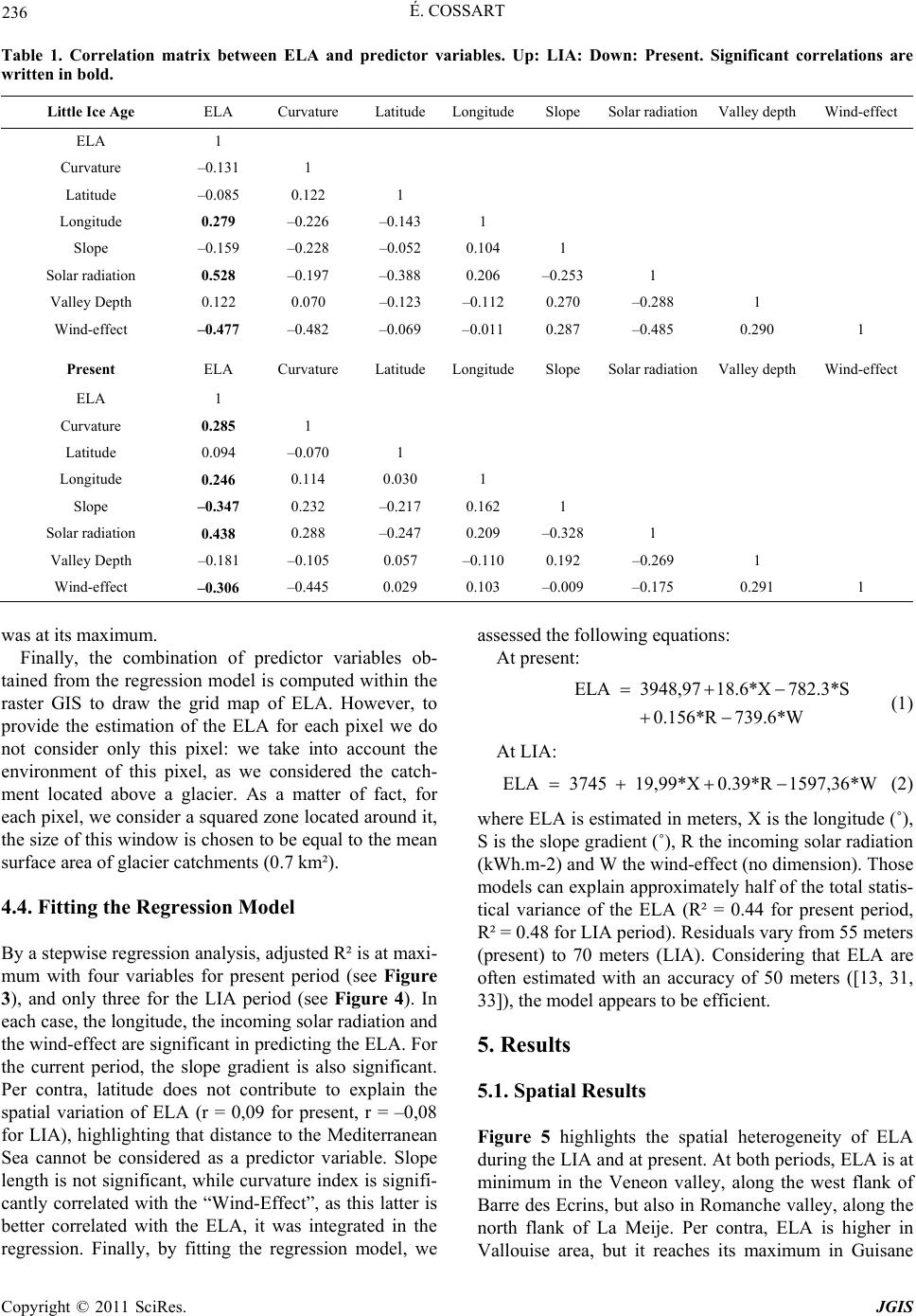 É. COSSART Copyright © 2011 SciRes. JGIS 236 Table 1. Correlation matrix between ELA and predictor variables. Up: LIA: Down: Present. Significant correlations are written in bold. Little Ice Age ELA Curvature Latitude LongitudeSlope Solar radiation Valley depth Wind-effect ELA 1 Curvature –0.131 1 Latitude –0.085 0.122 1 Longitude 0.279 –0.226 –0.143 1 Slope –0.159 –0.228 –0.052 0.104 1 Solar radiation 0.528 –0.197 –0.388 0.206 –0.253 1 Valley Depth 0.122 0.070 –0.123 –0.112 0.270 –0.288 1 Wind-effect –0.477 –0.482 –0.069 –0.011 0.287 –0.485 0.290 1 Present ELA Curvature Latitude LongitudeSlope Solar radiation Valley depth Wind-effect ELA 1 Curvature 0.285 1 Latitude 0.094 –0.070 1 Longitude 0.246 0.114 0.030 1 Slope –0.347 0.232 –0.217 0.162 1 Solar radiation 0.438 0.288 –0.247 0.209 –0.328 1 Valley Depth –0.181 –0.105 0.057 –0.110 0.192 –0.269 1 Wind-effect –0.306 –0.445 0.029 0.103 –0.009 –0.175 0.291 1 wa s at its maximum. Finally, the combination of predictor variables ob- tained from the regression model is computed within the raster GIS to draw the grid map of ELA. However, to provide the estimation of the ELA for each pixel we do not consider only this pixel: we take into account the environment of this pixel, as we considered the catch- ment located above a glacier. As a matter of fact, for each pixel, we consider a squared zone located around it, the size of this window is chosen to be equal to the mean surface area of glacier catchments (0.7 km²). 4.4. Fitting the Regression Model By a stepwise regression analysis, adjusted R² is at maxi- mum with four variables for present period (see Figure 3), and only three for the LIA period (see Figure 4). In each case, the longitude, the incoming solar radiation and the wind-effect are significant in predicting the ELA. For the current period, the slope gradient is also significant. Per contra, latitude does not contribute to explain the spatial variation of ELA (r = 0,09 for present, r = –0,08 for LIA), highlighting that distance to the Mediterranean Sea cannot be considered as a predictor variable. Slope length is not significant, while curvature index is signifi- cantly correlated with the “Wind-Effect”, as this latter is better correlated with the ELA, it was integrated in the regression. Finally, by fitting the regression model, we assessed the following equations : At present: ELA 3948,9718.6*X782.3*S 0.156*R 739.6*W (1) At LIA: ELA 3745 19,99*X0.39*R1597,36*W (2) where ELA is estimated in meters, X is the longitude (˚), S is the slope gradient (˚), R the incoming solar radiation (kWh.m-2) and W the wind-effect (no dimension). Those models can explain approximately half of the total statis- tical variance of the ELA (R² = 0.44 for present period, R² = 0.48 for LIA period). Residuals vary from 55 meters (present) to 70 meters (LIA). Considering that ELA are often estimated with an accuracy of 50 meters ([13, 31, 33]), the model appears to be efficient. 5. Results 5.1. Spatial Results Figure 5 highlights the spatial heterogeneity of ELA during the LIA and at presen t. At both periods, ELA is at minimum in the Veneon valley, along the west flank of Barre des Ecrins, but also in Romanche valley, along the north flank of La Meije. Per contra, ELA is higher in Vallouise area, but it reaches its maximum in Guisane
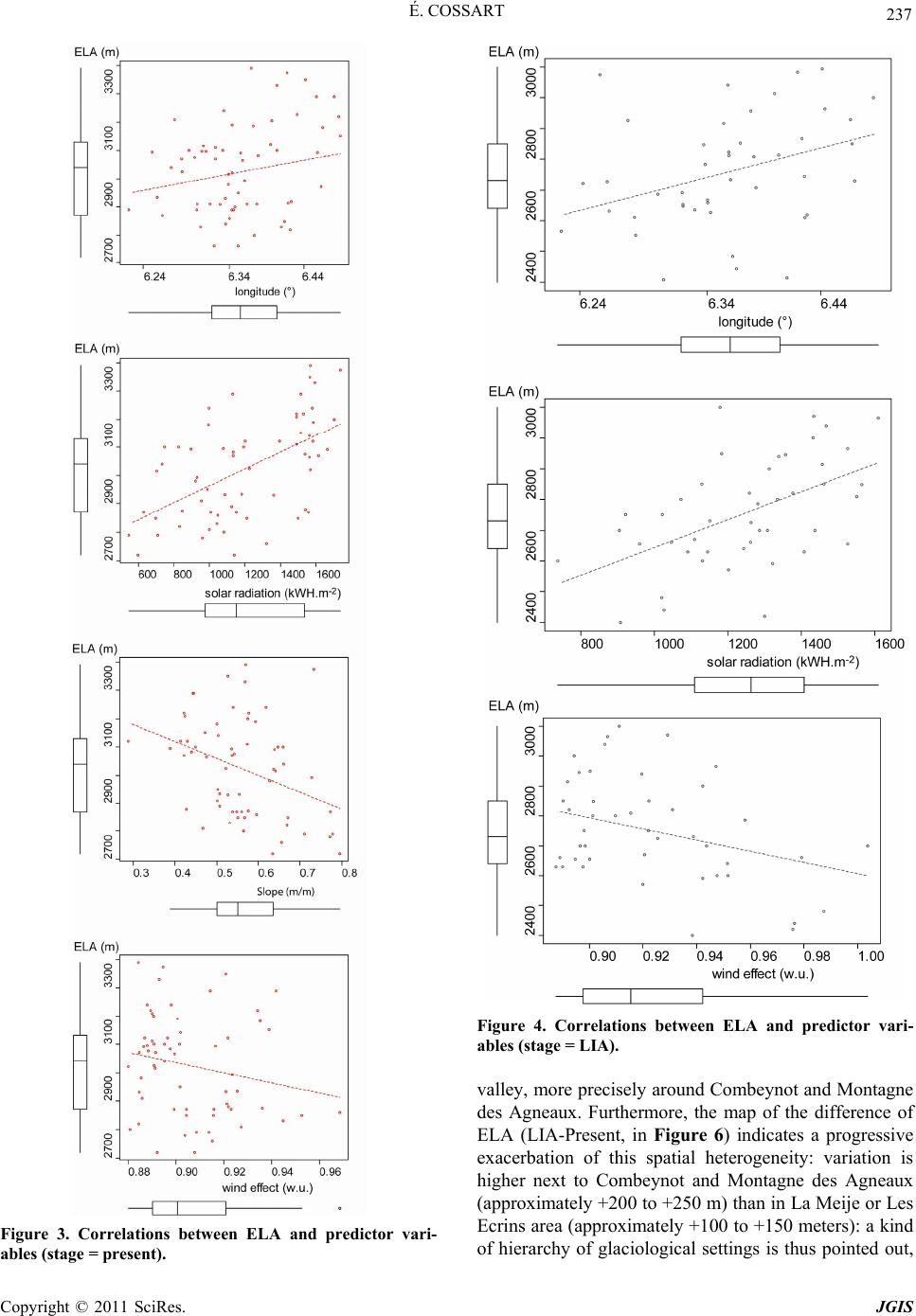 É. COSSART 237 Figure 3. Correlations between ELA and predictor vari- ables (stage = present). Figure 4. Correlations between ELA and predictor vari- ables (stage = LIA). valley, more precisely around Combeynot and Montagne des Agneaux. Furthermore, the map of the difference of ELA (LIA-Present, in Figure 6) indicates a progressive exacerbation of this spatial heterogeneity: variation is higher next to Combeynot and Montagne des Agneaux (approximately +200 to +2 50 m) than in La Meije or Les Ecrins area (approximately +100 to +150 meters): a kind of hierarchy of glaciological settings is thus pointed out, Copyright © 2011 SciRes. JGIS
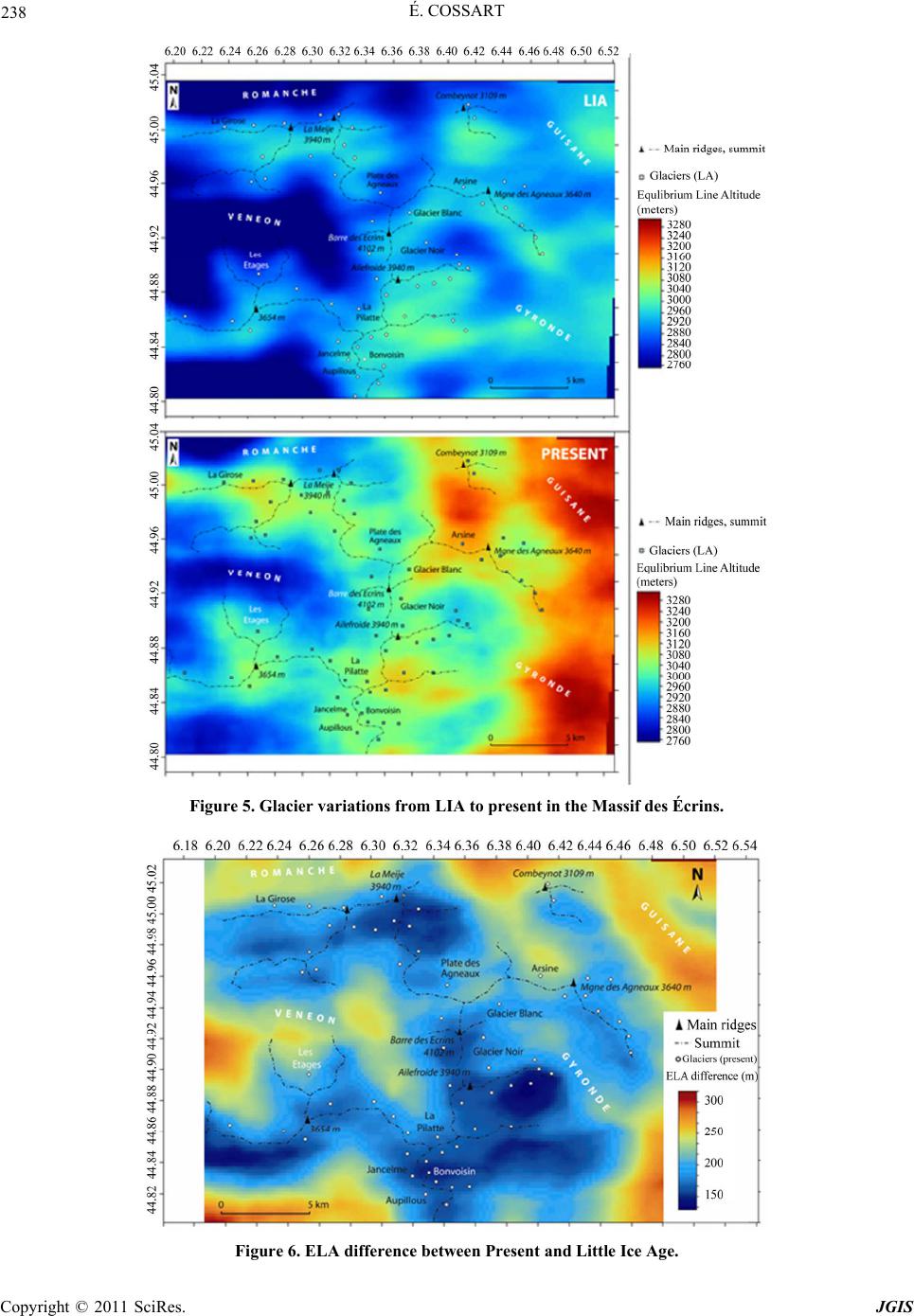 É. COSSART Copyright © 2011 SciRes. JGIS 238 Figure 5. Glacier variations from LIA to present in the Massif des Écrins. Figure 6. ELA difference between Present and Little Ice Age.
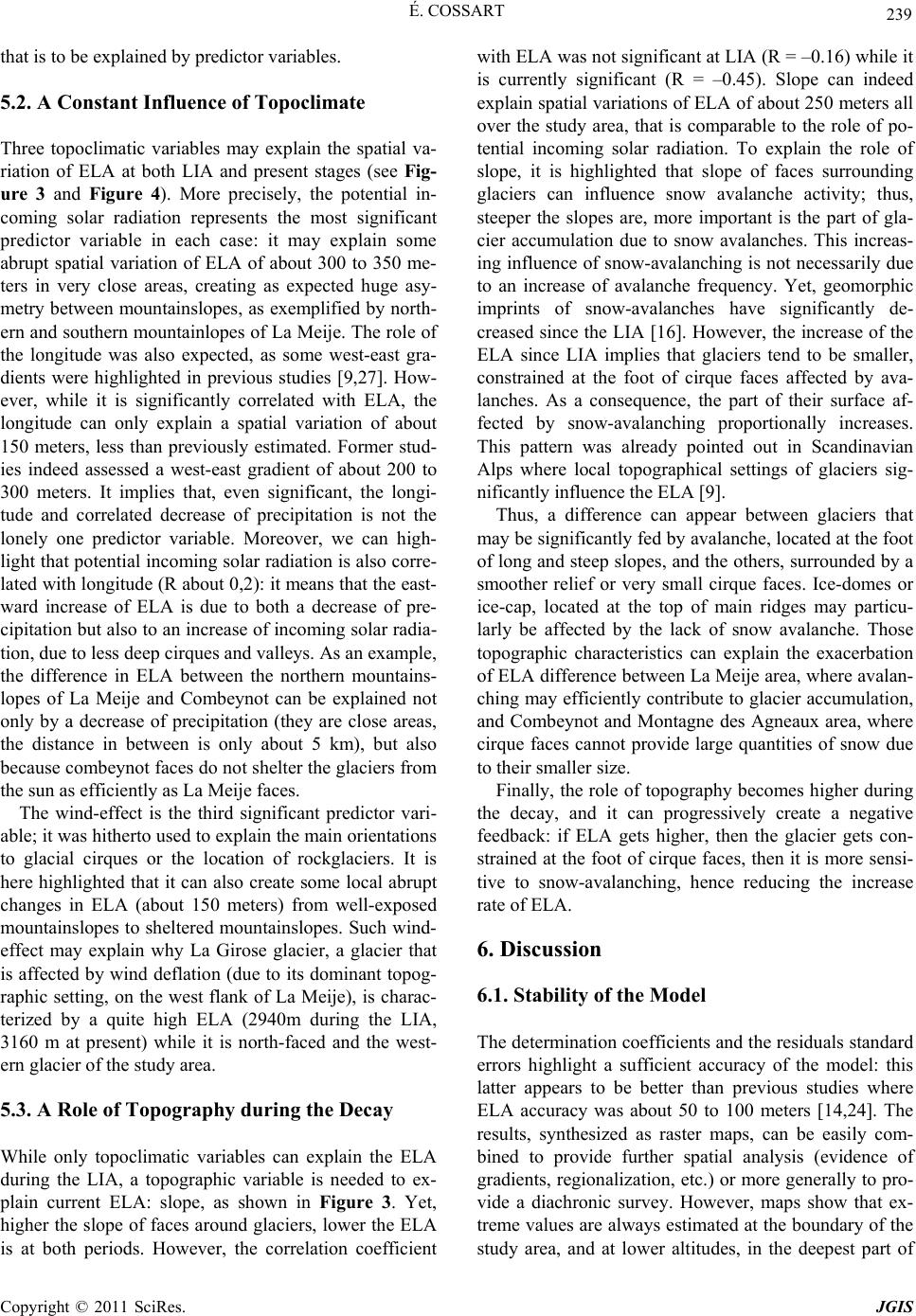 É. COSSART Copyright © 2011 SciRes. JGIS 239 that is to be explained b y predictor variables. 5.2. A Constant Influence of Topoclimate Three topoclimatic variables may explain the spatial va- riation of ELA at both LIA and present stages (see Fig- ure 3 and Figure 4). More precisely, the potential in- coming solar radiation represents the most significant predictor variable in each case: it may explain some abrupt spatial variation of ELA of about 300 to 350 me- ters in very close areas, creating as expected huge asy- metry between mountainslopes, as exemplified by north- ern and southern mountainlopes of La Meije. The role of the longitude was also expected, as some west-east gra- dients were highlighted in previous studies [9,27]. How- ever, while it is significantly correlated with ELA, the longitude can only explain a spatial variation of about 150 meters, less than previously estimated. Former stud- ies indeed assessed a west-east gradient of about 200 to 300 meters. It implies that, even significant, the longi- tude and correlated decrease of precipitation is not the lonely one predictor variable. Moreover, we can high- light that potential inco ming solar radiation is also corre- lated with longitude (R about 0,2 ): it means that the east- ward increase of ELA is due to both a decrease of pre- cipitation but also to an increase of incoming solar rad ia- tion, due to less deep cirques and valleys. As an example, the difference in ELA between the northern mountains- lopes of La Meije and Combeynot can be explained not only by a decrease of precipitation (they are close areas, the distance in between is only about 5 km), but also because combeynot faces do not shelter the glaciers from the sun as efficiently as La Meije faces. The wind-effect is the third significant predictor vari- able; it was hitherto used to explain the main orientations to glacial cirques or the location of rockglaciers. It is here highlighted that it can also create some local abrupt changes in ELA (about 150 meters) from well-exposed mountainslopes to sheltered mountainslopes. Such wind- effect may explain why La Girose glacier, a glacier that is affected by wind deflation (due to its domin ant topog- raphic setting, on the west flank of La Meije), is charac- terized by a quite high ELA (2940m during the LIA, 3160 m at present) while it is north-faced and the west- ern glacier of the study area. 5.3. A Role of Topography during the Decay While only topoclimatic variables can explain the ELA during the LIA, a topographic variable is needed to ex- plain current ELA: slope, as shown in Figure 3. Yet, higher the slope of faces around glaciers, lower the ELA is at both periods. However, the correlation coefficient with ELA was not significant at LIA (R = –0.16) while it is currently significant (R = –0.45). Slope can indeed explain spatial variations of ELA of abou t 250 meters all over the study area, that is comparable to the role of po- tential incoming solar radiation. To explain the role of slope, it is highlighted that slope of faces surrounding glaciers can influence snow avalanche activity; thus, steeper the slopes are, more important is the part of gla- cier accumulation due to snow avalanches. This increas- ing influence of snow-avalanching is not necessarily due to an increase of avalanche frequency. Yet, geomorphic imprints of snow-avalanches have significantly de- creased since the LIA [16]. However, the increase of the ELA since LIA implies that glaciers tend to be smaller, constrained at the foot of cirque faces affected by ava- lanches. As a consequence, the part of their surface af- fected by snow-avalanching proportionally increases. This pattern was already pointed out in Scandinavian Alps where local topographical settings of glaciers sig- nificantly influence the ELA [9]. Thus, a difference can appear between glaciers that may be significantly fe d by avalanche, located at the foot of long and steep slopes, and the others, surrounded by a smoother relief or very small cirque faces. Ice-domes or ice-cap, located at the top of main ridges may particu- larly be affected by the lack of snow avalanche. Those topographic characteristics can explain the exacerbation of ELA difference between La Meije area, where avalan- ching may efficiently contribute to glacier accumulation, and Combeynot and Montagne des Agneaux area, where cirque faces cannot provide large quantities of snow due to their smaller size. Finally, the role of topography becomes higher during the decay, and it can progressively create a negative feedback: if ELA gets higher, then the glacier gets con- strained at the foot of cirque faces, then it is more sensi- tive to snow-avalanching, hence reducing the increase rate of ELA. 6. Discussion 6.1. Stability of the Model The determination coefficients and the residuals standard errors highlight a sufficient accuracy of the model: this latter appears to be better than previous studies where ELA accuracy was about 50 to 100 meters [14,24]. The results, synthesized as raster maps, can be easily com- bined to provide further spatial analysis (evidence of gradients, regionalization, etc.) or more generally to pro- vide a diachronic survey. However, maps show that ex- treme values are always estimated at the boundary of the study area, and at lower altitudes, in the deepest part of
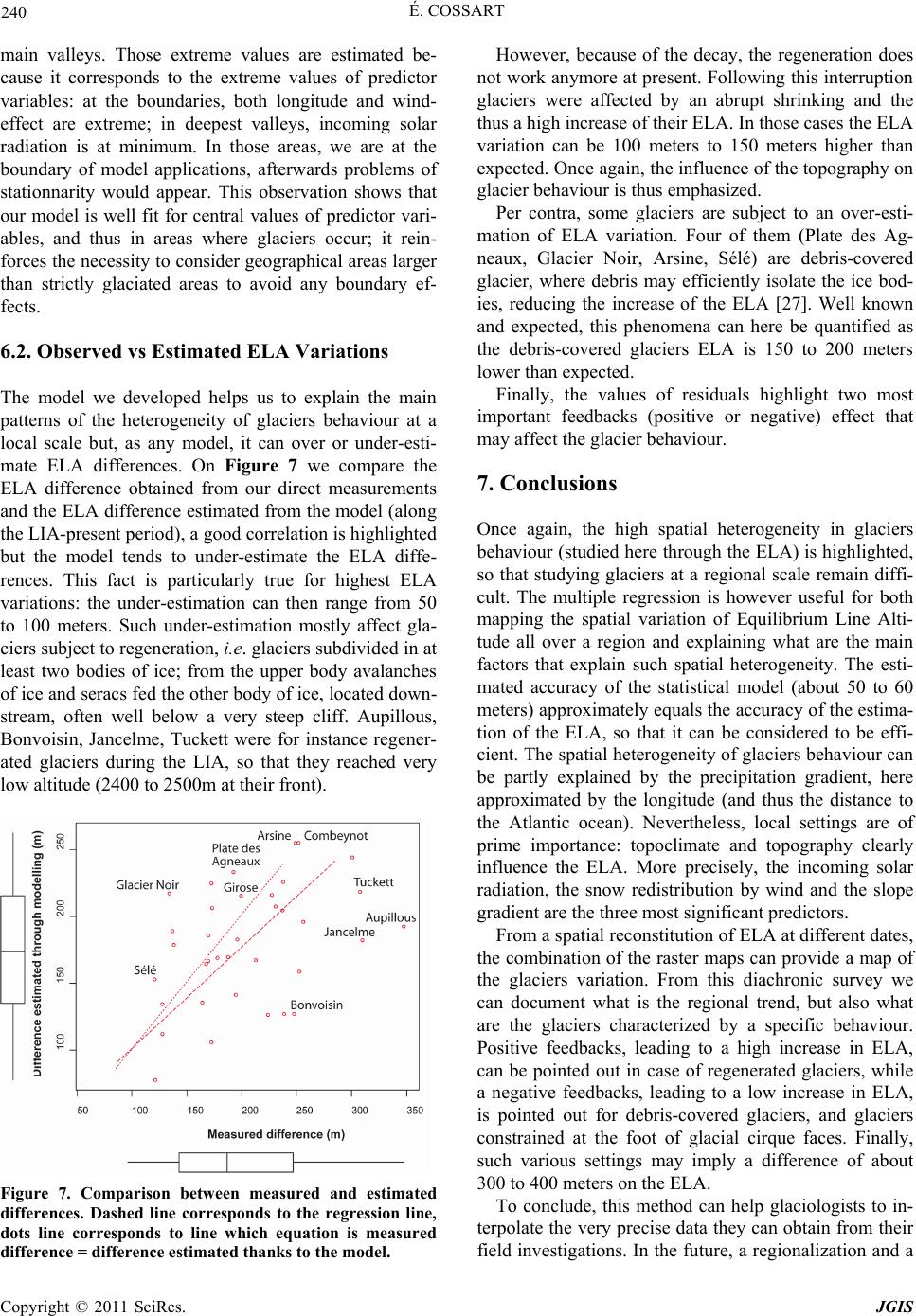 240 É. COSSART main valleys. Those extreme values are estimated be- cause it corresponds to the extreme values of predictor variables: at the boundaries, both longitude and wind- effect are extreme; in deepest valleys, incoming solar radiation is at minimum. In those areas, we are at the boundary of model applications, afterwards problems of stationnarity would appear. This observation shows that our model is well fit for central values of predictor vari- ables, and thus in areas where glaciers occur; it rein- forces the necessity to consider geographical areas larger than strictly glaciated areas to avoid any boundary ef- fects. 6.2. Observed vs Estimated ELA Variations The model we developed helps us to explain the main patterns of the heterogeneity of glaciers behaviour at a local scale but, as any model, it can over or under-esti- mate ELA differences. On Figure 7 we compare the ELA difference obtained from our direct measurements and the ELA difference estimated from the model (along the LIA-present per iod) , a good co rr elation is h igh ligh ted but the model tends to under-estimate the ELA diffe- rences. This fact is particularly true for highest ELA variations: the under-estimation can then range from 50 to 100 meters. Such under-estimation mostly affect gla- ciers subject to regeneration, i.e. glaciers subdivided in at least two bodies of ice; from the upper body avalanches of ice and seracs fed the other body of ice, located down- stream, often well below a very steep cliff. Aupillous, Bonvoisin, Jancelme, Tuckett were for instance regener- ated glaciers during the LIA, so that they reached very low altitude (2400 to 2500m at their front). Figure 7. Comparison between measured and estimated differences. Dashed line corresponds to the regression line, dots line corresponds to line which equation is measured difference = difference estimated thanks to the model. However, because of the decay, the regeneration does not work anymore at present. Following this interruption glaciers were affected by an abrupt shrinking and the thus a hig h in cr e as e of their ELA. In those cases th e EL A variation can be 100 meters to 150 meters higher than expected. Once again, the influence of the topogr aphy on glacier behaviour is thus emphasized. Per contra, some glaciers are subject to an over-esti- mation of ELA variation. Four of them (Plate des Ag- neaux, Glacier Noir, Arsine, Sélé) are debris-covered glacier, where debris may efficiently isolate the ice bod- ies, reducing the increase of the ELA [27]. Well known and expected, this phenomena can here be quantified as the debris-covered glaciers ELA is 150 to 200 meters lower than expected. Finally, the values of residuals highlight two most important feedbacks (positive or negative) effect that may affect the glacier behaviour. 7. Conclusions Once again, the high spatial heterogeneity in glaciers behaviour (studied here through the ELA) is highlighted, so that studying glaciers at a regional scale remain diffi- cult. The multiple regression is however useful for both mapping the spatial variation of Equilibrium Line Alti- tude all over a region and explaining what are the main factors that explain such spatial heterogeneity. The esti- mated accuracy of the statistical model (about 50 to 60 meters) approximately equals the accuracy of the estima- tion of the ELA, so that it can be considered to be effi- cient. The spatial heterogeneity of glaciers behaviour can be partly explained by the precipitation gradient, here approximated by the longitude (and thus the distance to the Atlantic ocean). Nevertheless, local settings are of prime importance: topoclimate and topography clearly influence the ELA. More precisely, the incoming solar radiation, the snow redistribution by wind and the slope gradient are the three most significant predictors. From a spatial reconstitution of ELA at differen t dates, the combination of the raster maps can provide a map of the glaciers variation. From this diachronic survey we can document what is the regional trend, but also what are the glaciers characterized by a specific behaviour. Positive feedbacks, leading to a high increase in ELA, can be pointed out in case of regenerated glaciers, while a negative feedbacks, leading to a low increase in ELA, is pointed out for debris-covered glaciers, and glaciers constrained at the foot of glacial cirque faces. Finally, such various settings may imply a difference of about 300 to 400 meters on the ELA. To conclude, this method can help glaciologists to in- terpolate the very precise d ata they can obtain from their field investigations. In the futu re, a regionalization and a Copyright © 2011 SciRes. JGIS
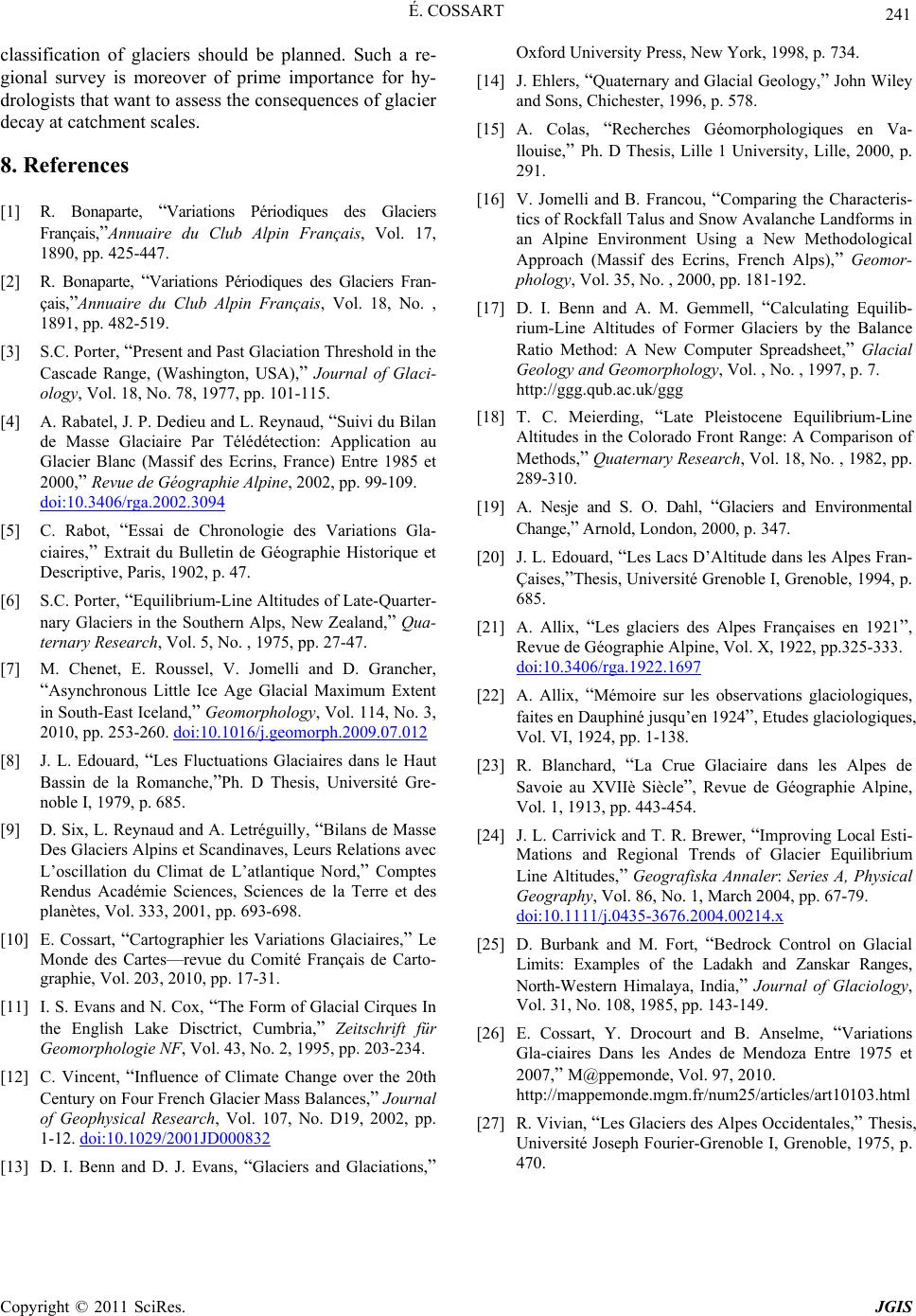 É. COSSART Copyright © 2011 SciRes. JGIS 241 classification of glaciers should be planned. Such a re- gional survey is moreover of prime importance for hy- drologists that want to assess the consequences of glacier decay at catchment scales. 8. References [1] R. Bonaparte, “Variations Périodiques des Glaciers Français,”Annuaire du Club Alpin Français, Vol. 17, 1890, pp. 425-447. [2] R. Bonaparte, “Variations Périodiques des Glaciers Fran- çais,”Annuaire du Club Alpin Français, Vol. 18, No. , 1891, pp. 482-519. [3] S.C. Porter, “Present and Past Glaciation Threshold in the Cascade Range, (Washington, USA),” Journal of Glaci- ology, Vol. 18, No. 78, 1977, pp. 101-115. [4] A. Rabatel, J. P. Dedieu and L. Reynaud, “Suivi du Bilan de Masse Glaciaire Par Télédétection: Application au Glacier Blanc (Massif des Ecrins, France) Entre 1985 et 2000,” Revue de Géographie Alpine, 2002, pp. 99-109. doi:10.3406/rga.2002.3094 [5] C. Rabot, “Essai de Chronologie des Variations Gla- ciaires,” Extrait du Bulletin de Géographie Historique et Descriptive, Paris, 1902, p. 47. [6] S.C. Porter, “Equilibrium-Line Altitudes of Late-Quarter- nary Glaciers in the Southern Alps, New Zealand,” Qua- ternary Research, Vol. 5, No. , 1975, pp. 27-47. [7] M. Chenet, E. Roussel, V. Jomelli and D. Grancher, “Asynchronous Little Ice Age Glacial Maximum Extent in South-East Iceland,” Geomorphology, Vol. 114, No. 3, 2010, pp. 253-260. doi:10.1016/j.geomorph.2009.07.012 [8] J. L. Edouard, “Les Fluctuations Glaciaires dans le Haut Bassin de la Romanche,”Ph. D Thesis, Université Gre- noble I, 1979, p. 685. [9] D. Six, L. Reynaud and A. Letréguilly, “Bilans de Masse Des Glaciers Alpins et Scandinaves, Leurs Relations avec L’oscillation du Climat de L’atlantique Nord,” Comptes Rendus Académie Sciences, Sciences de la Terre et des planètes, Vol. 333, 2001, pp. 693-698. [10] E. Cossart, “Cartographier les Variations Glaciaires,” Le Monde des Cartes—revue du Comité Français de Carto- graphie, Vol. 203, 2010, pp. 17-31. [11] I. S. Evans and N. Cox, “The Form of Glacial Cirques In the English Lake Disctrict, Cumbria,” Zeitschrift für Geomorphologie NF, Vol. 43, No. 2, 1995, pp. 203-234. [12] C. Vincent, “Influence of Climate Change over the 20th Century on Four French Glacier Mass Bala nces, ” Journal of Geophysical Research, Vol. 107, No. D19, 2002, pp. 1-12. doi:10.1029/2001JD000832 [13] D. I. Benn and D. J. Evans, “Glaciers and Glaciations,” Oxford University Press, New York, 1998, p. 734. [14] J. Ehlers, “Quaternary and Glacial Geology,” John Wiley and Sons, Chichester, 1996, p. 578. [15] A. Colas, “Recherches Géomorphologiques en Va- llouise,” Ph. D Thesis, Lille 1 University, Lille, 2000, p. 291. [16] V. Jomelli and B. Francou, “Comparing the Characteris- tics of Rockfall Talus and Snow Avalanche Landforms in an Alpine Environment Using a New Methodological Approach (Massif des Ecrins, French Alps),” Geomor- phology, Vol. 35, No. , 2000, pp. 181-192. [17] D. I. Benn and A. M. Gemmell, “Calculating Equilib- rium-Line Altitudes of Former Glaciers by the Balance Ratio Method: A New Computer Spreadsheet,” Glacial Geology and Geomorphology, Vol. , No. , 1997, p. 7. http://ggg.qub.ac.uk/ggg [18] T. C. Meierding, “Late Pleistocene Equilibrium-Line Altitudes in the Colorado Front Range: A Comparison of Methods,” Quaternary Research, Vol. 18, No. , 1982, pp. 289-310. [19] A. Nesje and S. O. Dahl, “Glaciers and Environmental Change,” Arnold, London, 2000, p. 347. [20] J. L. Edouard, “Les Lacs D’Altitude dans les Al pes Fran- Çaises,”Thesis, Université Grenoble I, Grenoble, 1994, p. 685. [21] A. Allix, “Les glaciers des Alpes Françaises en 1921”, Revue de Géographie Alpine, Vol. X, 1922, pp.325-333. doi:10.3406/rga.1922.1697 [22] A. Allix, “Mémoire sur les observations glaciologiques, faites en Dauphiné jusqu’en 1924”, Etudes glaciologiques, Vol. VI, 1924, pp. 1-138. [23] R. Blanchard, “La Crue Glaciaire dans les Alpes de Savoie au XVIIè Siècle”, Revue de Géographie Alpine, Vol. 1, 1913, pp. 443-454. [24] J. L. Carrivick and T. R. Brewer, “Improving Local Esti- Mations and Regional Trends of Glacier Equilibrium Line Altitudes,” Geografiska Annaler: Series A, Physical Geography, Vol. 86, No. 1, March 2004, pp. 67-79. doi:10.1111/j.0435-3676.2004.00214.x [25] D. Burbank and M. Fort, “Bedrock Control on Glacial Limits: Examples of the Ladakh and Zanskar Ranges, North-Western Himalaya, India,” Journal of Glaciology, Vol. 31, No. 108, 1985, pp. 143-149. [26] E. Cossart, Y. Drocourt and B. Anselme, “Variations Gla-ciaires Dans les Andes de Mendoza Entre 1975 et 2007,” M@ppemonde, Vol. 97, 2010. http://mappemonde.mgm.fr/num25/articles/art10103.html [27] R. Vivian, “Les Glaciers des Alpes Occidentales,” Thesis, Université Joseph Fourier-Grenoble I, Grenoble, 1975, p. 470.
|