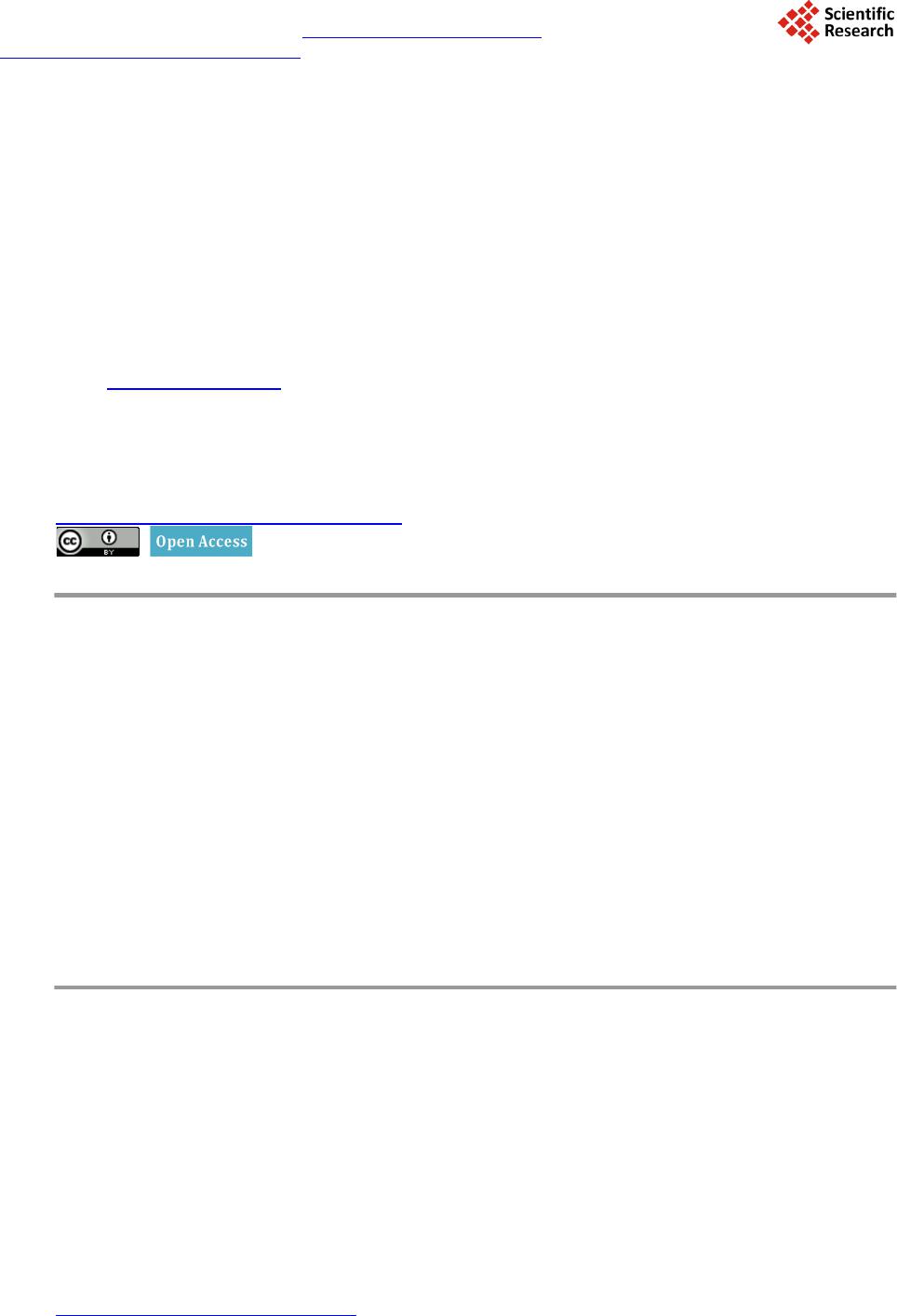 American Journal of Computational Mathematics, 2014, 4, 366-375 Published Online September 2014 in SciRes. http://www.scirp.org/journal/ajcm http://dx.doi.org/10.4236/ajcm.2014.44031 How to cite this paper: Zhang, Y., Duan, L. and Zhang, G.F. (2014) Risk Assessment of Agricultural Products Supply-Chain Finance Based on D-S Theory. American Journal of Computational Mathematics, 4, 366-375. http://dx.doi.org/10.4236/ajcm.2014.44031 Risk Assessment of Agricultural Products Supply-Chain Finance Based on D-S Theory Yuan Zhang1, Lian Duan2, Gefu Zhang1 1School of Economics and Management, University of South China, Hengyang, China 2The Agricultural Bank of China, Hengyang, China Email: melodyflly@gmail.c om Received 3 August 2014; revised 10 September 2014; accepted 18 September 2014 Copyright © 2014 by authors and Scientific Research Publishing Inc. This work is licensed under the Creative Commons Attribution International License (CC BY). http://creativecommons.org/licenses/by/4.0/ Abstract Agricultural products supply-chain finance, as one of the solutions to the issue of “capital prob- lems” of agriculture, countryside and farmers, has proposed a kind of characteristics model to as- sess the risk of agricultural production, processing and marketing, which can improve the issue of farmers and enterprises lacking of funds. This model is proposed on the basis of uncertain infor- mation processing method of D-S theory and its data combination rules, combined with the “dis- count rate” correction model, and it includes a risk assessment index system of agricultural prod- ucts supply-chai n finance, fully considering the five aspects of production, processing, marketing, cooperation of supply chain and collateral. At last, a taro supply chain is taken for example. And the risk assessment of its supply-chain finance based on this model has been discussed in detail. And the result has proved that the model and its algorithm are practical and feasible. Keywords Supply-Chain Finance, Risk, Agricultural Products, Taro, D-S Theory 1. Introduction Being in an important period of industrialization and large-scale transformation, China’s agriculture needs a lot of financial support, thus there exists a vast potential for growth in agricultural products’ financial markets. It was stated by Premier Wen Jiabao in the National Finance Working Conference in January 2007 that the estab- lishment of a rural financial system with high level, wide covering and great continuance which adapts to the characteristics of the agriculture, countryside and farmers in China should be accelerated [1]. Nowadays, how- ever, due to such characteristics of most agricultural products enterprises as small-scale, low credit rating, and strong dependence on the environment, the bank cannot give adequate financial support for these enterprises
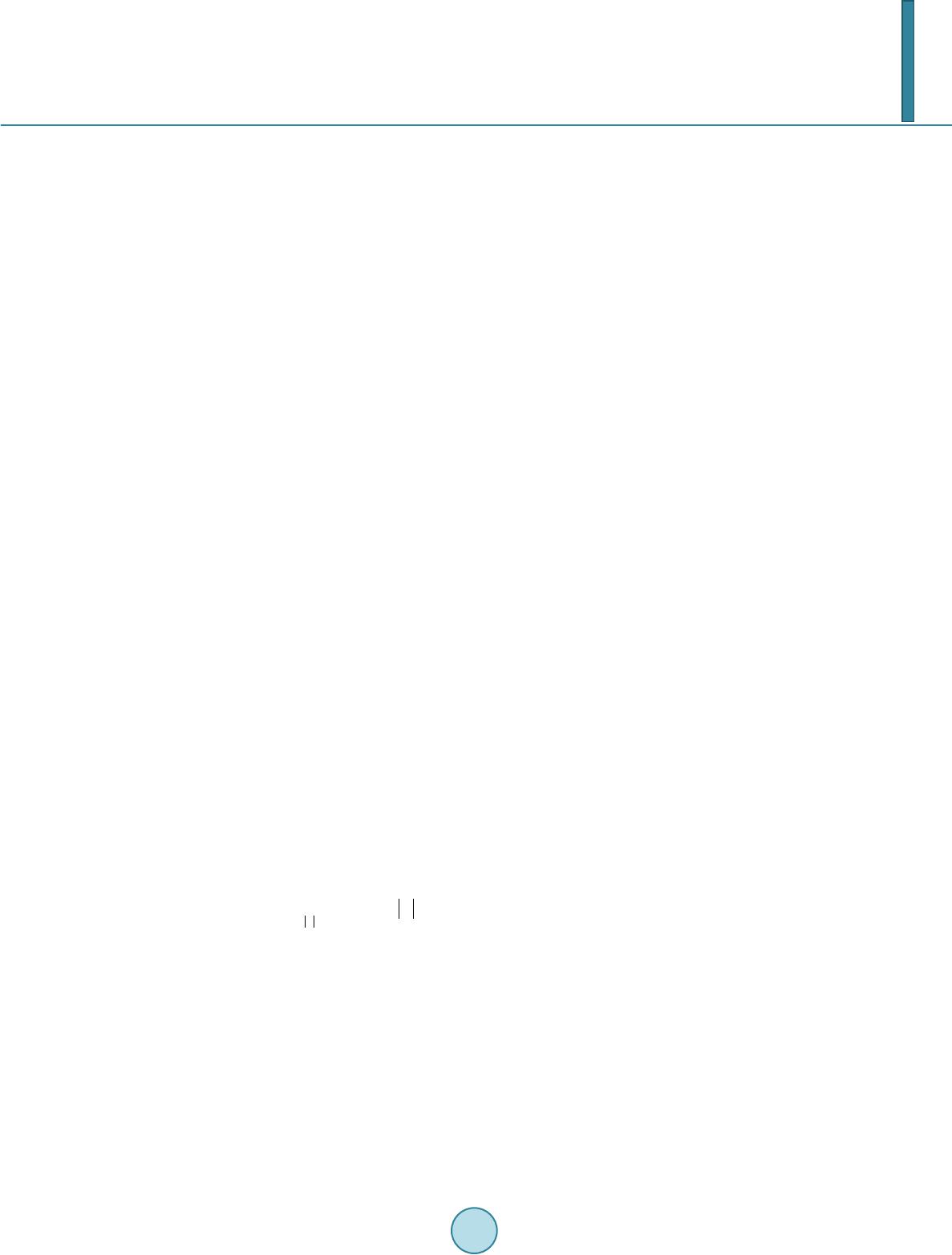 Y. Zhang et al. considering the risks of agricultural products in the supply chain, which becomes one of the main bottlenecks retarding the development of agricultural products markets. When conducting research in the trade risk issues of some small island developing states, Federica Angelucci [2] proposed that the inadequate capacity to regulate price and pr oduction was mainly caused by the lack of horizontal and vertical cooperation among the parties in the value chain and collaboration platform support, especially the credit support. Focused on the supply-chain operations under the background of real trade, supply chain finance services link the individual members of the supply chain together to promote the allocation of risks and benefits-sharing and provide comprehensive finan- cial services. This model, with low barriers to entry in corporate loans, has been applied widely in manufactur- ing, and in recent years, it has been proposed to solve the problems of inadequate financial support in the agri- cultural supply chains [3] [4]. However, because of the specificity and imperfection of the agricultural supply chains, the risk management and control problems still exist. Therefore, the study on the risk assessment of agricultural products supply-chain finance can not only offer help for the practice of agricultural supply chain finance businesses, but also be beneficial for banks to develop new businesses and explore new profit growth opportunities. However, the current research on agricultural products supply chain finance is just at the begin- ning, and the relevant practical applications are very limited, so it is of great urgency and vital importance to conduct related studies. 2. Brief Overview on the Algorithm of D-S Theory Evidence can be used to distinguish and represent the major concepts such as “uncertain”, “do not know”, etc. The most practical one is the Dempster-Shafer combinational rule, which can fuse lots of uncertain evidence from different sources so as to improve the accuracy of the reasoning for the event [5]. 2.1. Basic Thoughts about D-S Theory For a proposition, if there is a clear recognition f rame Θ containing multiple discrete elements Xi (which can contain multiple child elements Yj), the understanding and awareness of each element constitute a part of the overall comprehension of it. An element can be considered as an evidence to understand a proposition, and the sufficiency of the evidence, determined by the understanding of the subject to object x and the mastering degree Bel(x), is described with a probability value. Probability is a measure of uncertainty, a kind of risk. Therefore, as long as the uncertainty values of the individual elements in the proposition recognition frame can be identified, the overall risk characteristics of the proposition can be discerned by synthesizing the uncertainty values of var- ious elements. Definition 1: let Θ be the recognition frame, containing all possible independent and exclusive states of a sys- tem under consideration. The power set 2Θ is the set of all subsets of , including the empty set . Meanwhile, the theory of evidence assigns a belief mass to each element of the power set. Formally, a fun ction , when it adapts to the formula (1) which includes two implications: first, is the number of dimensions for the recognition frame; second, 2Θ means the collection of elements. m(x) is called a Basic Probability Assignment (BPA). Covering cr oss -understanding, vague understanding, totally ignorant understanding and complete under- standing, the Definition 1 is closed to the realistic features of the subject’s understanding to object. m() is a function to describe the belief degree, called mass function. m(X) refers to the degree of belief assigned in the focal elements, m(Θ) refers to the unc ertaint y degree of the whole proposition. Definition 2: let Θ be the recognition frame, and when any subset of X and Y adapts to the formula , (2) Bel(X) is called the belief function of focal elements X. Definition 2 clearly defined that the evidence set in a proposition, with the in-depth understanding, can be decomposed so as to adequately discern the features of the certainty of evidence from different sides. The idea is similar to the AHP -level analysis method s. Bel(X) represents the sum of the BPAs of all the subsets of the set X.
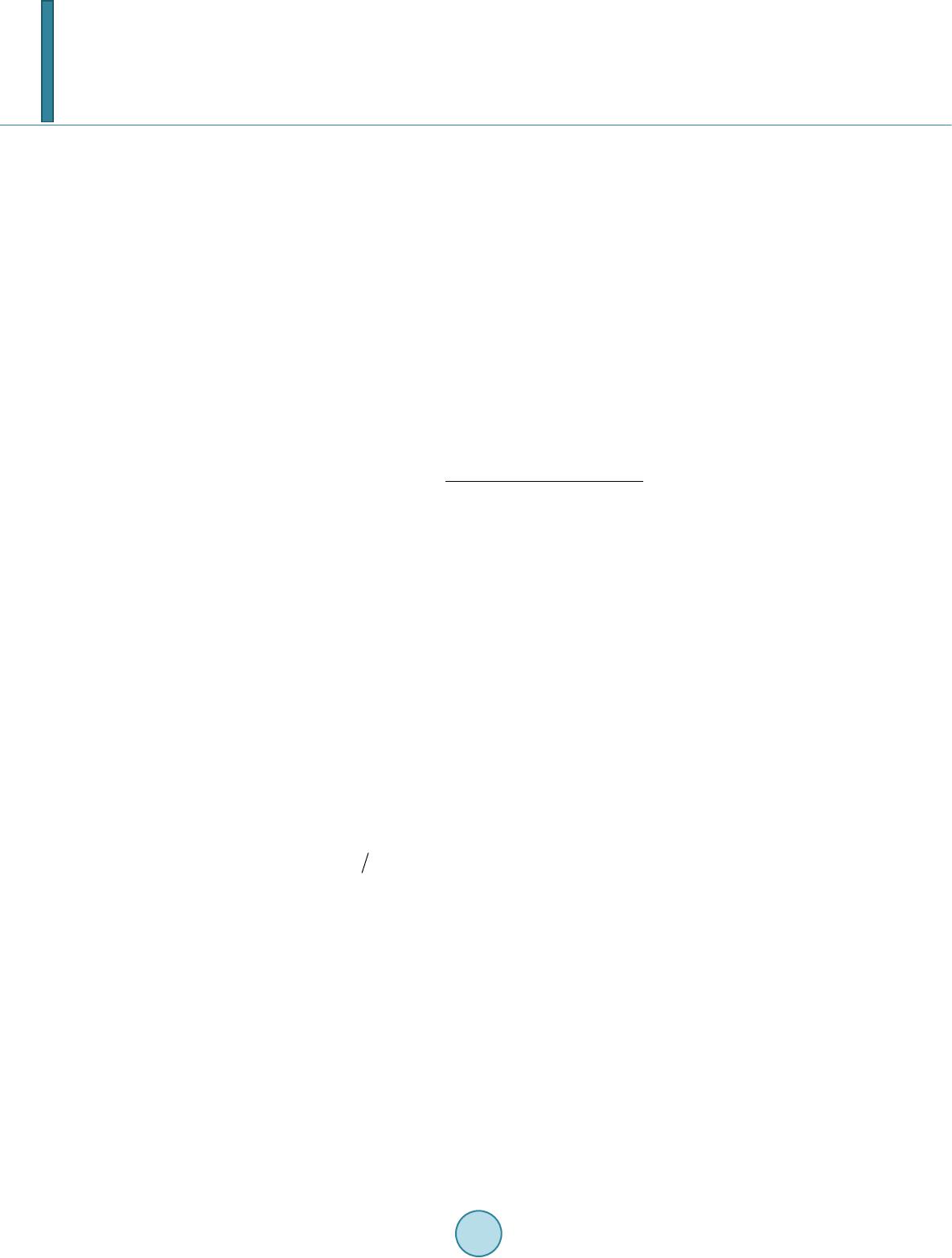 Y. Zhang et al. And Pl(X) represents the sum of the BPAs of all the focal elements that inter sect the set of X. Both of them are used to describe the uncertainty of focal elements X. Definition 3: let Θ be the recognition frame, and when any subset of X and Y adapts to the formula ()( ) ,,Y XXY PlXm Y φ ∩ ≠⊂Θ = ∑ , (3) Pl(X) is called plausibility function of focal elements X. Plausibility functio n is a method to describe the uncertainty of cross-understanding and vague understanding. When the focal elements X are not intersected with others, Pl(X) equals Bel(X). 2.2. Dempster-Shafer Combinational Rule In practical applications, the individual propositions discerned by lots of experts simultaneously can be used to constitute multiple information sources in order to reduce errors and avoid the bias of individual experts result from possible factors. Therefore, we should combine the multiple sets of BPAs in the same recognition frame Θ. The formula ( )()()()( ) ,, 0, 1 ij ij XY CXY ij XY mX mY mcm Xm YXY K φ φ ∩ =∀⊆Θ ∩= × =⊕= ∩≠ − ∑ (4) is called the Dempster-Shafer Combinational Rule, in which , called Conflict Factors. The situation where the fusion results are always contrary to the intuitive judgment is called Zadeh Pa- radox. 2.3. Dempster-Shafer Combinational Rule Based on Weighted Coefficients In the case of multiple evidence sources, all the evidences are treated equally in the Dempster -S h a fer combina- tional rule, which will be bound to damage the accuracy of estimates. As a result, some researchers [6] sug- gested that the actual risk preferences should be taken into consideration, which is called “Discount Method”. The method is as follows: At first, to discern the importance of each set o f focal elements, you can use Delphi method to constitute the weight vectors. Then, do the normalization processing, and make sure that the normalized values are corresponding to the original weight vectors, with each value called “Discount Rate”: ( )( ) ( )( ) ( ) ( ) 12 1 1 max,,,,1, 2,,,1,0 iK iiK ii n iini i i mA amA m ama a wwww inww = ′= ′Θ=Θ+ − === ≥ ∑ (5) Finally, according to the above formula, substitute the adjusted values of the basic belief degree of various evidences , into the original Dempster-Shafer combinational rule, which constitutes the Dempster-Shafer combinational rule based on the discount rates. 3. Risk Assessment Model of Agricultural Products Supply-Chain Finance In the agricultural products supp ly-chain finance model, the risk assessment of individual small businesses shifts to the operation assessment of the entire supply chain. As long as its core enterprises and the leading enterp rises runs in good condition, other upstream and downstream enterprises can have a good operating bas e, because they can support the enterprises in trouble by collaboration, such as financial guarantees, management and tech- nical services, so as to improve their oper ation situations, there by contributing to the overall development. To assess the risk of agricultural products supply-chain finance based on evidence theory, it is essential to build the evidence recognition frame. Combined with the existing studies about the risk assessment of supply- chain finance [7] [8], we will conduct the research from five aspects, the production section, processing section,
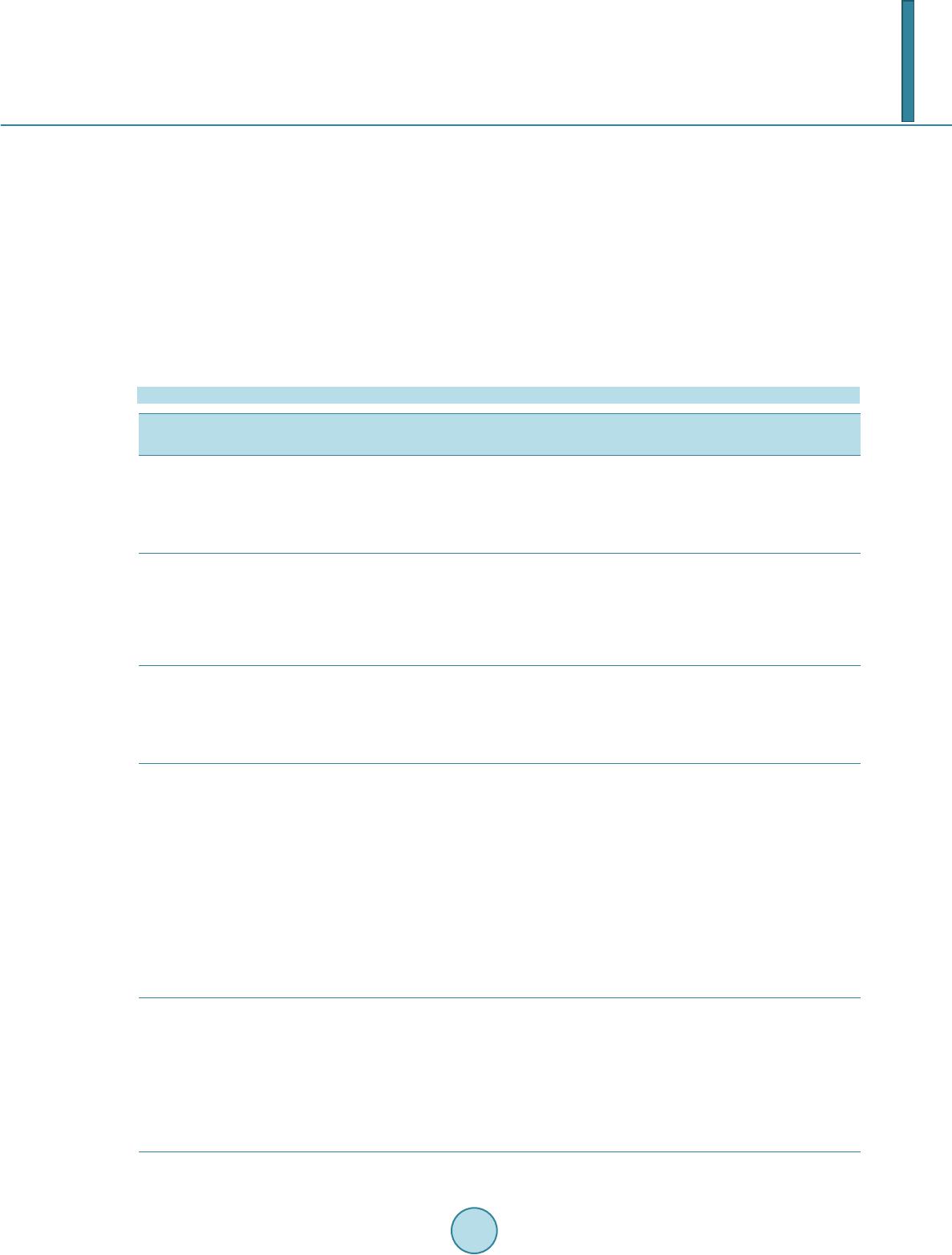 Y. Zhang et al. sales section, the whole supply-chain collaboration, the core enterprises, which constitute five basic evaluation standards, considering the operational characteristics of agricultural products supply-chain, especially the impact of the environment, logistics and technology, as well as the efficiency and cooperativity of every aspect of the agricultural products supply-chain. And then, each aspect is decomposed to conduct in-depth exploration to its uncertainty. The index syste m and its detailed explanation is shown in Figure 1 and Table 1 b elow. In the agricultural products trade, the upstream enterprises are usually the primitive production units and the shallow processing sectors, mainly dependent on the weather and logistics technology; the midstream enterpris- es are mainly the intermediate processing and deep processing enterprises in the agricultural supply-chain while the trade enterprises and vendors are in the downstream of the supply-chain. With large scale, those midstream enterprises generally have sound credit. It is the starting point of constructing this model to extend the impact of the credit and financial resources to the upstream and downstream enterprises and attach most importance to the coordination level in the supply chain and the ability to complete this trade successfully in examining SMEs. Table 1. Characteristics description of the risk assessment index system of agricultural products supply-chain. The first class indicators The secondary indicators The connotation of the indexes and evaluation basis Production section The risk of cr ops The toughne s s of the primary c rops as well as dependence on the environment. The stability of supply Stability of procurement contracts and yields. Crop quality expectations Quality passing rate of agricultural products, green ecology condition, and quality level. Processing section Technical level Including the packaging, preservation, and other pre-processing and deep- technology as well as the degree of mechanization and automation. Management level Management personnel quality of related processing enterprises; whether there is a sound management incentive system or not. Quality security Product quality and safety certification, etc. Sales section The stability of the sales channel Whether it has a stable sales network. Product competitiveness The market share and the sales rate of the products. Product market saturation The development prospects of agricultural products market as well as the supply and sales situation. The whole supply-chain conditions Informationization degree The use of EDI, bar code, radio frequency and other modern logistics technology. Standardization level Standardization degree of packaging, handling, etc. Logistics level Preservation technology, warehousing environment, operational efficiency, the usage of the cold chain technology and equipments. Enterprise comprehensive strength Enterprise scale, development prospects of the various areas of the supply chain, including the asset size and industry position of the core enterprises, etc. Constraining f orce among the members Whether a stable strategic partnership can be established among each participants; cooperation with the core enterprises and the security situation. The industry’s macro environment Whether the Government is supporting the development of agricultural products enterprises. Security situation The pledge If it is FTW or confirming warehouse financing, the stability of the collateral prices, liquidity and vulnerability degree should be fully taken into account; if it is accounts receivable financing, it is of vital importance to consider the aging and the account period of accounts receivable and other factors. The financial situation of the core enterprises Asset-liability ratio, cash ratio and other financial circumstances; whether there are sufficient funds to guarantee the repayment section. The credit standing of the core enterprises Credit ratings of core enterprises in the bank or rating agencies.
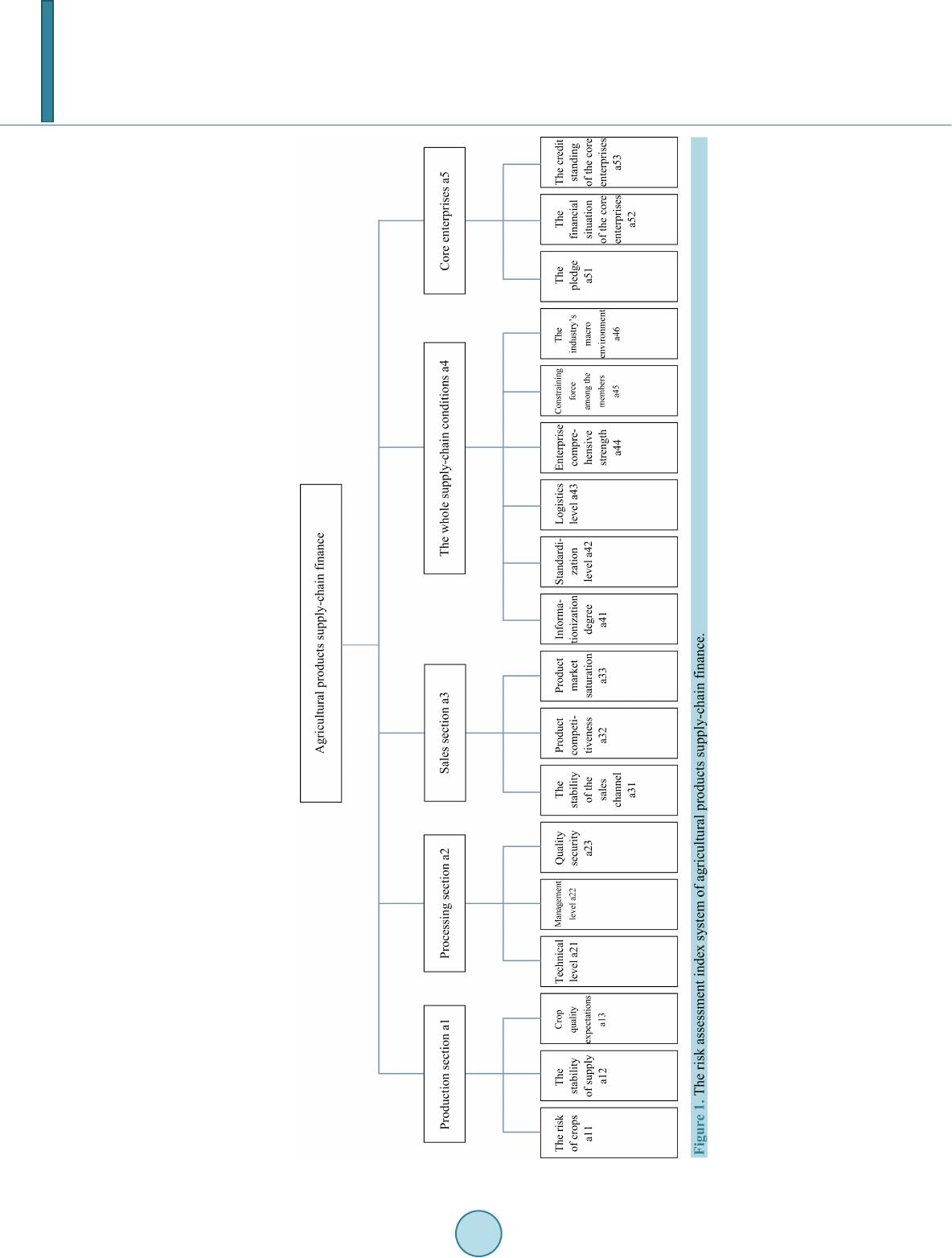 Y. Zhang et al.
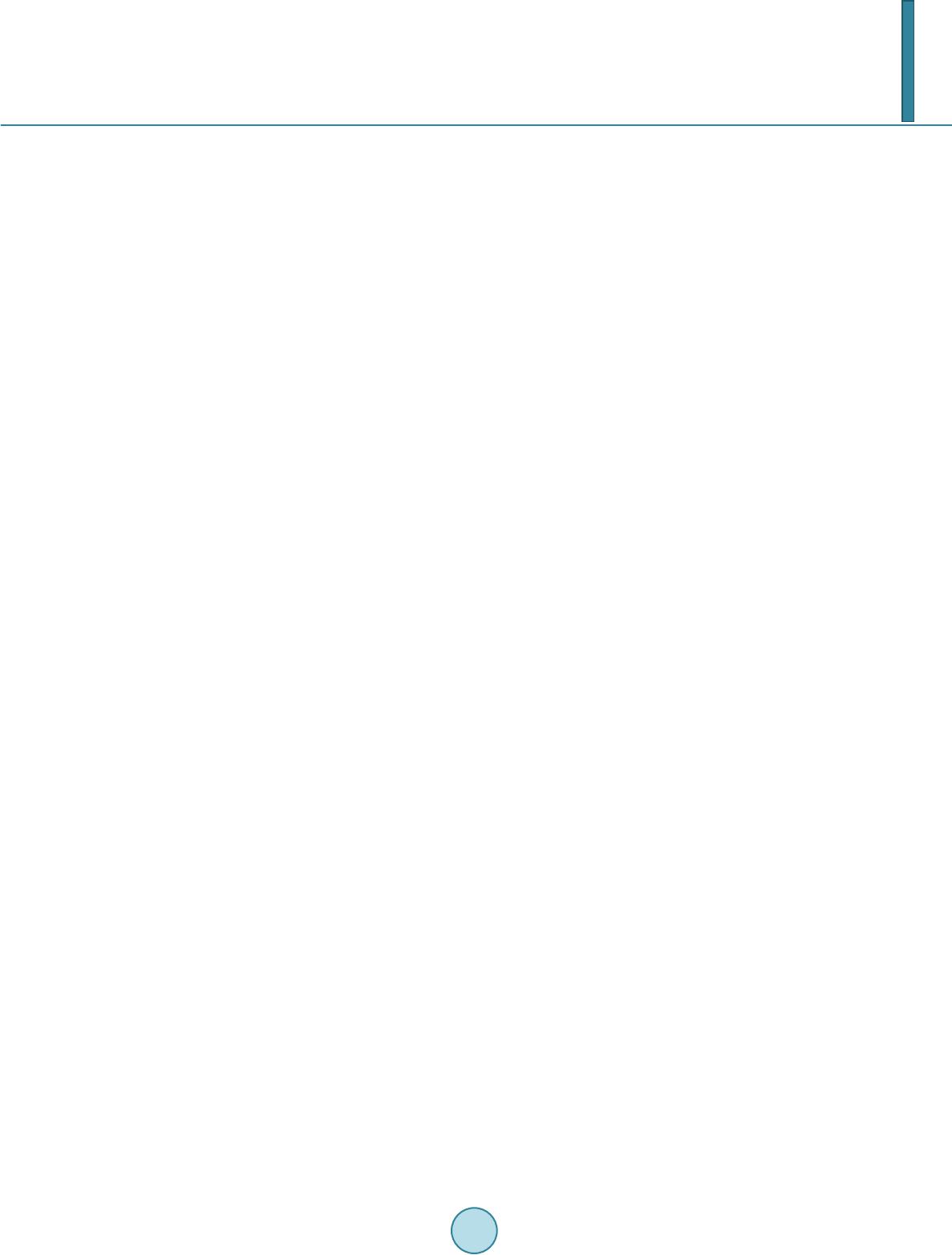 Y. Zhang et al. Differences in the characteristics of agricu ltural products can lead to those of the risks weighting on the oper- ation of the supply-chain. For instance, the supply-chain risk of fruits and vegetables, compared with wood and wool fabric, is less affected in the processing stage but is more likely to be affected in the production stage; while the supply-chain risk of aquatic pro ducts will be affected by the impact of logistics with a relatively high proportion. To reduce the complexity, the agricultural products supply-chain in this study is mainly compo sed of the trade activities of 11 categories of products, including fruits and vegetables, aquatic products, meat products, tea cakes and sweetmeats, tobacco, trees and flowers, medicines, grain and oil crops, livestock, mushrooms, forest products, e tc. I t co ver s the providers of original mea n s of pr oduction, grow ers, logistics enterprises, pa ck- aging and processing enterprises as well as trade and marketing enterprises. Obviously, with many sub-catego- ries of each type, the supply-chain differs greatly in its oper ational risk . Therefore, it is necessary to ask experts to do ass ignment on the vario us fa c t ors of each type. The Delphi method is used to determine the weights in this assessment index system model. The e xp er t s, in- volving operating personnel of agriculture bank credit departments, experts of agricultural technology sectors, marketing experts of agricultural products trade enterprises, use their experience in dealing with financial ser- vices and related businesses of agricultural products enterprises to assign the weight of each index in the agri- cultural products supply-chain index system, and reduce the possibility of evidence conflicts in the early stage through multiple consistent processing, such as judgment matrix construction, normalization, consistency check table, so as to increase the authority and accuracy of the weight item. 4. Case Study With a tradition of planting taro, many areas in China have formed the industry, such as Lechang of Guangdong, Qidong County of Hunan, but there exist different risks in the taro cultivation, preservation and storage, processing and marketing and other sectors. The farmers adopt the way of marketing their own products with baskets; though the taro has high yields yet no harvest. For example, according to 2013’s “Southern reports”, the taro farmers of Lechang, Guangdong looked disappointed with the unmarketable taros due to the stagnant mar- ket. While the situation in Qidong is nothing like that of Lechang. The taro farmers there have nearly one thou- sand dollars more income to plant an acre of taro than to plant an acre of rice with a coalition of growers through the cooperatives and joint ventures, the financial support to the fresh storage and processing enterprises, the rapid development of deep processing and the further deepened market, which stimulated the enthusiasm of farmers to expand growing taro and made financial issues highlighted. A typical taro supply-chain includes the following sectors (Figure 2): taro seeds and fertilizer providers, taro growers, storage and shallow processing enterprises, deep-processing enterprises, wholesalers and traders and retailers. To give financial support to any enterprises, the banks not only need to examine the situation of individual enterprises, but also need to investi- gate the ecology of the whole chain and the management status of core enterprises. With the changes in consumer’s tastes, personality characteristics become more evident and the product di- versification is in great demand. Hence, the businesses of deep-processing enterprises develop rapidly, driving the production of taro and giving strong support to the domestic and foreign trade of taro products. In this case, farmers loan from banks with the future receivables as collateral, and then the banks examine the situation of the entire chain and ultimately determine whether loan to taro farmers or not, which can also be extended to the seeds and fertilizer providers. However, as for the loan operations, how much risk do the banks face? Based on the above mentioned risk assessment model and the application of evidence theory, the risk status of the taro supply-chain can be calculated to lay a basis for the banks’ decision-making. The methods of calculating are as follows: 1) Use the Delphi method, in which several experts or credit business staffs assign the basic belief value with the related scoring criteria of the fruits and vegetables supply-chain and preset reference examples as a bench- mark. Table 2 shows the assessment results from an expert. 2) Use the discount rate model, namely the Formula (5), to adjust the correspo nding initial basic belief valu es of two indexes in Table 2 and form the Table 3. m(Θ) indicates the degree of uncertainty in the whole proposi- tion. Actually, the basic belief value of an indicator with the most weighted index value remains unchanged, while others are assigned to Θ in proportion. 3) Synthesize the basic belief values of secondary indexes to obtain the basic belief assignment values of in- dicators. The results are shown in Table 4.
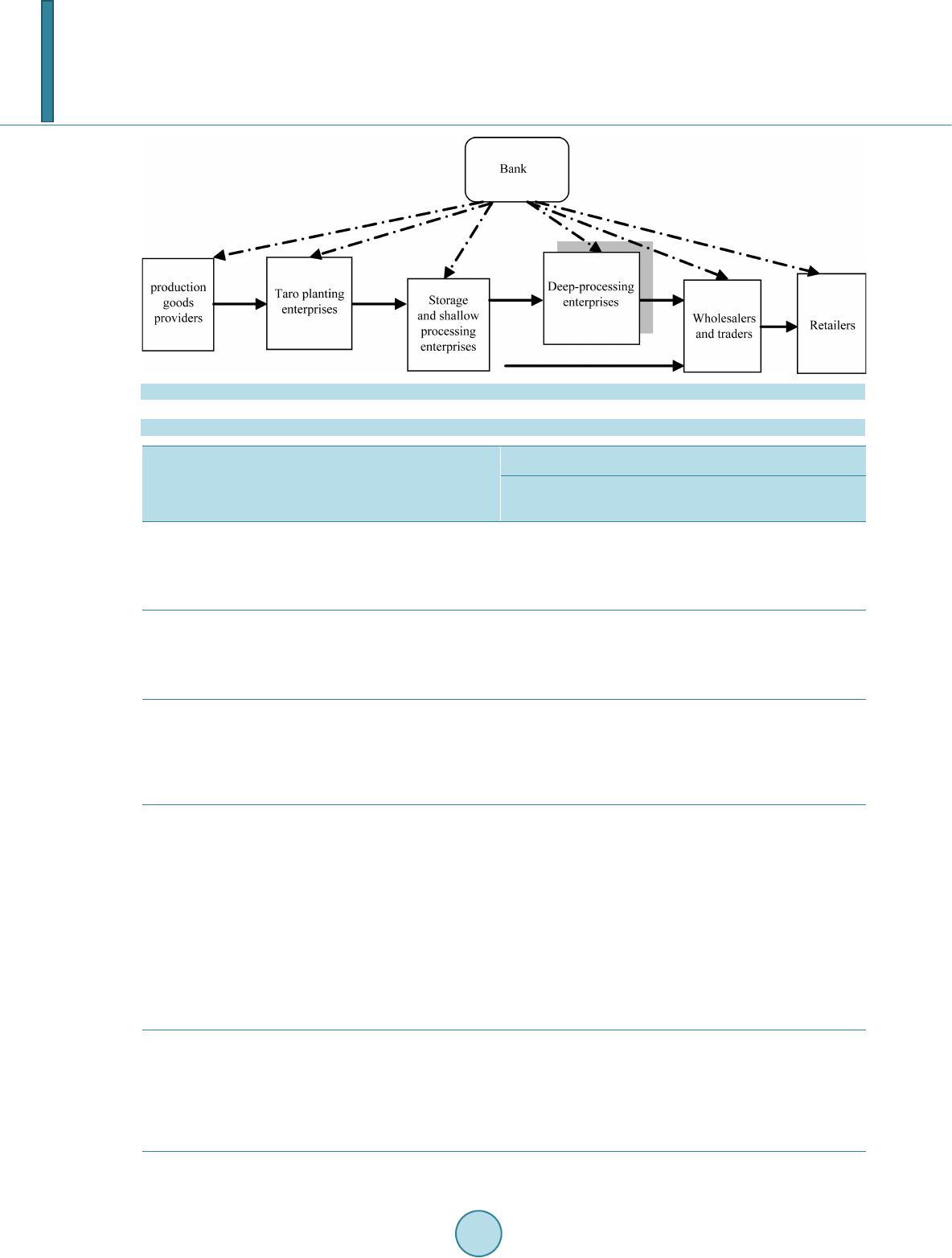 Y. Zhang et al. Figure 2. Taro supply-chain structures. Table 2. Risk weights and basic belief assignment value instance of agricultural products supply-chain finance. indicators Index weights Wi The secondary indicators Index weights Wij The basic belief βij Very high risk X1 High risk X2 Medium risk X3 Low risk X4 Very low risk X5 Θ Production section 0.1 The risk of cr ops 0.15 0 0.1 0.1 0.6 0.2 0 The stability of supply 0.35 0 0 0.15 0.75 0.1 0 Crop quality expectations 0.5 0 0.1 0.25 0.4 0.15 0.1 Processing section 0.1 Technical level 0.6 0 0 0.5 0.3 0.1 0.1 Management level 0.25 0.05 0.1 0.5 0.1 0.05 0.2 Quality security 0.15 0 0 0.1 0.8 0.1 0 Sales section 0.3 The stability of the sales channel 0.65 0 0 0.35 0.45 0 0.2 Product competitiveness 0.15 0 0 0.1 0.5 0.2 0 .2 Product market saturation 0.2 0 0.1 0.1 0.4 0.2 0.2 The whole supply-chain conditions 0.2 Informationization degree 0.1 0.1 0.1 0 .3 0 0 0.5 Standardization level 0.1 0.05 0.15 0.2 0.5 0.1 0 Logistics level 0.45 0 0.1 0.4 0.3 0.1 0 .1 Enterprise comprehensive strength 0.2 0.1 0.2 0.4 0.1 0 0.2 Constraining f orce among the members 0.1 0 0.2 0.3 0.1 0.1 0.3 The industry’s macro environment 0.05 0.2 0.2 0 .4 0.2 0 0 Security situation 0.3 The pledge 0.6 0 0 0.1 0.2 0.7 0 The financial situation of the core enterprises 0.2 0 0.2 0.3 0.2 0 0.3 The credit standing of the core enterprises 0.2 0 0 0.2 0.4 0.1 0.3
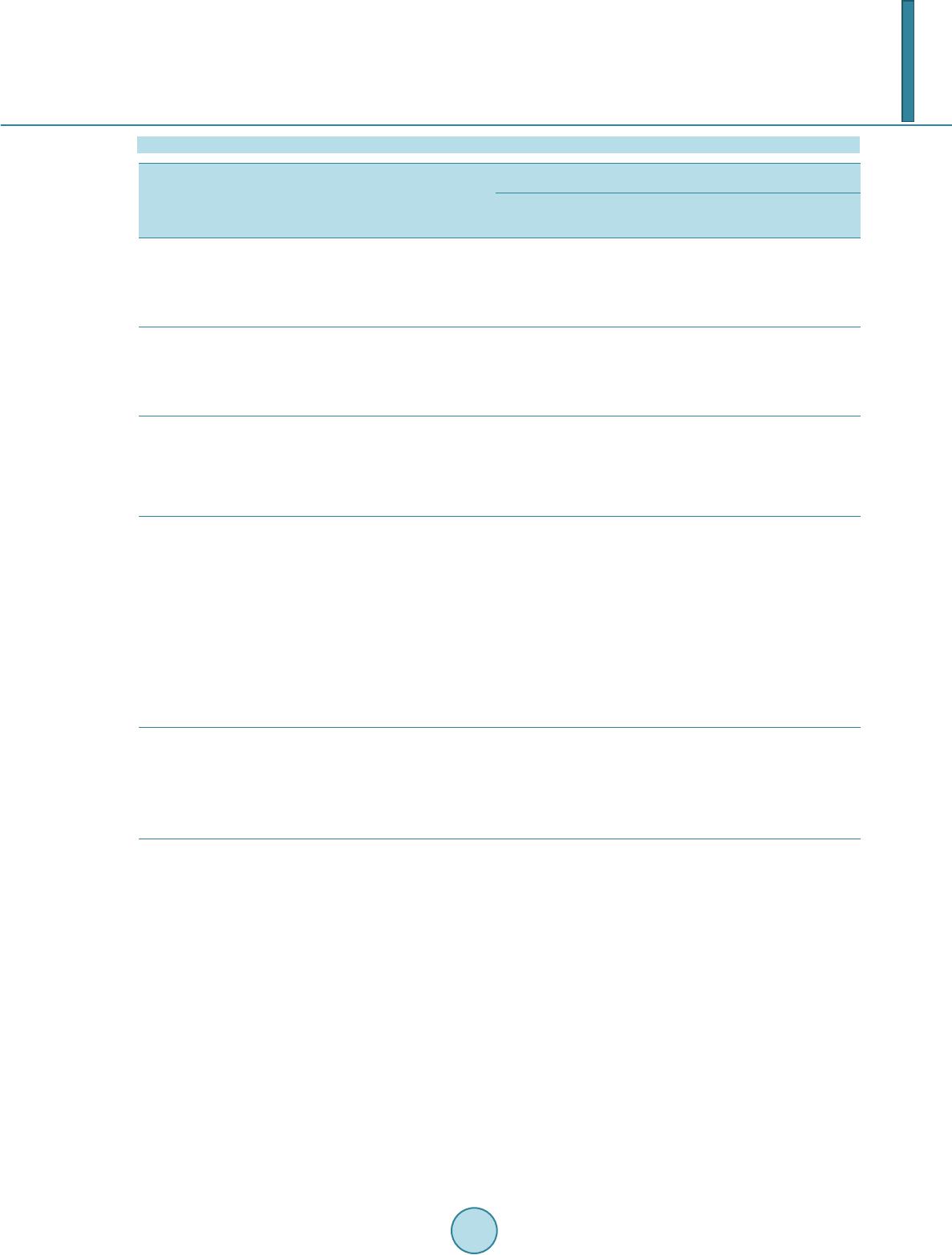 Y. Zhang et al. Table 3. The adjusted basic belief assignment of agricultural products supply-chain finance risks. indicators Index weights Wi The secondar y indicators Index weights Wij The basic belief βij Very high risk X1 High risk X2 Medium risk X3 Low risk X4 Very low risk X5 Θ Production section 0.1 The risk of cr ops 0.15 0 0.03 0.03 0.18 0.06 0.7 The stability of supply 0.35 0 0 0.105 0.525 0.07 0.3 Crop quality expectations 0.5 0 0.1 0.25 0.4 0.15 0.1 Processing section 0.1 Technical level 0.6 0 0 0.5 0.3 0.1 0.1 Management level 0.25 0.0208 0.042 0.208 0.042 0.021 0.667 Quality security 0.15 0 0 0.025 0.2 0.025 0.75 Sales section 0.3 The stability of the sales channel 0.65 0 0 0.35 0.45 0 0.2 Product competitiveness 0.15 0 0 0.023 0.115 0.046 0.8615 Product market saturation 0.2 0 0.0307 0.0307 0.123 0.0615 0.7538 The whole supply-chain conditions 0.2 Informationization degree 0.1 0.0222 0.0222 0.0667 0 0 0.8889 Standardization level 0.1 0.011 0.0333 0.0444 0.111 0.0222 0.7778 Logistics level 0.45 0 0.1 0.4 0.3 0.1 0.1 Enterprise comprehensive strength 0.2 0.0444 0.0889 0.1778 0.0444 0 0.6444 Constraining f orce among the members 0.1 0 0.0444 0.0667 0.0222 0.0222 0.8444 The industry’s macro environment 0.05 0.0222 0.0222 0.0444 0.0222 0 0.8889 Security situation 0.3 The pledge 0.6 0 0 0.1 0.2 0.7 0 The financial situation of the core enterprises 0.2 0 0.0667 0.1 0.0667 0 0.7667 The credit standing of the core enterprises 0.2 0 0 0.0667 0.1333 0.0333 0.7667 4) Use the discount rate model again, added to the index weights of the first class indicators. The results ad- justed are as follows in Table 5. 5) Synthesize the basic belief assignment of the first class indicators. The results are as shown in Table 6. 6) Finally, use the calculation formula of the agricultural products supply-chain risk to get the risk assessment value of this agricultural product supply-chain finance. Suppose P(x1) = 5, P(x2) = 4, P(x3) = 3, P(x4) = 2, P(x5) = 1, P(Θ) = 3.5, and then the supply-chain finance risk value R is 1.63308. (0 ≤ R ≤ 5; the b igger R is, the higher the risk is) . 5. Conclusions and Outlook According to the high, medium and low risk levels, obviously, the foregoing calculation result of risk belongs to a low risk level. Therefore, banks can loan to taro farmers, which is in line with the interests of the relevant par- ties. And it shows , in practice, that banks have called in loans, without bad debts. Then, the model itself and the
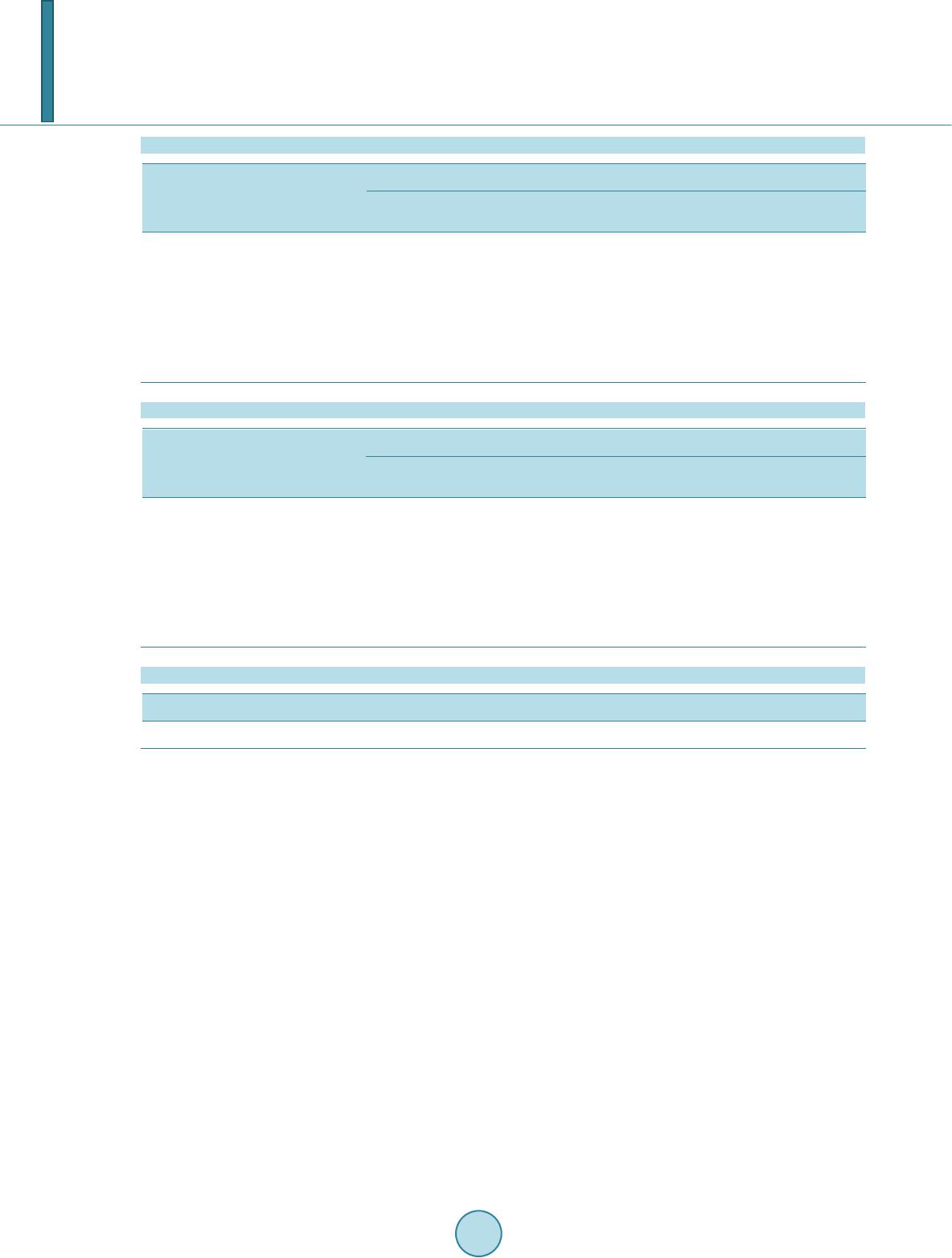 Y. Zhang et al. Table 4. The synthesized basic belief assignment of agricultural products supply-chain finance risks. The first class indicators Index weights The basic belief assignment Very high risk X1 High risk X2 Medium risk X3 Low risk X4 Very low risk X5 Θ Production section 0.1 0 0.04405 0.15936 0.66077 0.09525 0.04057 Processing section 0.1 0.00227 0.00455 0.51921 0.31889 0.08228 0.07274 Sales section 0.3 0 0.00634 0.30163 0.51464 0.02218 0.15522 The whole supply-chain conditions 0.2 0.00913 0.10669 0.47366 0.27190 0.07296 0.06567 Security situation 0.3 0 0 0.11085 0.23022 0.65892 0.00000 Table 5. The readjusted basic belief assignment of agricultural products supply-chai n finance risks. The first class indicators Index weights The basic belief assignment Very high risk X1 High risk X2 Medium risk X3 Low risk X4 Very low risk X5 Θ Production section 0.1 0 0.01468 0.05312 0.22026 0.03175 0.68019 Processing section 0.1 0.00076 0.00152 0.17307 0.10630 0.02743 0.69091 Sales section 0.3 0 0.00634 0.30163 0.51464 0.02218 0.15522 The whole supply-chain conditions 0.2 0.00609 0.07113 0.31577 0.18127 0.04864 0.37711 Security situation 0.3 0 0 0.11085 0.23022 0.65892 0 Table 6. The synthesized basic belief assignment of the first class indicators. Very high risk X1 High risk X2 Medium risk X3 Low risk X4 Very low risk X5 Θ 0 0 0.15836 0.54640 0.29506 0 application of evidence theory will be analyzed from the following three aspects. 1) Us e D-S combinational rule to fuse the belief function assignment of the financial risks of various agricul- tural products supply-chains, which can draw on collective w isdom and avoid large deviations. Hence, it is more reasonable compared with the simple weighted average data processing method. As for the method, when the views are consistent, the support degree after fusion will be higher, and vice versa reduced. 2) Use D-S combinational rule based on discount rates to fuse the corresponding risk degrees of different in- dex attribute, which has improved th e limitation of equal treatment for all indexes in the D-S combinational rule and reduced the impact of highly conflicting evidence. Taking the combination of the sales sectors for example, compared with the original belief value of the secondary indicators, the adjusted result is obviously closer to the item with the largest weight ratio, and thus the belief a s s ignment is more reasonable. 3) The risk assessment of agricultural products supp ly-chain finance covers many factors in the supply-chain and problems of various sectors such as the complex risk assessment indicators and the uncertainty of informa- tion sources, which are also difficult to quantify. Besid es, what it involves mostly are the s mall and medium en- terprises, even individual farmers, which lead to the lack of financial data and credit records, and as a result, it is difficult to obtain complete and accurate data in the data acquisition and processing. However, when initially used, the evidence theory does not require very precise quantitative information, and the fuzzification method can be applied to deal with the uncertainty of various scenarios, such as constructing the membership model. Therefore, this model has strong adaptability. However, the above model and the calculation method also have some flaws. On one hand, the calculation of risks doesn’t fully consider the characteristics of prospective borrowers. Taking taro farmers for example, their
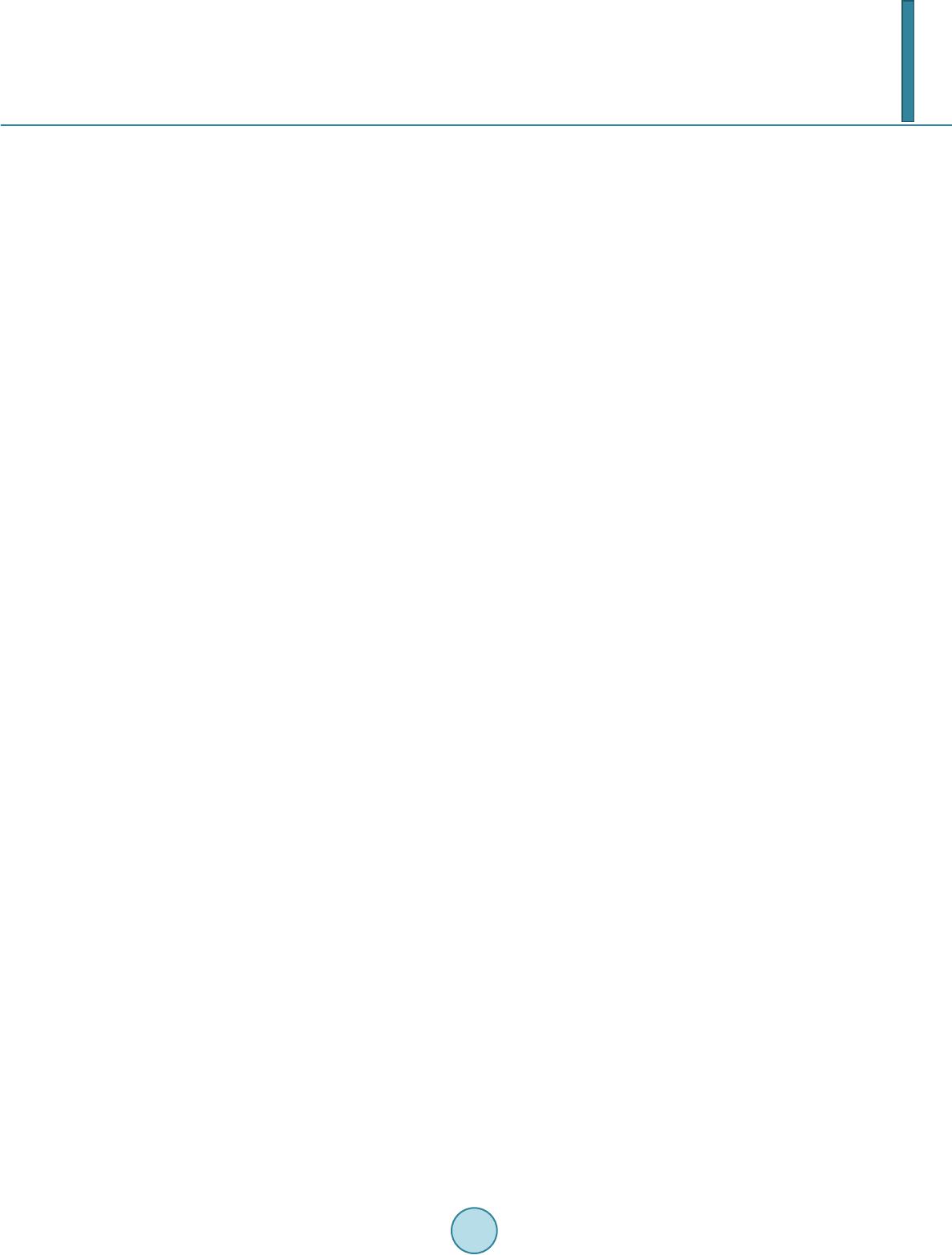 Y. Zhang et al. characteristics cannot be ignored because processors can reselect suppliers to reconstruct the taro supply-chain. On the other hand, for banks, because of the multiple types and various features of agricultural products, it is difficult for those loan officers who are unfamiliar with the product characteristics to carry out the work in an artificial way. So it is of vital importance to develop effective tools to support classification, calculation and analysis. This is our future research work. References [1] Zhou, C. (2012) Build a Policy Support System and Fill the Blank of the Financial Services. Financial Times, 28 Au- gust 2012. [2] Angelucci, F. and Conforti, P. (2010) Risk Management and Finance along Value Cha ins of Small Island Developing States. Evidence from the Caribbean and the Pacific. Food Policy, 12. [3] Yang, J.R. (2013) The Strategic Choice of Rural Financial Product Innovation Based on the Financial Perspective of Supply Chain. Finance and Economics, 3. [4] Y. Wang, (2012) Do a Good Job in Financial Support of Agricultural Products Supply Chai n. Securities Times, 27 De- cember 2012. [5] Li, B. and Pang, F.-W. (2013) An Approach of Vessel Collision Risk Assessment Based on the D-S Evidence The ory . Ocean Engineering, 12. [6] Gao, H.S. and Zhu, J. (2008) A Kind of Network Security Risk Assessment Model Based on D-S Evidence Theory. Computer Engineering and Applications, 6. [7] Li, Y.X. (2011) Supply Chain Finance Risk Assessment. Journal of Cent r al University of Finance and Economics, 10. [8] Tian, J.H. (2013) Supply Chain Finance and Its Credit Risk Control. Zhejiang Industry and Commerce University, Hangzhou.
|