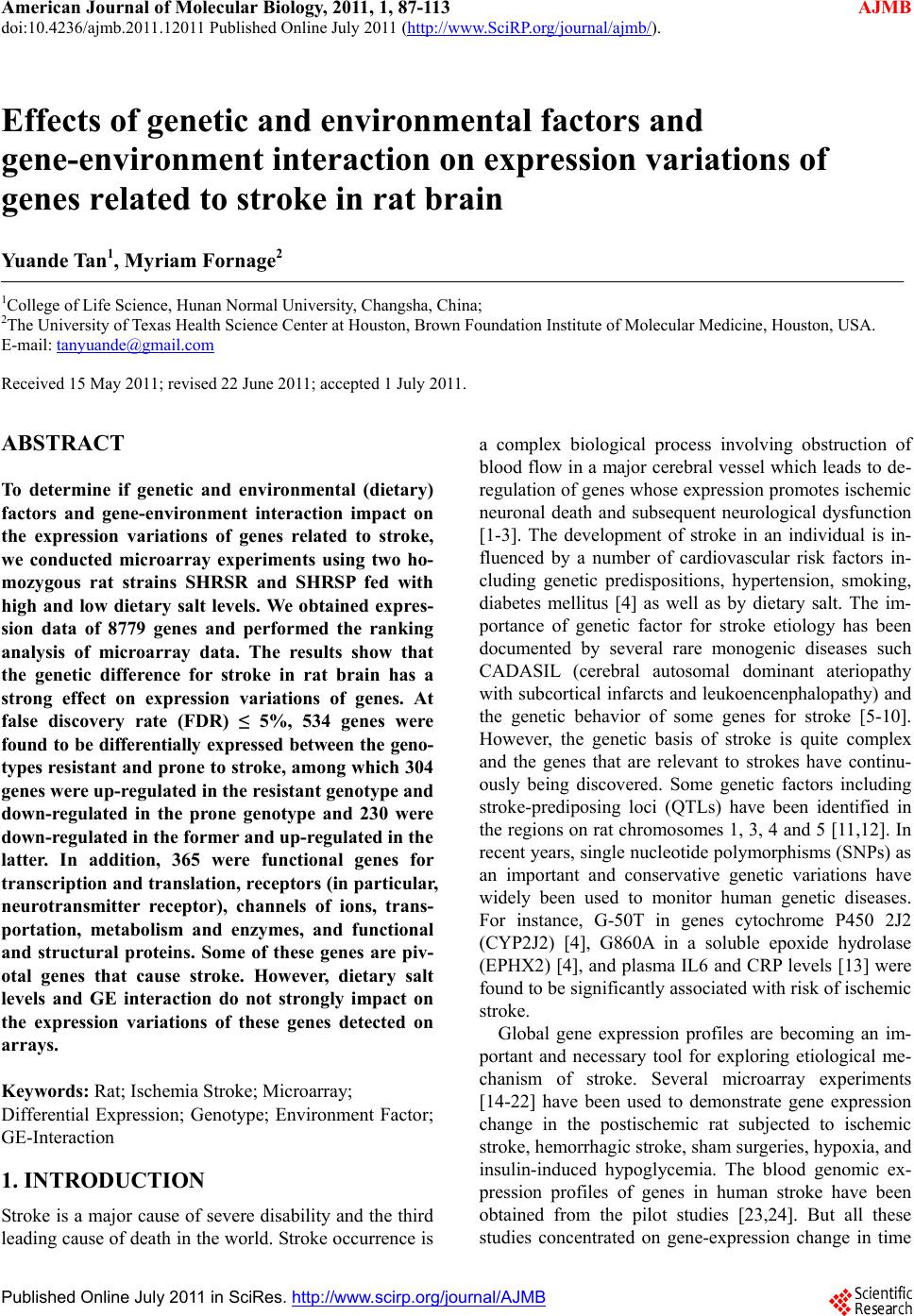 American Journal of Molecular Biology, 2011, 1, 87-113 AJMB doi:10.4236/ajmb.2011.12011 Published Online July 2011 (http://www.SciRP.org/journal/ajmb/). Published Online July 2011 in SciRes. http://www.scirp.org/journal/AJMB Effects of genetic and environmental factors and gene-environment interaction on expression variations of genes related to stroke in rat brain Yuande Tan1, Myriam Fornage2 1College of Life Science, Hunan Normal University, Changsha, China; 2The University of Texas Health Science Center at Houston, Brown Foundation Institute of Molecular Medicine, Houston, USA. E-mail: tanyuande@gmail.com Received 15 May 2011; revised 22 June 2011; accepted 1 July 2011. ABSTRACT To determine if genetic and environmental (dietary) factors and gene-environment interaction impact on the expression variations of genes related to stroke, we conducted microarray experiments using two ho- mozygous rat strains SHRSR and SHRSP fed with high and low dietary salt levels. We obtained expres- sion data of 8779 genes and performed the ranking analysis of microarray data. The results show that the genetic difference for stroke in rat brain has a strong effect on expression variations of genes. At false discovery rate (FDR) ≤ 5%, 534 genes were found to be differentially expressed between the geno- types resistant and prone to stroke, among which 304 genes were up-regulated in the resistant genotype and down-regulated in the prone genotype and 230 were down-regulated in the former and up-regulated in the latter. In addition, 365 were functional genes for transcription and translation, receptors (in particular, neurotransmitter receptor), channels of ions, trans- portation, metabolism and enzymes, and functional and structural proteins. Some of these genes are piv- otal genes that cause stroke. However, dietary salt levels and GE interaction do not strongly impact on the expression variations of these genes detected on arrays. Keywords: Rat; Ischemia Stroke; Microarray; Differential Expression; Genotype; Environment Factor; GE-Interaction 1. INTRODUCTION Stroke is a major cause of severe disability and the third leading cause of death in the world. Stroke occurrence is a complex biological process involving obstruction of blood flow in a major cerebral vessel which leads to de- regulation of genes whose expression promotes ischemic neuronal death and subsequent neurological dysfunction [1-3]. The development of stroke in an individual is in- fluenced by a number of cardiovascular risk factors in- cluding genetic predispositions, hypertension, smoking, diabetes mellitus [4] as well as by dietary salt. The im- portance of genetic factor for stroke etiology has been documented by several rare monogenic diseases such CADASIL (cerebral autosomal dominant ateriopathy with subcortical infarcts and leukoencenphalopathy) and the genetic behavior of some genes for stroke [5-10]. However, the genetic basis of stroke is quite complex and the genes that are relevant to strokes have continu- ously being discovered. Some genetic factors including stroke-prediposing loci (QTLs) have been identified in the regions on rat chromosomes 1, 3, 4 and 5 [11,12]. In recent years, single nucleotide polymorphisms (SNPs) as an important and conservative genetic variations have widely been used to monitor human genetic diseases. For instance, G-50T in genes cytochrome P450 2J2 (CYP2J2) [4], G860A in a soluble epoxide hydrolase (EPHX2) [4], and plasma IL6 and CRP levels [13] were found to be significantly associated with risk of ischemic stroke. Global gene expression profiles are becoming an im- portant and necessary tool for exploring etiological me- chanism of stroke. Several microarray experiments [14-22] have been used to demonstrate gene expression change in the postischemic rat subjected to ischemic stroke, hemorrhagic stroke, sham surgeries, hypoxia, and insulin-induced hypoglycemia. The blood genomic ex- pression profiles of genes in human stroke have been obtained from the pilot studies [23,24]. But all these studies concentrated on gene-expression change in time
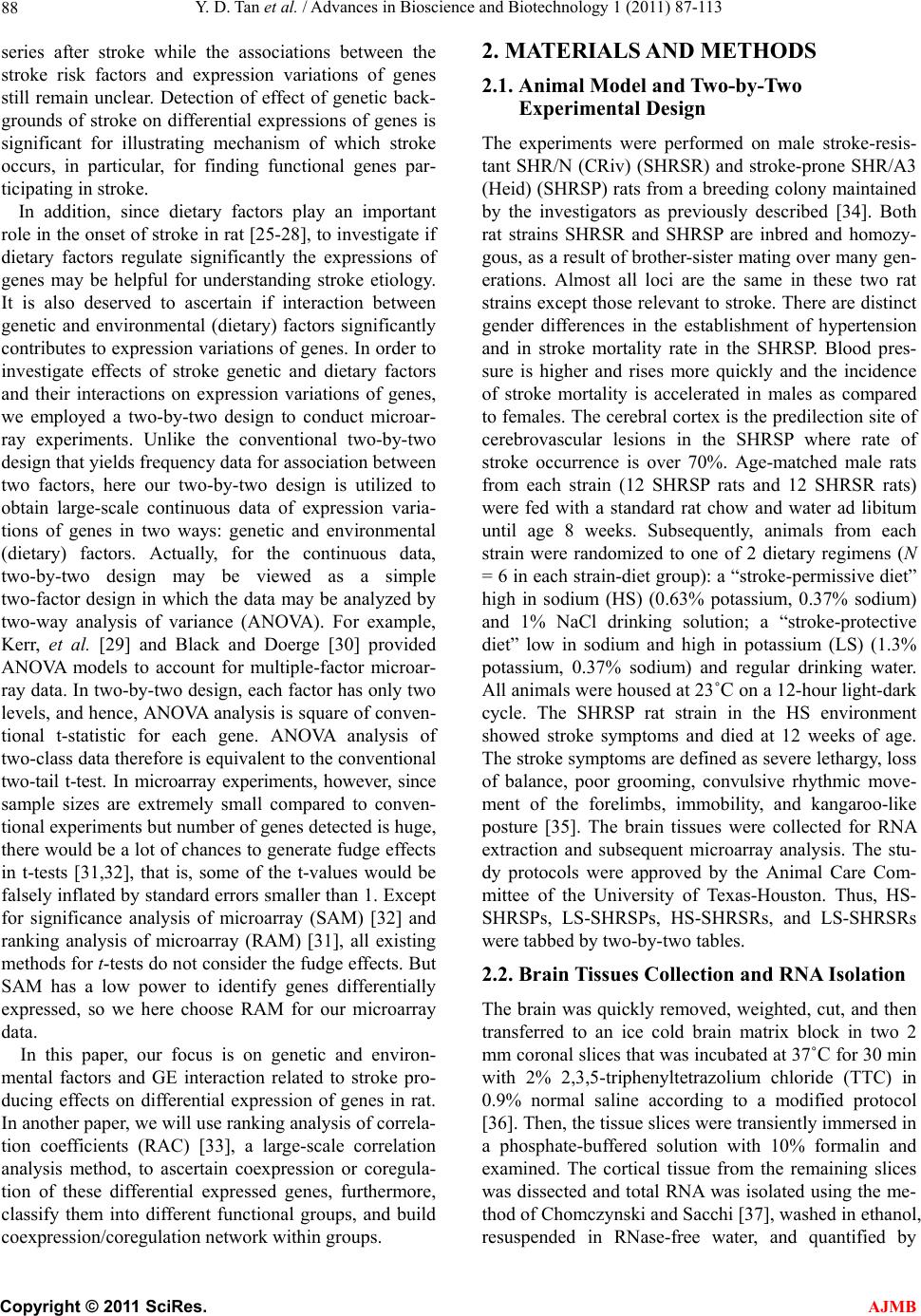 Y. D. Tan et al. / Advances in Bioscience and Biotechnology 1 (2011) 87-113 Copyright © 2011 SciRes. AJMB 88 series after stroke while the associations between the stroke risk factors and expression variations of genes still remain unclear. Detection of effect of genetic back- grounds of stroke on differential expressions of genes is significant for illustrating mechanism of which stroke occurs, in particular, for finding functional genes par- ticipating in stroke. In addition, since dietary factors play an important role in the onset of stroke in rat [25-28], to investigate if dietary factors regulate significantly the expressions of genes may be helpful for understanding stroke etiology. It is also deserved to ascertain if interaction between genetic and environmental (dietary) factors significantly contributes to expression variations of genes. In order to investigate effects of stroke genetic and dietary factors and their interactions on expression variations of genes, we employed a two-by-two design to conduct microar- ray experiments. Unlike the conventional two-by-two design that yields frequency data for association between two factors, here our two-by-two design is utilized to obtain large-scale continuous data of expression varia- tions of genes in two ways: genetic and environmental (dietary) factors. Actually, for the continuous data, two-by-two design may be viewed as a simple two-factor design in which the data may be analyzed by two-way analysis of variance (ANOVA). For example, Kerr, et al. [29] and Black and Doerge [30] provided ANOVA models to account for multiple-factor microar- ray data. In two-by-two design, each factor has only two levels, and hence, ANOVA analysis is square of conven- tional t-statistic for each gene. ANOVA analysis of two-class data therefore is equivalent to the conventional two-tail t-test. In microarray experiments, however, since sample sizes are extremely small compared to conven- tional experiments but number of genes detected is huge, there would be a lot of chances to generate fudge effects in t-tests [31,32], that is, some of the t-values would be falsely inflated by standard errors smaller than 1. Except for significance analysis of microarray (SAM) [32] and ranking analysis of microarray (RAM) [31], all existing methods for t-tests do not consider the fudge effects. But SAM has a low power to identify genes differentially expressed, so we here choose RAM for our microarray data. In this paper, our focus is on genetic and environ- mental factors and GE interaction related to stroke pro- ducing effects on differential expression of genes in rat. In another paper, we will use ranking analysis of correla- tion coefficients (RAC) [33], a large-scale correlation analysis method, to ascertain coexpression or coregula- tion of these differential expressed genes, furthermore, classify them into different functional groups, and build coexpression/coregulation network within groups. 2. MATERIALS AND METHODS 2.1. Animal Model and Two-by-Two Experimental Design The experiments were performed on male stroke-resis- tant SHR/N (CRiv) (SHRSR) and stroke-prone SHR/A3 (Heid) (SHRSP) rats from a breeding colony maintained by the investigators as previously described [34]. Both rat strains SHRSR and SHRSP are inbred and homozy- gous, as a result of brother-sister mating over many gen- erations. Almost all loci are the same in these two rat strains except those relevant to stroke. There are distinct gender differences in the establishment of hypertension and in stroke mortality rate in the SHRSP. Blood pres- sure is higher and rises more quickly and the incidence of stroke mortality is accelerated in males as compared to females. The cerebral cortex is the predilection site of cerebrovascular lesions in the SHRSP where rate of stroke occurrence is over 70%. Age-matched male rats from each strain (12 SHRSP rats and 12 SHRSR rats) were fed with a standard rat chow and water ad libitum until age 8 weeks. Subsequently, animals from each strain were randomized to one of 2 dietary regimens (N = 6 in each strain-diet group): a “stroke-permissive diet” high in sodium (HS) (0.63% potassium, 0.37% sodium) and 1% NaCl drinking solution; a “stroke-protective diet” low in sodium and high in potassium (LS) (1.3% potassium, 0.37% sodium) and regular drinking water. All animals were housed at 23˚C on a 12-hour light-dark cycle. The SHRSP rat strain in the HS environment showed stroke symptoms and died at 12 weeks of age. The stroke symptoms are defined as severe lethargy, loss of balance, poor grooming, convulsive rhythmic move- ment of the forelimbs, immobility, and kangaroo-like posture [35]. The brain tissues were collected for RNA extraction and subsequent microarray analysis. The stu- dy protocols were approved by the Animal Care Com- mittee of the University of Texas-Houston. Thus, HS- SHRSPs, LS-SHRSPs, HS-SHRSRs, and LS-SHRSRs were tabbed by two-by-two tables. 2.2. Brain Tissues Collection and RNA Isolation The brain was quickly removed, weighted, cut, and then transferred to an ice cold brain matrix block in two 2 mm coronal slices that was incubated at 37˚C for 30 min with 2% 2,3,5-triphenyltetrazolium chloride (TTC) in 0.9% normal saline according to a modified protocol [36]. Then, the tissue slices were transiently immersed in a phosphate-buffered solution with 10% formalin and examined. The cortical tissue from the remaining slices was dissected and total RNA was isolated using the me- thod of Chomczynski and Sacchi [37], washed in ethanol, resuspended in RNase-free water, and quantified by
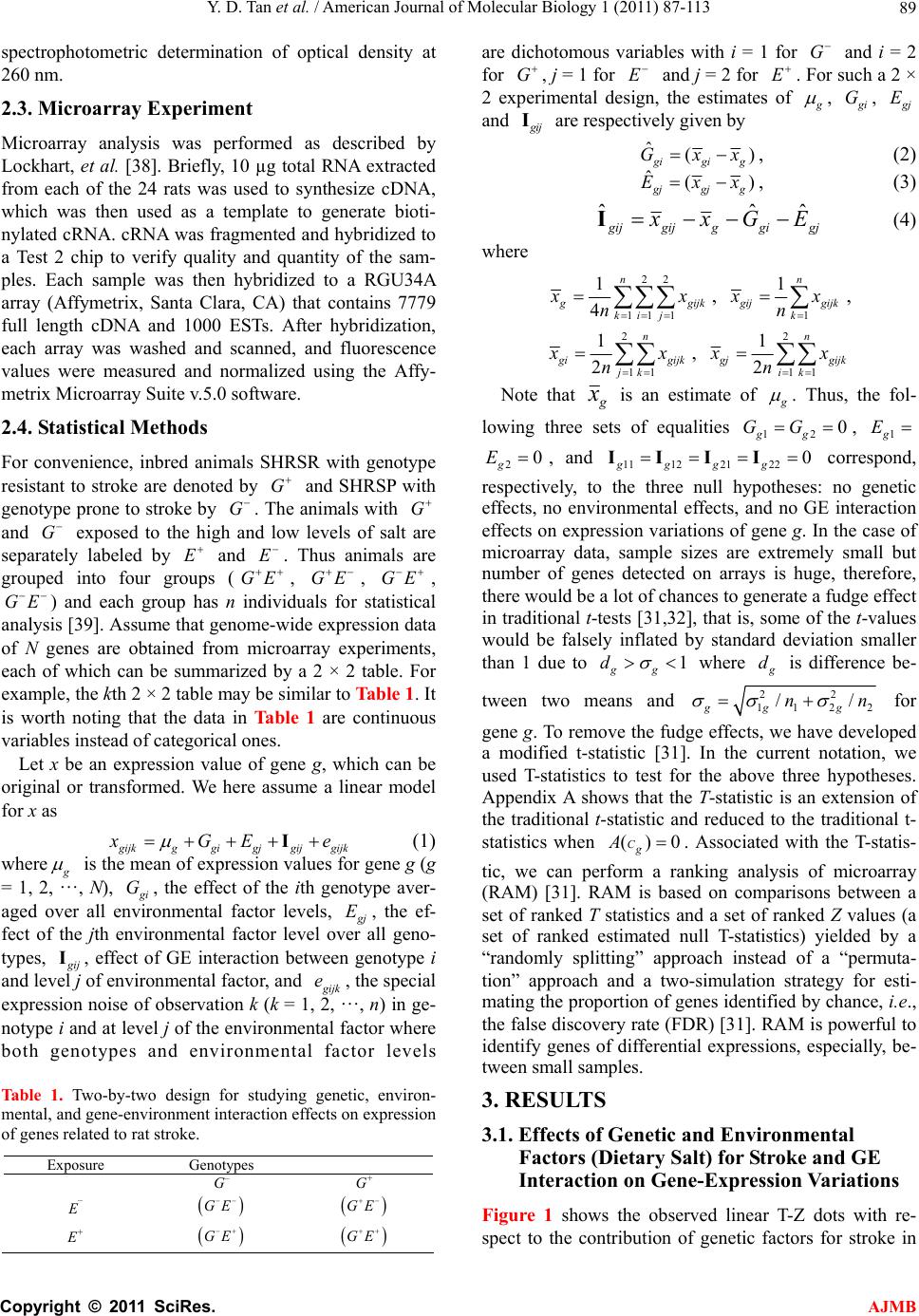 Y. D. Tan et al. / American Journal of Molecular Biology 1 (2011) 87-113 Copyright © 2011 SciRes. AJMB 89 spectrophotometric determination of optical density at 260 nm. 2.3. Microarray Experiment Microarray analysis was performed as described by Lockhart, et al. [38]. Briefly, 10 µg total RNA extracted from each of the 24 rats was used to synthesize cDNA, which was then used as a template to generate bioti- nylated cRNA. cRNA was fragmented and hybridized to a Test 2 chip to verify quality and quantity of the sam- ples. Each sample was then hybridized to a RGU34A array (Affymetrix, Santa Clara, CA) that contains 7779 full length cDNA and 1000 ESTs. After hybridization, each array was washed and scanned, and fluorescence values were measured and normalized using the Affy- metrix Microarray Suite v.5.0 software. 2.4. Statistical Methods For convenience, inbred animals SHRSR with genotype resistant to stroke are denoted by G and SHRSP with genotype prone to stroke by G. The animals with G and G exposed to the high and low levels of salt are separately labeled by E and E. Thus animals are grouped into four groups (GE , GE , GE , GE ) and each group has n individuals for statistical analysis [39]. Assume that genome-wide expression data of N genes are obtained from microarray experiments, each of which can be summarized by a 2 × 2 table. For example, the kth 2 × 2 table may be similar to Table 1. It is worth noting that the data in Table 1 are continuous variables instead of categorical ones. Let x be an expression value of gene g, which can be original or transformed. We here assume a linear model for x as ijkggigjgij gijk GE e I (1) where is the mean of expression values for gene g (g = 1, 2, …, N), i G, the effect of the ith genotype aver- aged over all environmental factor levels, j E, the ef- fect of the jth environmental factor level over all geno- types, ij I, effect of GE interaction between genotype i and level j of environmental factor, and ijk e, the special expression noise of observation k (k = 1, 2, …, n) in ge- notype i and at level j of the environmental factor where both genotypes and environmental factor levels Table 1. Two-by-two design for studying genetic, environ- mental, and gene-environment interaction effects on expression of genes related to rat stroke. Exposure Genotypes G – G+ E– GE GE E+ GE GE are dichotomous variables with i = 1 for G and i = 2 for G , j = 1 for E and j = 2 for E. For such a 2 × 2 experimental design, the estimates of , i G, j E and ij I are respectively given by ˆ() igig Gxx, (2) ˆ() jgjg Exx, (3) gjgiggijgij EGxx ˆ ˆ ˆI (4) where 22 11 1 1 4 n gijk ki j x n , 1 1n ij gijk k x n , 2 11 1 2 n i gijk jk x n , 2 11 1 2 n j gijk ik x n Note that g x is an estimate of . Thus, the fol- lowing three sets of equalities 12 0 gg GG, 1g E 20 g E , and 11122122 0 gg g g IIII correspond, respectively, to the three null hypotheses: no genetic effects, no environmental effects, and no GE interaction effects on expression variations of gene g. In the case of microarray data, sample sizes are extremely small but number of genes detected on arrays is huge, therefore, there would be a lot of chances to generate a fudge effect in traditional t-tests [31,32], that is, some of the t-values would be falsely inflated by standard deviation smaller than 1 due to 1 gg d where d is difference be- tween two means and 22 112 2 // gg g nn for gene g. To remove the fudge effects, we have developed a modified t-statistic [31]. In the current notation, we used T-statistics to test for the above three hypotheses. Appendix A shows that the T-statistic is an extension of the traditional t-statistic and reduced to the traditional t- statistics when ()0Cg A . Associated with the T-statis- tic, we can perform a ranking analysis of microarray (RAM) [31]. RAM is based on comparisons between a set of ranked T statistics and a set of ranked Z values (a set of ranked estimated null T-statistics) yielded by a “randomly splitting” approach instead of a “permuta- tion” approach and a two-simulation strategy for esti- mating the proportion of genes identified by chance, i.e., the false discovery rate (FDR) [31]. RAM is powerful to identify genes of differential expressions, especially, be- tween small samples. 3. RESULTS 3.1. Effects of Genetic and Environmental Factors (Dietary Salt) for Stroke and GE Interaction on Gene-Expression Variations Figure 1 shows the observed linear T-Z dots with re- spect to the contribution of genetic factors for stroke in
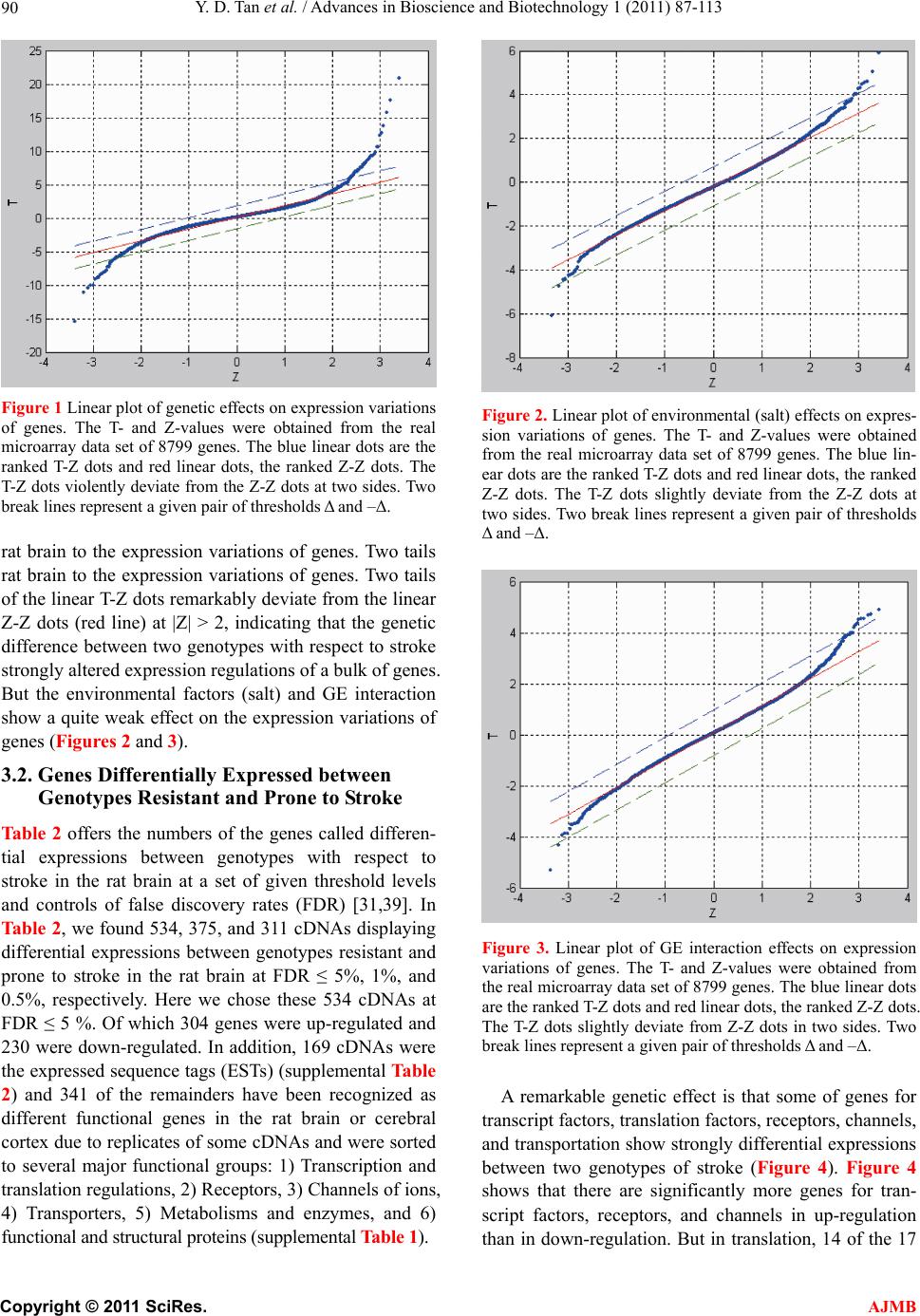 Y. D. Tan et al. / Advances in Bioscience and Biotechnology 1 (2011) 87-113 Copyright © 2011 SciRes. AJMB 90 Figure 1 Linear plot of genetic effects on expression variations of genes. The T- and Z-values were obtained from the real microarray data set of 8799 genes. The blue linear dots are the ranked T-Z dots and red linear dots, the ranked Z-Z dots. The T-Z dots violently deviate from the Z-Z dots at two sides. Two break lines represent a given pair of thresholds Δ and –Δ. rat brain to the expression variations of genes. Two tails rat brain to the expression variations of genes. Two tails of the linear T-Z dots remarkably deviate from the linear Z-Z dots (red line) at |Z| > 2, indicating that the genetic difference between two genotypes with respect to stroke strongly altered expression regulations of a bulk of genes. But the environmental factors (salt) and GE interaction show a quite weak effect on the expression variations of genes (Figures 2 and 3). 3.2. Genes Differentially Expressed between Genotypes Resistant and Prone to Stroke Table 2 offers the numbers of the genes called differen- tial expressions between genotypes with respect to stroke in the rat brain at a set of given threshold levels and controls of false discovery rates (FDR) [31,39]. In Table 2, we found 534, 375, and 311 cDNAs displaying differential expressions between genotypes resistant and prone to stroke in the rat brain at FDR ≤ 5%, 1%, and 0.5%, respectively. Here we chose these 534 cDNAs at FDR ≤ 5 %. Of which 304 genes were up-regulated and 230 were down-regulated. In addition, 169 cDNAs were the expressed sequence tags (ESTs) (supplemental Table 2) and 341 of the remainders have been recognized as different functional genes in the rat brain or cerebral cortex due to replicates of some cDNAs and were sorted to several major functional groups: 1) Transcription and translation regulations, 2) Receptors, 3) Channels of ions, 4) Transporters, 5) Metabolisms and enzymes, and 6) functional and structural proteins (supplemental Table 1). Figure 2. Linear plot of environmental (salt) effects on expres- sion variations of genes. The T- and Z-values were obtained from the real microarray data set of 8799 genes. The blue lin- ear dots are the ranked T-Z dots and red linear dots, the ranked Z-Z dots. The T-Z dots slightly deviate from the Z-Z dots at two sides. Two break lines represent a given pair of thresholds Δ and –Δ. Figure 3. Linear plot of GE interaction effects on expression variations of genes. The T- and Z-values were obtained from the real microarray data set of 8799 genes. The blue linear dots are the ranked T-Z dots and red linear dots, the ranked Z-Z dots. The T-Z dots slightly deviate from Z-Z dots in two sides. Two break lines represent a given pair of thresholds Δ and –Δ. A remarkable genetic effect is that some of genes for transcript factors, translation factors, receptors, channels, and transportation show strongly differential expressions between two genotypes of stroke (Figure 4). Figure 4 shows that there are significantly more genes for tran- script factors, receptors, and channels in up-regulation than in down-regulation. But in translation, 14 of the 17
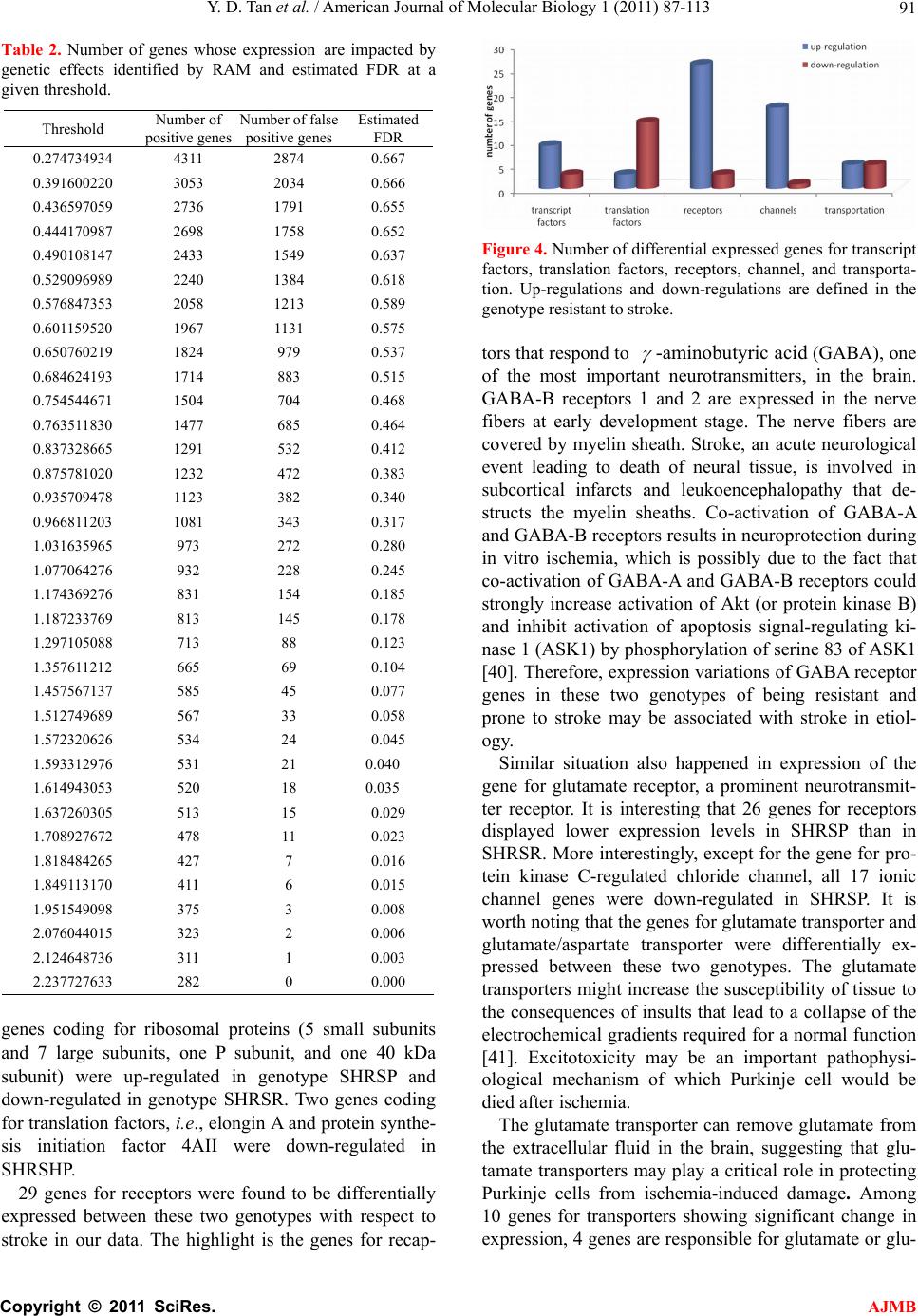 Y. D. Tan et al. / American Journal of Molecular Biology 1 (2011) 87-113 Copyright © 2011 SciRes. AJMB 91 Table 2. Number of genes whose expressionare impacted by genetic effects identified by RAM and estimated FDR at a given threshold. Threshold Number of positive genes Number of false positive genes Estimated FDR 0.274734934 4311 2874 0.667 0.391600220 3053 2034 0.666 0.436597059 2736 1791 0.655 0.444170987 2698 1758 0.652 0.490108147 2433 1549 0.637 0.529096989 2240 1384 0.618 0.576847353 2058 1213 0.589 0.601159520 1967 1131 0.575 0.650760219 1824 979 0.537 0.684624193 1714 883 0.515 0.754544671 1504 704 0.468 0.763511830 1477 685 0.464 0.837328665 1291 532 0.412 0.875781020 1232 472 0.383 0.935709478 1123 382 0.340 0.966811203 1081 343 0.317 1.031635965 973 272 0.280 1.077064276 932 228 0.245 1.174369276 831 154 0.185 1.187233769 813 145 0.178 1.297105088 713 88 0.123 1.357611212 665 69 0.104 1.457567137 585 45 0.077 1.512749689 567 33 0.058 1.572320626 534 24 0.045 1.593312976 531 21 0.040 1.614943053 520 18 0.035 1.637260305 513 15 0.029 1.708927672 478 11 0.023 1.818484265 427 7 0.016 1.849113170 411 6 0.015 1.951549098 375 3 0.008 2.076044015 323 2 0.006 2.124648736 311 1 0.003 2.237727633 282 0 0.000 genes coding for ribosomal proteins (5 small subunits and 7 large subunits, one P subunit, and one 40 kDa subunit) were up-regulated in genotype SHRSP and down-regulated in genotype SHRSR. Two genes coding for translation factors, i.e., elongin A and protein synthe- sis initiation factor 4AII were down-regulated in SHRSHP. 29 genes for receptors were found to be differentially expressed between these two genotypes with respect to stroke in our data. The highlight is the genes for recap- Figure 4. Number of differential expressed genes for transcript factors, translation factors, receptors, channel, and transporta- tion. Up-regulations and down-regulations are defined in the genotype resistant to stroke. tors that respond to -aminobutyric acid (GABA), one of the most important neurotransmitters, in the brain. GABA-B receptors 1 and 2 are expressed in the nerve fibers at early development stage. The nerve fibers are covered by myelin sheath. Stroke, an acute neurological event leading to death of neural tissue, is involved in subcortical infarcts and leukoencephalopathy that de- structs the myelin sheaths. Co-activation of GABA-A and GABA-B receptors results in neuroprotection during in vitro ischemia, which is possibly due to the fact that co-activation of GABA-A and GABA-B receptors could strongly increase activation of Akt (or protein kinase B) and inhibit activation of apoptosis signal-regulating ki- nase 1 (ASK1) by phosphorylation of serine 83 of ASK1 [40]. Therefore, expression variations of GABA receptor genes in these two genotypes of being resistant and prone to stroke may be associated with stroke in etiol- ogy. Similar situation also happened in expression of the gene for glutamate receptor, a prominent neurotransmit- ter receptor. It is interesting that 26 genes for receptors displayed lower expression levels in SHRSP than in SHRSR. More interestingly, except for the gene for pro- tein kinase C-regulated chloride channel, all 17 ionic channel genes were down-regulated in SHRSP. It is worth noting that the genes for glutamate transporter and glutamate/aspartate transporter were differentially ex- pressed between these two genotypes. The glutamate transporters might increase the susceptibility of tissue to the consequences of insults that lead to a collapse of the electrochemical gradients required for a normal function [41]. Excitotoxicity may be an important pathophysi- ological mechanism of which Purkinje cell would be died after ischemia. The glutamate transporter can remove glutamate from the extracellular fluid in the brain, suggesting that glu- tamate transporters may play a critical role in protecting Purkinje cells from ischemia-induced damage. Among 10 genes for transporters showing significant change in expression, 4 genes are responsible for glutamate or glu-
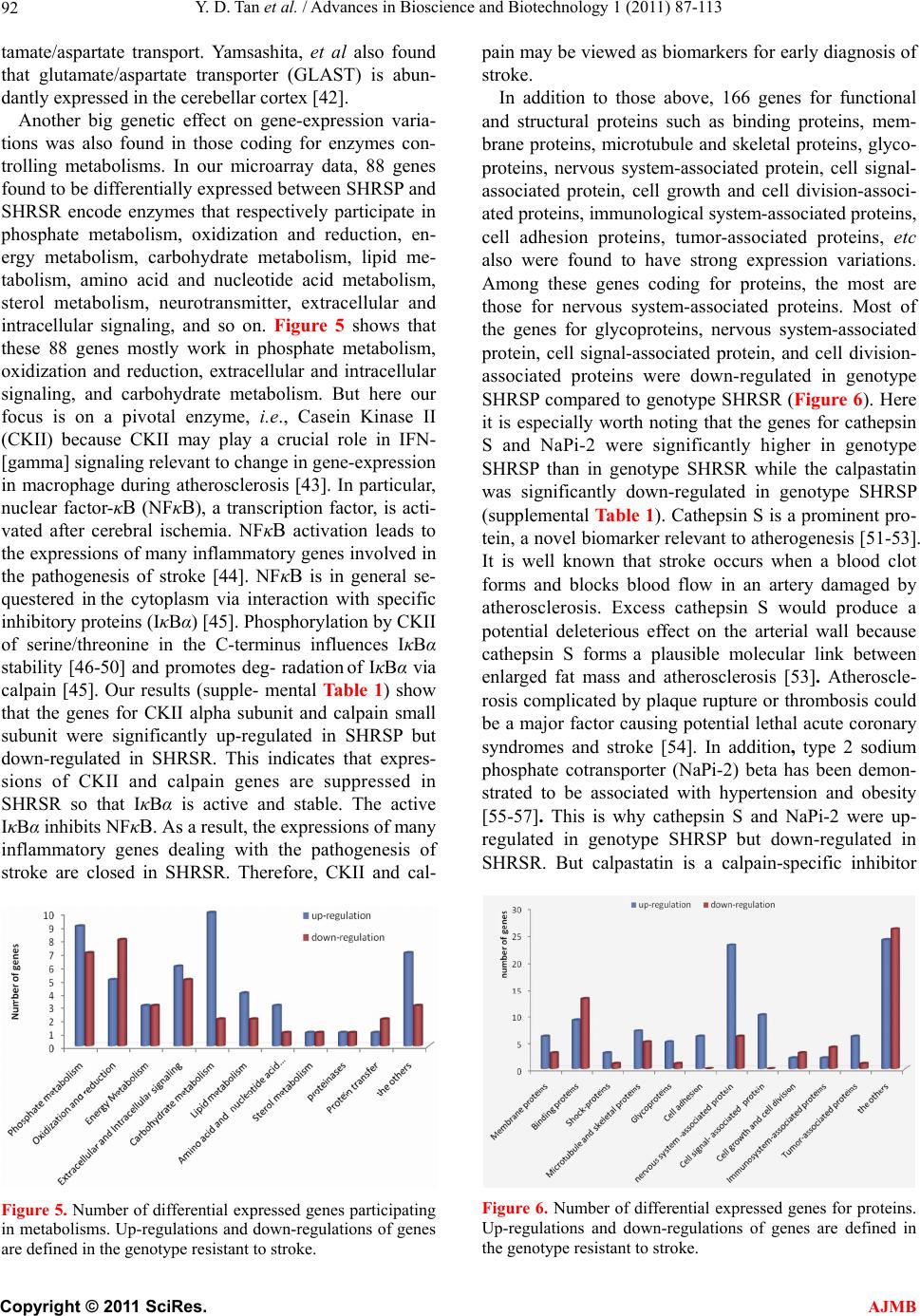 Y. D. Tan et al. / Advances in Bioscience and Biotechnology 1 (2011) 87-113 Copyright © 2011 SciRes. AJMB 92 tamate/aspartate transport. Yamsashita, et al also found that glutamate/aspartate transporter (GLAST) is abun- dantly expressed in the cerebellar cortex [42]. Another big genetic effect on gene-expression varia- tions was also found in those coding for enzymes con- trolling metabolisms. In our microarray data, 88 genes found to be differentially expressed between SHRSP and SHRSR encode enzymes that respectively participate in phosphate metabolism, oxidization and reduction, en- ergy metabolism, carbohydrate metabolism, lipid me- tabolism, amino acid and nucleotide acid metabolism, sterol metabolism, neurotransmitter, extracellular and intracellular signaling, and so on. Figure 5 shows that these 88 genes mostly work in phosphate metabolism, oxidization and reduction, extracellular and intracellular signaling, and carbohydrate metabolism. But here our focus is on a pivotal enzyme, i.e., Casein Kinase II (CKII) because CKII may play a crucial role in IFN- [gamma] signaling relevant to change in gene-expression in macrophage during atherosclerosis [43]. In particular, nuclear factor-κB (NFκB), a transcription factor, is acti- vated after cerebral ischemia. NFκB activation leads to the expressions of many inflammatory genes involved in the pathogenesis of stroke [44]. NFκB is in general se- questered in the cytoplasm via interaction with specific inhibitory proteins (IκBα) [45]. Phosphorylation by CKII of serine/threonine in the C-terminus influences IκBα stability [46-50] and promotes deg- radation of IκBα via calpain [45]. Our results (supple- mental Table 1) show that the genes for CKII alpha subunit and calpain small subunit were significantly up-regulated in SHRSP but down-regulated in SHRSR. This indicates that expres- sions of CKII and calpain genes are suppressed in SHRSR so that IκBα is active and stable. The active IκBα inhibits NFκB. As a result, the expressions of many inflammatory genes dealing with the pathogenesis of stroke are closed in SHRSR. Therefore, CKII and cal- Figure 5. Number of differential expressed genes participating in metabolisms. Up-regulations and down-regulations of genes are defined in the genotype resistant to stroke. pain may be viewed as biomarkers for early diagnosis of stroke. In addition to those above, 166 genes for functional and structural proteins such as binding proteins, mem- brane proteins, microtubule and skeletal proteins, glyco- proteins, nervous system-associated protein, cell signal- associated protein, cell growth and cell division-associ- ated proteins, immunological system-associated proteins, cell adhesion proteins, tumor-associated proteins, etc also were found to have strong expression variations. Among these genes coding for proteins, the most are those for nervous system-associated proteins. Most of the genes for glycoproteins, nervous system-associated protein, cell signal-associated protein, and cell division- associated proteins were down-regulated in genotype SHRSP compared to genotype SHRSR (Figure 6). Here it is especially worth noting that the genes for cathepsin S and NaPi-2 were significantly higher in genotype SHRSP than in genotype SHRSR while the calpastatin was significantly down-regulated in genotype SHRSP (supplemental Table 1). Cathepsin S is a prominent pro- tein, a novel biomarker relevant to atherogenesis [51-53]. It is well known that stroke occurs when a blood clot forms and blocks blood flow in an artery damaged by atherosclerosis. Excess cathepsin S would produce a potential deleterious effect on the arterial wall because cathepsin S forms a plausible molecular link between enlarged fat mass and atherosclerosis [53]. Atheroscle- rosis complicated by plaque rupture or thrombosis could be a major factor causing potential lethal acute coronary syndromes and stroke [54]. In addition, type 2 sodium phosphate cotransporter (NaPi-2) beta has been demon- strated to be associated with hypertension and obesity [55-57]. This is why cathepsin S and NaPi-2 were up- regulated in genotype SHRSP but down-regulated in SHRSR. But calpastatin is a calpain-specific inhibitor Figure 6. Number of differential expressed genes for proteins. Up-regulations and down-regulations of genes are defined in the genotype resistant to stroke.
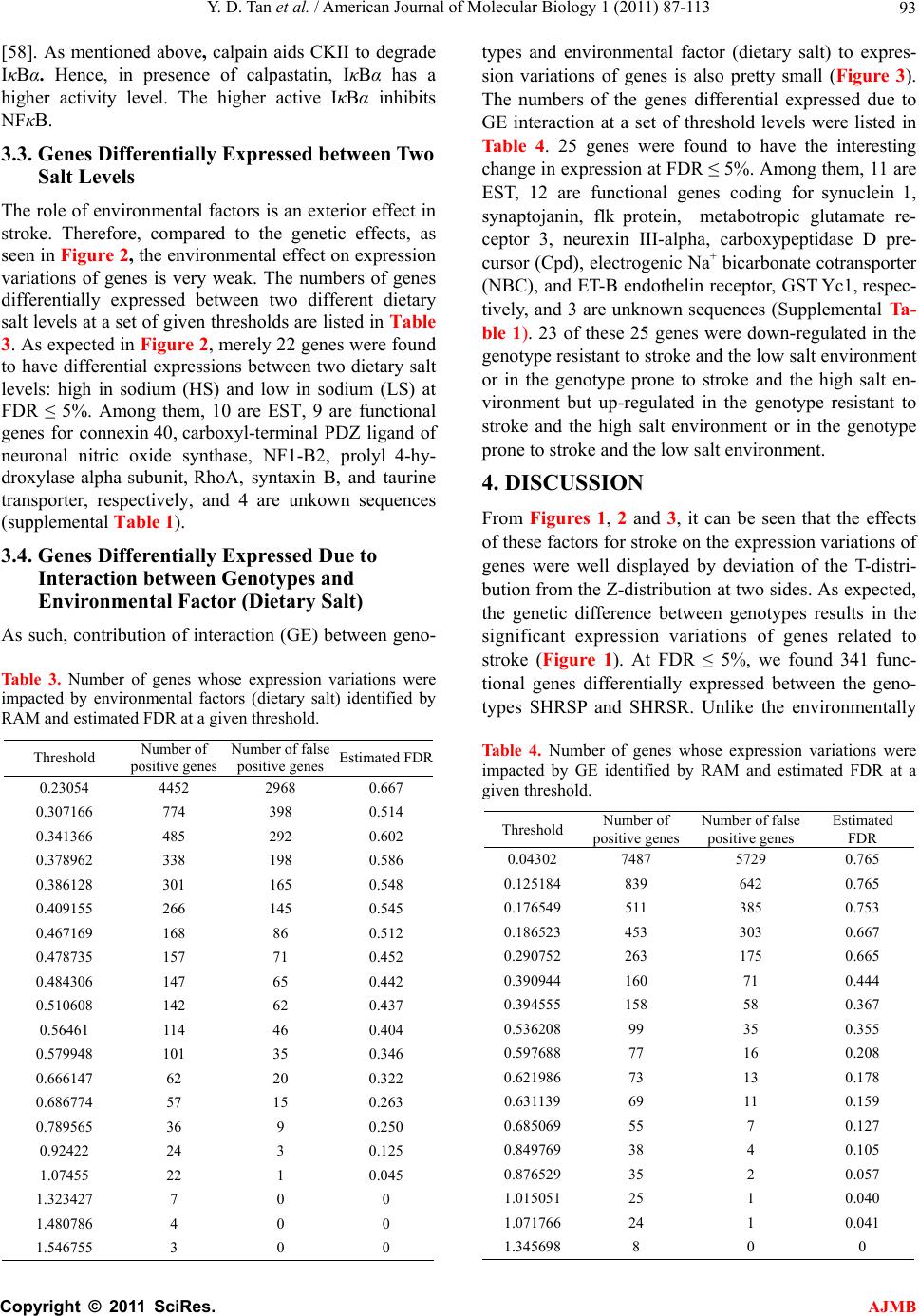 Y. D. Tan et al. / American Journal of Molecular Biology 1 (2011) 87-113 Copyright © 2011 SciRes. AJMB 93 [58]. As mentioned above, calpain aids CKII to degrade IκBα. Hence, in presence of calpastatin, IκBα has a higher activity level. The higher active IκBα inhibits NFκB. 3.3. Genes Differentially Expressed between Two Salt Levels The role of environmental factors is an exterior effect in stroke. Therefore, compared to the genetic effects, as seen in Figure 2, the environmental effect on expression variations of genes is very weak. The numbers of genes differentially expressed between two different dietary salt levels at a set of given thresholds are listed in Table 3. As expected in Figure 2, merely 22 genes were found to have differential expressions between two dietary salt levels: high in sodium (HS) and low in sodium (LS) at FDR ≤ 5%. Among them, 10 are EST, 9 are functional genes for connexin 40, carboxyl-terminal PDZ ligand of neuronal nitric oxide synthase, NF1-B2, prolyl 4-hy- droxylase alpha subunit, RhoA, syntaxin B, and taurine transporter, respectively, and 4 are unkown sequences (supplemental Table 1). 3.4. Genes Differentially Expressed Due to Interaction between Genotypes and Environmental Factor (Dietary Salt) As such, contribution of interaction (GE) between geno- Table 3. Number of genes whose expression variations were impacted by environmental factors (dietary salt) identified by RAM and estimated FDR at a given threshold. Threshold Number of positive genes Number of false positive genes Estimated FDR 0.23054 4452 2968 0.667 0.307166 774 398 0.514 0.341366 485 292 0.602 0.378962 338 198 0.586 0.386128 301 165 0.548 0.409155 266 145 0.545 0.467169 168 86 0.512 0.478735 157 71 0.452 0.484306 147 65 0.442 0.510608 142 62 0.437 0.56461 114 46 0.404 0.579948 101 35 0.346 0.666147 62 20 0.322 0.686774 57 15 0.263 0.789565 36 9 0.250 0.92422 24 3 0.125 1.07455 22 1 0.045 1.323427 7 0 0 1.480786 4 0 0 1.546755 3 0 0 types and environmental factor (dietary salt) to expres- sion variations of genes is also pretty small (Figure 3). The numbers of the genes differential expressed due to GE interaction at a set of threshold levels were listed in Table 4. 25 genes were found to have the interesting change in expression at FDR ≤ 5%. Among them, 11 are EST, 12 are functional genes coding for synuclein 1, synaptojanin, flk protein, metabotropic glutamate re- ceptor 3, neurexin III-alpha, carboxypeptidase D pre- cursor (Cpd), electrogenic Na+ bicarbonate cotransporter (NBC), and ET-B endothelin receptor, GST Yc1, respec- tively, and 3 are unknown sequences (Supplemental Ta- ble 1). 23 of these 25 genes were down-regulated in the genotype resistant to stroke and the low salt environment or in the genotype prone to stroke and the high salt en- vironment but up-regulated in the genotype resistant to stroke and the high salt environment or in the genotype prone to stroke and the low salt environment. 4. DISCUSSION From Figures 1, 2 and 3, it can be seen that the effects of these factors for stroke on the expression variations of genes were well displayed by deviation of the T-distri- bution from the Z-distribution at two sides. As expected, the genetic difference between genotypes results in the significant expression variations of genes related to stroke (Figure 1). At FDR ≤ 5%, we found 341 func- tional genes differentially expressed between the geno- types SHRSP and SHRSR. Unlike the environmentally Table 4. Number of genes whose expression variations were impacted by GE identified by RAM and estimated FDR at a given threshold. Threshold Number of positive genes Number of false positive genes Estimated FDR 0.04302 7487 5729 0.765 0.125184 839 642 0.765 0.176549 511 385 0.753 0.186523 453 303 0.667 0.290752 263 175 0.665 0.390944 160 71 0.444 0.394555 158 58 0.367 0.536208 99 35 0.355 0.597688 77 16 0.208 0.621986 73 13 0.178 0.631139 69 11 0.159 0.685069 55 7 0.127 0.849769 38 4 0.105 0.876529 35 2 0.057 1.015051 25 1 0.040 1.071766 24 1 0.041 1.345698 8 0 0
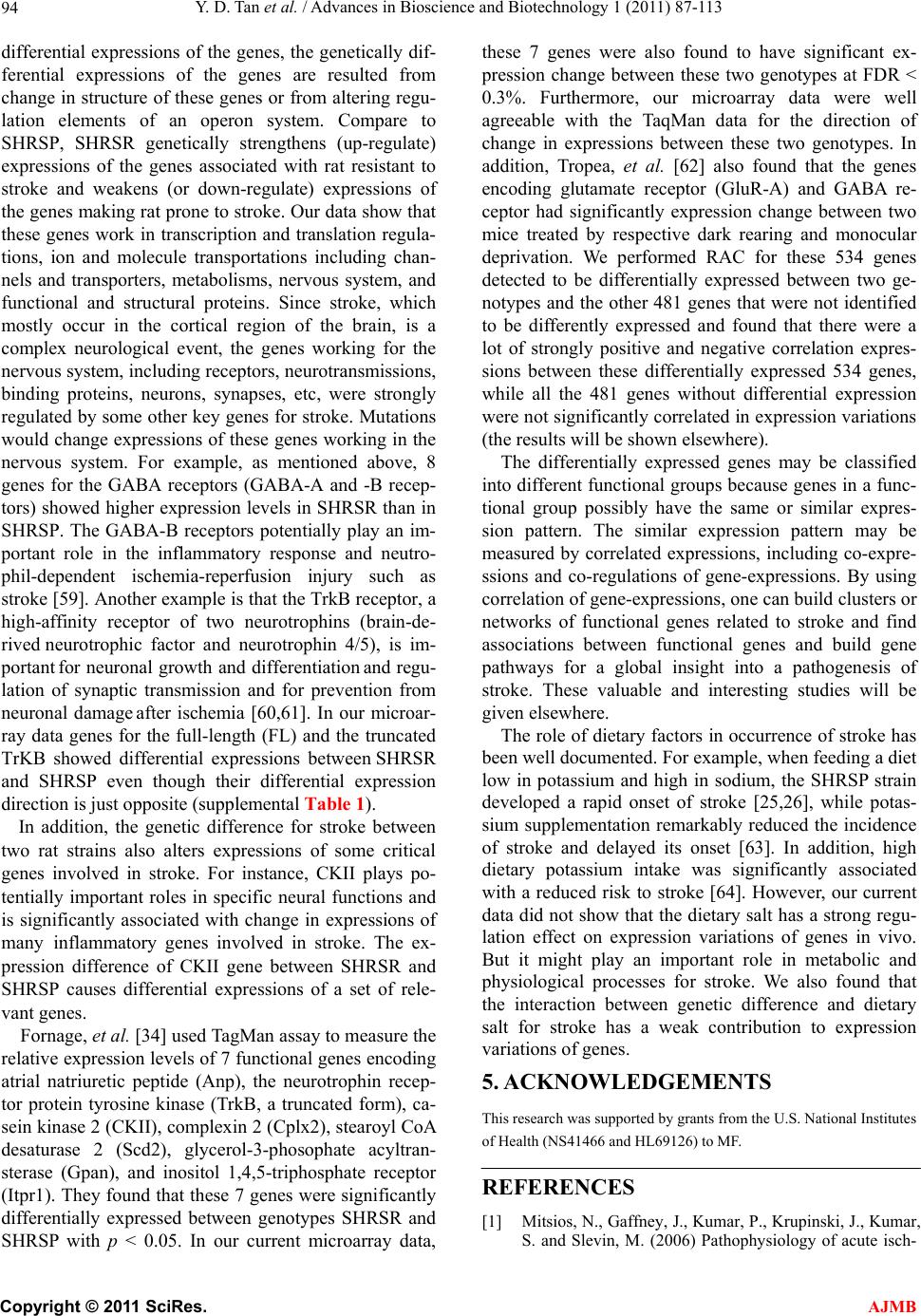 Y. D. Tan et al. / Advances in Bioscience and Biotechnology 1 (2011) 87-113 Copyright © 2011 SciRes. AJMB 94 differential expressions of the genes, the genetically dif- ferential expressions of the genes are resulted from change in structure of these genes or from altering regu- lation elements of an operon system. Compare to SHRSP, SHRSR genetically strengthens (up-regulate) expressions of the genes associated with rat resistant to stroke and weakens (or down-regulate) expressions of the genes making rat prone to stroke. Our data show that these genes work in transcription and translation regula- tions, ion and molecule transportations including chan- nels and transporters, metabolisms, nervous system, and functional and structural proteins. Since stroke, which mostly occur in the cortical region of the brain, is a complex neurological event, the genes working for the nervous system, including receptors, neurotransmissions, binding proteins, neurons, synapses, etc, were strongly regulated by some other key genes for stroke. Mutations would change expressions of these genes working in the nervous system. For example, as mentioned above, 8 genes for the GABA receptors (GABA-A and -B recep- tors) showed higher expression levels in SHRSR than in SHRSP. The GABA-B receptors potentially play an im- portant role in the inflammatory response and neutro- phil-dependent ischemia-reperfusion injury such as stroke [59]. Another example is that the TrkB receptor, a high-affinity receptor of two neurotrophins (brain-de- rived neurotrophic factor and neurotrophin 4/5), is im- portant for neuronal growth and differentiation and regu- lation of synaptic transmission and for prevention from neuronal damage after ischemia [60,61]. In our microar- ray data genes for the full-length (FL) and the truncated TrKB showed differential expressions between SHRSR and SHRSP even though their differential expression direction is just opposite (supplemental Table 1). In addition, the genetic difference for stroke between two rat strains also alters expressions of some critical genes involved in stroke. For instance, CKII plays po- tentially important roles in specific neural functions and is significantly associated with change in expressions of many inflammatory genes involved in stroke. The ex- pression difference of CKII gene between SHRSR and SHRSP causes differential expressions of a set of rele- vant genes. Fornage, et al. [34] used TagMan assay to measure the relative expression levels of 7 functional genes encoding atrial natriuretic peptide (Anp), the neurotrophin recep- tor protein tyrosine kinase (TrkB, a truncated form), ca- sein kinase 2 (CKII), complexin 2 (Cplx2), stearoyl CoA desaturase 2 (Scd2), glycerol-3-phosophate acyltran- sterase (Gpan), and inositol 1,4,5-triphosphate receptor (Itpr1). They found that these 7 genes were significantly differentially expressed between genotypes SHRSR and SHRSP with p < 0.05. In our current microarray data, these 7 genes were also found to have significant ex- pression change between these two genotypes at FDR < 0.3%. Furthermore, our microarray data were well agreeable with the TaqMan data for the direction of change in expressions between these two genotypes. In addition, Tropea, et al. [62] also found that the genes encoding glutamate receptor (GluR-A) and GABA re- ceptor had significantly expression change between two mice treated by respective dark rearing and monocular deprivation. We performed RAC for these 534 genes detected to be differentially expressed between two ge- notypes and the other 481 genes that were not identified to be differently expressed and found that there were a lot of strongly positive and negative correlation expres- sions between these differentially expressed 534 genes, while all the 481 genes without differential expression were not significantly correlated in expression variations (the results will be shown elsewhere). The differentially expressed genes may be classified into different functional groups because genes in a func- tional group possibly have the same or similar expres- sion pattern. The similar expression pattern may be measured by correlated expressions, including co-expre- ssions and co-regulations of gene-expressions. By using correlation of gene-expressions, one can build clusters or networks of functional genes related to stroke and find associations between functional genes and build gene pathways for a global insight into a pathogenesis of stroke. These valuable and interesting studies will be given elsewhere. The role of dietary factors in occurrence of stroke has been well documented. For example, when feeding a diet low in potassium and high in sodium, the SHRSP strain developed a rapid onset of stroke [25,26], while potas- sium supplementation remarkably reduced the incidence of stroke and delayed its onset [63]. In addition, high dietary potassium intake was significantly associated with a reduced risk to stroke [64]. However, our current data did not show that the dietary salt has a strong regu- lation effect on expression variations of genes in vivo. But it might play an important role in metabolic and physiological processes for stroke. We also found that the interaction between genetic difference and dietary salt for stroke has a weak contribution to expression variations of genes. 5. ACKNOWLEDGEMENTS This research was supported by grants from the U.S. National Institutes of Health (NS41466 and HL69126) to MF. REFERENCES [1] Mitsios, N., Gaffney, J., Kumar, P., Krupinski, J., Kumar, S. and Slevin, M. (2006) Pathophysiology of acute isch-
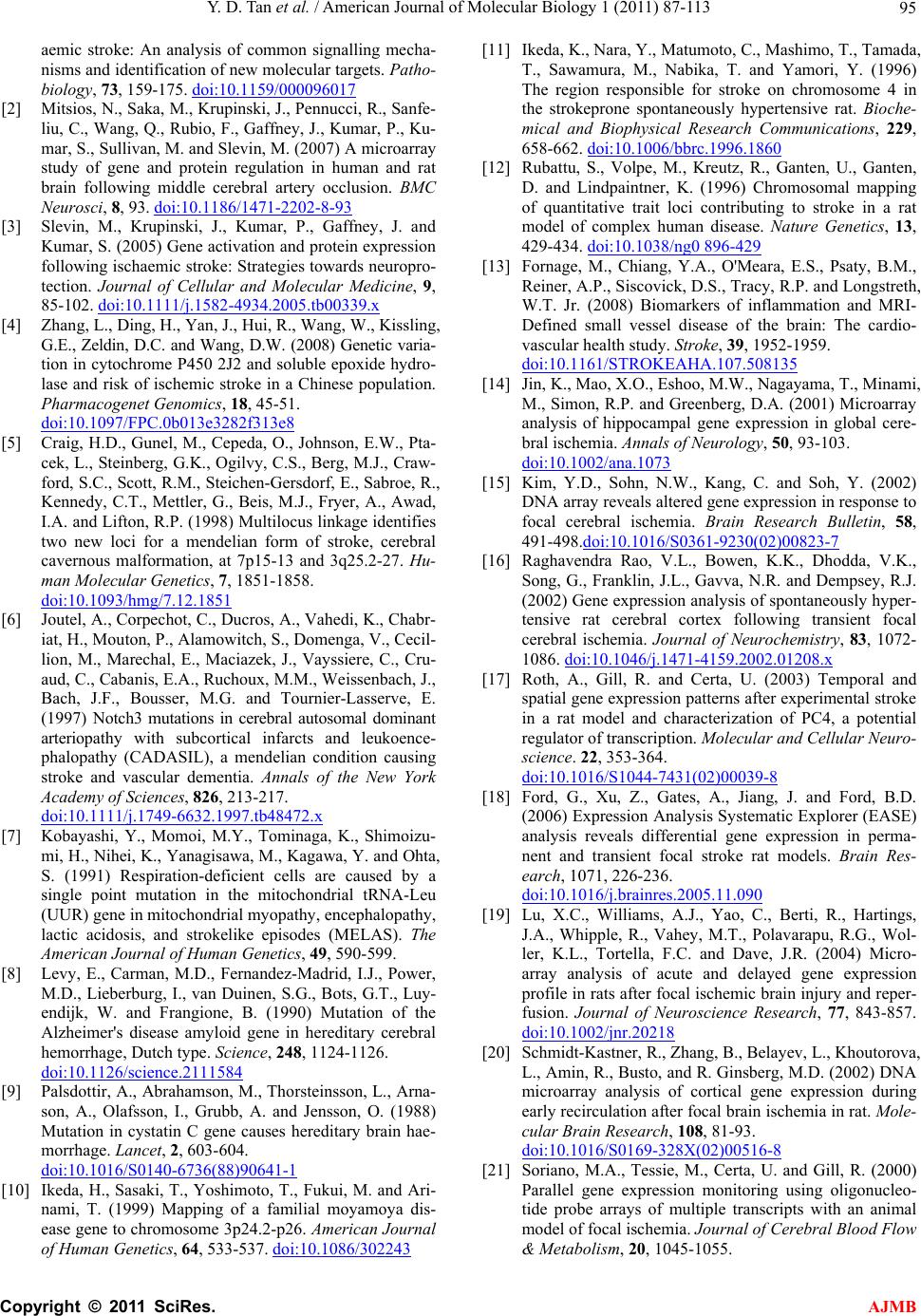 Y. D. Tan et al. / American Journal of Molecular Biology 1 (2011) 87-113 Copyright © 2011 SciRes. AJMB 95 aemic stroke: An analysis of common signalling mecha- nisms and identification of new molecular targets. Patho- biology, 73, 159-175. doi:10.1159/000096017 [2] Mitsios, N., Saka, M., Krupinski, J., Pennucci, R., Sanfe- liu, C., Wang, Q., Rubio, F., Gaffney, J., Kumar, P., Ku- mar, S., Sullivan, M. and Slevin, M. (2007) A microarray study of gene and protein regulation in human and rat brain following middle cerebral artery occlusion. BMC Neurosci, 8, 93. doi:10.1186/1471-2202-8-93 [3] Slevin, M., Krupinski, J., Kumar, P., Gaffney, J. and Kumar, S. (2005) Gene activation and protein expression following ischaemic stroke: Strategies towards neuropro- tection. Journal of Cellular and Molecular Medicine, 9, 85-102. doi:10.1111/j.1582-4934.2005.tb00339.x [4] Zhang, L., Ding, H., Yan, J., Hui, R., Wang, W., Kissling, G.E., Zeldin, D.C. and Wang, D.W. (2008) Genetic varia- tion in cytochrome P450 2J2 and soluble epoxide hydro- lase and risk of ischemic stroke in a Chinese population. Pharmacogenet Genomics, 18, 45-51. doi:10.1097/FPC.0b013e3282f313e8 [5] Craig, H.D., Gunel, M., Cepeda, O., Johnson, E.W., Pta- cek, L., Steinberg, G.K., Ogilvy, C.S., Berg, M.J., Craw- ford, S.C., Scott, R.M., Steichen-Gersdorf, E., Sabroe, R., Kennedy, C.T., Mettler, G., Beis, M.J., Fryer, A., Awad, I.A. and Lifton, R.P. (1998) Multilocus linkage identifies two new loci for a mendelian form of stroke, cerebral cavernous malformation, at 7p15-13 and 3q25.2-27. Hu- man Molecular Genetics, 7, 1851-1858. doi:10.1093/hmg/7.12.1851 [6] Joutel, A., Corpechot, C., Ducros, A., Vahedi, K., Chabr- iat, H., Mouton, P., Alamowitch, S., Domenga, V., Cecil- lion, M., Marechal, E., Maciazek, J., Vayssiere, C., Cru- aud, C., Cabanis, E.A., Ruchoux, M.M., Weissenbach, J., Bach, J.F., Bousser, M.G. and Tournier-Lasserve, E. (1997) Notch3 mutations in cerebral autosomal dominant arteriopathy with subcortical infarcts and leukoence- phalopathy (CADASIL), a mendelian condition causing stroke and vascular dementia. Annals of the New York Academy of Sciences, 826, 213-217. doi:10.1111/j.1749-6632.1997.tb48472.x [7] Kobayashi, Y., Momoi, M.Y., Tominaga, K., Shimoizu- mi, H., Nihei, K., Yanagisawa, M., Kagawa, Y. and Ohta, S. (1991) Respiration-deficient cells are caused by a single point mutation in the mitochondrial tRNA-Leu (UUR) gene in mitochondrial myopathy, encephalopathy, lactic acidosis, and strokelike episodes (MELAS). The American Journal of Human Genetics, 49, 590-599. [8] Levy, E., Carman, M.D., Fernandez-Madrid, I.J., Power, M.D., Lieberburg, I., van Duinen, S.G., Bots, G.T., Luy- endijk, W. and Frangione, B. (1990) Mutation of the Alzheimer's disease amyloid gene in hereditary cerebral hemorrhage, Dutch type. Science, 248, 1124-1126. doi:10.1126/science.2111584 [9] Palsdottir, A., Abrahamson, M., Thorsteinsson, L., Arna- son, A., Olafsson, I., Grubb, A. and Jensson, O. (1988) Mutation in cystatin C gene causes hereditary brain hae- morrhage. Lancet, 2, 603-604. doi:10.1016/S0140-6736(88)90641-1 [10] Ikeda, H., Sasaki, T., Yoshimoto, T., Fukui, M. and Ari- nami, T. (1999) Mapping of a familial moyamoya dis- ease gene to chromosome 3p24.2-p26. American Journal of Human Genetics, 64, 533-537. doi:10.1086/302243 [11] Ikeda, K., Nara, Y., Matumoto, C., Mashimo, T., Tamada, T., Sawamura, M., Nabika, T. and Yamori, Y. (1996) The region responsible for stroke on chromosome 4 in the strokeprone spontaneously hypertensive rat. Bioche- mical and Biophysical Research Communications, 229, 658-662. doi:10.1006/bbrc.1996.1860 [12] Rubattu, S., Volpe, M., Kreutz, R., Ganten, U., Ganten, D. and Lindpaintner, K. (1996) Chromosomal mapping of quantitative trait loci contributing to stroke in a rat model of complex human disease. Nature Genetics, 13, 429-434. doi:10.1038/ng0 896-429 [13] Fornage, M., Chiang, Y.A., O'Meara, E.S., Psaty, B.M., Reiner, A.P., Siscovick, D.S., Tracy, R.P. and Longstreth, W.T. Jr. (2008) Biomarkers of inflammation and MRI- Defined small vessel disease of the brain: The cardio- vascular health study. Stroke, 39, 1952-1959. doi:10.1161/STROKEAHA.107.508135 [14] Jin, K., Mao, X.O., Eshoo, M.W., Nagayama, T., Minami, M., Simon, R.P. and Greenberg, D.A. (2001) Microarray analysis of hippocampal gene expression in global cere- bral ischemia. Annals of Neurology, 50, 93-103. doi:10.1002/ana.1073 [15] Kim, Y.D., Sohn, N.W., Kang, C. and Soh, Y. (2002) DNA array reveals altered gene expression in response to focal cerebral ischemia. Brain Research Bulletin, 58, 491-498.doi:10.1016/S0361-9230(02)00823-7 [16] Raghavendra Rao, V.L., Bowen, K.K., Dhodda, V.K., Song, G., Franklin, J.L., Gavva, N.R. and Dempsey, R.J. (2002) Gene expression analysis of spontaneously hyper- tensive rat cerebral cortex following transient focal cerebral ischemia. Journal of Neurochemistry, 83, 1072- 1086. doi:10.1046/j.1471-4159.2002.01208.x [17] Roth, A., Gill, R. and Certa, U. (2003) Temporal and spatial gene expression patterns after experimental stroke in a rat model and characterization of PC4, a potential regulator of transcription. Molecular and Cellular Neuro- science. 22, 353-364. doi:10.1016/S1044-7431(02)00039-8 [18] Ford, G., Xu, Z., Gates, A., Jiang, J. and Ford, B.D. (2006) Expression Analysis Systematic Explorer (EASE) analysis reveals differential gene expression in perma- nent and transient focal stroke rat models. Brain Res- earch, 1071, 226-236. doi:10.1016/j.brainres.2005.11.090 [19] Lu, X.C., Williams, A.J., Yao, C., Berti, R., Hartings, J.A., Whipple, R., Vahey, M.T., Polavarapu, R.G., Wol- ler, K.L., Tortella, F.C. and Dave, J.R. (2004) Micro- array analysis of acute and delayed gene expression profile in rats after focal ischemic brain injury and reper- fusion. Journal of Neuroscience Research, 77, 843-857. doi:10.1002/jnr.20218 [20] Schmidt-Kastner, R., Zhang, B., Belayev, L., Khoutorova, L., Amin, R., Busto, and R. Ginsberg, M.D. (2002) DNA microarray analysis of cortical gene expression during early recirculation after focal brain ischemia in rat. Mole- cular Brain Research, 108, 81-93. doi:10.1016/S0169-328X(02)00516-8 [21] Soriano, M.A., Tessie, M., Certa, U. and Gill, R. (2000) Parallel gene expression monitoring using oligonucleo- tide probe arrays of multiple transcripts with an animal model of focal ischemia. Journal of Cerebral Blood Flow & Metabolism, 20, 1045-1055.
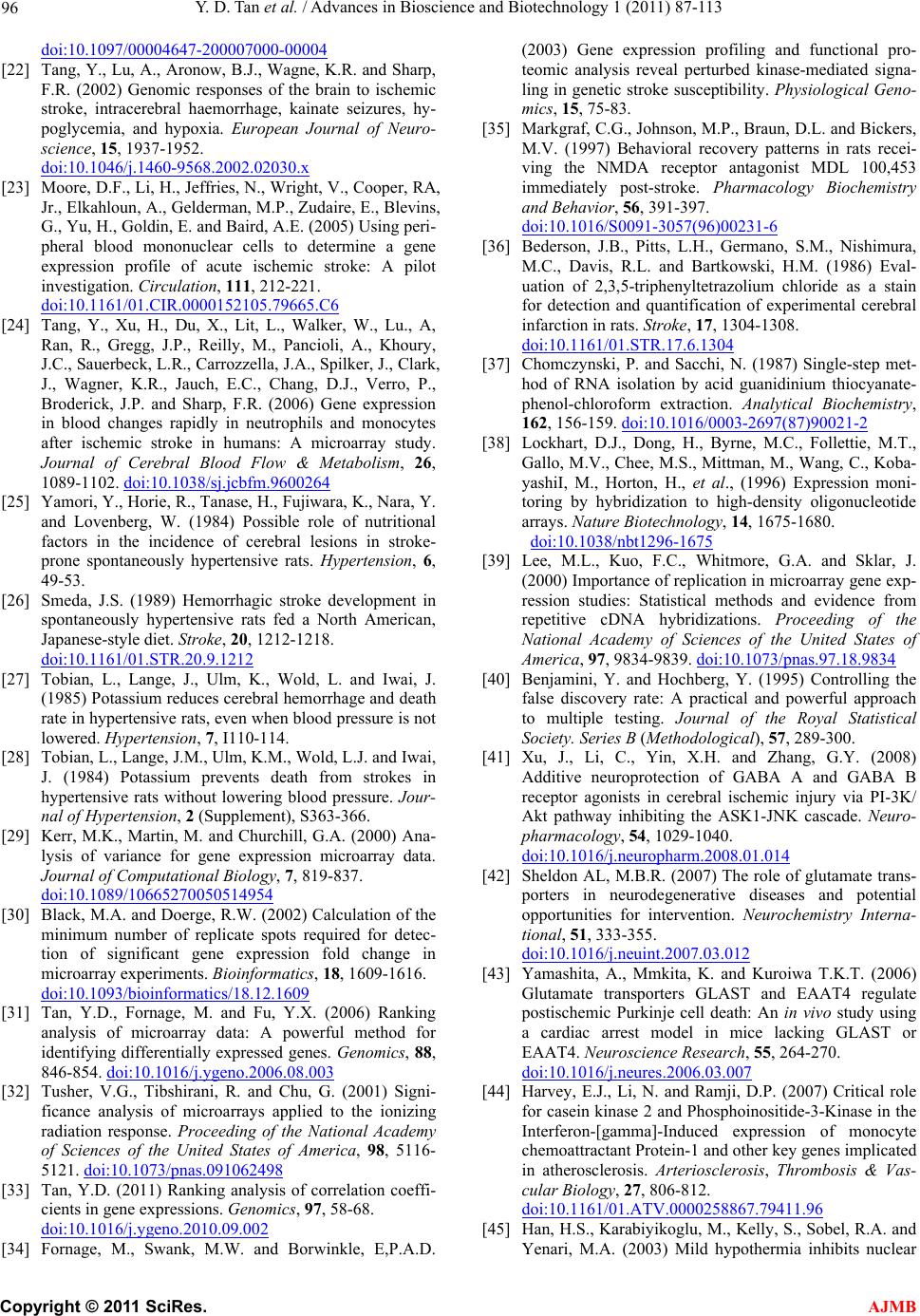 Y. D. Tan et al. / Advances in Bioscience and Biotechnology 1 (2011) 87-113 Copyright © 2011 SciRes. AJMB 96 doi:10.1097/00004647-200007000-00004 [22] Tang, Y., Lu, A., Aronow, B.J., Wagne, K.R. and Sharp, F.R. (2002) Genomic responses of the brain to ischemic stroke, intracerebral haemorrhage, kainate seizures, hy- poglycemia, and hypoxia. European Journal of Neuro- science, 15, 1937-1952. doi:10.1046/j.1460-9568.2002.02030.x [23] Moore, D.F., Li, H., Jeffries, N., Wright, V., Cooper, RA, Jr., Elkahloun, A., Gelderman, M.P., Zudaire, E., Blevins, G., Yu, H., Goldin, E. and Baird, A.E. (2005) Using peri- pheral blood mononuclear cells to determine a gene expression profile of acute ischemic stroke: A pilot investigation. Circulation, 111, 212-221. doi:10.1161/01.CIR.0000152105.79665.C6 [24] Tang, Y., Xu, H., Du, X., Lit, L., Walker, W., Lu., A, Ran, R., Gregg, J.P., Reilly, M., Pancioli, A., Khoury, J.C., Sauerbeck, L.R., Carrozzella, J.A., Spilker, J., Clark, J., Wagner, K.R., Jauch, E.C., Chang, D.J., Verro, P., Broderick, J.P. and Sharp, F.R. (2006) Gene expression in blood changes rapidly in neutrophils and monocytes after ischemic stroke in humans: A microarray study. Journal of Cerebral Blood Flow & Metabolism, 26, 1089-1102. doi:10.1038/sj.jcbfm.9600264 [25] Yamori, Y., Horie, R., Tanase, H., Fujiwara, K., Nara, Y. and Lovenberg, W. (1984) Possible role of nutritional factors in the incidence of cerebral lesions in stroke- prone spontaneously hypertensive rats. Hypertension, 6, 49-53. [26] Smeda, J.S. (1989) Hemorrhagic stroke development in spontaneously hypertensive rats fed a North American, Japanese-style diet. Stroke, 20, 1212-1218. doi:10.1161/01.STR.20.9.1212 [27] Tobian, L., Lange, J., Ulm, K., Wold, L. and Iwai, J. (1985) Potassium reduces cerebral hemorrhage and death rate in hypertensive rats, even when blood pressure is not lowered. Hypertension, 7, I110-114. [28] Tobian, L., Lange, J.M., Ulm, K.M., Wold, L.J. and Iwai, J. (1984) Potassium prevents death from strokes in hypertensive rats without lowering blood pressure. Jour- nal of Hypertension, 2 (Supplement), S363-366. [29] Kerr, M.K., Martin, M. and Churchill, G.A. (2000) Ana- lysis of variance for gene expression microarray data. Journal of Computational Biology, 7, 819-837. doi:10.1089/10665270050514954 [30] Black, M.A. and Doerge, R.W. (2002) Calculation of the minimum number of replicate spots required for detec- tion of significant gene expression fold change in microarray experiments. Bioinformatics, 18, 1609-1616. doi:10.1093/bioinformatics/18.12.1609 [31] Tan, Y.D., Fornage, M. and Fu, Y.X. (2006) Ranking analysis of microarray data: A powerful method for identifying differentially expressed genes. Genomics, 88, 846-854. doi:10.1016/j.ygeno.2006.08.003 [32] Tusher, V.G., Tibshirani, R. and Chu, G. (2001) Signi- ficance analysis of microarrays applied to the ionizing radiation response. Proceeding of the National Academy of Sciences of the United States of America, 98, 5116- 5121. doi:10.1073/pnas.091062498 [33] Tan, Y.D. (2011) Ranking analysis of correlation coeffi- cients in gene expressions. Genomics, 97, 58-68. doi:10.1016/j.ygeno.2010.09.002 [34] Fornage, M., Swank, M.W. and Borwinkle, E,P.A.D. (2003) Gene expression profiling and functional pro- teomic analysis reveal perturbed kinase-mediated signa- ling in genetic stroke susceptibility. Physiological Geno- mics, 15, 75-83. [35] Markgraf, C.G., Johnson, M.P., Braun, D.L. and Bickers, M.V. (1997) Behavioral recovery patterns in rats recei- ving the NMDA receptor antagonist MDL 100,453 immediately post-stroke. Pharmacology Biochemistry and Behavior, 56, 391-397. doi:10.1016/S0091-3057(96)00231-6 [36] Bederson, J.B., Pitts, L.H., Germano, S.M., Nishimura, M.C., Davis, R.L. and Bartkowski, H.M. (1986) Eval- uation of 2,3,5-triphenyltetrazolium chloride as a stain for detection and quantification of experimental cerebral infarction in rats. Stroke, 17, 1304-1308. doi:10.1161/01.STR.17.6.1304 [37] Chomczynski, P. and Sacchi, N. (1987) Single-step met- hod of RNA isolation by acid guanidinium thiocyanate- phenol-chloroform extraction. Analytical Biochemistry, 162, 156-159. doi:10.1016/0003-2697(87)90021-2 [38] Lockhart, D.J., Dong, H., Byrne, M.C., Follettie, M.T., Gallo, M.V., Chee, M.S., Mittman, M., Wang, C., Koba- yashiI, M., Horton, H., et al., (1996) Expression moni- toring by hybridization to high-density oligonucleotide arrays. Nature Biotechnology, 14, 1675-1680. doi:10.1038/nbt1296-1675 [39] Lee, M.L., Kuo, F.C., Whitmore, G.A. and Sklar, J. (2000) Importance of replication in microarray gene exp- ression studies: Statistical methods and evidence from repetitive cDNA hybridizations. Proceeding of the National Academy of Sciences of the United States of America, 97, 9834-9839. doi:10.1073/pnas.97.18.9834 [40] Benjamini, Y. and Hochberg, Y. (1995) Controlling the false discovery rate: A practical and powerful approach to multiple testing. Journal of the Royal Statistical Society. Series B (Methodological), 57, 289-300. [41] Xu, J., Li, C., Yin, X.H. and Zhang, G.Y. (2008) Additive neuroprotection of GABA A and GABA B receptor agonists in cerebral ischemic injury via PI-3K/ Akt pathway inhibiting the ASK1-JNK cascade. Neuro- pharmacology, 54, 1029-1040. doi:10.1016/j.neuropharm.2008.01.014 [42] Sheldon AL, M.B.R. (2007) The role of glutamate trans- porters in neurodegenerative diseases and potential opportunities for intervention. Neurochemistry Interna- tional, 51, 333-355. doi:10.1016/j.neuint.2007.03.012 [43] Yamashita, A., Mmkita, K. and Kuroiwa T.K.T. (2006) Glutamate transporters GLAST and EAAT4 regulate postischemic Purkinje cell death: An in vivo study using a cardiac arrest model in mice lacking GLAST or EAAT4. Neuroscience Research, 55, 264-270. doi:10.1016/j.neures.2006.03.007 [44] Harvey, E.J., Li, N. and Ramji, D.P. (2007) Critical role for casein kinase 2 and Phosphoinositide-3-Kinase in the Interferon-[gamma]-Induced expression of monocyte chemoattractant Protein-1 and other key genes implicated in atherosclerosis. Arteriosclerosis, Thrombosis & Vas- cular Biology, 27, 806-812. doi:10.1161/01.ATV.0000258867.79411.96 [45] Han, H.S., Karabiyikoglu, M., Kelly, S., Sobel, R.A. and Yenari, M.A. (2003) Mild hypothermia inhibits nuclear
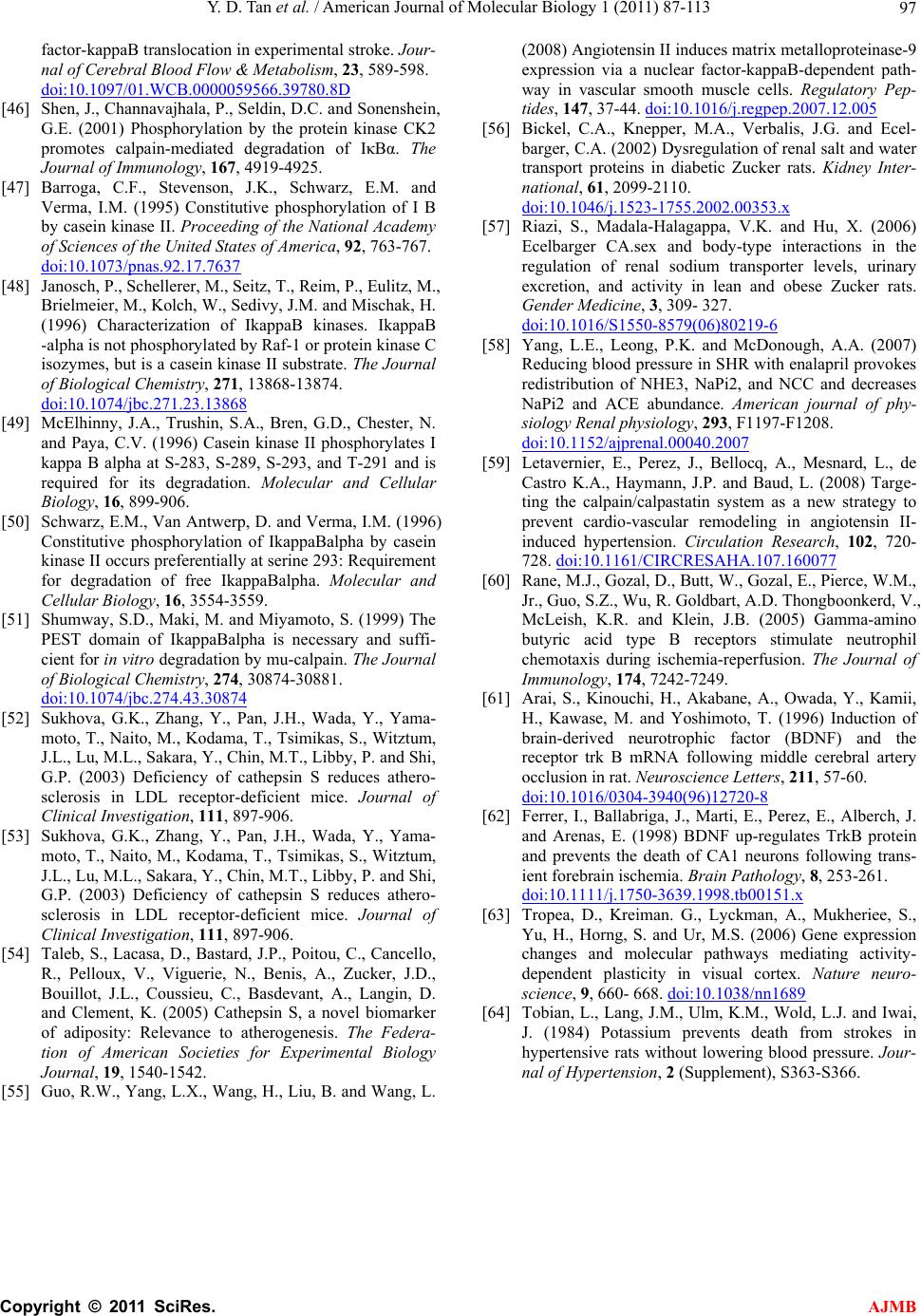 Y. D. Tan et al. / American Journal of Molecular Biology 1 (2011) 87-113 Copyright © 2011 SciRes. AJMB 97 factor-kappaB translocation in experimental stroke. Jour- nal of Cerebral Blood Flow & Metabolism, 23, 589-598. doi:10.1097/01.WCB.0000059566.39780.8D [46] Shen, J., Channavajhala, P., Seldin, D.C. and Sonenshein, G.E. (2001) Phosphorylation by the protein kinase CK2 promotes calpain-mediated degradation of IκBα. The Journal of Immunology, 167, 4919-4925. [47] Barroga, C.F., Stevenson, J.K., Schwarz, E.M. and Verma, I.M. (1995) Constitutive phosphorylation of I B by casein kinase II. Proceeding of the National Academy of Sciences of the United States of America, 92, 763-767. doi:10.1073/pnas.92.17.7637 [48] Janosch, P., Schellerer, M., Seitz, T., Reim, P., Eulitz, M., Brielmeier, M., Kolch, W., Sedivy, J.M. and Mischak, H. (1996) Characterization of IkappaB kinases. IkappaB -alpha is not phosphorylated by Raf-1 or protein kinase C isozymes, but is a casein kinase II substrate. The Journal of Biological Chemistry, 271, 13868-13874. doi:10.1074/jbc.271.23.13868 [49] McElhinny, J.A., Trushin, S.A., Bren, G.D., Chester, N. and Paya, C.V. (1996) Casein kinase II phosphorylates I kappa B alpha at S-283, S-289, S-293, and T-291 and is required for its degradation. Molecular and Cellular Biology, 16, 899-906. [50] Schwarz, E.M., Van Antwerp, D. and Verma, I.M. (1996) Constitutive phosphorylation of IkappaBalpha by casein kinase II occurs preferentially at serine 293: Requirement for degradation of free IkappaBalpha. Molecular and Cellular Biology, 16, 3554-3559. [51] Shumway, S.D., Maki, M. and Miyamoto, S. (1999) The PEST domain of IkappaBalpha is necessary and suffi- cient for in vitro degradation by mu-calpain. The Journal of Biological Chemistry, 274, 30874-30881. doi:10.1074/jbc.274.43.30874 [52] Sukhova, G.K., Zhang, Y., Pan, J.H., Wada, Y., Yama- moto, T., Naito, M., Kodama, T., Tsimikas, S., Witztum, J.L., Lu, M.L., Sakara, Y., Chin, M.T., Libby, P. and Shi, G.P. (2003) Deficiency of cathepsin S reduces athero- sclerosis in LDL receptor-deficient mice. Journal of Clinical Investigation, 111, 897-906. [53] Sukhova, G.K., Zhang, Y., Pan, J.H., Wada, Y., Yama- moto, T., Naito, M., Kodama, T., Tsimikas, S., Witztum, J.L., Lu, M.L., Sakara, Y., Chin, M.T., Libby, P. and Shi, G.P. (2003) Deficiency of cathepsin S reduces athero- sclerosis in LDL receptor-deficient mice. Journal of Clinical Investigation, 111, 897-906. [54] Taleb, S., Lacasa, D., Bastard, J.P., Poitou, C., Cancello, R., Pelloux, V., Viguerie, N., Benis, A., Zucker, J.D., Bouillot, J.L., Coussieu, C., Basdevant, A., Langin, D. and Clement, K. (2005) Cathepsin S, a novel biomarker of adiposity: Relevance to atherogenesis. The Federa- tion of American Societies for Experimental Biology Journal, 19, 1540-1542. [55] Guo, R.W., Yang, L.X., Wang, H., Liu, B. and Wang, L. (2008) Angiotensin II induces matrix metalloproteinase-9 expression via a nuclear factor-kappaB-dependent path- way in vascular smooth muscle cells. Regulatory Pep- tides, 147, 37-44. doi:10.1016/j.regpep.2007.12.005 [56] Bickel, C.A., Knepper, M.A., Verbalis, J.G. and Ecel- barger, C.A. (2002) Dysregulation of renal salt and water transport proteins in diabetic Zucker rats. Kidney Inter- national, 61, 2099-2110. doi:10.1046/j.1523-1755.2002.00353.x [57] Riazi, S., Madala-Halagappa, V.K. and Hu, X. (2006) Ecelbarger CA.sex and body-type interactions in the regulation of renal sodium transporter levels, urinary excretion, and activity in lean and obese Zucker rats. Gender Medicine, 3, 309- 327. doi:10.1016/S1550-8579(06)80219-6 [58] Yang, L.E., Leong, P.K. and McDonough, A.A. (2007) Reducing blood pressure in SHR with enalapril provokes redistribution of NHE3, NaPi2, and NCC and decreases NaPi2 and ACE abundance. American journal of phy- siology Renal physiology, 293, F1197-F1208. doi:10.1152/ajprenal.00040.2007 [59] Letavernier, E., Perez, J., Bellocq, A., Mesnard, L., de Castro K.A., Haymann, J.P. and Baud, L. (2008) Targe- ting the calpain/calpastatin system as a new strategy to prevent cardio-vascular remodeling in angiotensin II- induced hypertension. Circulation Research, 102, 720- 728. doi:10.1161/CIRCRESAHA.107.160077 [60] Rane, M.J., Gozal, D., Butt, W., Gozal, E., Pierce, W.M., Jr., Guo, S.Z., Wu, R. Goldbart, A.D. Thongboonkerd, V., McLeish, K.R. and Klein, J.B. (2005) Gamma-amino butyric acid type B receptors stimulate neutrophil chemotaxis during ischemia-reperfusion. The Journal of Immunology, 174, 7242-7249. [61] Arai, S., Kinouchi, H., Akabane, A., Owada, Y., Kamii, H., Kawase, M. and Yoshimoto, T. (1996) Induction of brain-derived neurotrophic factor (BDNF) and the receptor trk B mRNA following middle cerebral artery occlusion in rat. Neuroscience Letters, 211, 57-60. doi:10.1016/0304-3940(96)12720-8 [62] Ferrer, I., Ballabriga, J., Marti, E., Perez, E., Alberch, J. and Arenas, E. (1998) BDNF up-regulates TrkB protein and prevents the death of CA1 neurons following trans- ient forebrain ischemia. Brain Pathology, 8, 253-261. doi:10.1111/j.1750-3639.1998.tb00151.x [63] Tropea, D., Kreiman. G., Lyckman, A., Mukheriee, S., Yu, H., Horng, S. and Ur, M.S. (2006) Gene expression changes and molecular pathways mediating activity- dependent plasticity in visual cortex. Nature neuro- science, 9, 660- 668. doi:10.1038/nn1689 [64] Tobian, L., Lang, J.M., Ulm, K.M., Wold, L.J. and Iwai, J. (1984) Potassium prevents death from strokes in hypertensive rats without lowering blood pressure. Jour- nal of Hypertension, 2 (Supplement), S363-S366.
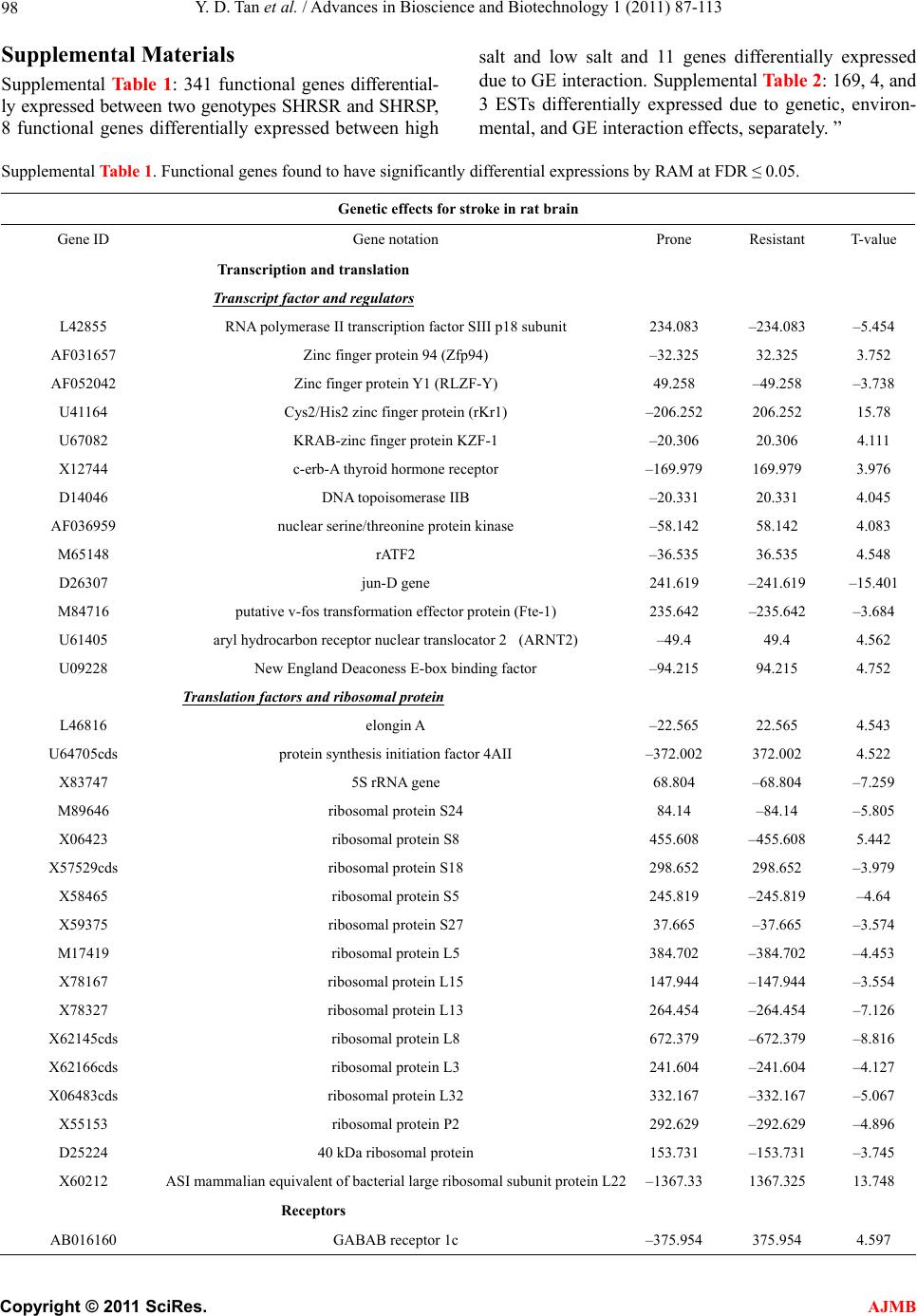 Y. D. Tan et al. / Advances in Bioscience and Biotechnology 1 (2011) 87-113 Copyright © 2011 SciRes. AJMB 98 Supplemental Materials Supplemental Table 1: 341 functional genes differential- ly expressed between two genotypes SHRSR and SHRSP, 8 functional genes differentially expressed between high salt and low salt and 11 genes differentially expressed due to GE interaction. Supplemental Table 2: 169, 4, and 3 ESTs differentially expressed due to genetic, environ- mental, and GE interaction effects, separately. ” Supplemental Table 1. Functional genes found to have significantly differential expressions by RAM at FDR ≤ 0.05. Genetic effects for stroke in rat brain Gene ID Gene notation Prone Resistant T-value Transcription and translation Transcript factor and regulators L42855 RNA polymerase II transcription factor SIII p18 subunit 234.083 –234.083 –5.454 AF031657 Zinc finger protein 94 (Zfp94) –32.325 32.325 3.752 AF052042 Zinc finger protein Y1 (RLZF-Y) 49.258 –49.258 –3.738 U41164 Cys2/His2 zinc finger protein (rKr1) –206.252 206.252 15.78 U67082 KRAB-zinc finger protein KZF-1 –20.306 20.306 4.111 X12744 c-erb-A thyroid hormone receptor –169.979 169.979 3.976 D14046 DNA topoisomerase IIB –20.331 20.331 4.045 AF036959 nuclear serine/threonine protein kinase –58.142 58.142 4.083 M65148 rATF2 –36.535 36.535 4.548 D26307 jun-D gene 241.619 –241.619 –15.401 M84716 putative v-fos transformation effector protein (Fte-1) 235.642 –235.642 –3.684 U61405 aryl hydrocarbon receptor nuclear translocator 2 (ARNT2) –49.4 49.4 4.562 U09228 New England Deaconess E-box binding factor –94.215 94.215 4.752 Translation factors and ribosomal protein L46816 elongin A –22.565 22.565 4.543 U64705cds protein synthesis initiation factor 4AII –372.002 372.002 4.522 X83747 5S rRNA gene 68.804 –68.804 –7.259 M89646 ribosomal protein S24 84.14 –84.14 –5.805 X06423 ribosomal protein S8 455.608 –455.608 5.442 X57529cds ribosomal protein S18 298.652 298.652 –3.979 X58465 ribosomal protein S5 245.819 –245.819 –4.64 X59375 ribosomal protein S27 37.665 –37.665 –3.574 M17419 ribosomal protein L5 384.702 –384.702 –4.453 X78167 ribosomal protein L15 147.944 –147.944 –3.554 X78327 ribosomal protein L13 264.454 –264.454 –7.126 X62145cds ribosomal protein L8 672.379 –672.379 –8.816 X62166cds ribosomal protein L3 241.604 –241.604 –4.127 X06483cds ribosomal protein L32 332.167 –332.167 –5.067 X55153 ribosomal protein P2 292.629 –292.629 –4.896 D25224 40 kDa ribosomal protein 153.731 –153.731 –3.745 X60212 ASI mammalian equivalent of bacterial large ribosomal subunit protein L22–1367.33 1367.325 13.748 Receptors AB016160 GABAB receptor 1c –375.954 375.954 4.597
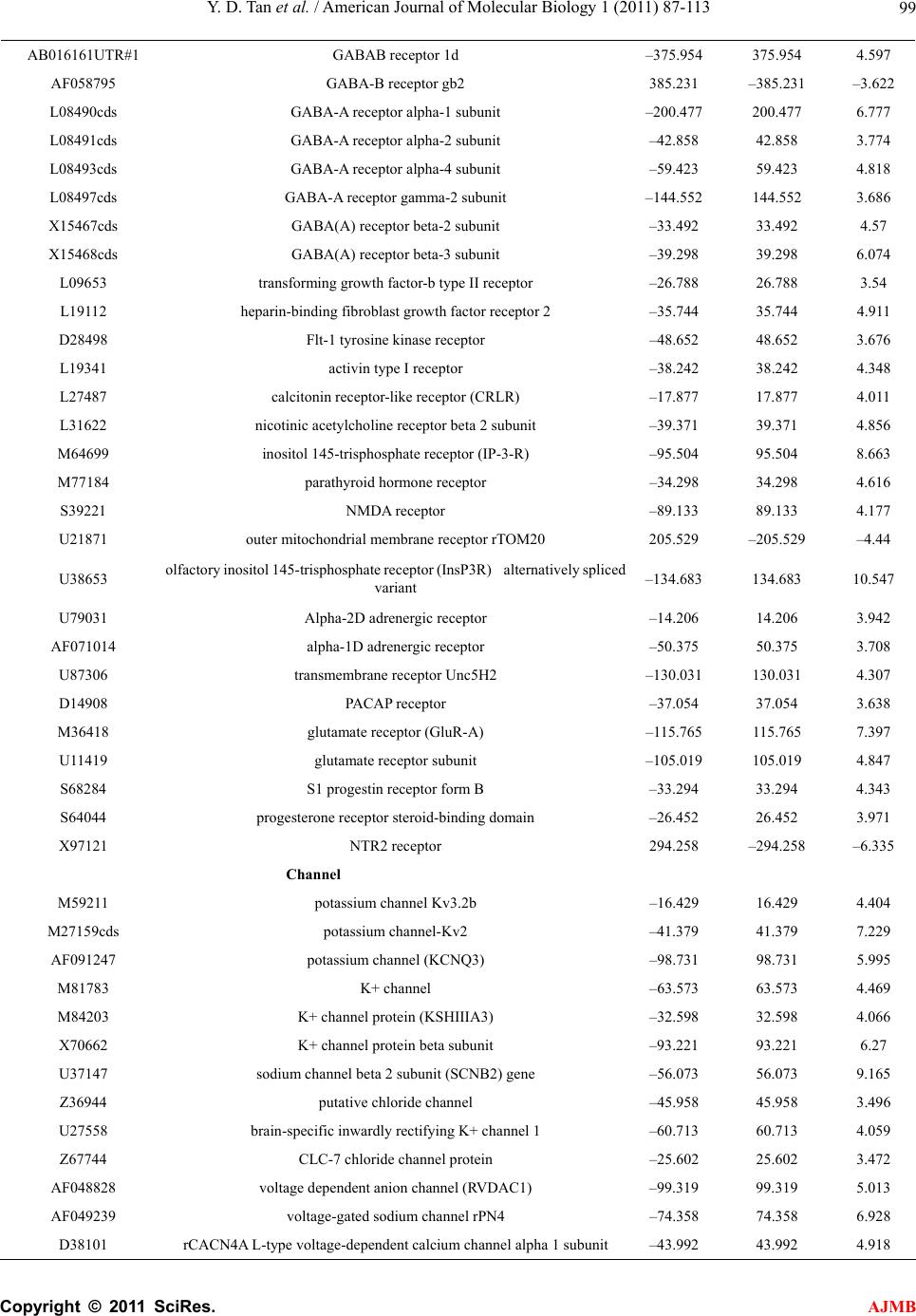 Y. D. Tan et al. / American Journal of Molecular Biology 1 (2011) 87-113 Copyright © 2011 SciRes. AJMB 99 AB016161UTR#1 GABAB receptor 1d –375.954 375.954 4.597 AF058795 GABA-B receptor gb2 385.231 –385.231 –3.622 L08490cds GABA-A receptor alpha-1 subunit –200.477 200.477 6.777 L08491cds GABA-A receptor alpha-2 subunit –42.858 42.858 3.774 L08493cds GABA-A receptor alpha-4 subunit –59.423 59.423 4.818 L08497cds GABA-A receptor gamma-2 subunit –144.552 144.552 3.686 X15467cds GABA(A) receptor beta-2 subunit –33.492 33.492 4.57 X15468cds GABA(A) receptor beta-3 subunit –39.298 39.298 6.074 L09653 transforming growth factor-b type II receptor –26.788 26.788 3.54 L19112 heparin-binding fibroblast growth factor receptor 2 –35.744 35.744 4.911 D28498 Flt-1 tyrosine kinase receptor –48.652 48.652 3.676 L19341 activin type I receptor –38.242 38.242 4.348 L27487 calcitonin receptor-like receptor (CRLR) –17.877 17.877 4.011 L31622 nicotinic acetylcholine receptor beta 2 subunit –39.371 39.371 4.856 M64699 inositol 145-trisphosphate receptor (IP-3-R) –95.504 95.504 8.663 M77184 parathyroid hormone receptor –34.298 34.298 4.616 S39221 NMDA receptor –89.133 89.133 4.177 U21871 outer mitochondrial membrane receptor rTOM20 205.529 –205.529 –4.44 U38653 olfactory inositol 145-trisphosphate receptor (InsP3R) alternatively spliced variant –134.683 134.683 10.547 U79031 Alpha-2D adrenergic receptor –14.206 14.206 3.942 AF071014 alpha-1D adrenergic receptor –50.375 50.375 3.708 U87306 transmembrane receptor Unc5H2 –130.031 130.031 4.307 D14908 PACAP receptor –37.054 37.054 3.638 M36418 glutamate receptor (GluR-A) –115.765 115.765 7.397 U11419 glutamate receptor subunit –105.019 105.019 4.847 S68284 S1 progestin receptor form B –33.294 33.294 4.343 S64044 progesterone receptor steroid-binding domain –26.452 26.452 3.971 X97121 NTR2 receptor 294.258 –294.258 –6.335 Channel M59211 potassium channel Kv3.2b –16.429 16.429 4.404 M27159cds potassium channel-Kv2 –41.379 41.379 7.229 AF091247 potassium channel (KCNQ3) –98.731 98.731 5.995 M81783 K+ channel –63.573 63.573 4.469 M84203 K+ channel protein (KSHIIIA3) –32.598 32.598 4.066 X70662 K+ channel protein beta subunit –93.221 93.221 6.27 U37147 sodium channel beta 2 subunit (SCNB2) gene –56.073 56.073 9.165 Z36944 putative chloride channel –45.958 45.958 3.496 U27558 brain-specific inwardly rectifying K+ channel 1 –60.713 60.713 4.059 Z67744 CLC-7 chloride channel protein –25.602 25.602 3.472 AF048828 voltage dependent anion channel (RVDAC1) –99.319 99.319 5.013 AF049239 voltage-gated sodium channel rPN4 –74.358 74.358 6.928 D38101 rCACN4A L-type voltage-dependent calcium channel alpha 1 subunit –43.992 43.992 4.918
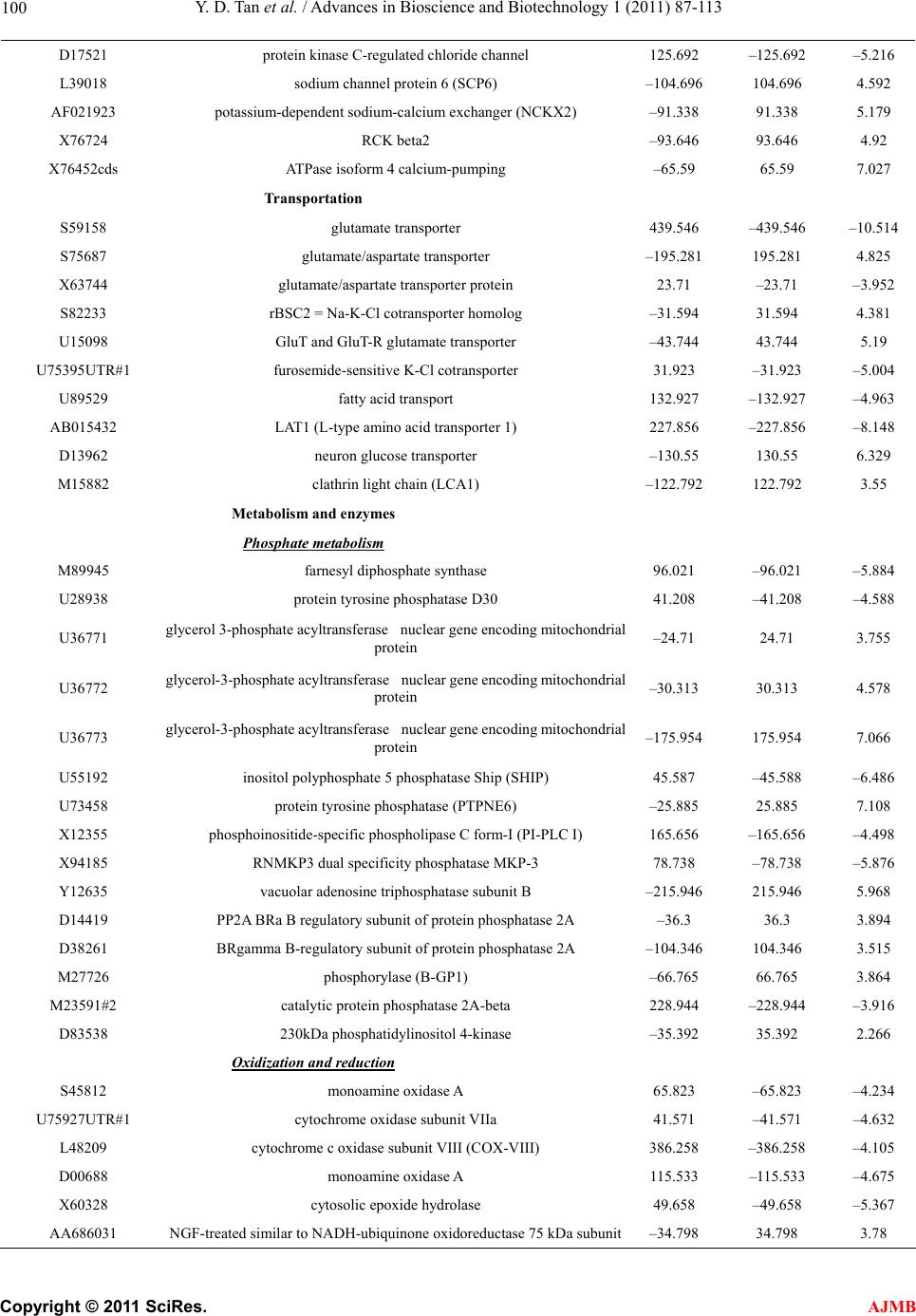 Y. D. Tan et al. / Advances in Bioscience and Biotechnology 1 (2011) 87-113 Copyright © 2011 SciRes. AJMB 100 D17521 protein kinase C-regulated chloride channel 125.692 –125.692 –5.216 L39018 sodium channel protein 6 (SCP6) –104.696 104.696 4.592 AF021923 potassium-dependent sodium-calcium exchanger (NCKX2) –91.338 91.338 5.179 X76724 RCK beta2 –93.646 93.646 4.92 X76452cds ATPase isoform 4 calcium-pumping –65.59 65.59 7.027 Transportation S59158 glutamate transporter 439.546 –439.546 –10.514 S75687 glutamate/aspartate transporter –195.281 195.281 4.825 X63744 glutamate/aspartate transporter protein 23.71 –23.71 –3.952 S82233 rBSC2 = Na-K-Cl cotransporter homolog –31.594 31.594 4.381 U15098 GluT and GluT-R glutamate transporter –43.744 43.744 5.19 U75395UTR#1 furosemide-sensitive K-Cl cotransporter 31.923 –31.923 –5.004 U89529 fatty acid transport 132.927 –132.927 –4.963 AB015432 LAT1 (L-type amino acid transporter 1) 227.856 –227.856 –8.148 D13962 neuron glucose transporter –130.55 130.55 6.329 M15882 clathrin light chain (LCA1) –122.792 122.792 3.55 Metabolism and enzymes Phosphate metabolism M89945 farnesyl diphosphate synthase 96.021 –96.021 –5.884 U28938 protein tyrosine phosphatase D30 41.208 –41.208 –4.588 U36771 glycerol 3-phosphate acyltransferase nuclear gene encoding mitochondrial protein –24.71 24.71 3.755 U36772 glycerol-3-phosphate acyltransferase nuclear gene encoding mitochondrial protein –30.313 30.313 4.578 U36773 glycerol-3-phosphate acyltransferase nuclear gene encoding mitochondrial protein –175.954 175.954 7.066 U55192 inositol polyphosphate 5 phosphatase Ship (SHIP) 45.587 –45.588 –6.486 U73458 protein tyrosine phosphatase (PTPNE6) –25.885 25.885 7.108 X12355 phosphoinositide-specific phospholipase C form-I (PI-PLC I) 165.656 –165.656 –4.498 X94185 RNMKP3 dual specificity phosphatase MKP-3 78.738 –78.738 –5.876 Y12635 vacuolar adenosine triphosphatase subunit B –215.946 215.946 5.968 D14419 PP2A BRa B regulatory subunit of protein phosphatase 2A –36.3 36.3 3.894 D38261 BRgamma B-regulatory subunit of protein phosphatase 2A –104.346 104.346 3.515 M27726 phosphorylase (B-GP1) –66.765 66.765 3.864 M23591#2 catalytic protein phosphatase 2A-beta 228.944 –228.944 –3.916 D83538 230kDa phosphatidylinositol 4-kinase –35.392 35.392 2.266 Oxidization and reduction S45812 monoamine oxidase A 65.823 –65.823 –4.234 U75927UTR#1 cytochrome oxidase subunit VIIa 41.571 –41.571 –4.632 L48209 cytochrome c oxidase subunit VIII (COX-VIII) 386.258 –386.258 –4.105 D00688 monoamine oxidase A 115.533 –115.533 –4.675 X60328 cytosolic epoxide hydrolase 49.658 –49.658 –5.367 AA686031 NGF-treated similar to NADH-ubiquinone oxidoreductase 75 kDa subunit–34.798 34.798 3.78
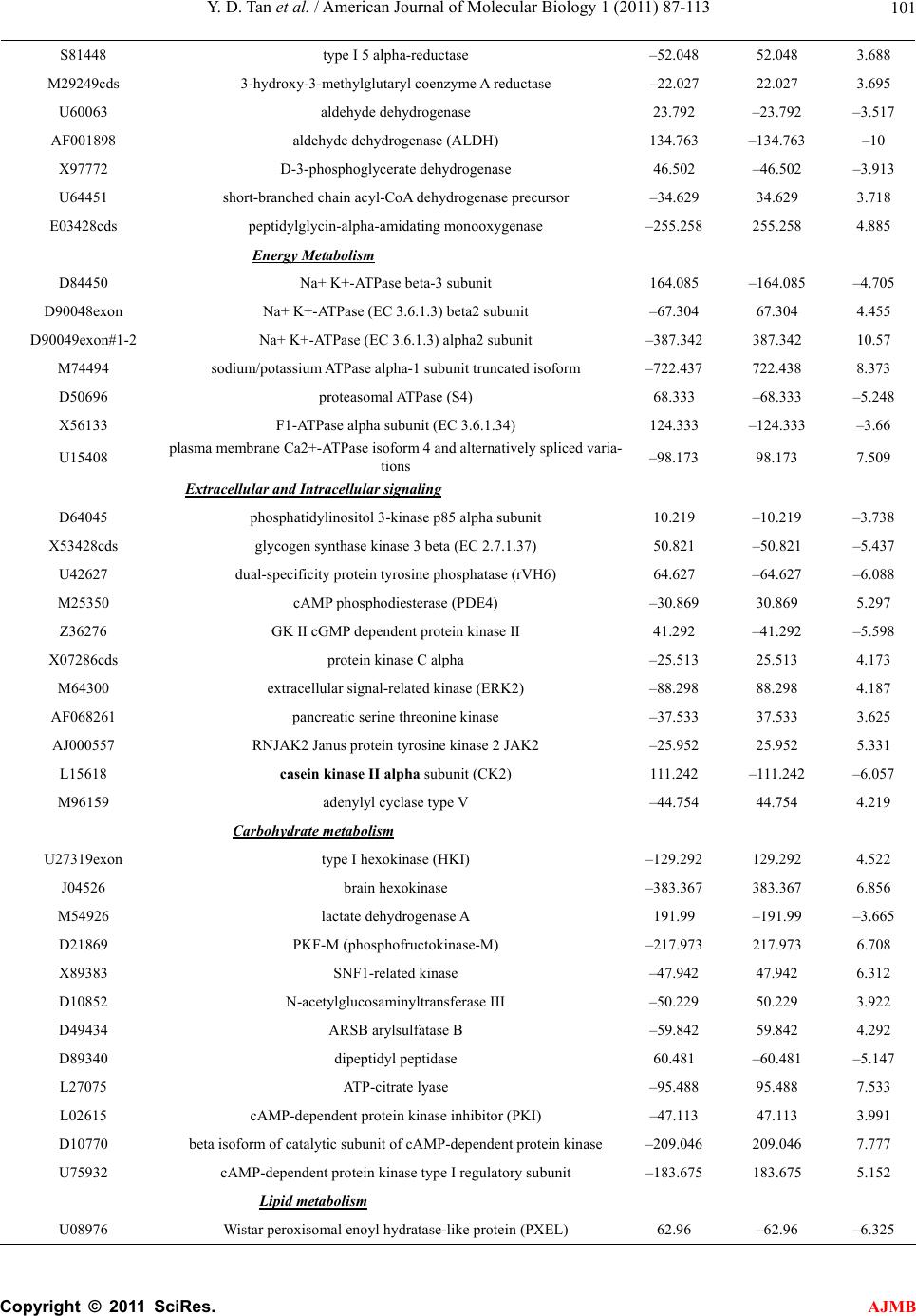 Y. D. Tan et al. / American Journal of Molecular Biology 1 (2011) 87-113 Copyright © 2011 SciRes. AJMB 101 S81448 type I 5 alpha-reductase –52.048 52.048 3.688 M29249cds 3-hydroxy-3-methylglutaryl coenzyme A reductase –22.027 22.027 3.695 U60063 aldehyde dehydrogenase 23.792 –23.792 –3.517 AF001898 aldehyde dehydrogenase (ALDH) 134.763 –134.763 –10 X97772 D-3-phosphoglycerate dehydrogenase 46.502 –46.502 –3.913 U64451 short-branched chain acyl-CoA dehydrogenase precursor –34.629 34.629 3.718 E03428cds peptidylglycin-alpha-amidating monooxygenase –255.258 255.258 4.885 Energy Metabolism D84450 Na+ K+-ATPase beta-3 subunit 164.085 –164.085 –4.705 D90048exon Na+ K+-ATPase (EC 3.6.1.3) beta2 subunit –67.304 67.304 4.455 D90049exon#1-2 Na+ K+-ATPase (EC 3.6.1.3) alpha2 subunit –387.342 387.342 10.57 M74494 sodium/potassium ATPase alpha-1 subunit truncated isoform –722.437 722.438 8.373 D50696 proteasomal ATPase (S4) 68.333 –68.333 –5.248 X56133 F1-ATPase alpha subunit (EC 3.6.1.34) 124.333 –124.333 –3.66 U15408 plasma membrane Ca2+-ATPase isoform 4 and alternatively spliced varia- tions –98.173 98.173 7.509 Extracellular and Intracellular signaling D64045 phosphatidylinositol 3-kinase p85 alpha subunit 10.219 –10.219 –3.738 X53428cds glycogen synthase kinase 3 beta (EC 2.7.1.37) 50.821 –50.821 –5.437 U42627 dual-specificity protein tyrosine phosphatase (rVH6) 64.627 –64.627 –6.088 M25350 cAMP phosphodiesterase (PDE4) –30.869 30.869 5.297 Z36276 GK II cGMP dependent protein kinase II 41.292 –41.292 –5.598 X07286cds protein kinase C alpha –25.513 25.513 4.173 M64300 extracellular signal-related kinase (ERK2) –88.298 88.298 4.187 AF068261 pancreatic serine threonine kinase –37.533 37.533 3.625 AJ000557 RNJAK2 Janus protein tyrosine kinase 2 JAK2 –25.952 25.952 5.331 L15618 casein kinase II alpha subunit (CK2) 111.242 –111.242 –6.057 M96159 adenylyl cyclase type V –44.754 44.754 4.219 Carbohydrate metabolism U27319exon type I hexokinase (HKI) –129.292 129.292 4.522 J04526 brain hexokinase –383.367 383.367 6.856 M54926 lactate dehydrogenase A 191.99 –191.99 –3.665 D21869 PKF-M (phosphofructokinase-M) –217.973 217.973 6.708 X89383 SNF1-related kinase –47.942 47.942 6.312 D10852 N-acetylglucosaminyltransferase III –50.229 50.229 3.922 D49434 ARSB arylsulfatase B –59.842 59.842 4.292 D89340 dipeptidyl peptidase 60.481 –60.481 –5.147 L27075 ATP-citrate lyase –95.488 95.488 7.533 L02615 cAMP-dependent protein kinase inhibitor (PKI) –47.113 47.113 3.991 D10770 beta isoform of catalytic subunit of cAMP-dependent protein kinase –209.046 209.046 7.777 U75932 cAMP-dependent protein kinase type I regulatory subunit –183.675 183.675 5.152 Lipid metabolism U08976 Wistar peroxisomal enoyl hydratase-like protein (PXEL) 62.96 –62.96 –6.325
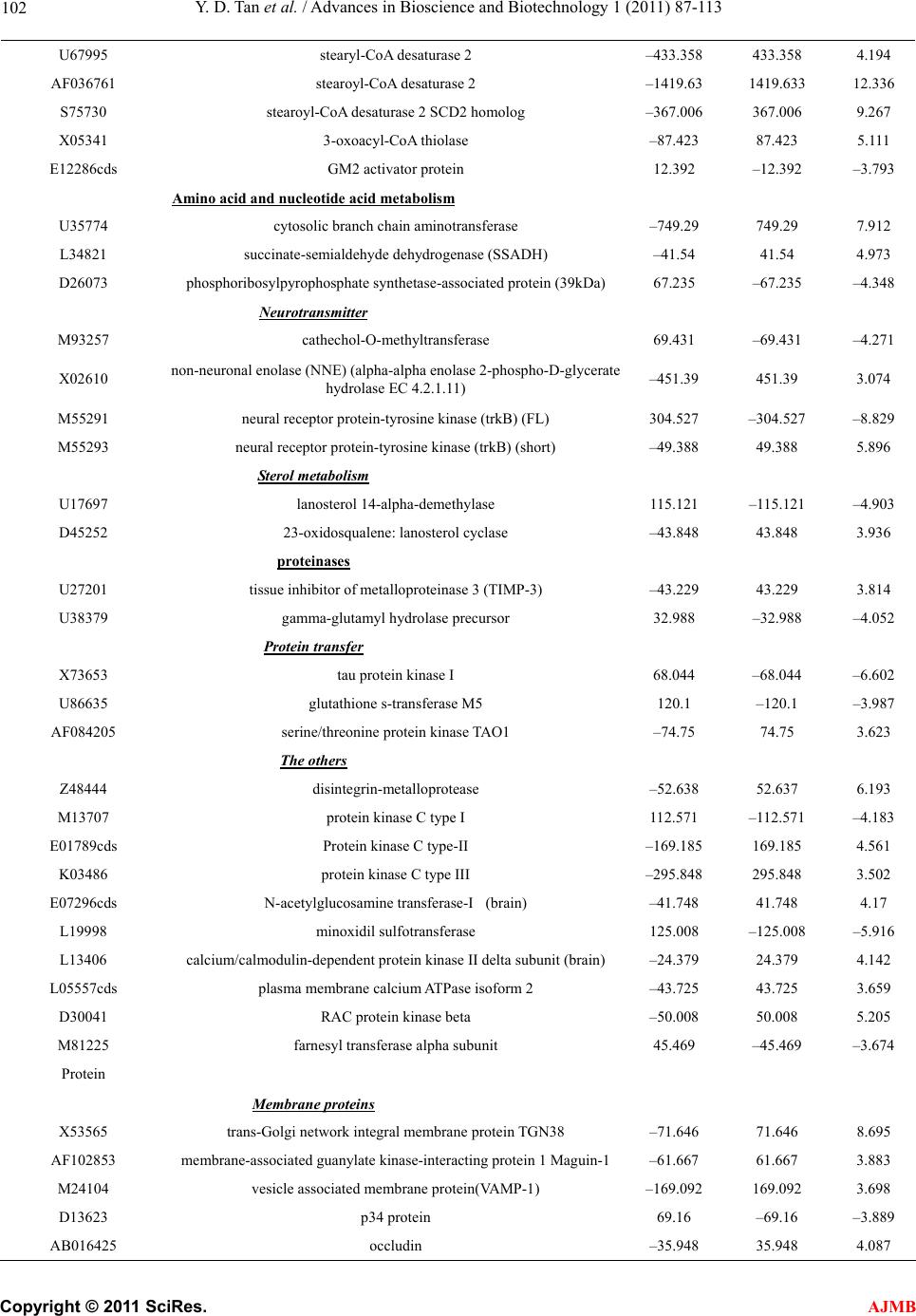 Y. D. Tan et al. / Advances in Bioscience and Biotechnology 1 (2011) 87-113 Copyright © 2011 SciRes. AJMB 102 U67995 stearyl-CoA desaturase 2 –433.358 433.358 4.194 AF036761 stearoyl-CoA desaturase 2 –1419.63 1419.633 12.336 S75730 stearoyl-CoA desaturase 2 SCD2 homolog –367.006 367.006 9.267 X05341 3-oxoacyl-CoA thiolase –87.423 87.423 5.111 E12286cds GM2 activator protein 12.392 –12.392 –3.793 Amino acid and nucleotide acid metabolism U35774 cytosolic branch chain aminotransferase –749.29 749.29 7.912 L34821 succinate-semialdehyde dehydrogenase (SSADH) –41.54 41.54 4.973 D26073 phosphoribosylpyrophosphate synthetase-associated protein (39kDa) 67.235 –67.235 –4.348 Neurotransmitter M93257 cathechol-O-methyltransferase 69.431 –69.431 –4.271 X02610 non-neuronal enolase (NNE) (alpha-alpha enolase 2-phospho-D-glycerate hydrolase EC 4.2.1.11) –451.39 451.39 3.074 M55291 neural receptor protein-tyrosine kinase (trkB) (FL) 304.527 –304.527 –8.829 M55293 neural receptor protein-tyrosine kinase (trkB) (short) –49.388 49.388 5.896 Ster ol metabolism U17697 lanosterol 14-alpha-demethylase 115.121 –115.121 –4.903 D45252 23-oxidosqualene: lanosterol cyclase –43.848 43.848 3.936 proteinases U27201 tissue inhibitor of metalloproteinase 3 (TIMP-3) –43.229 43.229 3.814 U38379 gamma-glutamyl hydrolase precursor 32.988 –32.988 –4.052 Protein transfer X73653 tau protein kinase I 68.044 –68.044 –6.602 U86635 glutathione s-transferase M5 120.1 –120.1 –3.987 AF084205 serine/threonine protein kinase TAO1 –74.75 74.75 3.623 The others Z48444 disintegrin-metalloprotease –52.638 52.637 6.193 M13707 protein kinase C type I 112.571 –112.571 –4.183 E01789cds Protein kinase C type-II –169.185 169.185 4.561 K03486 protein kinase C type III –295.848 295.848 3.502 E07296cds N-acetylglucosamine transferase-I (brain) –41.748 41.748 4.17 L19998 minoxidil sulfotransferase 125.008 –125.008 –5.916 L13406 calcium/calmodulin-dependent protein kinase II delta subunit (brain) –24.379 24.379 4.142 L05557cds plasma membrane calcium ATPase isoform 2 –43.725 43.725 3.659 D30041 RAC protein kinase beta –50.008 50.008 5.205 M81225 farnesyl transferase alpha subunit 45.469 –45.469 –3.674 Protein Membrane prot ei ns X53565 trans-Golgi network integral membrane protein TGN38 –71.646 71.646 8.695 AF102853 membrane-associated guanylate kinase-interacting protein 1 Maguin-1 –61.667 61.667 3.883 M24104 vesicle associated membrane protein(VAMP-1) –169.092 169.092 3.698 D13623 p34 protein 69.16 –69.16 –3.889 AB016425 occludin –35.948 35.948 4.087
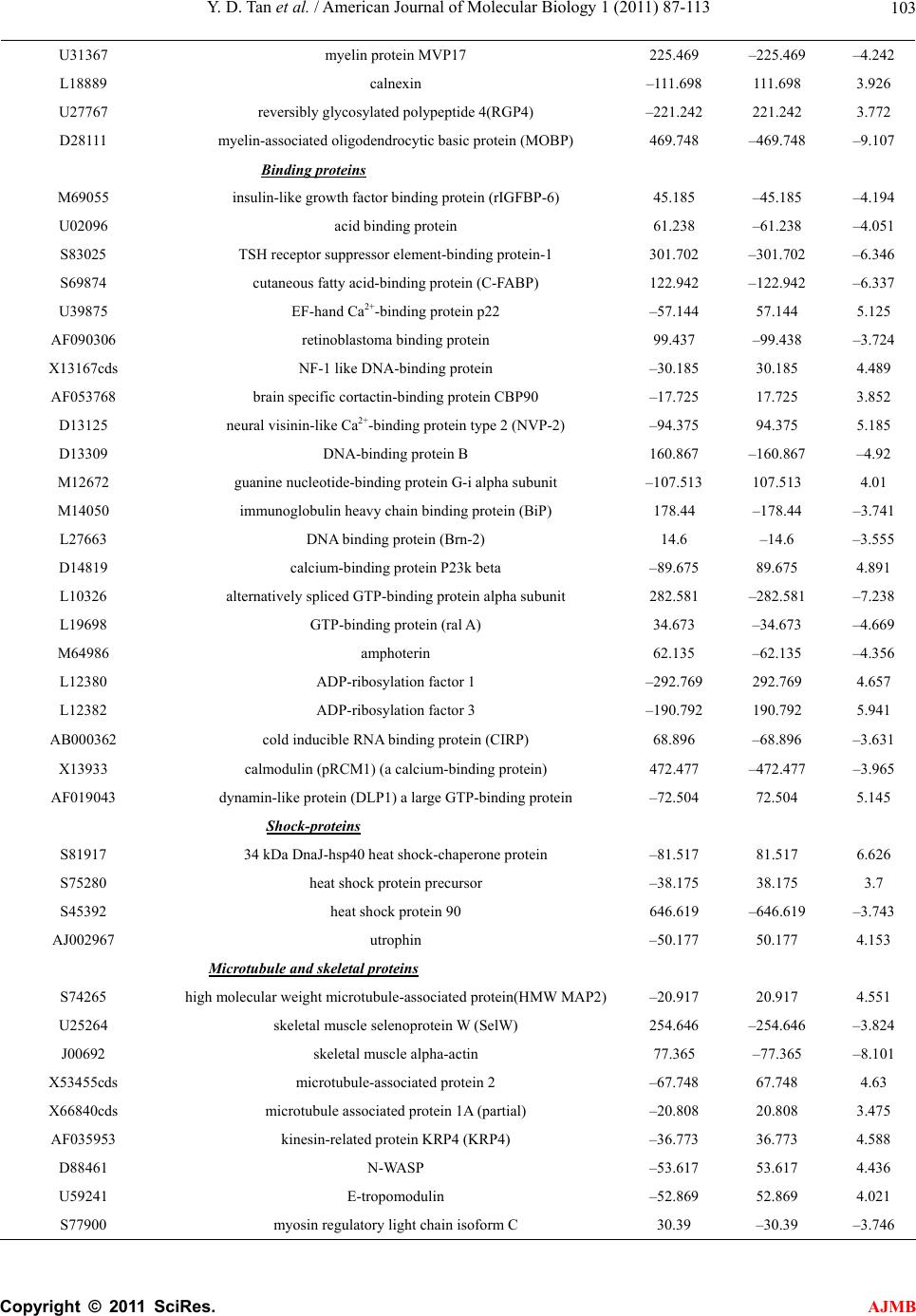 Y. D. Tan et al. / American Journal of Molecular Biology 1 (2011) 87-113 Copyright © 2011 SciRes. AJMB 103 U31367 myelin protein MVP17 225.469 –225.469 –4.242 L18889 calnexin –111.698 111.698 3.926 U27767 reversibly glycosylated polypeptide 4(RGP4) –221.242 221.242 3.772 D28111 myelin-associated oligodendrocytic basic protein (MOBP) 469.748 –469.748 –9.107 Binding proteins M69055 insulin-like growth factor binding protein (rIGFBP-6) 45.185 –45.185 –4.194 U02096 acid binding protein 61.238 –61.238 –4.051 S83025 TSH receptor suppressor element-binding protein-1 301.702 –301.702 –6.346 S69874 cutaneous fatty acid-binding protein (C-FABP) 122.942 –122.942 –6.337 U39875 EF-hand Ca2+-binding protein p22 –57.144 57.144 5.125 AF090306 retinoblastoma binding protein 99.437 –99.438 –3.724 X13167cds NF-1 like DNA-binding protein –30.185 30.185 4.489 AF053768 brain specific cortactin-binding protein CBP90 –17.725 17.725 3.852 D13125 neural visinin-like Ca2+-binding protein type 2 (NVP-2) –94.375 94.375 5.185 D13309 DNA-binding protein B 160.867 –160.867 –4.92 M12672 guanine nucleotide-binding protein G-i alpha subunit –107.513 107.513 4.01 M14050 immunoglobulin heavy chain binding protein (BiP) 178.44 –178.44 –3.741 L27663 DNA binding protein (Brn-2) 14.6 –14.6 –3.555 D14819 calcium-binding protein P23k beta –89.675 89.675 4.891 L10326 alternatively spliced GTP-binding protein alpha subunit 282.581 –282.581 –7.238 L19698 GTP-binding protein (ral A) 34.673 –34.673 –4.669 M64986 amphoterin 62.135 –62.135 –4.356 L12380 ADP-ribosylation factor 1 –292.769 292.769 4.657 L12382 ADP-ribosylation factor 3 –190.792 190.792 5.941 AB000362 cold inducible RNA binding protein (CIRP) 68.896 –68.896 –3.631 X13933 calmodulin (pRCM1) (a calcium-binding protein) 472.477 –472.477 –3.965 AF019043 dynamin-like protein (DLP1) a large GTP-binding protein –72.504 72.504 5.145 Shock-proteins S81917 34 kDa DnaJ-hsp40 heat shock-chaperone protein –81.517 81.517 6.626 S75280 heat shock protein precursor –38.175 38.175 3.7 S45392 heat shock protein 90 646.619 –646.619 –3.743 AJ002967 utrophin –50.177 50.177 4.153 Microtubule and s ke l e tal proteins S74265 high molecular weight microtubule-associated protein(HMW MAP2) –20.917 20.917 4.551 U25264 skeletal muscle selenoprotein W (SelW) 254.646 –254.646 –3.824 J00692 skeletal muscle alpha-actin 77.365 –77.365 –8.101 X53455cds microtubule-associated protein 2 –67.748 67.748 4.63 X66840cds microtubule associated protein 1A (partial) –20.808 20.808 3.475 AF035953 kinesin-related protein KRP4 (KRP4) –36.773 36.773 4.588 D88461 N-WASP –53.617 53.617 4.436 U59241 E-tropomodulin –52.869 52.869 4.021 S77900 myosin regulatory light chain isoform C 30.39 –30.39 –3.746
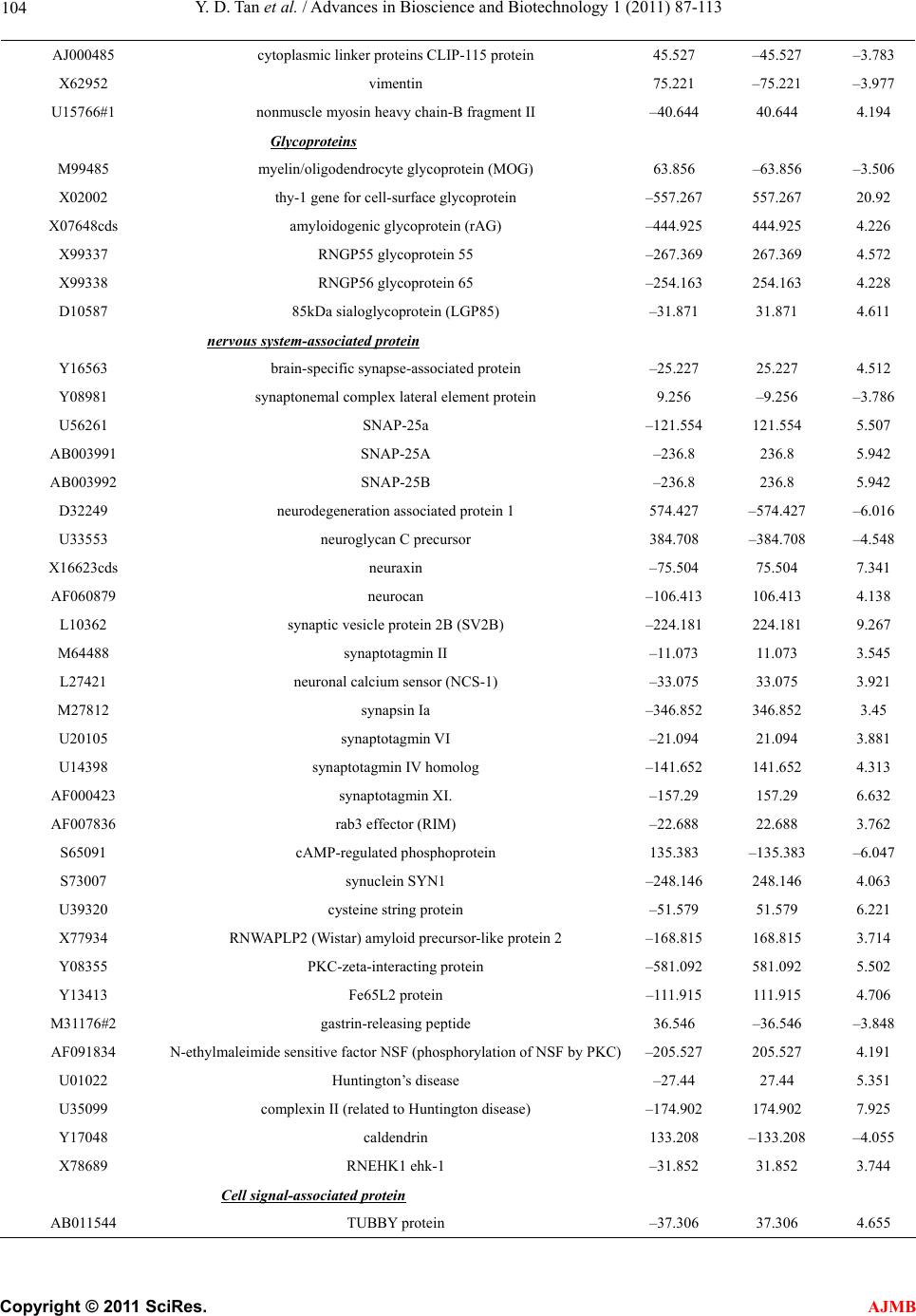 Y. D. Tan et al. / Advances in Bioscience and Biotechnology 1 (2011) 87-113 Copyright © 2011 SciRes. AJMB 104 AJ000485 cytoplasmic linker proteins CLIP-115 protein 45.527 –45.527 –3.783 X62952 vimentin 75.221 –75.221 –3.977 U15766#1 nonmuscle myosin heavy chain-B fragment II –40.644 40.644 4.194 Glycoproteins M99485 myelin/oligodendrocyte glycoprotein (MOG) 63.856 –63.856 –3.506 X02002 thy-1 gene for cell-surface glycoprotein –557.267 557.267 20.92 X07648cds amyloidogenic glycoprotein (rAG) –444.925 444.925 4.226 X99337 RNGP55 glycoprotein 55 –267.369 267.369 4.572 X99338 RNGP56 glycoprotein 65 –254.163 254.163 4.228 D10587 85kDa sialoglycoprotein (LGP85) –31.871 31.871 4.611 nervous system-associated protein Y16563 brain-specific synapse-associated protein –25.227 25.227 4.512 Y08981 synaptonemal complex lateral element protein 9.256 –9.256 –3.786 U56261 SNAP-25a –121.554 121.554 5.507 AB003991 SNAP-25A –236.8 236.8 5.942 AB003992 SNAP-25B –236.8 236.8 5.942 D32249 neurodegeneration associated protein 1 574.427 –574.427 –6.016 U33553 neuroglycan C precursor 384.708 –384.708 –4.548 X16623cds neuraxin –75.504 75.504 7.341 AF060879 neurocan –106.413 106.413 4.138 L10362 synaptic vesicle protein 2B (SV2B) –224.181 224.181 9.267 M64488 synaptotagmin II –11.073 11.073 3.545 L27421 neuronal calcium sensor (NCS-1) –33.075 33.075 3.921 M27812 synapsin Ia –346.852 346.852 3.45 U20105 synaptotagmin VI –21.094 21.094 3.881 U14398 synaptotagmin IV homolog –141.652 141.652 4.313 AF000423 synaptotagmin XI. –157.29 157.29 6.632 AF007836 rab3 effector (RIM) –22.688 22.688 3.762 S65091 cAMP-regulated phosphoprotein 135.383 –135.383 –6.047 S73007 synuclein SYN1 –248.146 248.146 4.063 U39320 cysteine string protein –51.579 51.579 6.221 X77934 RNWAPLP2 (Wistar) amyloid precursor-like protein 2 –168.815 168.815 3.714 Y08355 PKC-zeta-interacting protein –581.092 581.092 5.502 Y13413 Fe65L2 protein –111.915 111.915 4.706 M31176#2 gastrin-releasing peptide 36.546 –36.546 –3.848 AF091834 N-ethylmaleimide sensitive factor NSF (phosphorylation of NSF by PKC)–205.527 205.527 4.191 U01022 Huntington’s disease –27.44 27.44 5.351 U35099 complexin II (related to Huntington disease) –174.902 174.902 7.925 Y17048 caldendrin 133.208 –133.208 –4.055 X78689 RNEHK1 ehk-1 –31.852 31.852 3.744 Cell signal-associated protein AB011544 TUBBY protein –37.306 37.306 4.655
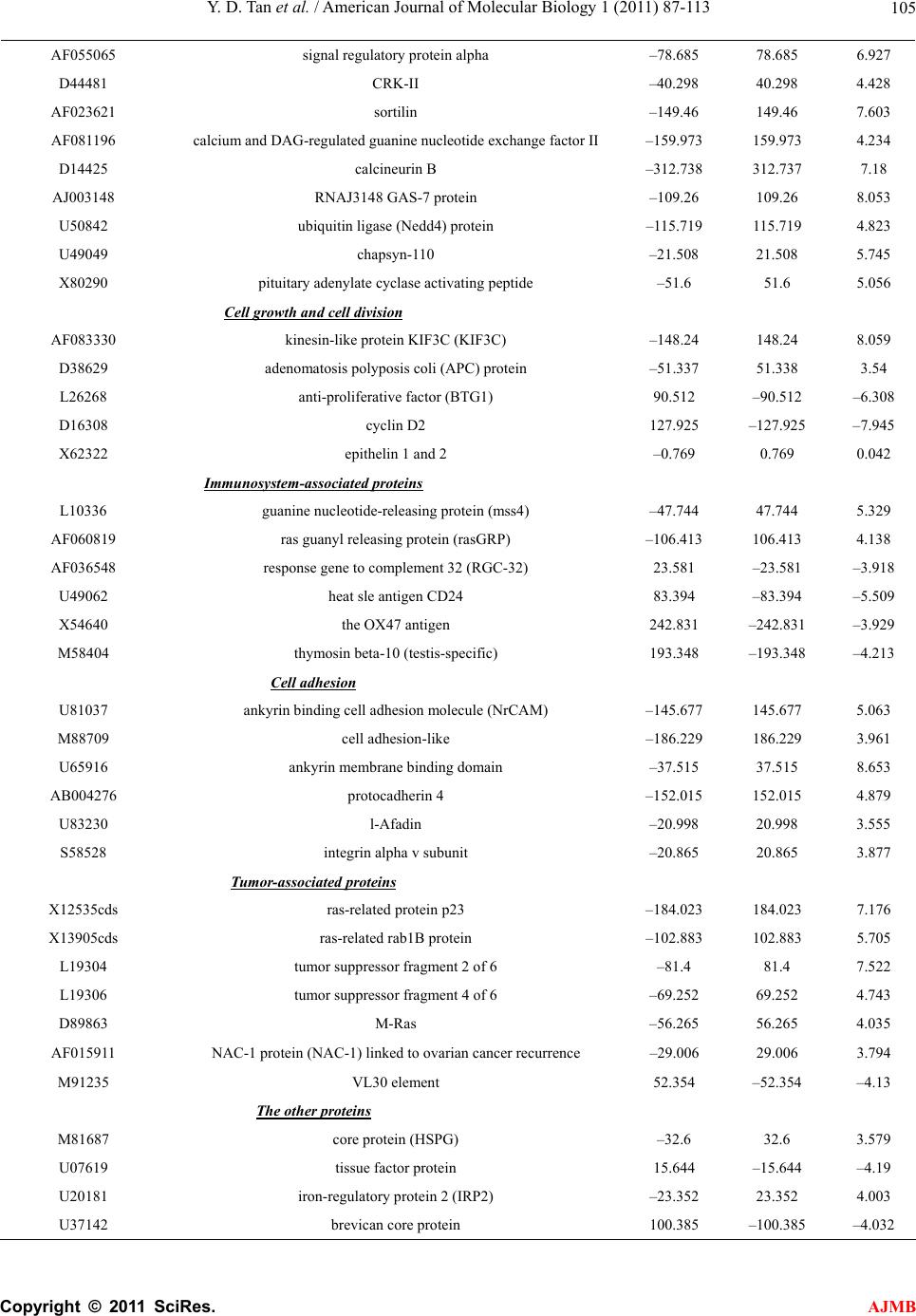 Y. D. Tan et al. / American Journal of Molecular Biology 1 (2011) 87-113 Copyright © 2011 SciRes. AJMB 105 AF055065 signal regulatory protein alpha –78.685 78.685 6.927 D44481 CRK-II –40.298 40.298 4.428 AF023621 sortilin –149.46 149.46 7.603 AF081196 calcium and DAG-regulated guanine nucleotide exchange factor II –159.973 159.973 4.234 D14425 calcineurin B –312.738 312.737 7.18 AJ003148 RNAJ3148 GAS-7 protein –109.26 109.26 8.053 U50842 ubiquitin ligase (Nedd4) protein –115.719 115.719 4.823 U49049 chapsyn-110 –21.508 21.508 5.745 X80290 pituitary adenylate cyclase activating peptide –51.6 51.6 5.056 Cell growth and cell divi si on AF083330 kinesin-like protein KIF3C (KIF3C) –148.24 148.24 8.059 D38629 adenomatosis polyposis coli (APC) protein –51.337 51.338 3.54 L26268 anti-proliferative factor (BTG1) 90.512 –90.512 –6.308 D16308 cyclin D2 127.925 –127.925 –7.945 X62322 epithelin 1 and 2 –0.769 0.769 0.042 Immunosystem-associated proteins L10336 guanine nucleotide-releasing protein (mss4) –47.744 47.744 5.329 AF060819 ras guanyl releasing protein (rasGRP) –106.413 106.413 4.138 AF036548 response gene to complement 32 (RGC-32) 23.581 –23.581 –3.918 U49062 heat sle antigen CD24 83.394 –83.394 –5.509 X54640 the OX47 antigen 242.831 –242.831 –3.929 M58404 thymosin beta-10 (testis-specific) 193.348 –193.348 –4.213 Cell adhesion U81037 ankyrin binding cell adhesion molecule (NrCAM) –145.677 145.677 5.063 M88709 cell adhesion-like –186.229 186.229 3.961 U65916 ankyrin membrane binding domain –37.515 37.515 8.653 AB004276 protocadherin 4 –152.015 152.015 4.879 U83230 l-Afadin –20.998 20.998 3.555 S58528 integrin alpha v subunit –20.865 20.865 3.877 Tumor-associated proteins X12535cds ras-related protein p23 –184.023 184.023 7.176 X13905cds ras-related rab1B protein –102.883 102.883 5.705 L19304 tumor suppressor fragment 2 of 6 –81.4 81.4 7.522 L19306 tumor suppressor fragment 4 of 6 –69.252 69.252 4.743 D89863 M-Ras –56.265 56.265 4.035 AF015911 NAC-1 protein (NAC-1) linked to ovarian cancer recurrence –29.006 29.006 3.794 M91235 VL30 element 52.354 –52.354 –4.13 The other proteins M81687 core protein (HSPG) –32.6 32.6 3.579 U07619 tissue factor protein 15.644 –15.644 –4.19 U20181 iron-regulatory protein 2 (IRP2) –23.352 23.352 4.003 U37142 brevican core protein 100.385 –100.385 –4.032
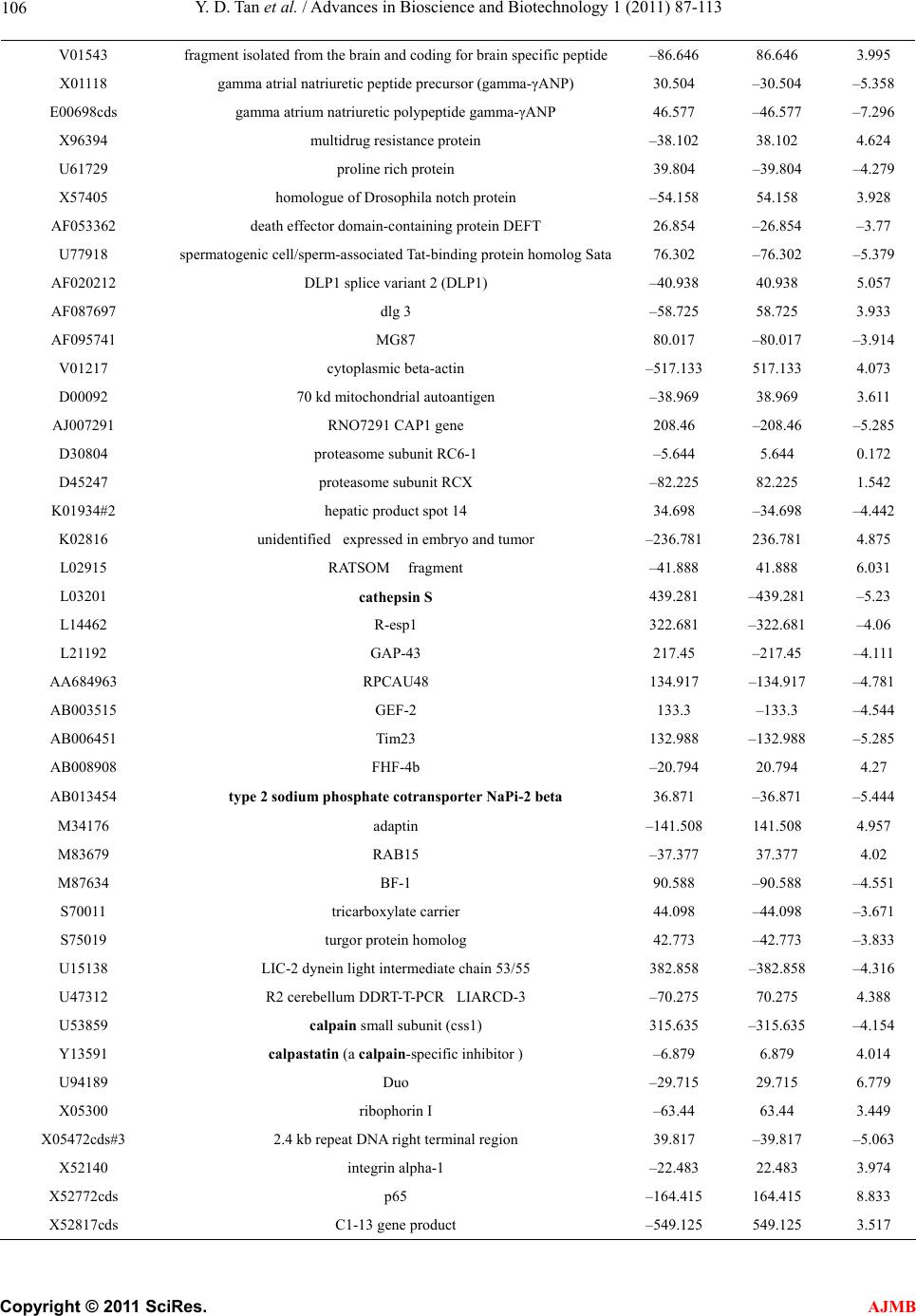 Y. D. Tan et al. / Advances in Bioscience and Biotechnology 1 (2011) 87-113 Copyright © 2011 SciRes. AJMB 106 V01543 fragment isolated from the brain and coding for brain specific peptide –86.646 86.646 3.995 X01118 gamma atrial natriuretic peptide precursor (gamma-γANP) 30.504 –30.504 –5.358 E00698cds gamma atrium natriuretic polypeptide gamma-γANP 46.577 –46.577 –7.296 X96394 multidrug resistance protein –38.102 38.102 4.624 U61729 proline rich protein 39.804 –39.804 –4.279 X57405 homologue of Drosophila notch protein –54.158 54.158 3.928 AF053362 death effector domain-containing protein DEFT 26.854 –26.854 –3.77 U77918 spermatogenic cell/sperm-associated Tat-binding protein homolog Sata76.302 –76.302 –5.379 AF020212 DLP1 splice variant 2 (DLP1) –40.938 40.938 5.057 AF087697 dlg 3 –58.725 58.725 3.933 AF095741 MG87 80.017 –80.017 –3.914 V01217 cytoplasmic beta-actin –517.133 517.133 4.073 D00092 70 kd mitochondrial autoantigen –38.969 38.969 3.611 AJ007291 RNO7291 CAP1 gene 208.46 –208.46 –5.285 D30804 proteasome subunit RC6-1 –5.644 5.644 0.172 D45247 proteasome subunit RCX –82.225 82.225 1.542 K01934#2 hepatic product spot 14 34.698 –34.698 –4.442 K02816 unidentified expressed in embryo and tumor –236.781 236.781 4.875 L02915 RATSOM fragment –41.888 41.888 6.031 L03201 cathepsin S 439.281 –439.281 –5.23 L14462 R-esp1 322.681 –322.681 –4.06 L21192 GAP-43 217.45 –217.45 –4.111 AA684963 RPCAU48 134.917 –134.917 –4.781 AB003515 GEF-2 133.3 –133.3 –4.544 AB006451 Tim23 132.988 –132.988 –5.285 AB008908 FHF-4b –20.794 20.794 4.27 AB013454 type 2 sodium phosphate cotransporter NaPi-2 beta 36.871 –36.871 –5.444 M34176 adaptin –141.508 141.508 4.957 M83679 RAB15 –37.377 37.377 4.02 M87634 BF-1 90.588 –90.588 –4.551 S70011 tricarboxylate carrier 44.098 –44.098 –3.671 S75019 turgor protein homolog 42.773 –42.773 –3.833 U15138 LIC-2 dynein light intermediate chain 53/55 382.858 –382.858 –4.316 U47312 R2 cerebellum DDRT-T-PCR LIARCD-3 –70.275 70.275 4.388 U53859 calpain small subunit (css1) 315.635 –315.635 –4.154 Y13591 calpastatin (a calpain-specific inhibitor ) –6.879 6.879 4.014 U94189 Duo –29.715 29.715 6.779 X05300 ribophorin I –63.44 63.44 3.449 X05472cds#3 2.4 kb repeat DNA right terminal region 39.817 –39.817 –5.063 X52140 integrin alpha-1 –22.483 22.483 3.974 X52772cds p65 –164.415 164.415 8.833 X52817cds C1-13 gene product –549.125 549.125 3.517
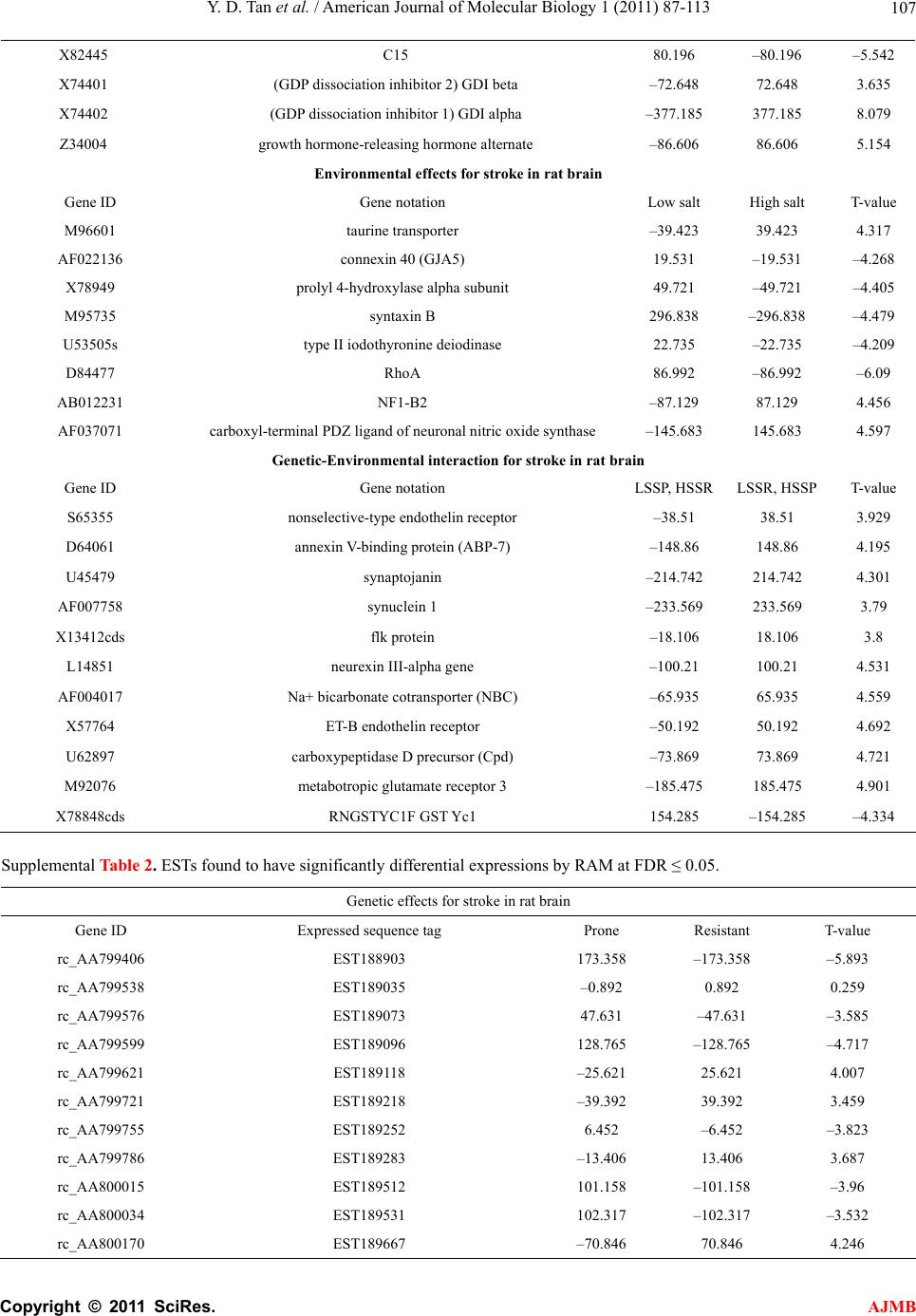 Y. D. Tan et al. / American Journal of Molecular Biology 1 (2011) 87-113 Copyright © 2011 SciRes. AJMB 107 X82445 C15 80.196 –80.196 –5.542 X74401 (GDP dissociation inhibitor 2) GDI beta –72.648 72.648 3.635 X74402 (GDP dissociation inhibitor 1) GDI alpha –377.185 377.185 8.079 Z34004 growth hormone-releasing hormone alternate –86.606 86.606 5.154 Environmental effects for stroke in rat brain Gene ID Gene notation Low salt High salt T-value M96601 taurine transporter –39.423 39.423 4.317 AF022136 connexin 40 (GJA5) 19.531 –19.531 –4.268 X78949 prolyl 4-hydroxylase alpha subunit 49.721 –49.721 –4.405 M95735 syntaxin B 296.838 –296.838 –4.479 U53505s type II iodothyronine deiodinase 22.735 –22.735 –4.209 D84477 RhoA 86.992 –86.992 –6.09 AB012231 NF1-B2 –87.129 87.129 4.456 AF037071 carboxyl-terminal PDZ ligand of neuronal nitric oxide synthase –145.683 145.683 4.597 Genetic-Environmental interaction for stroke in rat brain Gene ID Gene notation LSSP, HSSR LSSR, HSSP T-value S65355 nonselective-type endothelin receptor –38.51 38.51 3.929 D64061 annexin V-binding protein (ABP-7) –148.86 148.86 4.195 U45479 synaptojanin –214.742 214.742 4.301 AF007758 synuclein 1 –233.569 233.569 3.79 X13412cds flk protein –18.106 18.106 3.8 L14851 neurexin III-alpha gene –100.21 100.21 4.531 AF004017 Na+ bicarbonate cotransporter (NBC) –65.935 65.935 4.559 X57764 ET-B endothelin receptor –50.192 50.192 4.692 U62897 carboxypeptidase D precursor (Cpd) –73.869 73.869 4.721 M92076 metabotropic glutamate receptor 3 –185.475 185.475 4.901 X78848cds RNGSTYC1F GST Yc1 154.285 –154.285 –4.334 Supplemental Table 2. ESTs found to have significantly differential expressions by RAM at FDR ≤ 0.05. Genetic effects for stroke in rat brain Gene ID Expressed sequence tag Prone Resistant T-value rc_AA799406 EST188903 173.358 –173.358 –5.893 rc_AA799538 EST189035 –0.892 0.892 0.259 rc_AA799576 EST189073 47.631 –47.631 –3.585 rc_AA799599 EST189096 128.765 –128.765 –4.717 rc_AA799621 EST189118 –25.621 25.621 4.007 rc_AA799721 EST189218 –39.392 39.392 3.459 rc_AA799755 EST189252 6.452 –6.452 –3.823 rc_AA799786 EST189283 –13.406 13.406 3.687 rc_AA800015 EST189512 101.158 –101.158 –3.96 rc_AA800034 EST189531 102.317 –102.317 –3.532 rc_AA800170 EST189667 –70.846 70.846 4.246
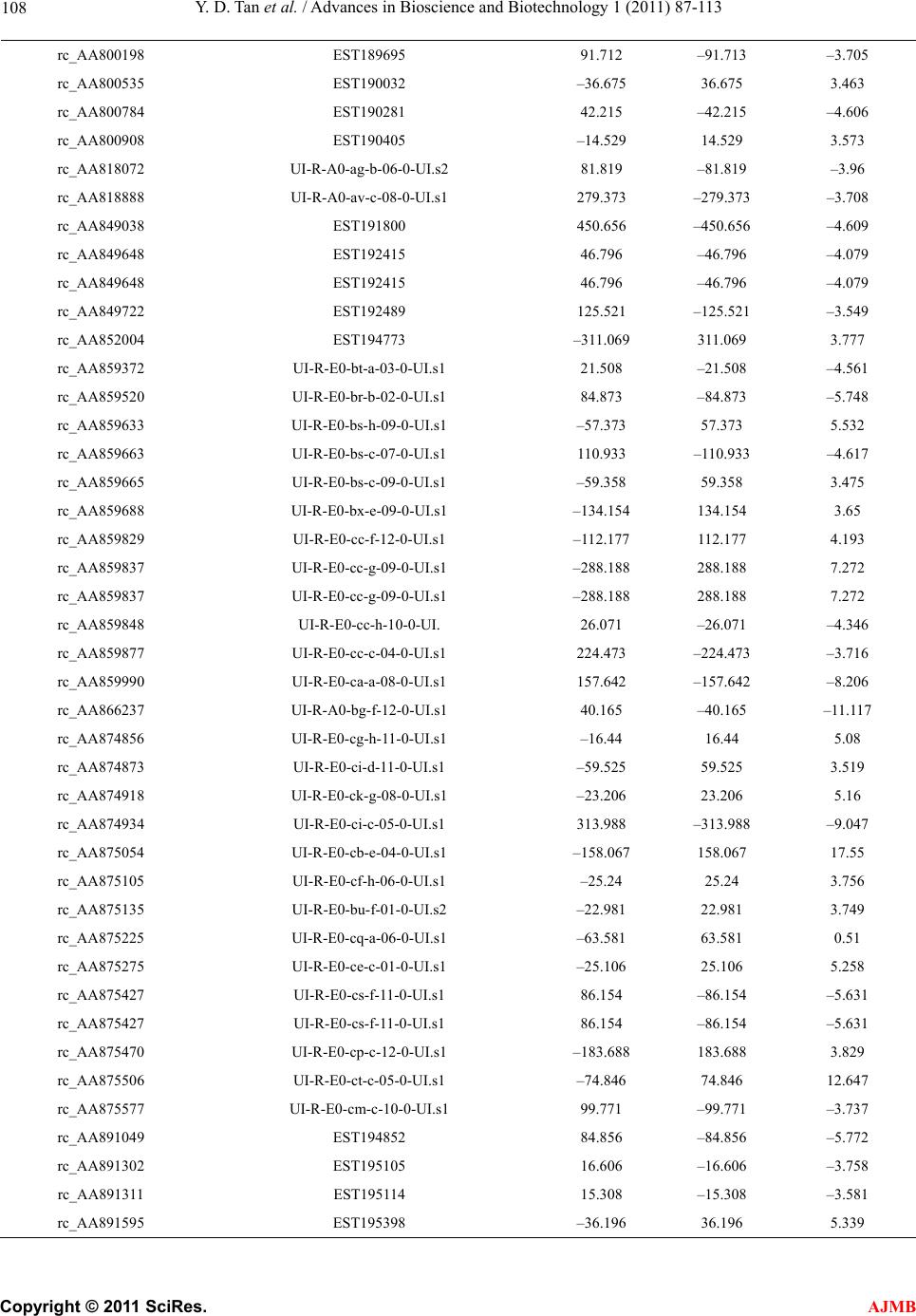 Y. D. Tan et al. / Advances in Bioscience and Biotechnology 1 (2011) 87-113 Copyright © 2011 SciRes. AJMB 108 rc_AA800198 EST189695 91.712 –91.713 –3.705 rc_AA800535 EST190032 –36.675 36.675 3.463 rc_AA800784 EST190281 42.215 –42.215 –4.606 rc_AA800908 EST190405 –14.529 14.529 3.573 rc_AA818072 UI-R-A0-ag-b-06-0-UI.s2 81.819 –81.819 –3.96 rc_AA818888 UI-R-A0-av-c-08-0-UI.s1 279.373 –279.373 –3.708 rc_AA849038 EST191800 450.656 –450.656 –4.609 rc_AA849648 EST192415 46.796 –46.796 –4.079 rc_AA849648 EST192415 46.796 –46.796 –4.079 rc_AA849722 EST192489 125.521 –125.521 –3.549 rc_AA852004 EST194773 –311.069 311.069 3.777 rc_AA859372 UI-R-E0-bt-a-03-0-UI.s1 21.508 –21.508 –4.561 rc_AA859520 UI-R-E0-br-b-02-0-UI.s1 84.873 –84.873 –5.748 rc_AA859633 UI-R-E0-bs-h-09-0-UI.s1 –57.373 57.373 5.532 rc_AA859663 UI-R-E0-bs-c-07-0-UI.s1 110.933 –110.933 –4.617 rc_AA859665 UI-R-E0-bs-c-09-0-UI.s1 –59.358 59.358 3.475 rc_AA859688 UI-R-E0-bx-e-09-0-UI.s1 –134.154 134.154 3.65 rc_AA859829 UI-R-E0-cc-f-12-0-UI.s1 –112.177 112.177 4.193 rc_AA859837 UI-R-E0-cc-g-09-0-UI.s1 –288.188 288.188 7.272 rc_AA859837 UI-R-E0-cc-g-09-0-UI.s1 –288.188 288.188 7.272 rc_AA859848 UI-R-E0-cc-h-10-0-UI. 26.071 –26.071 –4.346 rc_AA859877 UI-R-E0-cc-c-04-0-UI.s1 224.473 –224.473 –3.716 rc_AA859990 UI-R-E0-ca-a-08-0-UI.s1 157.642 –157.642 –8.206 rc_AA866237 UI-R-A0-bg-f-12-0-UI.s1 40.165 –40.165 –11.117 rc_AA874856 UI-R-E0-cg-h-11-0-UI.s1 –16.44 16.44 5.08 rc_AA874873 UI-R-E0-ci-d-11-0-UI.s1 –59.525 59.525 3.519 rc_AA874918 UI-R-E0-ck-g-08-0-UI.s1 –23.206 23.206 5.16 rc_AA874934 UI-R-E0-ci-c-05-0-UI.s1 313.988 –313.988 –9.047 rc_AA875054 UI-R-E0-cb-e-04-0-UI.s1 –158.067 158.067 17.55 rc_AA875105 UI-R-E0-cf-h-06-0-UI.s1 –25.24 25.24 3.756 rc_AA875135 UI-R-E0-bu-f-01-0-UI.s2 –22.981 22.981 3.749 rc_AA875225 UI-R-E0-cq-a-06-0-UI.s1 –63.581 63.581 0.51 rc_AA875275 UI-R-E0-ce-c-01-0-UI.s1 –25.106 25.106 5.258 rc_AA875427 UI-R-E0-cs-f-11-0-UI.s1 86.154 –86.154 –5.631 rc_AA875427 UI-R-E0-cs-f-11-0-UI.s1 86.154 –86.154 –5.631 rc_AA875470 UI-R-E0-cp-c-12-0-UI.s1 –183.688 183.688 3.829 rc_AA875506 UI-R-E0-ct-c-05-0-UI.s1 –74.846 74.846 12.647 rc_AA875577 UI-R-E0-cm-c-10-0-UI.s1 99.771 –99.771 –3.737 rc_AA891049 EST194852 84.856 –84.856 –5.772 rc_AA891302 EST195105 16.606 –16.606 –3.758 rc_AA891311 EST195114 15.308 –15.308 –3.581 rc_AA891595 EST195398 –36.196 36.196 5.339
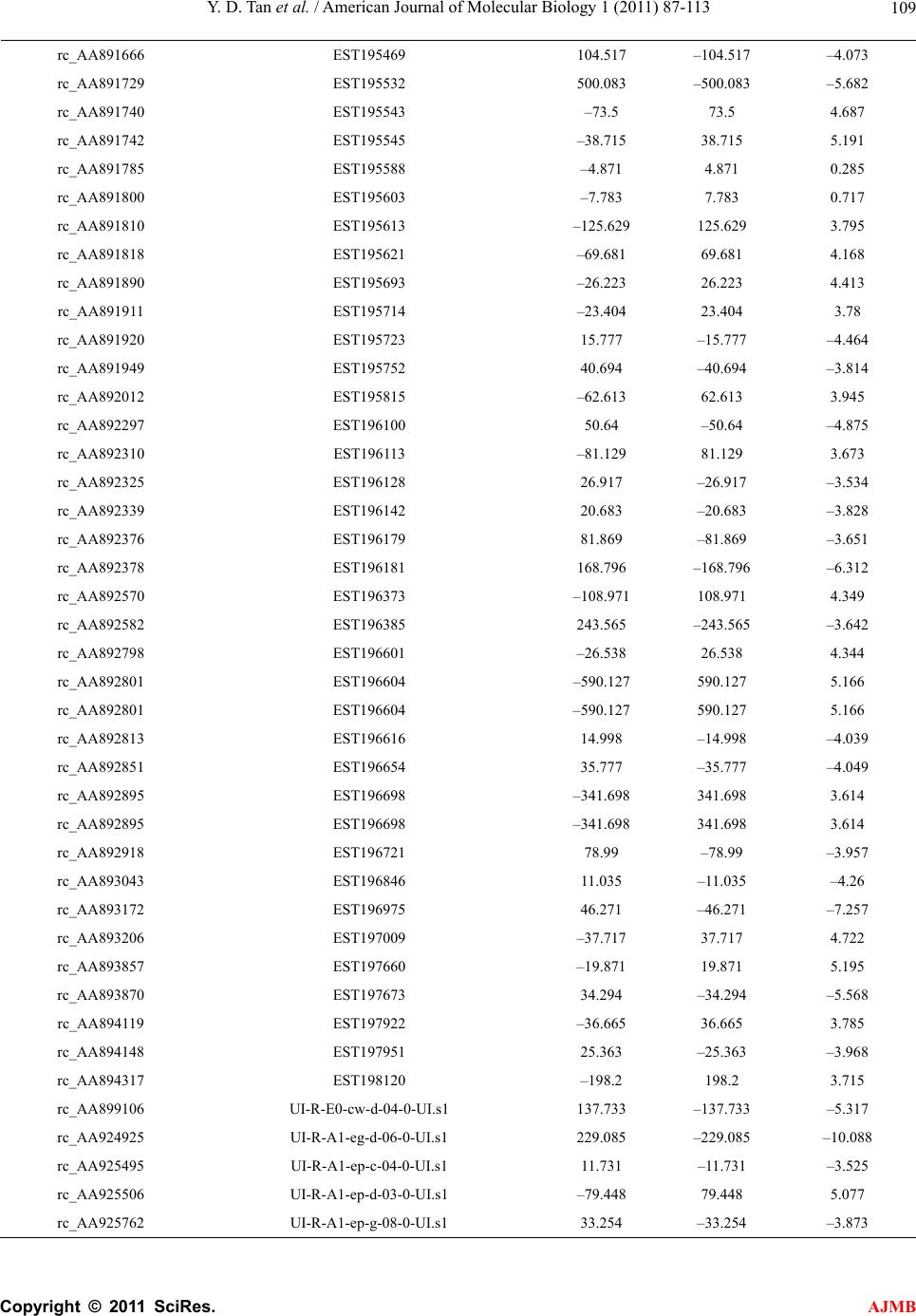 Y. D. Tan et al. / American Journal of Molecular Biology 1 (2011) 87-113 Copyright © 2011 SciRes. AJMB 109 rc_AA891666 EST195469 104.517 –104.517 –4.073 rc_AA891729 EST195532 500.083 –500.083 –5.682 rc_AA891740 EST195543 –73.5 73.5 4.687 rc_AA891742 EST195545 –38.715 38.715 5.191 rc_AA891785 EST195588 –4.871 4.871 0.285 rc_AA891800 EST195603 –7.783 7.783 0.717 rc_AA891810 EST195613 –125.629 125.629 3.795 rc_AA891818 EST195621 –69.681 69.681 4.168 rc_AA891890 EST195693 –26.223 26.223 4.413 rc_AA891911 EST195714 –23.404 23.404 3.78 rc_AA891920 EST195723 15.777 –15.777 –4.464 rc_AA891949 EST195752 40.694 –40.694 –3.814 rc_AA892012 EST195815 –62.613 62.613 3.945 rc_AA892297 EST196100 50.64 –50.64 –4.875 rc_AA892310 EST196113 –81.129 81.129 3.673 rc_AA892325 EST196128 26.917 –26.917 –3.534 rc_AA892339 EST196142 20.683 –20.683 –3.828 rc_AA892376 EST196179 81.869 –81.869 –3.651 rc_AA892378 EST196181 168.796 –168.796 –6.312 rc_AA892570 EST196373 –108.971 108.971 4.349 rc_AA892582 EST196385 243.565 –243.565 –3.642 rc_AA892798 EST196601 –26.538 26.538 4.344 rc_AA892801 EST196604 –590.127 590.127 5.166 rc_AA892801 EST196604 –590.127 590.127 5.166 rc_AA892813 EST196616 14.998 –14.998 –4.039 rc_AA892851 EST196654 35.777 –35.777 –4.049 rc_AA892895 EST196698 –341.698 341.698 3.614 rc_AA892895 EST196698 –341.698 341.698 3.614 rc_AA892918 EST196721 78.99 –78.99 –3.957 rc_AA893043 EST196846 11.035 –11.035 –4.26 rc_AA893172 EST196975 46.271 –46.271 –7.257 rc_AA893206 EST197009 –37.717 37.717 4.722 rc_AA893857 EST197660 –19.871 19.871 5.195 rc_AA893870 EST197673 34.294 –34.294 –5.568 rc_AA894119 EST197922 –36.665 36.665 3.785 rc_AA894148 EST197951 25.363 –25.363 –3.968 rc_AA894317 EST198120 –198.2 198.2 3.715 rc_AA899106 UI-R-E0-cw-d-04-0-UI.s1 137.733 –137.733 –5.317 rc_AA924925 UI-R-A1-eg-d-06-0-UI.s1 229.085 –229.085 –10.088 rc_AA925495 UI-R-A1-ep-c-04-0-UI.s1 11.731 –11.731 –3.525 rc_AA925506 UI-R-A1-ep-d-03-0-UI.s1 –79.448 79.448 5.077 rc_AA925762 UI-R-A1-ep-g-08-0-UI.s1 33.254 –33.254 –3.873
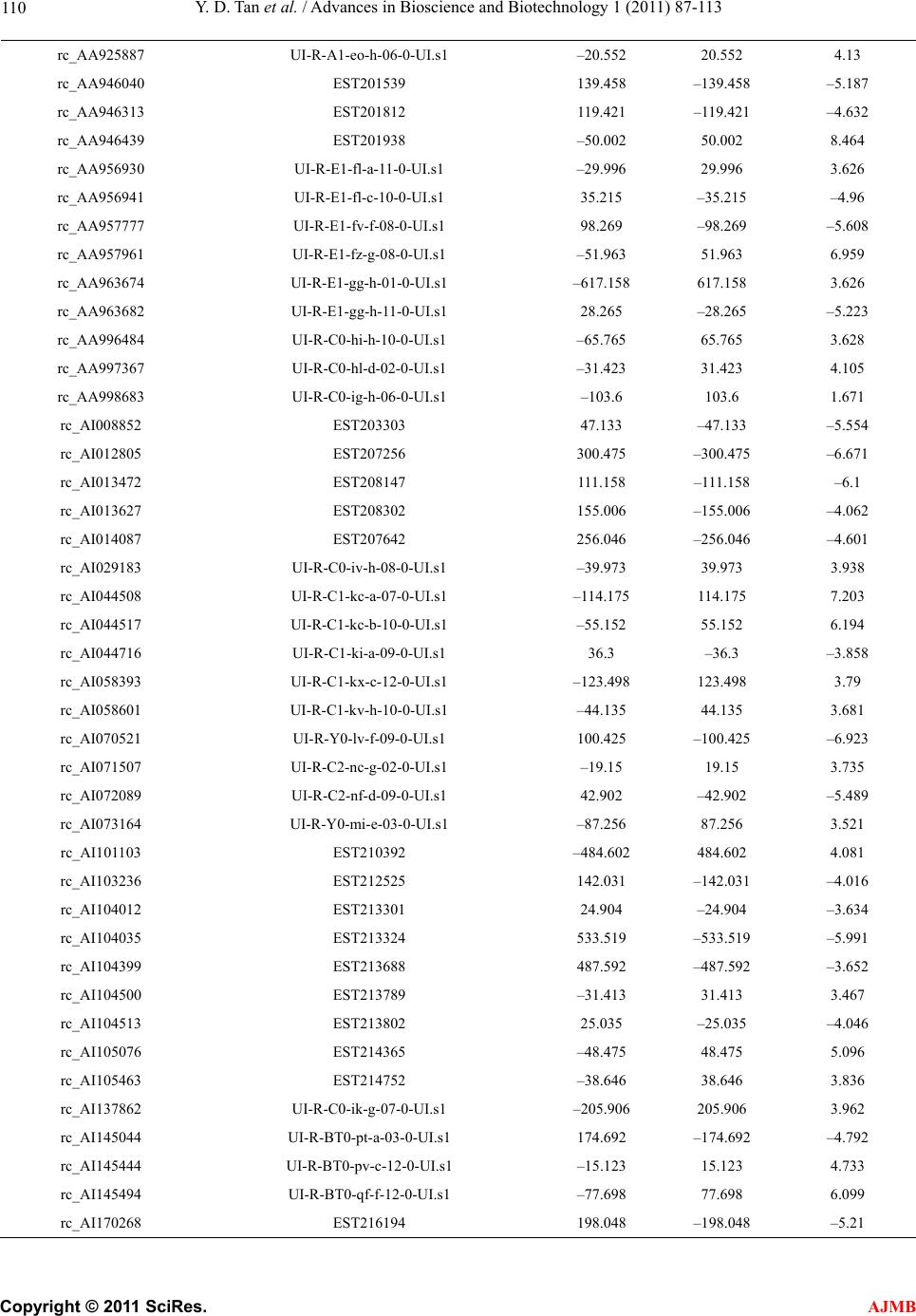 Y. D. Tan et al. / Advances in Bioscience and Biotechnology 1 (2011) 87-113 Copyright © 2011 SciRes. AJMB 110 rc_AA925887 UI-R-A1-eo-h-06-0-UI.s1 –20.552 20.552 4.13 rc_AA946040 EST201539 139.458 –139.458 –5.187 rc_AA946313 EST201812 119.421 –119.421 –4.632 rc_AA946439 EST201938 –50.002 50.002 8.464 rc_AA956930 UI-R-E1-fl-a-11-0-UI.s1 –29.996 29.996 3.626 rc_AA956941 UI-R-E1-fl-c-10-0-UI.s1 35.215 –35.215 –4.96 rc_AA957777 UI-R-E1-fv-f-08-0-UI.s1 98.269 –98.269 –5.608 rc_AA957961 UI-R-E1-fz-g-08-0-UI.s1 –51.963 51.963 6.959 rc_AA963674 UI-R-E1-gg-h-01-0-UI.s1 –617.158 617.158 3.626 rc_AA963682 UI-R-E1-gg-h-11-0-UI.s1 28.265 –28.265 –5.223 rc_AA996484 UI-R-C0-hi-h-10-0-UI.s1 –65.765 65.765 3.628 rc_AA997367 UI-R-C0-hl-d-02-0-UI.s1 –31.423 31.423 4.105 rc_AA998683 UI-R-C0-ig-h-06-0-UI.s1 –103.6 103.6 1.671 rc_AI008852 EST203303 47.133 –47.133 –5.554 rc_AI012805 EST207256 300.475 –300.475 –6.671 rc_AI013472 EST208147 111.158 –111.158 –6.1 rc_AI013627 EST208302 155.006 –155.006 –4.062 rc_AI014087 EST207642 256.046 –256.046 –4.601 rc_AI029183 UI-R-C0-iv-h-08-0-UI.s1 –39.973 39.973 3.938 rc_AI044508 UI-R-C1-kc-a-07-0-UI.s1 –114.175 114.175 7.203 rc_AI044517 UI-R-C1-kc-b-10-0-UI.s1 –55.152 55.152 6.194 rc_AI044716 UI-R-C1-ki-a-09-0-UI.s1 36.3 –36.3 –3.858 rc_AI058393 UI-R-C1-kx-c-12-0-UI.s1 –123.498 123.498 3.79 rc_AI058601 UI-R-C1-kv-h-10-0-UI.s1 –44.135 44.135 3.681 rc_AI070521 UI-R-Y0-lv-f-09-0-UI.s1 100.425 –100.425 –6.923 rc_AI071507 UI-R-C2-nc-g-02-0-UI.s1 –19.15 19.15 3.735 rc_AI072089 UI-R-C2-nf-d-09-0-UI.s1 42.902 –42.902 –5.489 rc_AI073164 UI-R-Y0-mi-e-03-0-UI.s1 –87.256 87.256 3.521 rc_AI101103 EST210392 –484.602 484.602 4.081 rc_AI103236 EST212525 142.031 –142.031 –4.016 rc_AI104012 EST213301 24.904 –24.904 –3.634 rc_AI104035 EST213324 533.519 –533.519 –5.991 rc_AI104399 EST213688 487.592 –487.592 –3.652 rc_AI104500 EST213789 –31.413 31.413 3.467 rc_AI104513 EST213802 25.035 –25.035 –4.046 rc_AI105076 EST214365 –48.475 48.475 5.096 rc_AI105463 EST214752 –38.646 38.646 3.836 rc_AI137862 UI-R-C0-ik-g-07-0-UI.s1 –205.906 205.906 3.962 rc_AI145044 UI-R-BT0-pt-a-03-0-UI.s1 174.692 –174.692 –4.792 rc_AI145444 UI-R-BT0-pv-c-12-0-UI.s1 –15.123 15.123 4.733 rc_AI145494 UI-R-BT0-qf-f-12-0-UI.s1 –77.698 77.698 6.099 rc_AI170268 EST216194 198.048 –198.048 –5.21
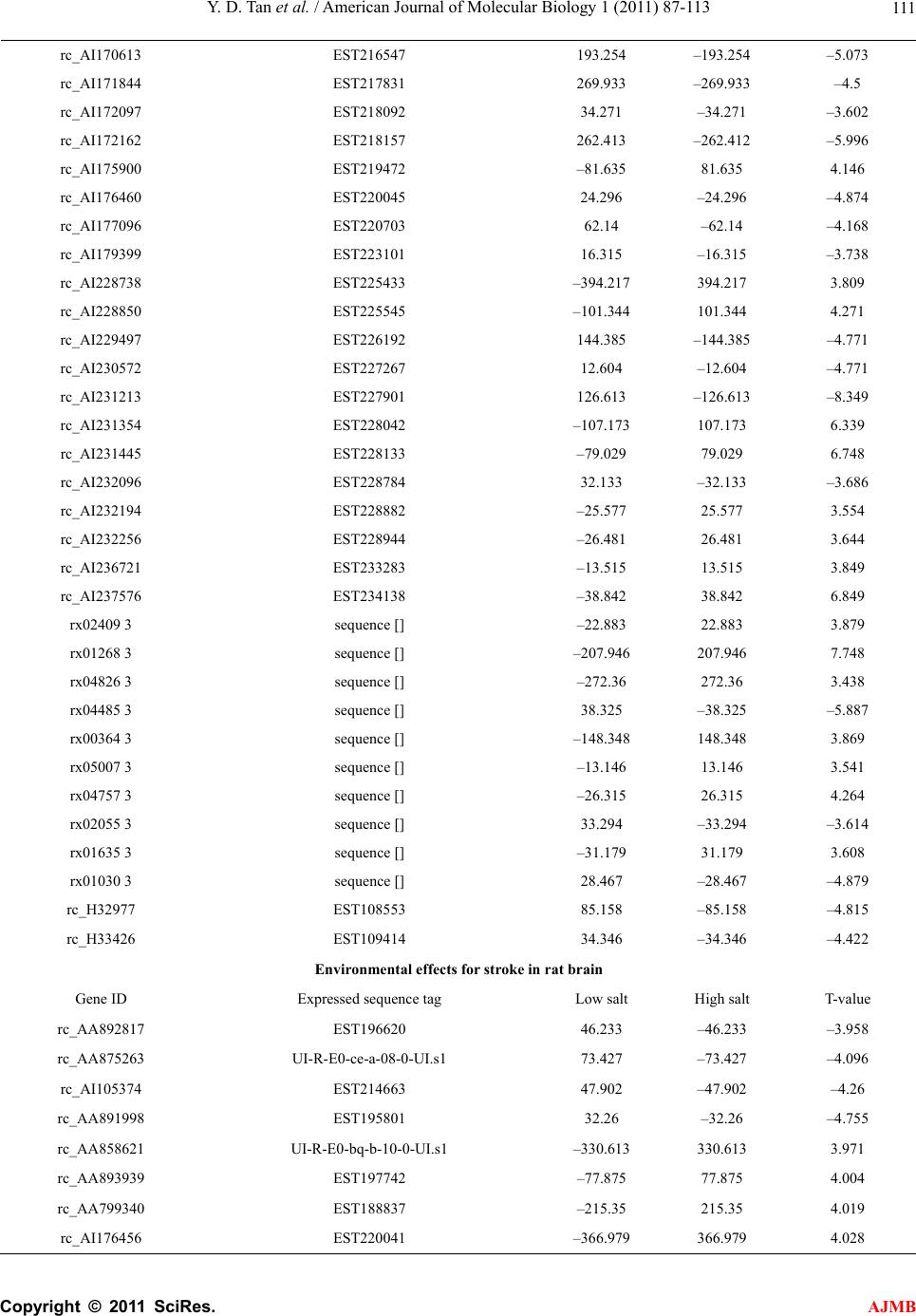 Y. D. Tan et al. / American Journal of Molecular Biology 1 (2011) 87-113 Copyright © 2011 SciRes. AJMB 111 rc_AI170613 EST216547 193.254 –193.254 –5.073 rc_AI171844 EST217831 269.933 –269.933 –4.5 rc_AI172097 EST218092 34.271 –34.271 –3.602 rc_AI172162 EST218157 262.413 –262.412 –5.996 rc_AI175900 EST219472 –81.635 81.635 4.146 rc_AI176460 EST220045 24.296 –24.296 –4.874 rc_AI177096 EST220703 62.14 –62.14 –4.168 rc_AI179399 EST223101 16.315 –16.315 –3.738 rc_AI228738 EST225433 –394.217 394.217 3.809 rc_AI228850 EST225545 –101.344 101.344 4.271 rc_AI229497 EST226192 144.385 –144.385 –4.771 rc_AI230572 EST227267 12.604 –12.604 –4.771 rc_AI231213 EST227901 126.613 –126.613 –8.349 rc_AI231354 EST228042 –107.173 107.173 6.339 rc_AI231445 EST228133 –79.029 79.029 6.748 rc_AI232096 EST228784 32.133 –32.133 –3.686 rc_AI232194 EST228882 –25.577 25.577 3.554 rc_AI232256 EST228944 –26.481 26.481 3.644 rc_AI236721 EST233283 –13.515 13.515 3.849 rc_AI237576 EST234138 –38.842 38.842 6.849 rx02409 3 sequence [] –22.883 22.883 3.879 rx01268 3 sequence [] –207.946 207.946 7.748 rx04826 3 sequence [] –272.36 272.36 3.438 rx04485 3 sequence [] 38.325 –38.325 –5.887 rx00364 3 sequence [] –148.348 148.348 3.869 rx05007 3 sequence [] –13.146 13.146 3.541 rx04757 3 sequence [] –26.315 26.315 4.264 rx02055 3 sequence [] 33.294 –33.294 –3.614 rx01635 3 sequence [] –31.179 31.179 3.608 rx01030 3 sequence [] 28.467 –28.467 –4.879 rc_H32977 EST108553 85.158 –85.158 –4.815 rc_H33426 EST109414 34.346 –34.346 –4.422 Environmental effects for stroke in rat brain Gene ID Expressed sequence tag Low salt High salt T-value rc_AA892817 EST196620 46.233 –46.233 –3.958 rc_AA875263 UI-R-E0-ce-a-08-0-UI.s1 73.427 –73.427 –4.096 rc_AI105374 EST214663 47.902 –47.902 –4.26 rc_AA891998 EST195801 32.26 –32.26 –4.755 rc_AA858621 UI-R-E0-bq-b-10-0-UI.s1 –330.613 330.613 3.971 rc_AA893939 EST197742 –77.875 77.875 4.004 rc_AA799340 EST188837 –215.35 215.35 4.019 rc_AI176456 EST220041 –366.979 366.979 4.028
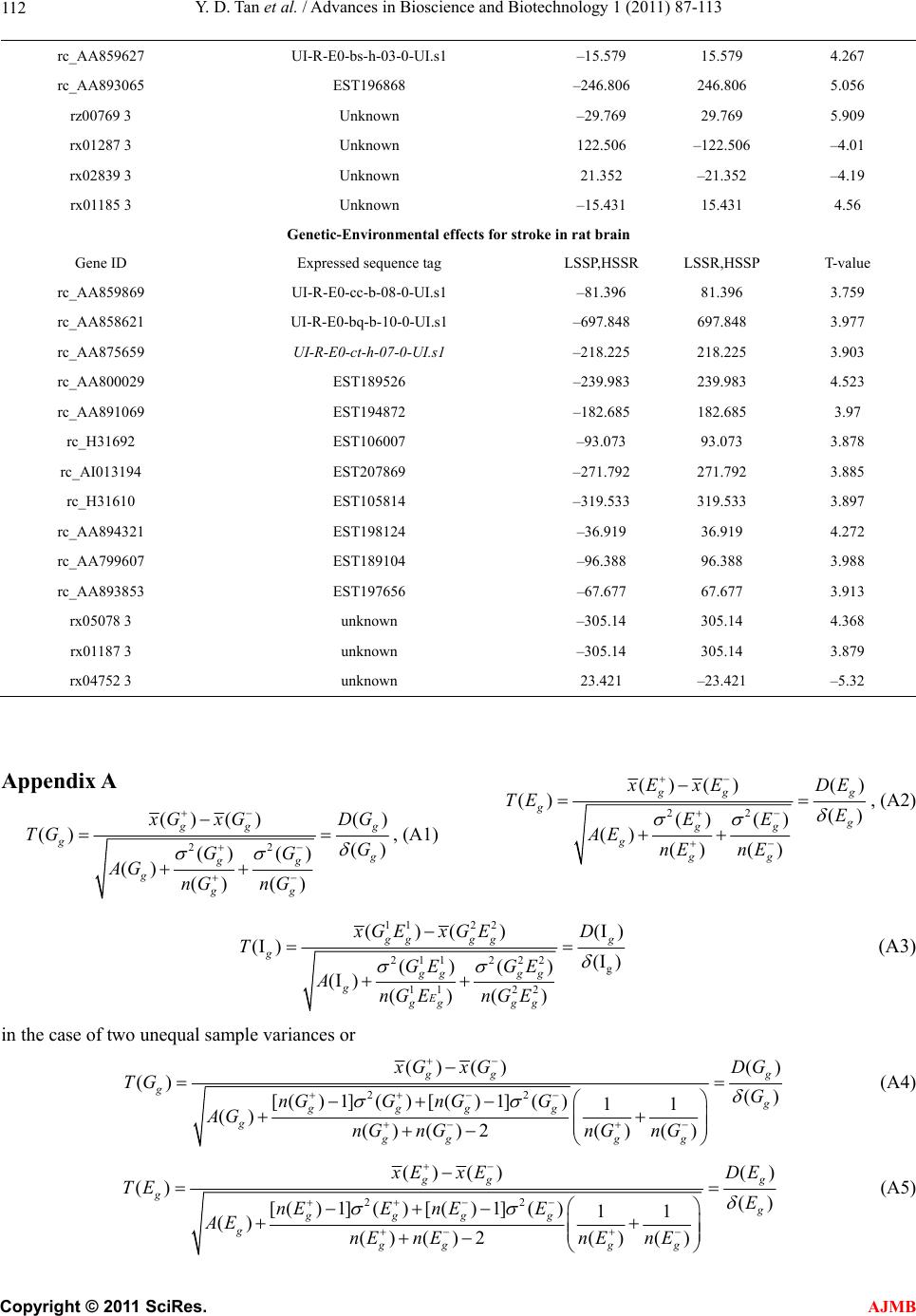 Y. D. Tan et al. / Advances in Bioscience and Biotechnology 1 (2011) 87-113 Copyright © 2011 SciRes. AJMB 112 rc_AA859627 UI-R-E0-bs-h-03-0-UI.s1 –15.579 15.579 4.267 rc_AA893065 EST196868 –246.806 246.806 5.056 rz00769 3 Unknown –29.769 29.769 5.909 rx01287 3 Unknown 122.506 –122.506 –4.01 rx02839 3 Unknown 21.352 –21.352 –4.19 rx01185 3 Unknown –15.431 15.431 4.56 Genetic-Environmental effects for stroke in rat brain Gene ID Expressed sequence tag LSSP,HSSR LSSR,HSSP T-value rc_AA859869 UI-R-E0-cc-b-08-0-UI.s1 –81.396 81.396 3.759 rc_AA858621 UI-R-E0-bq-b-10-0-UI.s1 –697.848 697.848 3.977 rc_AA875659 UI-R-E0-ct-h-07-0-UI.s1 –218.225 218.225 3.903 rc_AA800029 EST189526 –239.983 239.983 4.523 rc_AA891069 EST194872 –182.685 182.685 3.97 rc_H31692 EST106007 –93.073 93.073 3.878 rc_AI013194 EST207869 –271.792 271.792 3.885 rc_H31610 EST105814 –319.533 319.533 3.897 rc_AA894321 EST198124 –36.919 36.919 4.272 rc_AA799607 EST189104 –96.388 96.388 3.988 rc_AA893853 EST197656 –67.677 67.677 3.913 rx05078 3 unknown –305.14 305.14 4.368 rx01187 3 unknown –305.14 305.14 3.879 rx04752 3 unknown 23.421 –23.421 –5.32 Appendix A 22 () ()() () () () () () () () gg g gg g gg GxG DG TG G GG AG nG nG , (A1) 22 () ()() () () () () () () () gg g g gg g gg ExEDE TE E EE AE nE nE , (A2) 11 22 211 222g 11 22 ()() (I) (I )(I ) ()() (I )()() ggg g g gg gg gE gg gg xGE xGED T GE GE AnGEnG E (A3) in the case of two unequal sample variances or 22 () ()() () () [()1]()[()1]()11 () () ()2()() gg g g g gggg g ggg g xG xGDG TG G nGG nGG AG nG nGnGnG (A4) 22 () ()() () () [()1]()[()1]()11 () () ()2()() gg g g g gggg g ggg g xE xEDE TE E nEE nEE AE nE nEnEnE (A5)
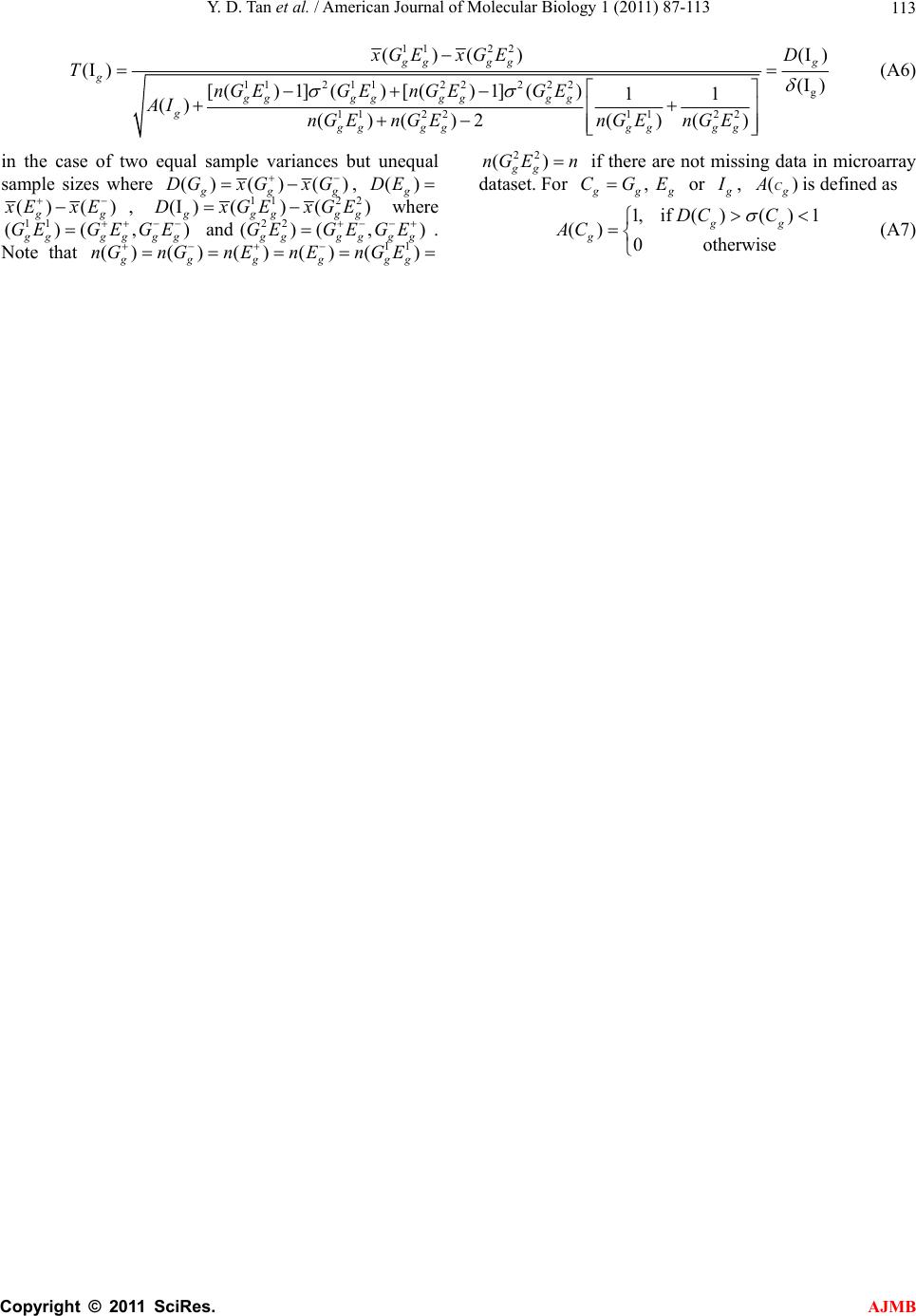 Y. D. Tan et al. / American Journal of Molecular Biology 1 (2011) 87-113 Copyright © 2011 SciRes. AJMB 11 3 11 22 112 1122222g 11 221122 ()() (I) (I ) (I ) [()1]()[()1]()11 () ()()2 ()() gg ggg g gggg gggg g gg gggggg xGE xGED T nGEGE nGEGE AI nGEnGEnGEnGE (A6) in the case of two equal sample variances but unequal sample sizes where ()() () gg DGxG xG , () g DE () () g ExE , 1122 (I )()() gg gg DxGExGE where 11 ()(,) ggggg GEGE GE and22 ()(, ) ggggg GEGE GE . Note that 11 () () () () () gggggg nG nG nEnEnGE 22 () gg nG En if there are not missing data in microarray dataset. For , gg CGE or , ()C Ais defined as 1,if ()()1 () 0otherwise gg g DC C AC (A7)
|