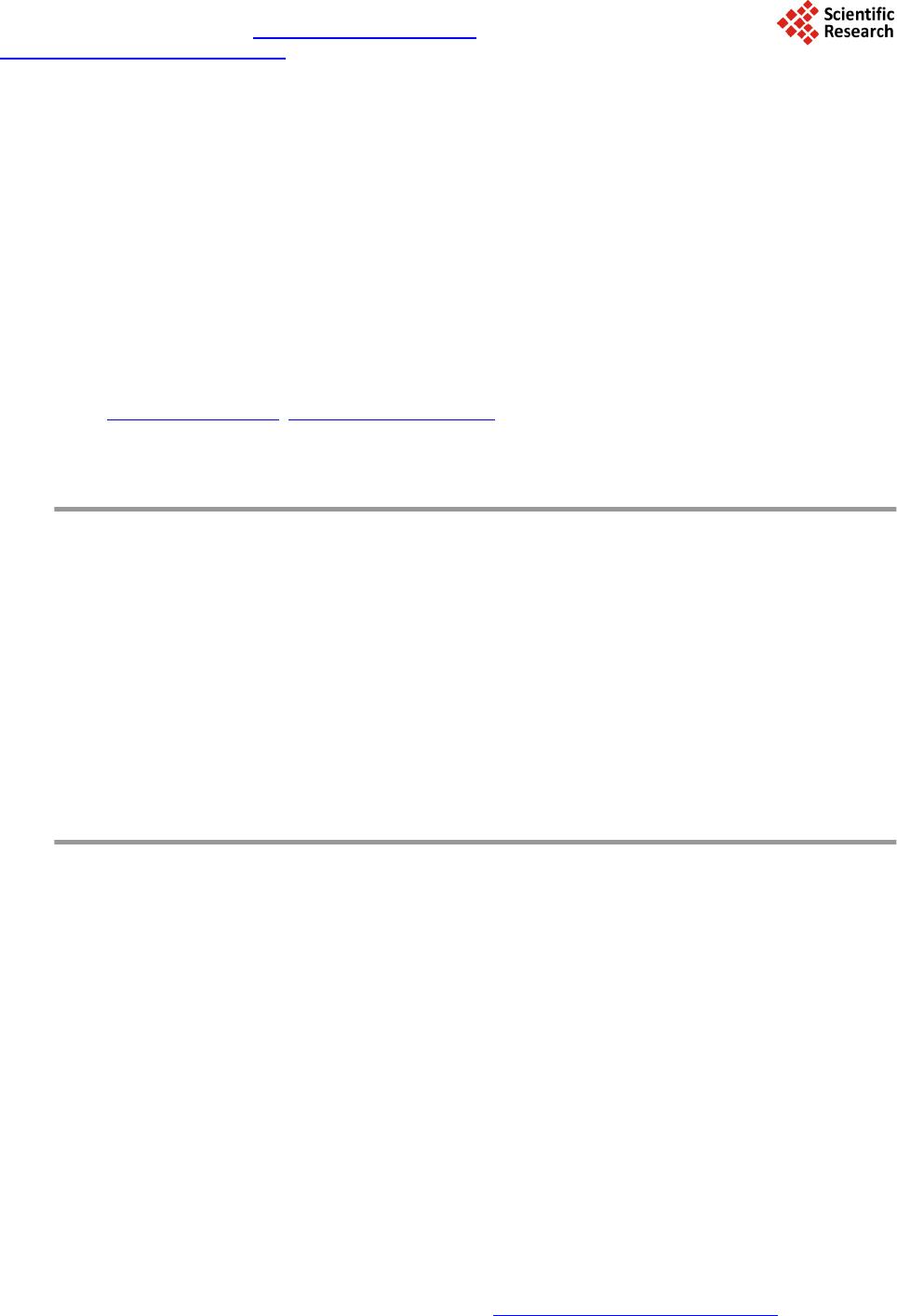 Open Journal of Social Sciences, 2014, 2, 30-38 Published Online July 2014 in SciRes. http://www.scirp.org/journal/jss http://dx.doi.org/10.4236/jss.2014.27006 How to cite this paper: Yang, Y. and Copeland, L. (2014) The Cross-Sectional Risk Premium of Decomposed Market Volatili- ty in UK Stock Market. Open Journal of Social Sciences, 2, 30-38. http://dx.doi.org/10.4236/jss.2014.27006 The Cross-Sectional Risk Premium of Decomposed Market Volatility in UK Stock Market Yan Yang, Laurence Copeland Cardiff Business School, Cardiff University, Car diff, UK Email: yangy16@cardiff.ac.uk, grace_yang@hotmail.co.uk Received May 2014 Abstract We decompose UK market volatility into short- and long-run components using EGARCH compo- nent model and examine the cross-sectional prices of the two components. Our empirical results suggest that these two components are significantly priced in the cross-section and the negative risk premia are consistent with the existing literature. The Fama-French three-factor model is improved by the inclusion of the two volatility components. However, our ICAPM model using market excess return and the decomposed volatility components as state variables compares infe- riorly to the traditional three-factor model. Keywords ICAPM, Decomposition of Stock Volatility 1. Introduction The most fundamental and best known model in asset-pricing theory is the Capital Asset Pricing Model (CAPM) which is essentially a “single factor” model of portfolio returns. However, the assumption of a single risk factor (market beta) limits the validity of this model and the effects of other risk factors have put this model under crit- icism. Specially, in the late 1970s, a research begins to uncover variables like size, various price ratios, and mo- mentum that add to the explanation of average returns associated with . [1] present evidence that beta almost has no explanatory. Their study demonstrates that size and book-to-mar- ket equity (BE/ME), combined to capture the cross-sectional variation in average stock returns together with the market , leverage, and earnings-price (E/P) ratios. [2] further suggest that the traditional CAPM does not ac- count for returns of size and book-to-market sorted portfolios. They show that there are common return factors related to size and BE/ME that help capture the cross-section of average stock returns in a way that is consistent with multifactor asset-pricing models. [3] report the momentum effect that is left unexplained by the three-factor model. [4] constructs a 4-factor model by including a momentum factor to three-factor model to capture the one-year momentum anomaly. FF
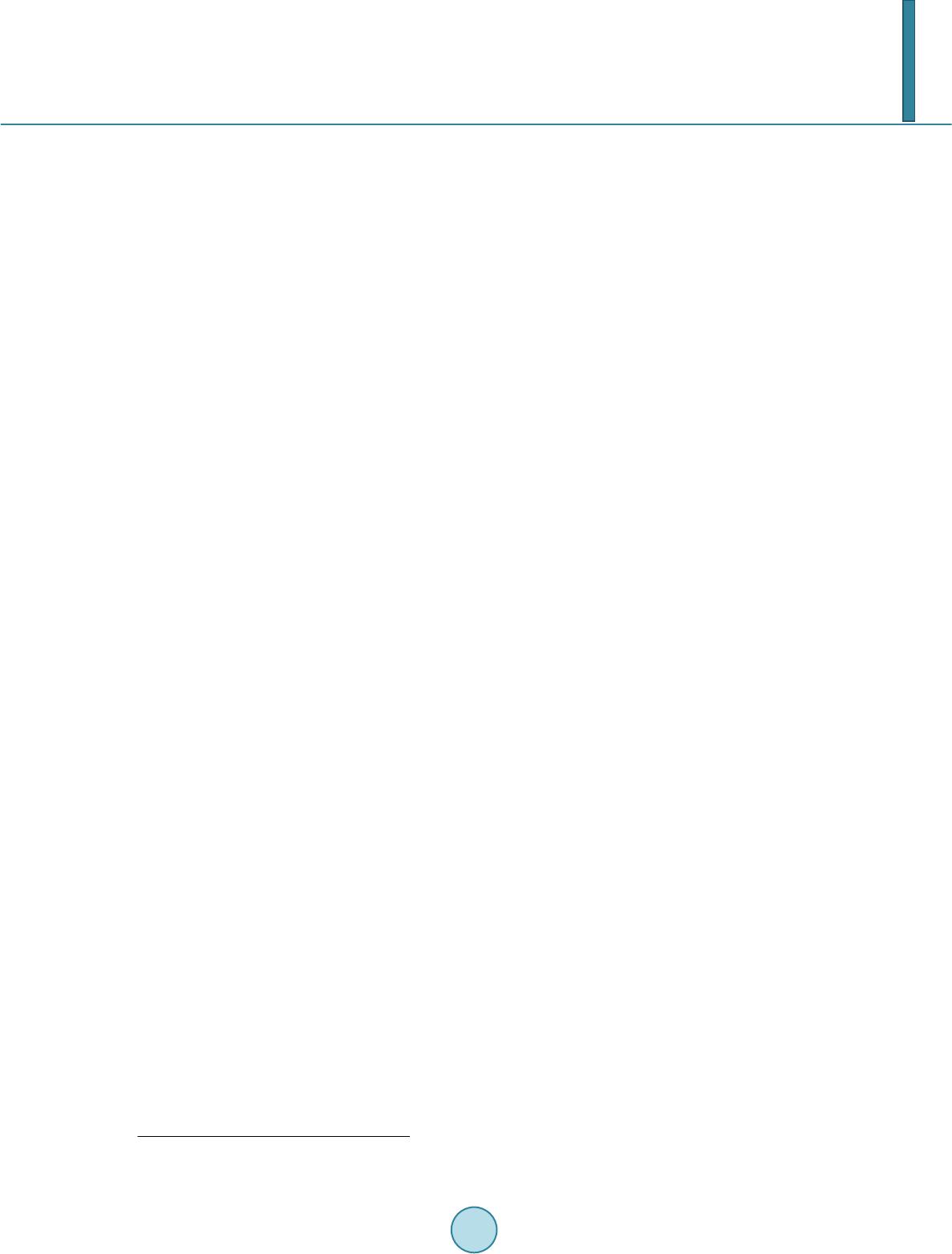 Y. Yang, L. Copeland three -factor model and Carhart’s four-factor model have been widely accepted and employed in empirical ana- lyses. In contrast to the lack of theoretical support of three (or four)-factor model, alternative responses to the poor performance of CAPM are to make modifications to the standard CAPM. Among all the developments, inter- temporal CAPM and conditional CAPM are most widely applied and various further extensions are proposed to better interpret the risk-return relation and portfolio structure. The advocates of conditional CAPM argue that the poor empirical performance of CAPM might be due to the failure to account for time-variation in conditional moments. Conditional CAPM tries to preserve the single fac- tor structure of the standard CAPM and assumes that all investors have the same conditional expectations for their asset returns. The ICAPM of [5] suggests that when there is stochastic variation in investment opportunities, asset risk pre- mia are not only determined by covariation of returns with the market return, but also associated with innova- tions in the state variables that describe the investment opportunities. [6] & [7] points out, in cross-sectional as- set pricing studies, the factors in the model should be related to innovations in state variables that forecast future investment opportunities. There is no doubt that stock market volatility changes over time, but whether or not volatility represents a priced risk factor remains less certain. [6] & [7] tests the ICAPM and shows that investors care about risks both from the market return and from changes in forecasts of future market returns. Time-varying market volatility induces changes in the investment opportunity set by changing the expectation of future market returns, or by changing the risk-return trade off. [8] develops the ICAPM in a framework in which the conditional means and variances of state variables are time varying and reflect changes in the investment opportunity set. Risk-averse investors tend to hedge against exposures to future market volatility. [9] set up a standard two-factor pricing kernel with the market return and stochastic volatility as factors. They show that market volatility is a significant cross-sectional asset pricing factor. [10] develop a new specification to model volatility process based on GARCH. They decompose volatility into permanent and transitory components. Following [10], the component GARCH model is applied to nu mer- ous economic areas and different countries1. [ 11] incorporate the component GARCH model of [10] and the EGARCH model of [12] to build up a new specification of volatility dynamics, the EGARCH component model. They present that the short- and long-run volatility components have negative, highly significant prices of risk which is robust across sets of portfolios, sub -periods, and volatility model specifications. The motivation for this paper stems from the fact that there are a growing number of papers dealing with the decomposition of the market volatility into components using Engle and Lee’s component GARCH Model. However, to my best knowledge, no studies examine the cross-sectional effect of the two decomposed compo- nents of market volatility, especially on UK stock market. Contrary to the existing empirical studies that simply employ Engle and Lee’s component GARCH model to explore the time-series effect of volatility, this thesis at- tempts to understand the cross-sectional effects of the transitory and permanent components of volatility. Fur- thermore, the EGARCH component framework is implemented together with the simple GARCH component model. Although Adrian and Rosenberg demonstrate that the short- and long-run volatility components are sig- nificantly priced in US stock market and their volatility components model compares favorably to the traditional CAPM, Fama-French model and several other model specifications, we are less confident that their volatility components are well priced in UK stock market and the superiority of their model. To test these, we apply the [11] decomposition of market risk to UK stock market and investigate the pricing of short- and long-run volatility risk in the cross-section of stock returns. The object of this chapter is threefold. First, we attempt to both determine whether the two components of market volatility are priced risk factors and estimate the prices of these components. Second, we try to examine whether the short- and long-run component model remains superior in UK stock markets. Third, we examine the robustness of the volatility component model across sets of portfolios, sub-periods, and model specifications. The reminder of this article is organized as follows. Methodology and data description are presented in part II and part III. Empirical results are reported in part IV. The final section offers concluding remarks. Research include [13] on futures market; [14] on bond market; [15] exploring US stock market; [16] exploring middle- [17] exploring Malaysia stock market..
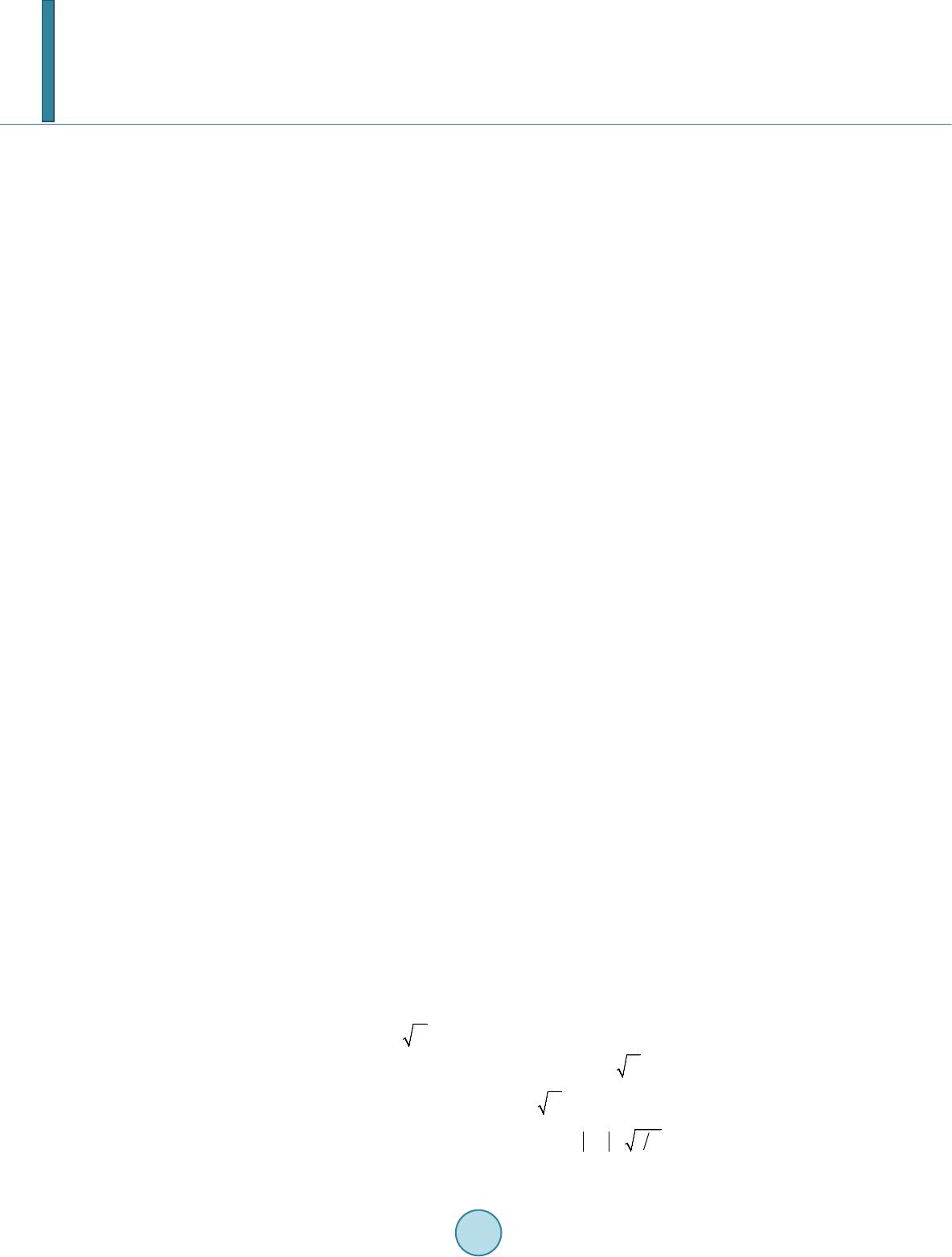 Y. Yang, L. Copeland 2. Methodology 2.1. Theoretical Motivation of the Pricing of Volatility Risk The most parsimonious pricing ICAPM framework in which to study the relationship between innovations of state variables and expected return is given as: ( ) ( ) ( ) 2 ,, M ERrra covRRcovRz λ ≈ +∆ (1) R denotes return in excess of the risk-free rate and is stock market excess return. The state variables are the variables that determine how well the investor can do in his maximization. is the elasticity of mar- ginal value with respect to wealth and is often referred as the coefficient of relative risk aversion. When investment opportunities vary over time, the multifactor models of [5] and [18] show that risk premia are associated with the conditional covariance between asset returns and innovations in state variables that de- scribe the time-variation of the investment opportunities. And hence, covariance with these innovations will therefore be priced. In the [6] & [7] ICAPM framework, investors care about risks both from the market return and from changes in forecasts of future market returns. [8] extends Campbell’s model and shows that risk-averse investors tend to directly hedge against changes in future market volatility. Motivated by these multifactor models, [9] express market volatility risk explicitly in Equation (1), ( ) ( ) ( ) ( ) 1112111,, 1 1 ,, K M t tttttktkt k ERrracov RRcov Rvcov Rf λλ ++++++ + = ≈+ ∆+ ∑ (2) where represent other factors other than aggregate volatility that induce changes in the investment opportu- nity set. For the convenience of empirical application, the above model can be written in terms of factor innovations. , and represent innovation in the market return, in aggregate volatility risk, and innovations to the other factors respectively. A true conditional multifactor representation of expected re- turns in the cross-section would take the following form: 1,1,,1,, ,1, 1 ()( )() K i iiMii ttmttmtvtt vtktktkt k Ra Rvf βγβ γβγ ++ ++ = =+− +−+− ∑ (3) where is the excess return on stock i. , and are the loadings on the excess market return, on market volatility risk, and on other risk factors, respectively. The conditional mean of the market return, ag- gregate volatility and other factors are denoted by , and , respectively. In equilibrium, the condi- tional mean of stock i is given by 1 () K i ii ii mm vvkk K a ER β λβλβλ = == ++ ∑ (4) where , and are the price of risk of the market factor, the price of aggregate volatility risk, and the prices of risk of the other factors, respectively. 2.2. Econometric Methodology—EGARCH Component Model As an extension of GARCH model, [10] introduce a component GARCH model where the conditional variance is decomposed into transitory and permanent components. In this two-component model, transitory and perma- nent components are used to capture short- and long-term effects of shock, respectively. Many studies find that two-component volatility model is superior to one-component specification in ex- plaining equity market volatility and the log-normal model of EGARCH performs better than square-root or af- fine volatility specifications. Appealed to the merits of the component GARCH and the EGARCH models, [11] incorporate the features of these two models and specify the dynamics of the market return in excess of risk-free rate and the conditional volatility as: Market return: (1a) Market volatility: (1b) Shor t-run component: ( ) 1451 61 2 t ttt s szz θθ θπ + ++ =++ − (1c)
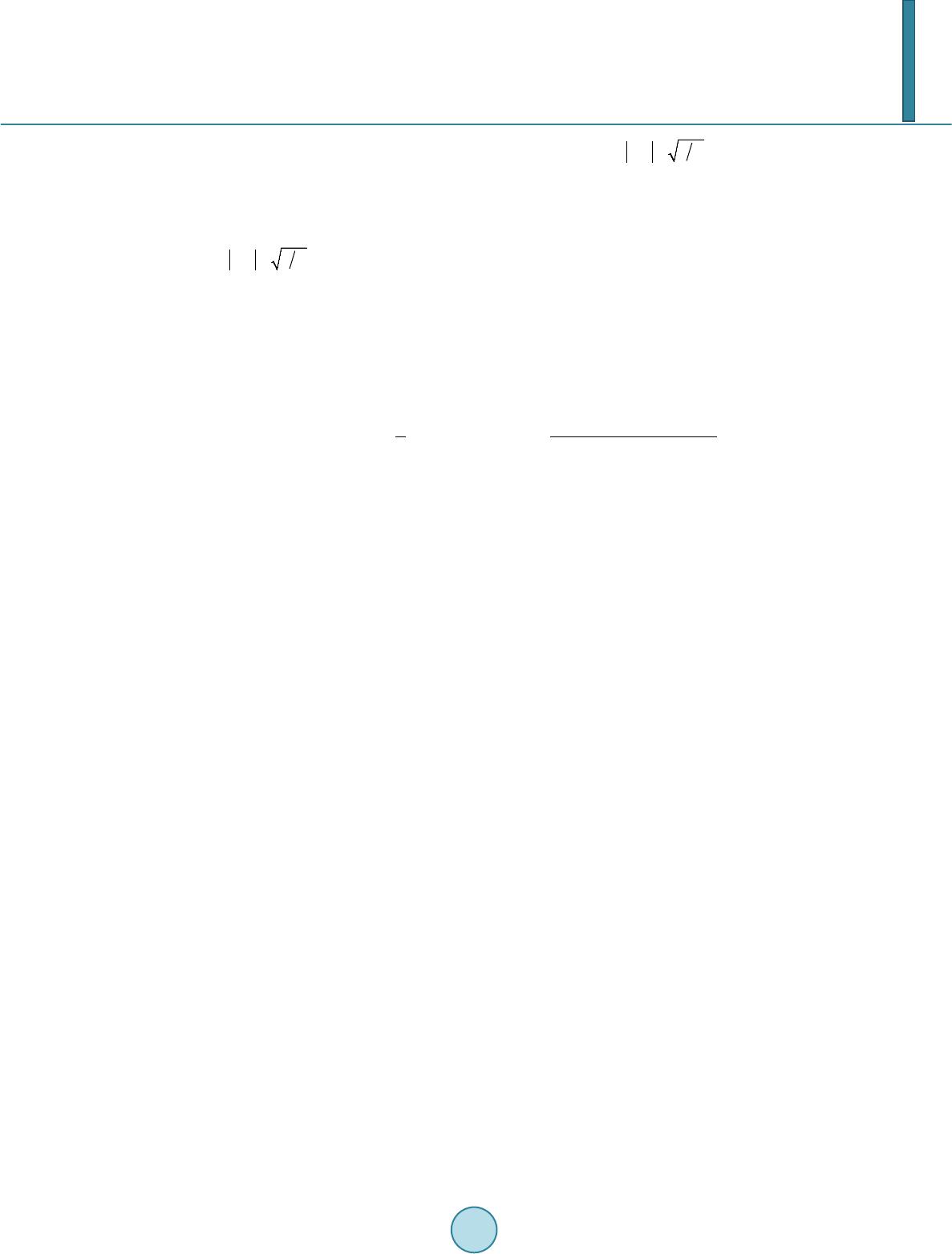 Y. Yang, L. Copeland Long-run component: 1789 1101 ( 2) ttt t lsz z θθ θθπ + ++ =++ +− (1d) and is the one-period expected excess return. The log-volatility is the sum of two components and . Each component is an AR(1) processes with its own rate of mean reversion. Without loss of generality, let be the slowly mean-reverting, long-run component and be the quickly mean-re- verting, short-run component . The unconditional mean of is normalized to zero. The terms in Equations (1c) and (1d) are the shocks to the volatility components. Their ex- pected values are equal to zero, given the normality of . For these error terms, equal-sized positive or nega- tive innovations results in the same volatility change, although the magnitude can be different for the short- and long -run components ( and ). The asymmetric effect of returns on volatility is allowed by including the market innovation in Equations (1c) and (1d) with corresponding coefficients and . The market model defined by Equations (1a)-(1d) converges to a continuous-time, two-factor stochastic vola- tility process. An advantage of this specification is that it can be estimated in discrete time via maximum like- lihood. The daily log-likelihood function is: ( ) ( ) ( ) 2 12 131 11 1 () 1 ;,|ln 2, 22 M Mt tt ttt tttt R sl fs lRslh θθ θ θπ −− −− − −− − =−− +− where t = 1,…, T is the daily time index, T is the total number of daily observations, and is the daily mar- ket excess return. 3. Data We estimate the EGARCH-component volatility model using daily excess returns. The daily data are used in order to improve the estimation precision and then aggregated to a monthly frequency for the cross-sectional analysis. FTSE All Share Index with its dividend yield is used as the proxy for the market return, , and one month return on Treasury Bills for the risk free rate, . The daily data range from 01/09/1980 to 31/12/2012 and are collected from LSPD (London Stock Price Database) and data stream. For the cross-sectional price test of the ICAPM, we apply the Fama and French 25 size and BE/ME portfolios and the portfolio returns and monthly factors are taken directly from [19] website. In the spirit of French’s pro- vision of the data to the international academic community, [19] construct the Fama and French size and B/M portfolio and the SMB (size factor), HML (value factor) and UMD (the momentum factor) of UK stock market and make all portfolios and factors downloadable from their website. 4. Empirical Results 4.1. Results of the Time Series Regression If the short- and long-run volatility components are also asset pricing factor, in the spirit of the ICAPM, the equilibrium pricing kernel thus depends on both short- and long-run volatility components as well as the excess market returns. Denote returns on asset i in excess of the risk free rate by . The equilibrium expected return for asset i is: ( )()()() 11111 111 , ,, iiMii ttttsttltt ERcov RRcov RscovRl λ λλ ++++ +++ = ++ (5) whe r e is the coefficient of relative risk aversion, and and are proportional to changes in the margin- al utility of wealth due to changes in the state variable and . Equation (5) shows that expected returns depend on three risk premia. The first risk premium arises from the covariance of the asset return with the excess market return, multiplied by relative risk a version . This is the risk-return tradeoff in a static CAPM. The second and third risk premia depend on the covariance of the asset return with the innovations in the short- and long-run factors. Market expected return is defined as (6) Examination of the risk-return relation is of fundamental importance to the asset pricing literature. Many au- thors either fail to detect a statistically significant intertemporal relation between risk and return of the market
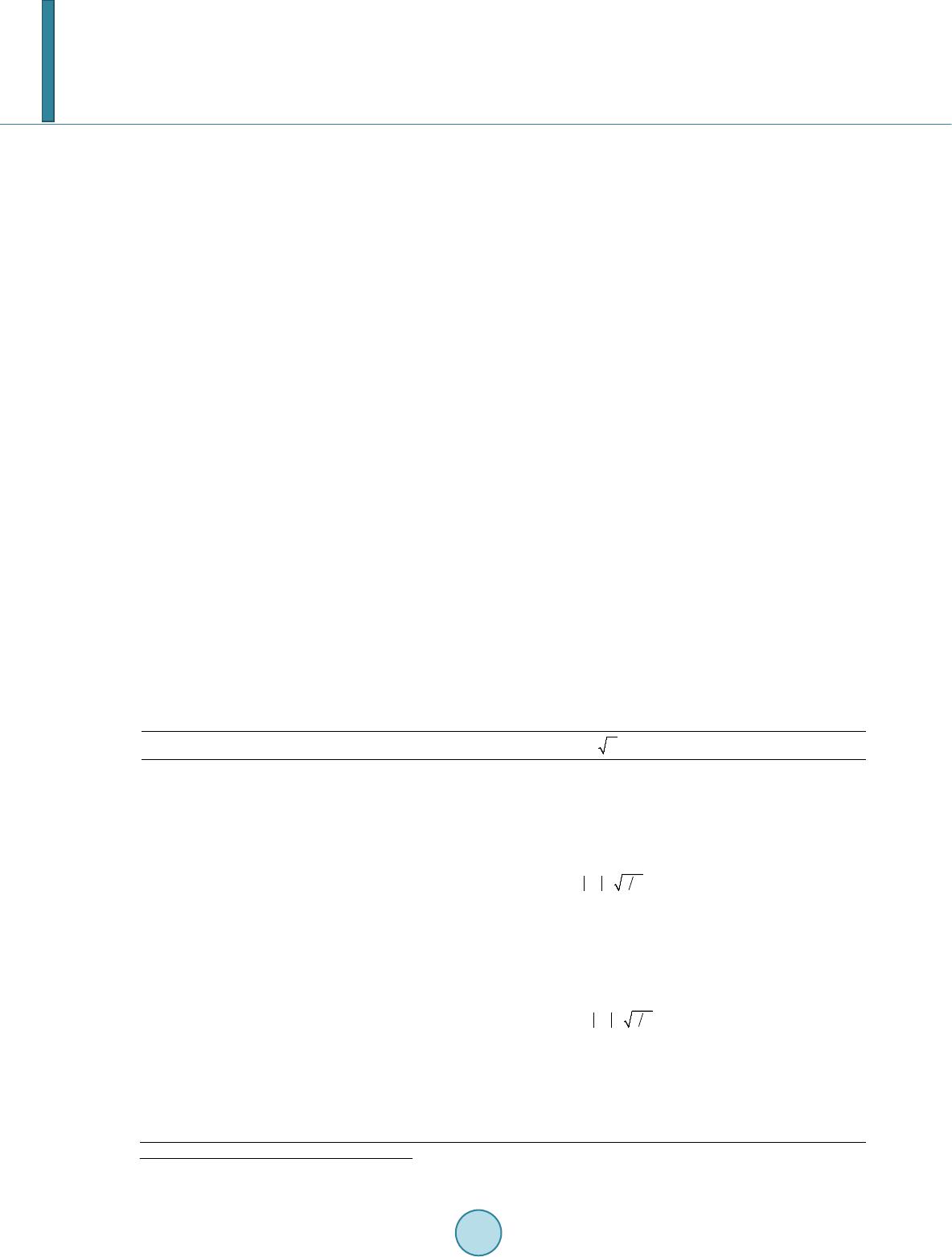 Y. Yang, L. Copeland portfolio or find a negative relation2. The estimation results for the volatility model are shown in Table 1. In the expected return equation, short-run volatility has a significant positive coefficient , while , the coefficient of long-run volatility is signifi- cantly negative. The market excess return thus depends positively on short-run volatility but negatively on long -run volatility. [11] identify a negative relationship between short-run volatility and market excess return but a positive relationship between long-run volatility and market excess return. Hence, short-run and long-run vola- tilities seem to have opposite effects on market excess return. This result might explain why previous research often have difficulty identifying a time-series relationship or mixed results of risk and return relation. We identify the short- and long-run components by their relative degrees of autocorrelation: the short-run vo- latility has an autoregressive coefficient of 0.807, and the long-run component has an autoregressive coefficient of 0.994. The long-run component is highly persistent. However, it’s not permanent, the hypothesis that is rejected at the 1% significant level. The estimate of is smaller than that of , which indicates the short -run volatility is less persistent compared to long-run component. Because the short- and long-run compo- nents determine log-volatility additively, it’s impossible to identify the means of the two components separately, and only the mean the long-run component is estimated. and detect the asymmetric effect on volatility. Both the estimates of and are significantly negative and large than minus one. This suggests that a pos- itive surprise increases both the short- and long-run volatility less than a negative surprise. Ljun g -box Q statistic suggests that there isn’t remaining serial correlation in the mean equation of the market excess return, while the ARCH-LM test reveals that there’s no additional ARCH effect exhibiting in the standar- dized residuals. 4.2. Results of the C ross -Sectional Tests Monthly data are employed to carry out the cross-sectional tests. The daily short- and long-run volatility com- ponents are obtained from the time-series regression. The 21-step out-of-sample forecasts of the short- and long -run components are made respectively. Daily innovations of the volatility components are calculated by subtracting the short- and long-run component from the forecasted values. The daily innovations in each month are then aggregated to a monthly frequency, and providing us with the monthly innovations of the short- and long -run component. Table 1. Time series estimation of the volatility components, daily, 01/09/1980 to 31/12/2012. Market excess return: 112 31 M tt ttt Rsl hz θθ θ ++ =+ ++ Coef. −0.007 0.267 −0.499 Std.err. 0.010 0.106 0.031 p-value 0.475 0.012 0.000 Short -run component: 1451 6 1 ( 2) t ttt s szz θθ θπ + ++ =++ − Coef. 0.807 −0.046 −0.009 Std.err. 0.030 0.004 0.042 p-value 0.000 0.000 0.829 Long-run component: 178 91101 ( 2) ttt t llz z θθ θθπ + ++ =++ +− Coef. 0.002 0.994 −0.032 0.028 Std.err. 0.000 0.001 0.001 0.002 p-value 0.000 0.000 0.000 0.000 2Examples include, [20], [21], and [22].
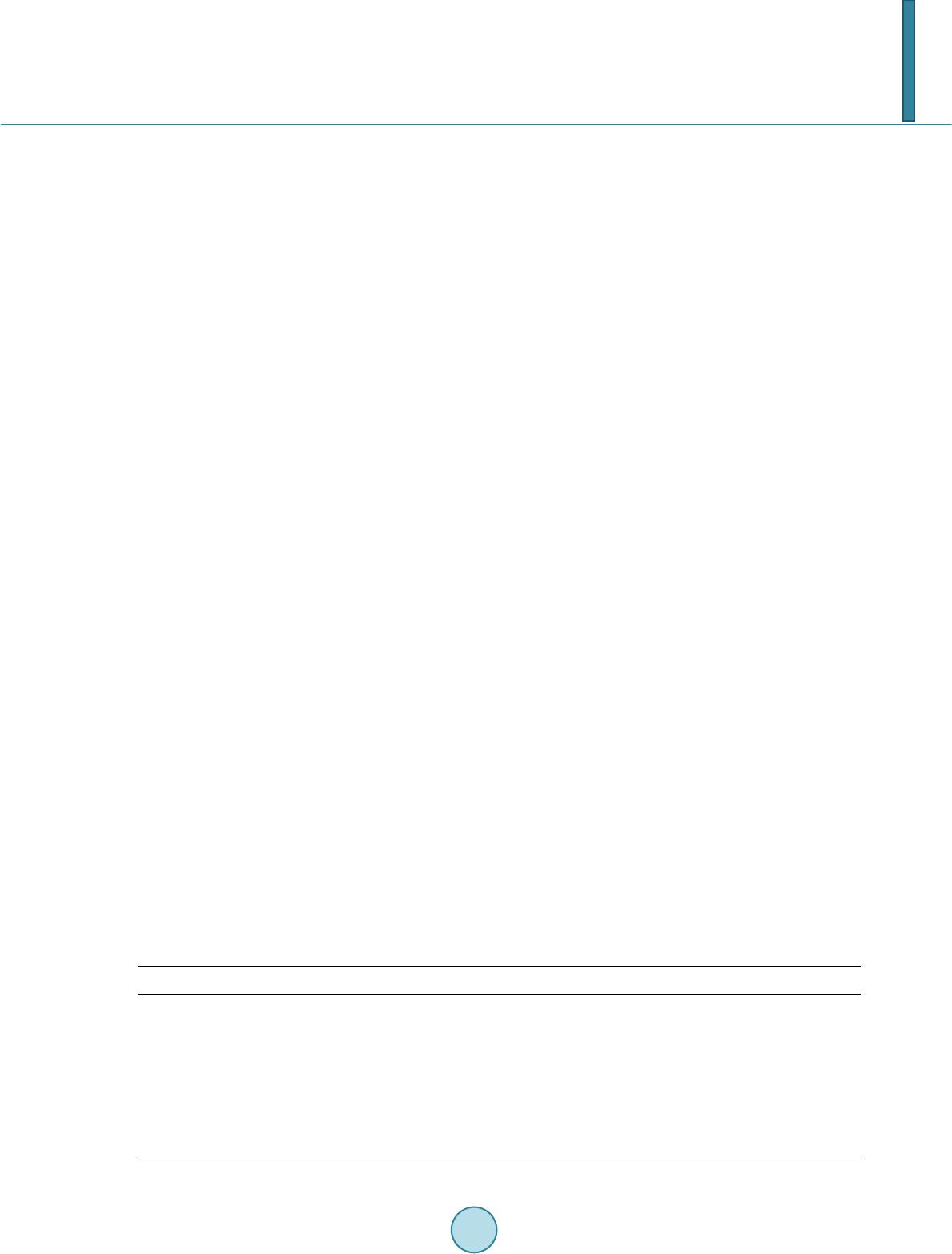 Y. Yang, L. Copeland 21 1([ ]), N mttt t sress Es − = = − ∑ (7) 21 1 ([ ]), N m ttt t lresl El − = = − ∑ (8) where sres and lres denote innovations of short- and long-run volatility respectively. N is the trading days in month m. The market variance v is aggregated to a monthly frequency, and the time series follows an AR(2) process. Hence, variance innovations (vres) are estimated as residuals of a monthly autoregressive process with two lags. The statistics of the innovations and the other pricing factors are summarized in Table 2. Under the ICAPM described in Section 2.1, the pricing kernel is a linear function of the excess return on the market portfolio and the innovations in the state variables, so that the unconditional risk premium on asset i may be written as: () iiiii mmssl l a ER β λβλβλ == ++ (9) where is the price of risk of the market factor, is the price of the short-run volatility risk, and the is the price of risk of the long-run volatility. The implication of the ICAPM for stock returns can be tested directly by implementing the two-stage Fa- ma-MacBeth procedure. We use the correction procedure proposed by [23] to account for the errors-in-variables problem. In the first stage, the 25 size and BE/ME portfolio returns are regressed on market excess returns, sres and lres. In the second stage, the portfolio returns are regressed on the estimated betas from the first stage to obtain the prices of market risk, short-run volatility risk and long-run volatility risk. Table 3 reports the pricing of volatility risk in the cross-section. The regressions of CAPM (column i), the FF three factor model (column ii), Carhart’s momentum model (column iii), and a model analogous to [9] with in- novations to market variance as risk factor (column iv) are also presented in Table 3. In column (v), we can see that the short- and long-run volatility components are significant pricing factors at the 5% level. The prices of short- and long-run components are −0.334 and −0.842 respectively. This implies that an asset with a short-run volatility beta of unity requires a 0.334% lower returns than an asset with zero ex- posure to the short-run component. These results are consistent with hypothesis that the cro ss-section of stock returns reflects exposure to volatility risk. In column (vi) and (vii), the prices of risk when the short- and long -run volatility enters as separate factors are reported. Each of the components has a negative price of risk at 10% significant level. [6]-[8] show that investors intend to hedge against market volatility and they are willing to pay a premium for market downside risk. The hedge motive is indicative of a negative price of market volatil- ity. The negative prices of the decomposed components of market volatility would suggest that risk-averse in- vestors tempt to hedge the overall exposures to market risk, no matter whether the exposures are transitory or persistent. However, the short- and long-run volatility factor compares inferiorly to the FF three-factor model and Car- hart momentum model, in terms of pricing performance. The RMSPE (root-mean-squared pricing error) are re- ported to evaluate the pricing performance of the different models. The models with FF factors (column ii and viii) achieve the lowest pricing errors. It’s worth noting that adding the two volatility components to FF three-factor model and momentum model reduces the pricing errors. This suggests that the volatility components and the Fama and French factors (or Table 2. Summary statistics of the monthly pricing fact o r s . Pricing factors Mean Std. Dev. Skewness Kurtosis Short -run volatility(sres) 0.000 1.064 −0.309 3.192 Long-run volatility(lres ) 0.000 2.989 1.697 11.083 Market variance (vres) 0.000 14.520 4.429 47. 87 Value factor (HM L) 0.004 0.037 0.270 11.092 Size factor (SMB) 0.001 0.034 0.712 7.200 Momentum factor (UMD) 0.008 0.042 −1.258 10.844
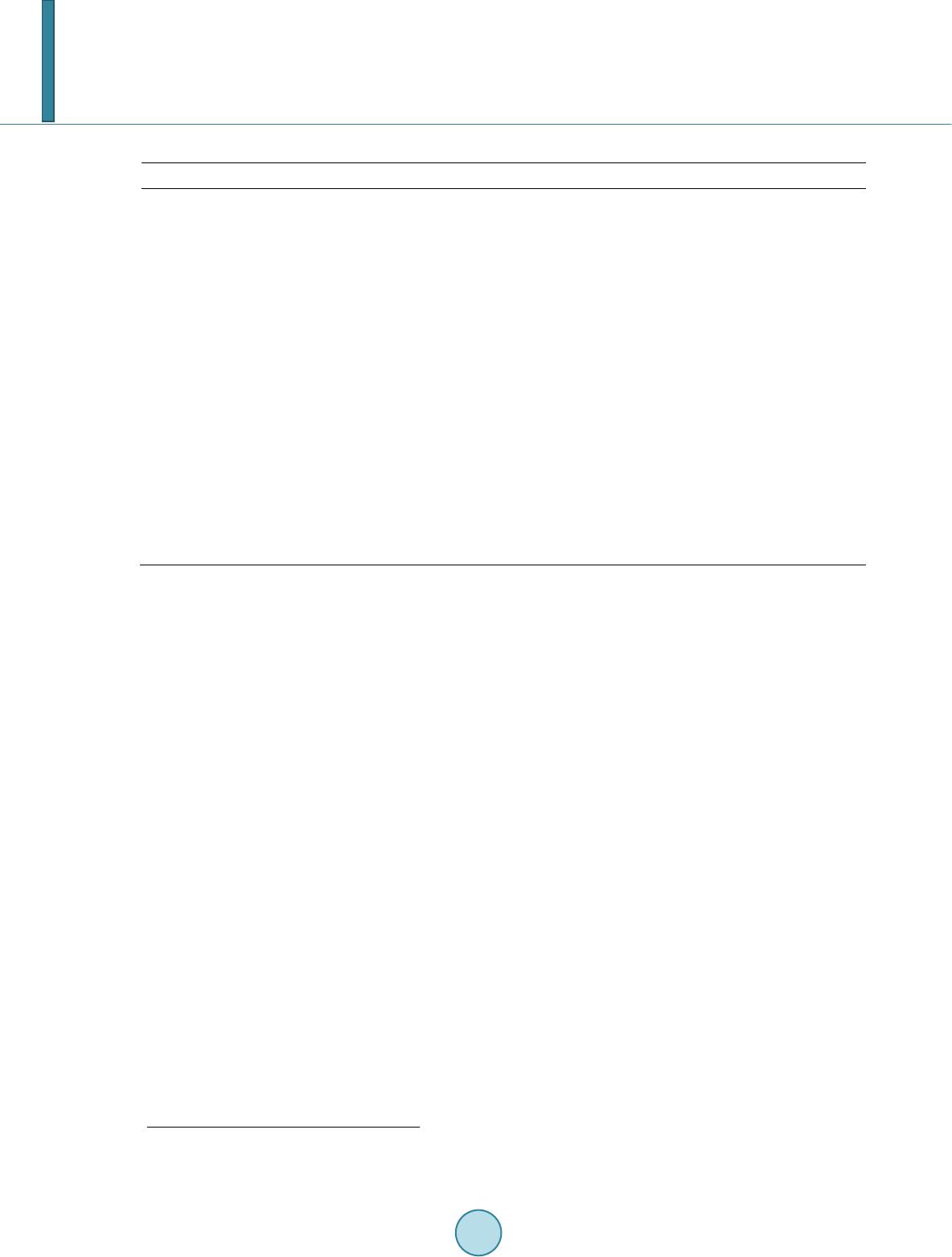 Y. Yang, L. Copeland Table 3. Pricing the cross section of the 25 size and B/M sorted portfolios. (i) (ii) (iii) (iv) (v) (vi) (vii ) (viii) (ix) Excess market return Coef. 0.596 0.454 0.498 0.439 0.453 0.608 0.465 0.443 0.438 p-valu e 0.033 0.073 0.073 0.083 0.073 0.026 0.066 0.080 0.084 Short -run volatility (sres) Coef. −0.334 −0.265 −0.232 −0.287 p-valu e 0.047 0.097 0.091 0.062 Long-run volatility (lres) Coef. −0.842 −0.602 −0.623 −0.689 p-valu e 0.034 0.071 0.074 0.057 Market variance (vres) Coef. −3.251 p-valu e 0.049 Value factor (HML) Coef. 0.518 0.518 p-valu e 0.025 0.024 Size factor (SMB) Coef. 0.165 0.156 p-valu e 0.386 0.212 Momentum factor Coef. 0.890 0.801 (UMD) p-value 0.029 0.076 RMSPE 0.210 0.120 0.142 0.245 0.188 0.210 0.197 0.113 0.138 This table reports the two-stage cr oss-sectional regression results for the 25 size and B/M sorted portfolios under various model specifications, in- cluding ICAPM, FF three-factor model, and the CAPM. The t-ratios are calculated using Jagannathan and Wang (1998) and Newey and West (1987) procedures to account for the estimation errors in first-stage estimation and correct for the possible heteroskedasticity and autocorrelation. The cor- responding p-values are reported according to the adjusted t-ratios. Root mean square pricing errors (RMPSE) are reported. momentum factors) capture some orthogonal source of priced risk. The first-stage FM regression shows that the factor loadings have significant variation across the size di- mension, however but less significant varation across the B/M dimension3. Table 4 shows the risk premium on the 25 size and BE/ME portfolios. The price spread of market risk between small firms and large firms is −0.107, which translate into an annualized premium spread due to market risk of −1.3% per year. Similarly, the annualized premium spreads between small and large firms due to short -run volatility and long-run volatility are −1.76% and 4.14% respectively. Only the risk premium of the long -run volatility is positive, but with much bigger magnitude. Hence, combining the three risk-premium dif- ferences yields an average excess risk premium for small firm relative to large firms of 1.08%. The analysis suggests that the size effect of small cap firms earning higher risk adjusted returns may be attributed to the long -run volatility component. The difference of annualized risk premium between the high B/M firms and low B/M firms due to the risk of short -run volatility component is 0.50% per year, while the difference due to the long-run component is 0.33% per year. Hence, the short- and long-run volatility components have the same effect on the B/M firm portfolio. Combining the value spread due to market risk, the total effect that the high B/M firm portfolio earns 1.32% higher risk premium per year relative to low B/M firm portfolio. The analysis suggests that the B/M effect that high B/M firms earn higher returns may be explained by both the short- and long-run volatility components. 4.3. Robustness Tests4 In order to test the robustness of the results we find above, we proceed the following tests: 1) Estimate the models using three sub-periods of the whole sample periods. 2) The cross-sectional procedure is regressed on returns of different portfolios sorted by other criteria. 3) Different mean equations are specified to test the EGARCH component model. 4) We compare EGARCH component model with GARCH and GARCH component model by evaluate their 3For reasons of space, the results are available upon a request from the author. 4The test procedures and results are available upon a request.
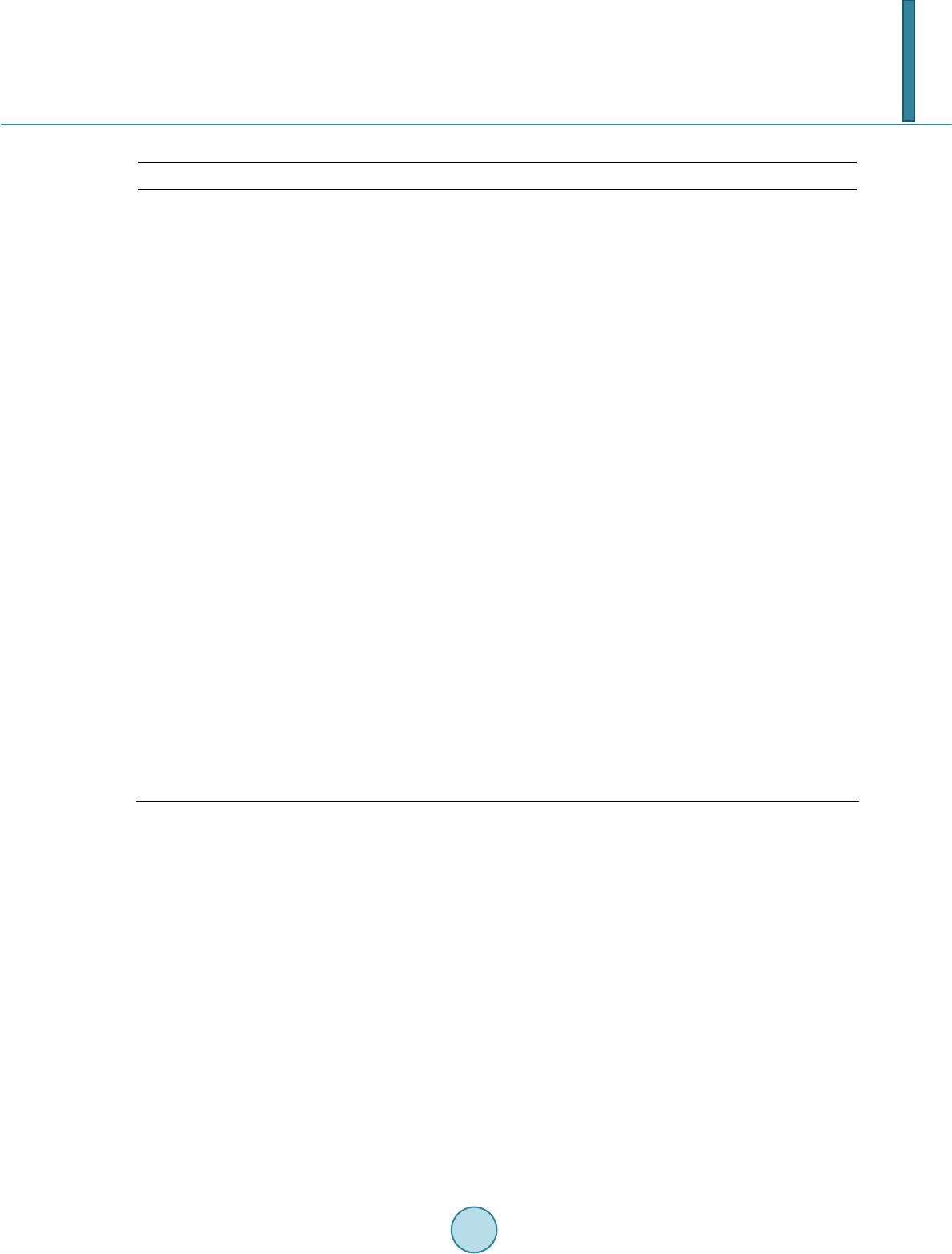 Y. Yang, L. Copeland Table 4. Factor risk premia of the 25 size and B/M sorted portfolios. Market risk premium Small Size 2 Size 3 Size 4 Big Average Gr o wt h 0.392 0.421 0.4489 0.443 0.427 0.427 B/M 2 0.332 0.380 0.423 0.417 0.435 0.398 B/M 3 0.305 0.368 0.403 0.396 0.480 0.390 B/M 4 0.327 0.357 0.404 0.480 0.431 0.400 Value 0.313 0.378 0.403 0.462 0.429 0.397 Average 0.334 0.381 0.416 0.439 0.441 0.402 Short -run volatility risk premium Small Size 2 Size 3 Size 4 Big Average Gr o wt h −0.213 −0.102 −0.124 −0.072 −0.069 −0.106 B/M 2 −0.247 −0.184 −0.059 0.042 −0.037 −0.092 B/M 3 −0.139 −0.186 −0.109 0.079 −0.080 −0.056 B/M 4 −0.152 0.045 0 .0 11 −0.084 0.054 −0.039 Value −0.125 −0.050 0.022 −0. 113 0.000 −0.065 Average −0.175 −0.096 −0.052 −0.029 −0.027 −0.076 Long-run volatility risk premium Small Size 2 Size 3 Size 4 Big Average Gr o wt h 0.357 0.212 0.104 0.249 −0.035 0.177 B/M 2 0.393 0.341 0.074 0.184 0.032 0.205 B/M 3 0.339 0.286 0.105 0.225 0.045 0.200 B/M 4 0.332 0.286 0.069 0.215 0.109 0.202 Value 0.361 0.341 0.116 0.287 −0.079 0.205 Average 0.357 0.293 0.094 0.232 0.014 0.198 This table reports the risk premia of portfolio returns on the market excess return, short-run volatility innovations and long-run volatility innovations. The risk premia are computed by multiplying factor loadings from Fama-Macbeth first-stage regression and prices of risk of Table 3, column V, second stage Fama-Mac Bethregressoin. performance in the cross-sectional pricing. The magnitudes of the prices of risk for the volatility components are fairly similar across different sets of assets and sample periods. The benchmark specification is superior to the alternatives with lowest root-mean-squared error. 5. Conclusions ICAPM predicts that financial asset risk premia are not only due to covariation of returns with the market excess return, but also associated with innovations in the state variables that describe the investment opportunities. Multifactor models of risk already predict that aggregate volatility should be a cross-sectional risk factor. [11] further decompose the aggregate volatility into a transitory and a permanent component. They conclude that the short - and long-run volatility components have negative, highly significant prices of risk using American stock market data. Applying decomposition of market risk to UK stock market, we find that the short- and long-run volatility components also have significantly negative prices of risk. The results are robust across sets of portfolios, sam- ple periods and model specifications. The short- and long-run volatility might provide an explanation of the size and value anomaly of financial market. Specifically, the size effect of small cap firms earning higher risk ad-
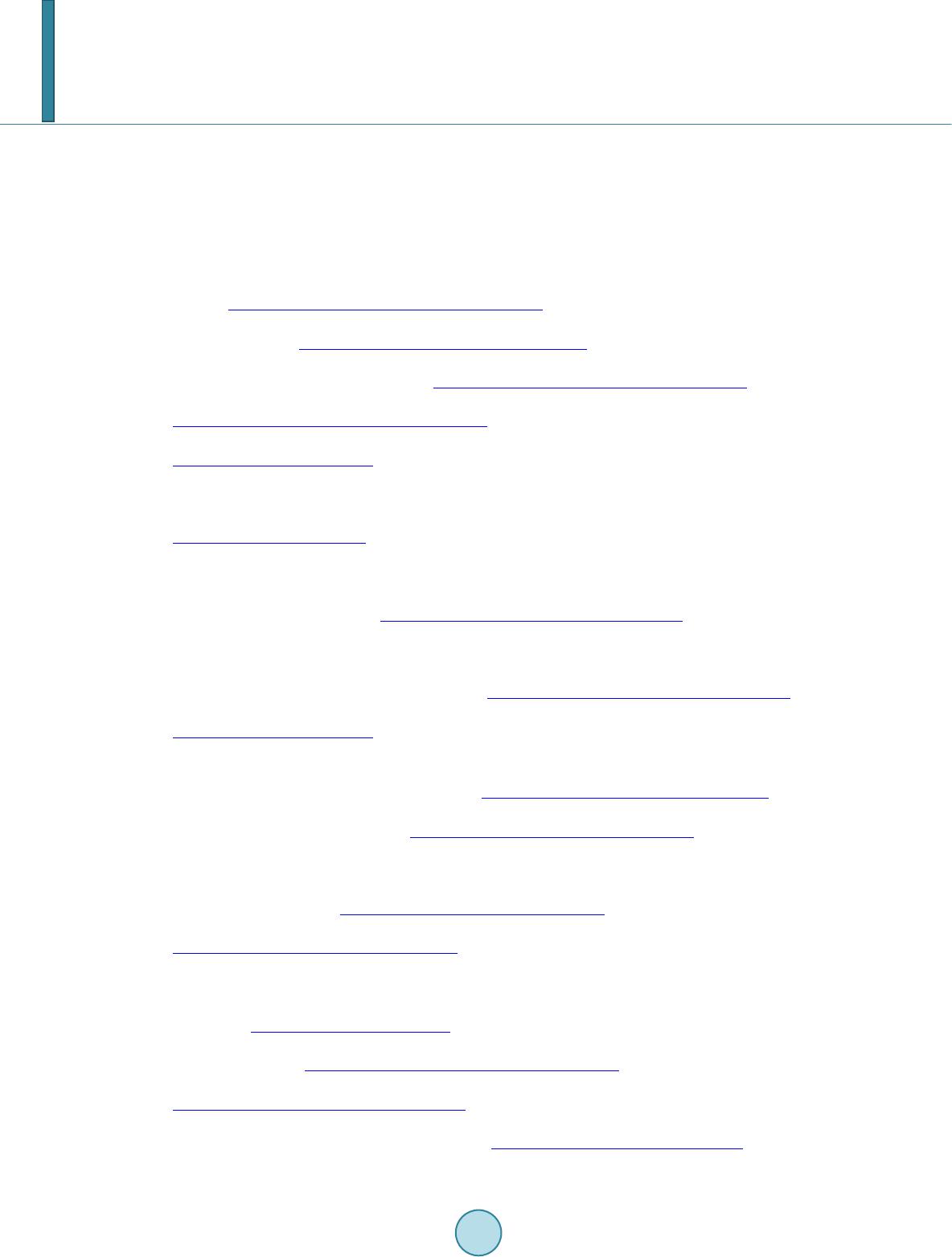 Y. Yang, L. Copeland justed returns may be attributed to the long-run volatility component. Whereas, the value effect that high B/M firms earn higher returns may be explained by both the short- and long-run volatility components. However, the performance of the decomposition model is superior to the Fama-French three factor model, and [9] market va- riance model. This might suggest further investigation and improvement on Adrian and Rosenberg’s volatility decomposition model. References [1] Fama, E.F. and French, K.R. (1992) The Cross-Section of Expected Stock Returns. The Journal of Finance, XLVII, 427-465. http://dx.doi.org/10.1111/j.1540-6261.1992.tb04398.x [2] Fama, E.F. and French, K.R . (199 3) Common Risk Factors in the Returns on Stocks and Bonds. Journal of Financial Economics, 33, 3-56. htt p:// d x.do i.o rg/1 0.10 16 /03 04-405X(93)90023-5 [3] Jegadeesh, N. and Titman, S. (199 3) Returns to Buying Winners and Selling Losers: Implications for Stock Market Ef- ficiency. The Journal of Finance, 48, 65-91. http://dx.doi.org/10.1111/j.1540-6261.1993.tb04702.x [4] Carhart, M.M. (1997) On Persistence in Mutual Fund Performance. The Journal of Finance, 52, 57-82. http://dx.doi.org/10.1111/j.1540-6261.1997.tb03808.x [5] Merton , R.C. (1973) An Intertemporal Capital Asset Pricing Model. Econometrica , 51, 867-887. http://dx.doi.org/10.2307/1913811 [6] Campbell, J.Y. (1993) Intertemporal Asset Pricing without Consumption Data. American Economic Review, 83, 487 - 512. [7] Campbell, J.Y. (1996) Understanding Risk and Return. Journal of Political Economy, 104, 298 -34 5. http://dx.doi.org/10.1086/262026 [8] Chen, J. (2002) In tertempo ral, CAPM and the Cross-section of Stock Returns. Working P aper , University of Southern California, Los Angeles. [9] Ang, A. , Hodrick, R. J., Xing, Y.H. and Zhang, X.Y. (2006) The Cross-Section of Volatility and Expected Returns. The Journal of Finance, LXI , 259-299. http://dx.doi.org/10.1111/j.1540-6261 .20 06 .00 836. x [10] Engl e, R.F. and Lee, G. (1999) A Permanent and Transitory Component Model of Stock Return Volatility. Cointegra- tion, Causality and Forecasting: A Festschrift in Honor of Clive W.J. Granger. Oxford University Press, New York. [11] Adr ian, T. and Rosenberg, J. (2008) Stock Returns and Volatility: Pricing the Short-Run and Long-Run Components of Market Risk. The Journal of Finance, 63, 2997 -30 30. http://dx.doi.org/10.1111/j.1540-6261.2008.01419.x [12] Nelson, D.B. (19 91 ) Conditional Heteroskedasticity in Asset Returns: A New Approach. Economet rica, 59 , 347 -370. http://dx.doi.org/10.2307/2938260 [13] Macmillan, H. and Tampoe, M. (2000) Strategic Management. Oxford University Press, Oxford. [14] Simó n, S.R. and Amalia, M.Z. (2012) Volatility in EMU Sovereign Bond Yields: Permanent and Transitory Compo- nents. Applied Financial Economics, 22, 14 53-14 64. http://dx.doi.org/10.1080/09603107.2012.661397 [15] Hertog, R.G.J.D. (1994) Pricing of Permanent and Transitory Volatility for US Stock Returns: A Composite GARCH Model. Economics Letters, 44, 421 -426. http://dx.doi.org/10.1016/0165-1765(94 )90 115 -5 [16] Zarour, B . A. and Siriopoulos, C .P . (2008) Transitory and Permanent Volatility Components: The Case of the Middle East Stock Markets. Review of Middle East Economics and Finance, 4, 80-92. [17] Mansor, H.I and Huson, J.A.A. (2014) Permanent and Transitory Oil Volatility and Aggregate Investment in Malaysia. Energy Po lic y, 67 , 552 -563. http://dx.doi.org/10.1016/j.enpol.2013.11.072 [18] Ross, S.A. (1976) The Arbitrage Theory of Capital Asset Pricing. Journal of Economic Theory, 13, 341-360. http://dx.doi.org/10.1016/0022-0531(76)90046-6 [19] Grego ry, A., Thar yan, R. and Huang, A. (2009) The Fama-French and Momentum Portfolios and Factors in the UK. University of Exeter Business School, XFI Centre for Finance and Investment Paper No. 09/05. [20] Baillie, R.T. and Degennaro, R.P. (1990) Stock Returns and Volatility. Journal of Financial and Quantitative Analysis, 25, 203-214. http://dx.doi.org/10.2307/2330824 [21] Whitelaw, R. (1994) Time Variations and Covariations in the Expectation and Volatility of Stock Returns. Journal of Finance, 49, 515-541. http://dx.doi.org/10.1111/j.1540-6261.1994.tb05150.x [22] Harve y, C.R. (2001) The Specification of Conditional Expectations. Journal of Empirical Finance, 8, 573-638. http://dx.doi.org/10.1016/S0927-5398(01)00036-6 [23] Jagannat han, R. and Wang, Z.Y. (1998) An Asymptotic Theory for Estimating Beta-Pricing Models Using Cross-Sec- tional Regression. Journal of Finance, 53, 1285-1309. ht tp :// dx. doi.o rg/ 10. 11 11/ 0022 -10 82.0 0053
|