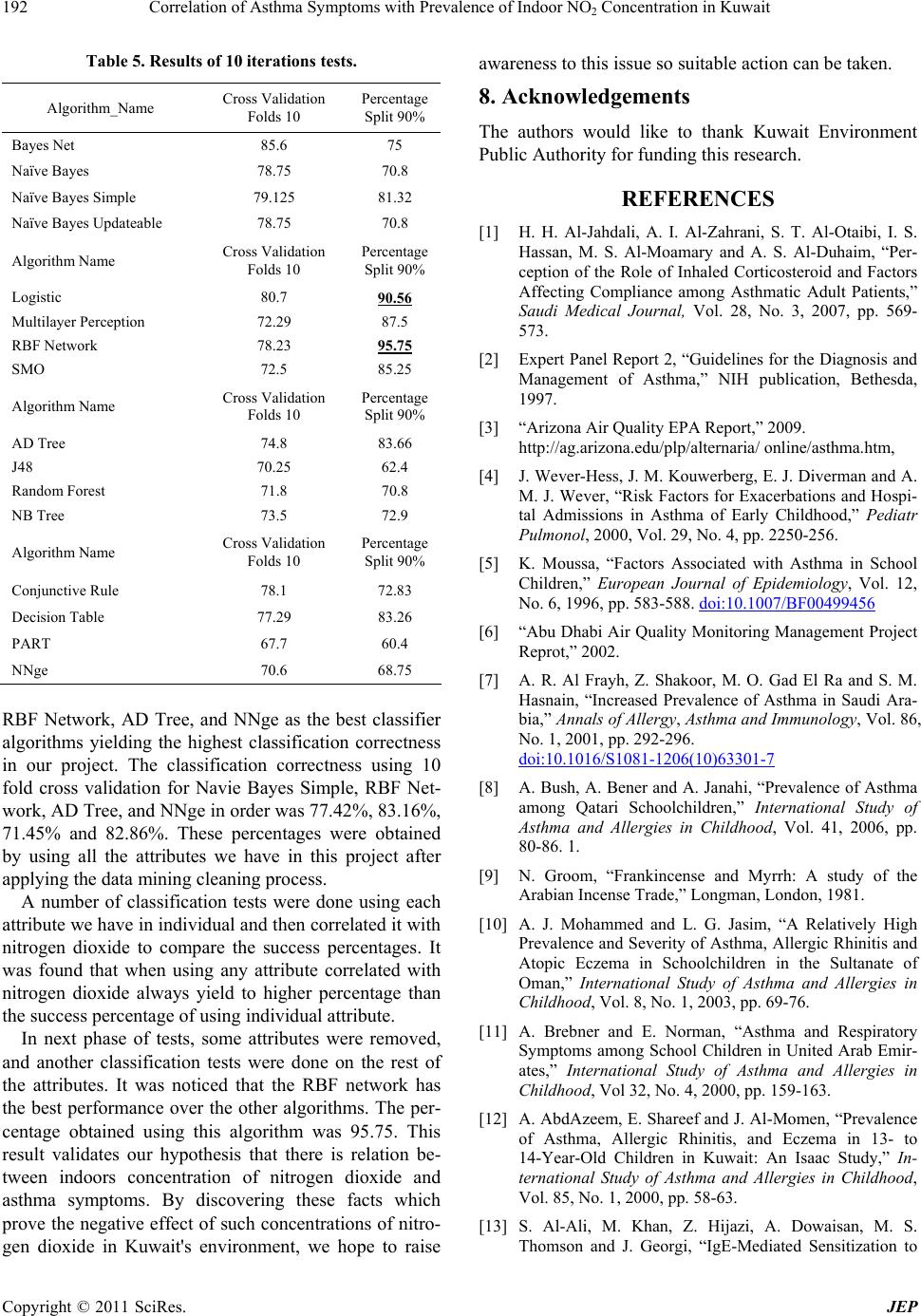
Correlation of Asthma Symptoms with Prevalence of Indoor NO Concentration in Kuwait
192 2
Table 5. Results of 10 iterations tests.
Algorithm_Name Cross Validation
Folds 10
Percentage
Split 90%
Bayes Net 85.6 75
Naïve Bayes 78.75 70.8
Naïve Bayes Simple 79.125 81.32
Naïve Bayes Updateable 78.75 70.8
Algorithm Name Cross Validation
Folds 10
Percentage
Split 90%
Logistic 80.7
90.56
Multilayer Perception 72.29 87.5
RBF Network 78.23 95.75
SMO 72.5 85.25
Algorithm Name Cross Validation
Folds 10
Percentage
Split 90%
AD Tree 74.8 83.66
J48 70.25 62.4
Random Forest 71.8 70.8
NB Tree 73.5 72.9
Algorithm Name Cross Validation
Folds 10
Percentage
Split 90%
Conjunctive Rule 78.1 72.83
Decision Table 77.29 83.26
PART 67.7 60.4
NNge 70.6 68.75
RBF Network, AD Tree, and NNge as the best classifier
algorithms yielding the highest classification correctness
in our project. The classification correctness using 10
fold cross validation for Navie Bayes Simple, RBF Net-
work, AD Tree, and NNge in order was 77.42%, 83.16%,
71.45% and 82.86%. These percentages were obtained
by using all the attributes we have in this project after
applying the data mining cleaning process.
A number of classification tests were done using each
attribute we have in individual and then correlated it with
nitrogen dioxide to compare the success percentages. It
was found that when using any attribute correlated with
nitrogen dioxide always yield to higher percentage than
the success percentage of using individual attribute.
In next phase of tests, some attributes were removed,
and another classification tests were done on the rest of
the attributes. It was noticed that the RBF network has
the best performance over the other algorithms. The per-
centage obtained using this algorithm was 95.75. This
result validates our hypothesis that there is relation be-
tween indoors concentration of nitrogen dioxide and
asthma symptoms. By discovering these facts which
prove the negative effect of such concentrations of nitro-
gen dioxide in Kuwait's environment, we hope to raise
awareness to this issue so suitable action can be taken.
8. Acknowledgements
The authors would like to thank Kuwait Environment
Public Authority for funding this research.
REFERENCES
[1] H. H. Al-Jahdali, A. I. Al-Zahrani, S. T. Al-Otaibi, I. S.
Hassan, M. S. Al-Moamary and A. S. Al-Duhaim, “Per-
ception of the Role of Inhaled Corticosteroid and Factors
Affecting Compliance among Asthmatic Adult Patients,”
Saudi Medical Journal, Vol. 28, No. 3, 2007, pp. 569-
573.
[2] Expert Panel Report 2, “Guidelines for the Diagnosis and
Management of Asthma,” NIH publication, Bethesda,
1997.
[3] “Arizona Air Quality EPA Report,” 2009.
http://ag.arizona.edu/plp/alternaria/ online/asthma.htm,
[4] J. Wever-Hess, J. M. Kouwerberg, E. J. Diverman and A.
M. J. Wever, “Risk Factors for Exacerbations and Hospi-
tal Admissions in Asthma of Early Childhood,” Pediatr
Pulmonol, 2000, Vol. 29, No. 4, pp. 2250-256.
[5] K. Moussa, “Factors Associated with Asthma in School
Children,” European Journal of Epidemiology, Vol. 12,
No. 6, 1996, pp. 583-588. doi:10.1007/BF00499456
[6] “Abu Dhabi Air Quality Monitoring Management Project
Reprot,” 2002.
[7] A. R. Al Frayh, Z. Shakoor, M. O. Gad El Ra and S. M.
Hasnain, “Increased Prevalence of Asthma in Saudi Ara-
bia,” Annals of Allergy, Asthma and Immunology, Vol. 86,
No. 1, 2001, pp. 292-296.
doi:10.1016/S1081-1206(10)63301-7
[8] A. Bush, A. Bener and A. Janahi, “Prevalence of Asthma
among Qatari Schoolchildren,” International Study of
Asthma and Allergies in Childhood, Vol. 41, 2006, pp.
80-86. 1.
[9] N. Groom, “Frankincense and Myrrh: A study of the
Arabian Incense Trade,” Longman, London, 1981.
[10] A. J. Mohammed and L. G. Jasim, “A Relatively High
Prevalence and Severity of Asthma, Allergic Rhinitis and
Atopic Eczema in Schoolchildren in the Sultanate of
Oman,” International Study of Asthma and Allergies in
Childhood, Vol. 8, No. 1, 2003, pp. 69-76.
[11] A. Brebner and E. Norman, “Asthma and Respiratory
Symptoms among School Children in United Arab Emir-
ates,” International Study of Asthma and Allergies in
Childhood, Vol 32, No. 4, 2000, pp. 159-163.
[12] A. AbdAzeem, E. Shareef and J. Al-Momen, “Prevalence
of Asthma, Allergic Rhinitis, and Eczema in 13- to
14-Year-Old Children in Kuwait: An Isaac Study,” In-
ternational Study of Asthma and Allergies in Childhood,
Vol. 85, No. 1, 2000, pp. 58-63.
[13] S. Al-Ali, M. Khan, Z. Hijazi, A. Dowaisan, M. S.
Thomson and J. Georgi, “IgE-Mediated Sensitization to
Copyright © 2011 SciRes. JEP