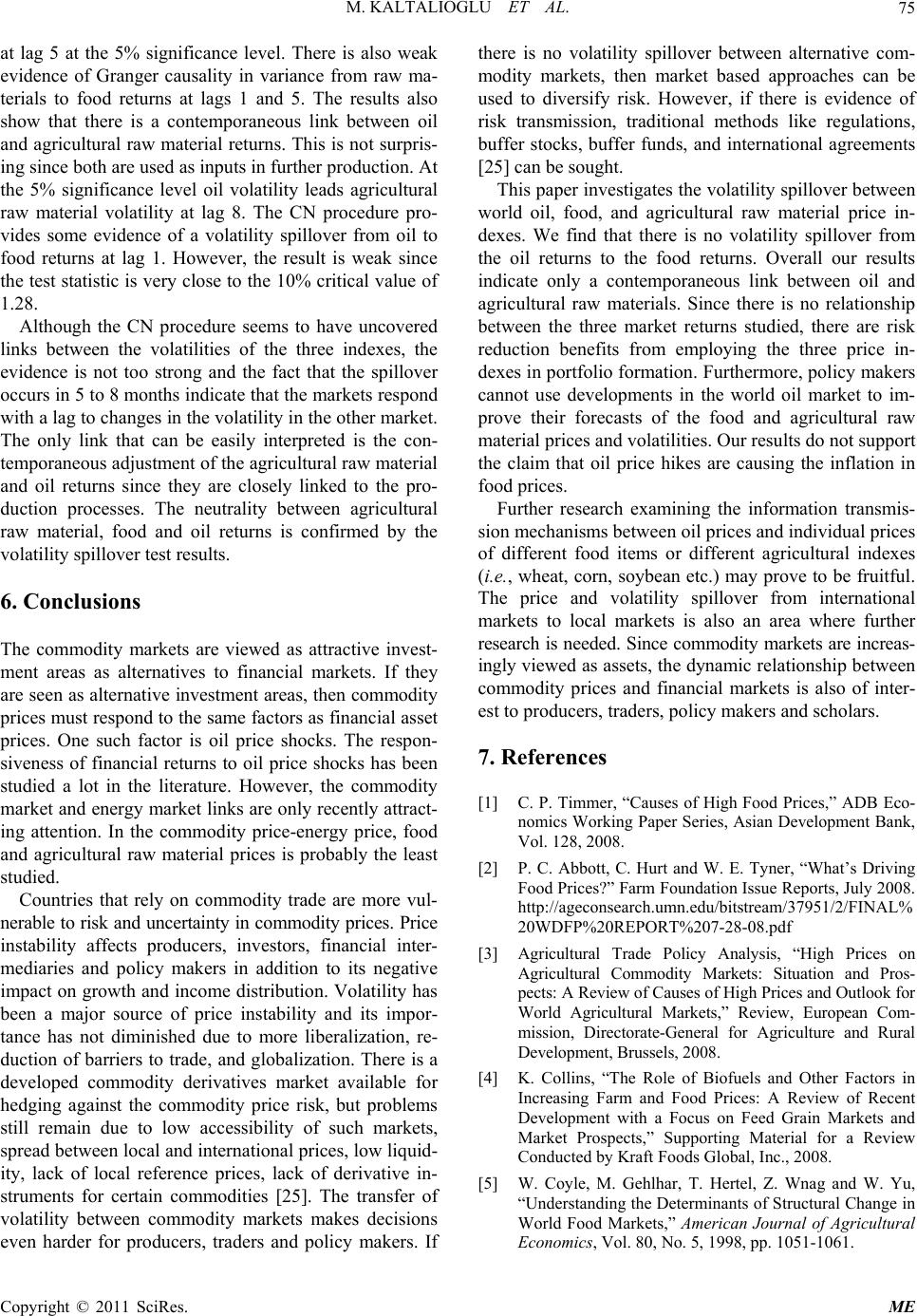
M. KALTALIOGLU ET AL.
Copyright © 2011 SciRes. ME
75
at lag 5 at the 5% significance level. There is also weak
evidence of Granger causality in variance from raw ma-
terials to food returns at lags 1 and 5. The results also
show that there is a contemporaneous link between oil
and agricultural raw material returns. This is not surpris-
ing since both are used as inputs in further production. At
the 5% significance level oil volatility leads agricultural
raw material volatility at lag 8. The CN procedure pro-
vides some evidence of a volatility spillover from oil to
food returns at lag 1. However, the result is weak since
the test statistic is very close to the 10% critical value of
1.28.
Although the CN procedure seems to have uncovered
links between the volatilities of the three indexes, the
evidence is not too strong and the fact that the spillover
occurs in 5 to 8 months indicate that the markets respond
with a lag to changes in the volatility in the other market.
The only link that can be easily interpreted is the con-
temporaneous adjustment of the agricultural raw material
and oil returns since they are closely linked to the pro-
duction processes. The neutrality between agricultural
raw material, food and oil returns is confirmed by the
volatility spillover test results.
6. Conclusions
The commodity markets are viewed as attractive invest-
ment areas as alternatives to financial markets. If they
are seen as alternative investment areas, then commodity
prices must respond to the same factors as financial asset
prices. One such factor is oil price shocks. The respon-
siveness of financial returns to oil price shocks has been
studied a lot in the literature. However, the commodity
market and energy market links are only recently attract-
ing attention. In the commodity price-energy price, food
and agricultural raw material prices is probably the least
studied.
Countries that rely on commodity trade are more vul-
nerable to risk and uncertainty in commodity prices. Price
instability affects producers, investors, financial inter-
mediaries and policy makers in addition to its negative
impact on growth and income distribution. Volatility has
been a major source of price instability and its impor-
tance has not diminished due to more liberalization, re-
duction of barriers to trade, and globalization. There is a
developed commodity derivatives market available for
hedging against the commodity price risk, but problems
still remain due to low accessibility of such markets,
spread between local and international prices, low liquid-
ity, lack of local reference prices, lack of derivative in-
struments for certain commodities [25]. The transfer of
volatility between commodity markets makes decisions
even harder for producers, traders and policy makers. If
there is no volatility spillover between alternative com-
modity markets, then market based approaches can be
used to diversify risk. However, if there is evidence of
risk transmission, traditional methods like regulations,
buffer stocks, buffer funds, and international agreements
[25] can be sought.
This paper investigates the volatility spillover between
world oil, food, and agricultural raw material price in-
dexes. We find that there is no volatility spillover from
the oil returns to the food returns. Overall our results
indicate only a contemporaneous link between oil and
agricultural raw materials. Since there is no relationship
between the three market returns studied, there are risk
reduction benefits from employing the three price in-
dexes in portfolio formation. Furthermore, policy makers
cannot use developments in the world oil market to im-
prove their forecasts of the food and agricultural raw
material prices and volatilities. Our results do not support
the claim that oil price hikes are causing the inflation in
food prices.
Further research examining the information transmis-
sion mechanisms between oil prices and individual prices
of different food items or different agricultural indexes
(i.e., wheat, corn, soybean etc.) may prove to be fruitful.
The price and volatility spillover from international
markets to local markets is also an area where further
research is needed. Since commodity markets are increas-
ingly viewed as assets, the dynamic relationship between
commodity prices and financial markets is also of inter-
est to producers, traders, policy makers and scholars.
7. References
[1] C. P. Timmer, “Causes of High Food Prices,” ADB Eco-
nomics Working Paper Series, Asian Development Bank,
Vol. 128, 2008.
[2] P. C. Abbott, C. Hurt and W. E. Tyner, “What’s Driving
Food Prices?” Farm Foundation Issue Reports, July 2008.
http://ageconsearch.umn.edu/bitstream/37951/2/FINAL%
20WDFP%20REPORT%207-28-08.pdf
[3] Agricultural Trade Policy Analysis, “High Prices on
Agricultural Commodity Markets: Situation and Pros-
pects: A Review of Causes of High Prices and Outlook for
World Agricultural Markets,” Review, European Com-
mission, Directorate-General for Agriculture and Rural
Development, Brussels, 2008.
[4] K. Collins, “The Role of Biofuels and Other Factors in
Increasing Farm and Food Prices: A Review of Recent
Development with a Focus on Feed Grain Markets and
Market Prospects,” Supporting Material for a Review
Conducted by Kraft Foods Global, Inc., 2008.
[5] W. Coyle, M. Gehlhar, T. Hertel, Z. Wnag and W. Yu,
“Understanding the Determinants of Structural Change in
World Food Markets,” American Journal of Agricultural
Economics, Vol. 80, No. 5, 1998, pp. 1051-1061.