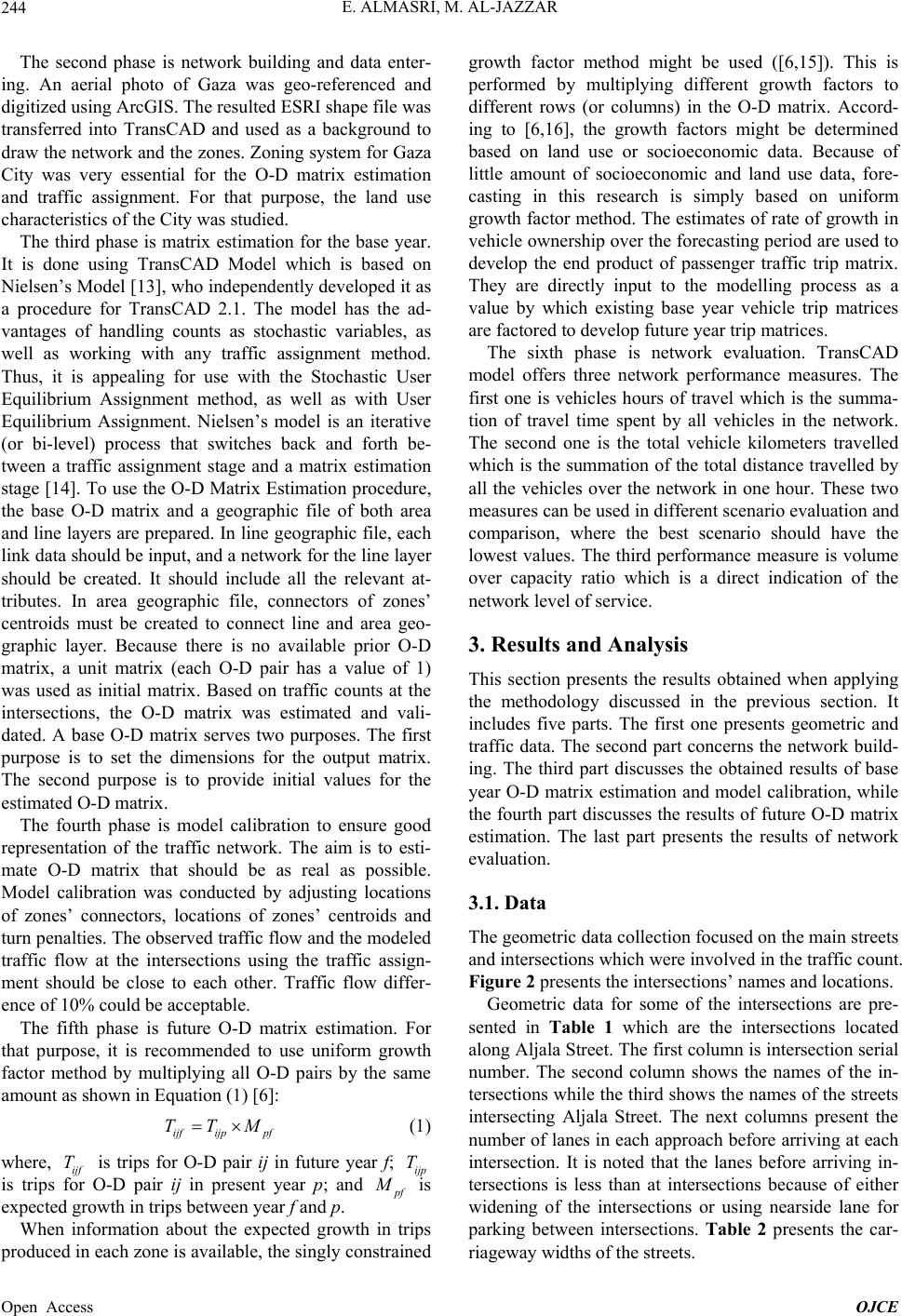
E. ALMASRI, M. AL-JAZZAR
244
The second phase is network building and data enter-
ing. An aerial photo of Gaza was geo-referenced and
digitized using ArcGIS. The resulted ESRI shape file was
transferred into TransCAD and used as a background to
draw the network and the zones. Zoning system for Gaza
City was very essential for the O-D matrix estimation
and traffic assignment. For that purpose, the land use
characteristics of the City was studied.
The third phase is matrix estimation for the base year.
It is done using TransCAD Model which is based on
Nielsen’s Model [13], who independently developed it as
a procedure for TransCAD 2.1. The model has the ad-
vantages of handling counts as stochastic variables, as
well as working with any traffic assignment method.
Thus, it is appealing for use with the Stochastic User
Equilibrium Assignment method, as well as with User
Equilibrium Assignment. Nielsen’s model is an iterative
(or bi-level) process that switches back and forth be-
tween a traffic assignment stage and a matrix estimation
stage [14]. To use the O-D Matrix Estimation procedure,
the base O-D matrix and a geographic file of both area
and line laye rs are prepar ed. In line geographic file, each
link data should be input, and a network for the line layer
should be created. It should include all the relevant at-
tributes. In area geographic file, connectors of zones’
centroids must be created to connect line and area geo-
graphic layer. Because there is no available prior O-D
matrix, a unit matrix (each O-D pair has a value of 1)
was used as initial matrix. Based on traffic counts at the
intersections, the O-D matrix was estimated and vali-
dated. A base O-D matrix serves two purposes. The first
purpose is to set the dimensions for the output matrix.
The second purpose is to provide initial values for the
estimated O-D matrix.
The fourth phase is model calibration to ensure good
representation of the traffic network. The aim is to esti-
mate O-D matrix that should be as real as possible.
Model calibration was conducted by adjusting locations
of zones’ connectors, locations of zones’ centroids and
turn penalties. The observed traffic flow and the modeled
traffic flow at the intersections using the traffic assign-
ment should be close to each other. Traffic flow differ-
ence of 10% could be acceptable.
The fifth phase is future O-D matrix estimation. For
that purpose, it is recommended to use uniform growth
factor method by multiplying all O-D pairs by the same
amount as shown in Equation (1) [6]:
ijf ijppf
TTM (1)
where, ijf is trips for O-D pair ij in future year f; ijp
is trips for O-D pair ij in present year p; and
T T
f
is
expected growth in trips between year f and p.
When information about the expected growth in trips
produced in each zone is available, the singly constrained
growth factor method might be used ([6,15]). This is
performed by multiplying different growth factors to
different rows (or columns) in the O-D matrix. Accord-
ing to [6,16], the growth factors might be determined
based on land use or socioeconomic data. Because of
little amount of socioeconomic and land use data, fore-
casting in this research is simply based on uniform
growth factor method. The estimates of rate of growth in
vehicle ownership over the forecasting period are used to
develop the end product of passenger traffic trip matrix.
They are directly input to the modelling process as a
value by which existing base year vehicle trip matrices
are factored to develop future year trip matrices.
The sixth phase is network evaluation. TransCAD
model offers three network performance measures. The
first one is vehicles hours of travel which is the summa-
tion of travel time spent by all vehicles in the network.
The second one is the total vehicle kilometers travelled
which is the summation of the total distance travelled by
all the vehicles over the network in one hour. These two
measures can be used in different scenario evaluation and
comparison, where the best scenario should have the
lowest values. The third performance measure is volume
over capacity ratio which is a direct indication of the
network level of service.
3. Results and Analysis
This section presents the results obtained when applying
the methodology discussed in the previous section. It
includes five parts. The first one presents geometric and
traffic data. The second part concerns the network build-
ing. The third part discusses the obtained results of base
year O-D matrix estimation and model calibration, while
the fourth part discusses the results of future O-D matrix
estimation. The last part presents the results of network
evaluation.
3.1. Data
The geometric data collection focused on the main streets
and intersections which were involved in th e traffic count.
Figure 2 presents the intersectio ns’ names and locations.
Geometric data for some of the intersections are pre-
sented in Table 1 which are the intersections located
along Aljala Street. The first column is intersection serial
number. The second column shows the names of the in-
tersections while the third shows the names of the streets
intersecting Aljala Street. The next columns present the
number of lanes in each approach before arriving at each
intersection. It is noted that the lanes before arriving in-
tersections is less than at intersections because of either
widening of the intersections or using nearside lane for
parking between intersections. Table 2 presents the car-
riageway widths of the streets.
Open Access OJCE