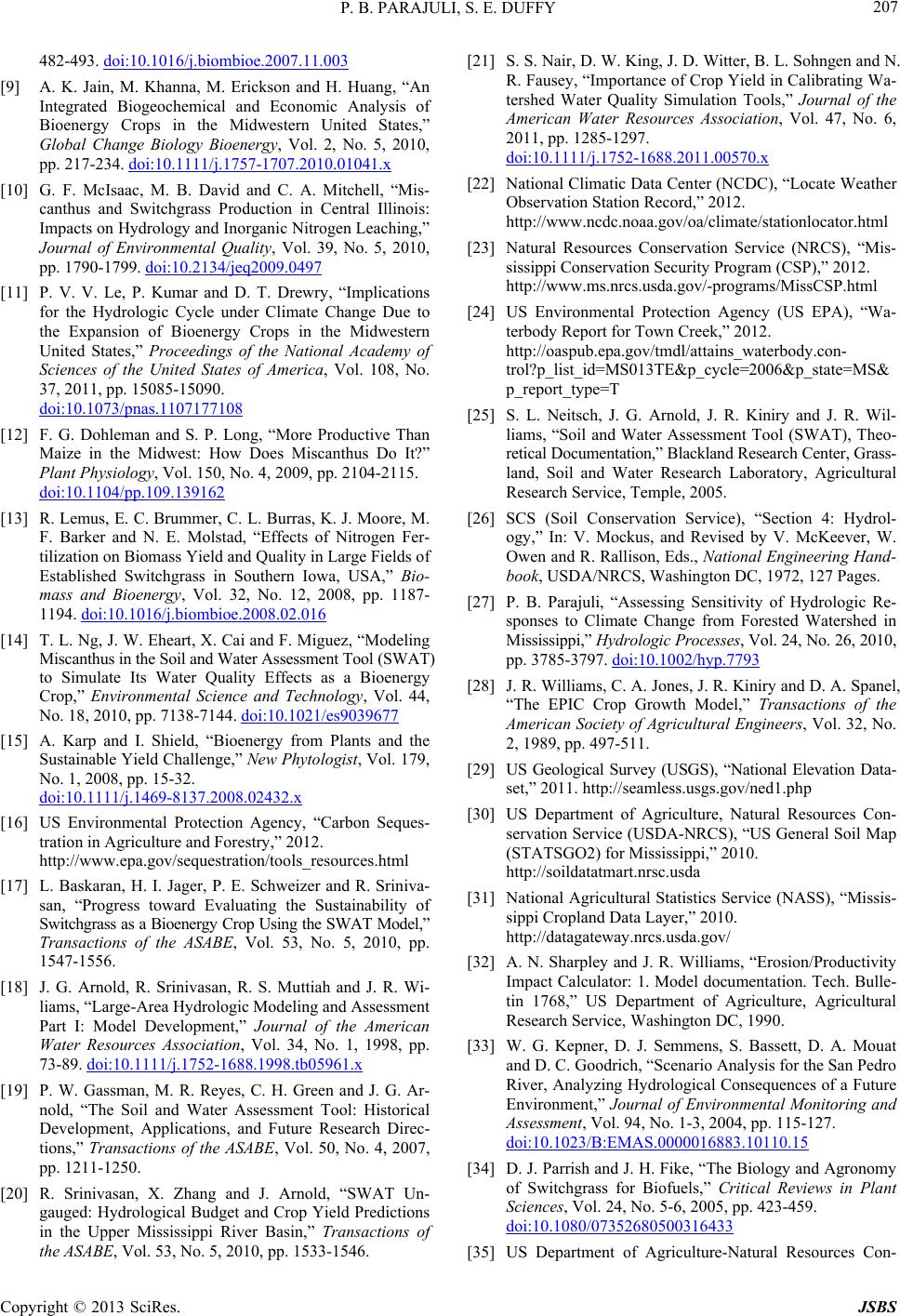
P. B. PARAJULI, S. E. DUFFY 207
482-493. doi:10.1016/j.biombioe.2007.11.003
[9] A. K. Jain, M. Khanna, M. Erickson and H. Huang, “An
Integrated Biogeochemical and Economic Analysis of
Bioenergy Crops in the Midwestern United States,”
Global Change Biology Bioenergy, Vol. 2, No. 5, 2010,
pp. 217-234. doi:10.1111/j.1757-1707.2010.01041.x
[10] G. F. McIsaac, M. B. David and C. A. Mitchell, “Mis-
canthus and Switchgrass Production in Central Illinois:
Impacts on Hydrology and Inorganic Nitrogen Leaching,”
Journal of Environmental Quality, Vol. 39, No. 5, 2010,
pp. 1790-1799. doi:10.2134/jeq2009.0497
[11] P. V. V. Le, P. Kumar and D. T. Drewry, “Implications
for the Hydrologic Cycle under Climate Change Due to
the Expansion of Bioenergy Crops in the Midwestern
United States,” Proceedings of the National Academy of
Sciences of the United States of America, Vol. 108, No.
37, 2011, pp. 15085-15090.
doi:10.1073/pnas.1107177108
[12] F. G. Dohleman and S. P. Long, “More Productive Than
Maize in the Midwest: How Does Miscanthus Do It?”
Plant Physiology, Vol. 150, No. 4, 2009, pp. 2104-2115.
doi:10.1104/pp.109.139162
[13] R. Lemus, E. C. Brummer, C. L. Burras, K. J. Moore, M.
F. Barker and N. E. Molstad, “Effects of Nitrogen Fer-
tilization on Biomass Yield and Quality in Large Fields of
Established Switchgrass in Southern Iowa, USA,” Bio-
mass and Bioenergy, Vol. 32, No. 12, 2008, pp. 1187-
1194. doi:10.1016/j.biombioe.2008.02.016
[14] T. L. Ng, J. W. Eheart, X. Cai and F. Miguez, “Modeling
Miscanthus in the Soil and Water Assessment Tool (SWAT)
to Simulate Its Water Quality Effects as a Bioenergy
Crop,” Environmental Science and Technology, Vol. 44,
No. 18, 2010, pp. 7138-7144. doi:10.1021/es9039677
[15] A. Karp and I. Shield, “Bioenergy from Plants and the
Sustainable Yield Challenge,” New Phytologist, Vol. 179,
No. 1, 2008, pp. 15-32.
doi:10.1111/j.1469-8137.2008.02432.x
[16] US Environmental Protection Agency, “Carbon Seques-
tration in Agriculture and Forestry,” 2012.
http://www.epa.gov/sequestration/tools_resources.html
[17] L. Baskaran, H. I. Jager, P. E. Schweizer and R. Sriniva-
san, “Progress toward Evaluating the Sustainability of
Switchgrass as a Bioenergy Crop Using the SWAT Model,”
Transactions of the ASABE, Vol. 53, No. 5, 2010, pp.
1547-1556.
[18] J. G. Arnold, R. Srinivasan, R. S. Muttiah and J. R. Wi-
liams, “Large-Area Hydrologic Modeling and Assessment
Part I: Model Development,” Journal of the American
Water Resources Association, Vol. 34, No. 1, 1998, pp.
73-89. doi:10.1111/j.1752-1688.1998.tb05961.x
[19] P. W. Gassman, M. R. Reyes, C. H. Green and J. G. Ar-
nold, “The Soil and Water Assessment Tool: Historical
Development, Applications, and Future Research Direc-
tions,” Transactions of the ASABE, Vol. 50, No. 4, 2007,
pp. 1211-1250.
[20] R. Srinivasan, X. Zhang and J. Arnold, “SWAT Un-
gauged: Hydrological Budget and Crop Yield Predictions
in the Upper Mississippi River Basin,” Transactions of
the ASABE, Vol. 53, No. 5, 2010, pp. 1533-1546.
[21] S. S. Nair, D. W. King, J. D. Witter, B. L. Sohngen and N.
R. Fausey, “Importance of Crop Yield in Calibrating Wa-
tershed Water Quality Simulation Tools,” Journal of the
American Water Resources Association, Vol. 47, No. 6,
2011, pp. 1285-1297.
doi:10.1111/j.1752-1688.2011.00570.x
[22] National Climatic Data Center (NCDC), “Locate Weather
Observation Station Record,” 2012.
http://www.ncdc.noaa.gov/oa/climate/stationlocator.html
[23] Natural Resources Conservation Service (NRCS), “Mis-
sissippi Conservation Security Program (CSP),” 2012.
http://www.ms.nrcs.usda.gov/-programs/MissCSP.html
[24] US Environmental Protection Agency (US EPA), “Wa-
terbody Report for Town Creek,” 2012.
http://oaspub.epa.gov/tmdl/attains_waterbody.con-
trol?p_list_id=MS013TE&p_cycle=2006&p_state=MS&
p_report_type=T
[25] S. L. Neitsch, J. G. Arnold, J. R. Kiniry and J. R. Wil-
liams, “Soil and Water Assessment Tool (SWAT), Theo-
retical Documentation,” Blackland Research Center, Grass-
land, Soil and Water Research Laboratory, Agricultural
Research Service, Temple, 2005.
[26] SCS (Soil Conservation Service), “Section 4: Hydrol-
ogy,” In: V. Mockus, and Revised by V. McKeever, W.
Owen and R. Rallison, Eds., National Engineering Hand-
book, USDA/NRCS, Washington DC, 1972, 127 Pages.
[27] P. B. Parajuli, “Assessing Sensitivity of Hydrologic Re-
sponses to Climate Change from Forested Watershed in
Mississippi,” Hy drologic Processes, Vol. 24, No. 26, 2010,
pp. 3785-3797. doi:10.1002/hyp.7793
[28] J. R. Williams, C. A. Jones, J. R. Kiniry and D. A. Spanel,
“The EPIC Crop Growth Model,” Transactions of the
American Society of Agricultural Engineers, Vol. 32, No.
2, 1989, pp. 497-511.
[29] US Geological Survey (USGS), “National Elevation Data-
set,” 2011. http://seamless.usgs.gov/ned1.php
[30] US Department of Agriculture, Natural Resources Con-
servation Service (USDA-NRCS), “US General Soil Map
(STATSGO2) for Mississippi,” 2010.
http://soildatatmart.nrsc.usda
[31] National Agricultural Statistics Service (NASS), “Missis-
sippi Cropland Data Layer,” 2010.
http://datagateway.nrcs.usda.gov/
[32] A. N. Sharpley and J. R. Williams, “Erosion/Productivity
Impact Calculator: 1. Model documentation. Tech. Bulle-
tin 1768,” US Department of Agriculture, Agricultural
Research Service, Washington DC, 1990.
[33] W. G. Kepner, D. J. Semmens, S. Bassett, D. A. Mouat
and D. C. Goodrich, “Scenario Analysis for the San Pedro
River, Analyzing Hydrological Consequences of a Future
Environment,” Journal of Environmental Monitoring and
Assessment, Vol. 94, No. 1-3, 2004, pp. 115-127.
doi:10.1023/B:EMAS.0000016883.10110.15
[34] D. J. Parrish and J. H. Fike, “The Biology and Agronomy
of Switchgrass for Biofuels,” Critical Reviews in Plant
Sciences, Vol. 24, No. 5-6, 2005, pp. 423-459.
doi:10.1080/07352680500316433
[35] US Department of Agriculture-Natural Resources Con-
Copyright © 2013 SciRes. JSBS