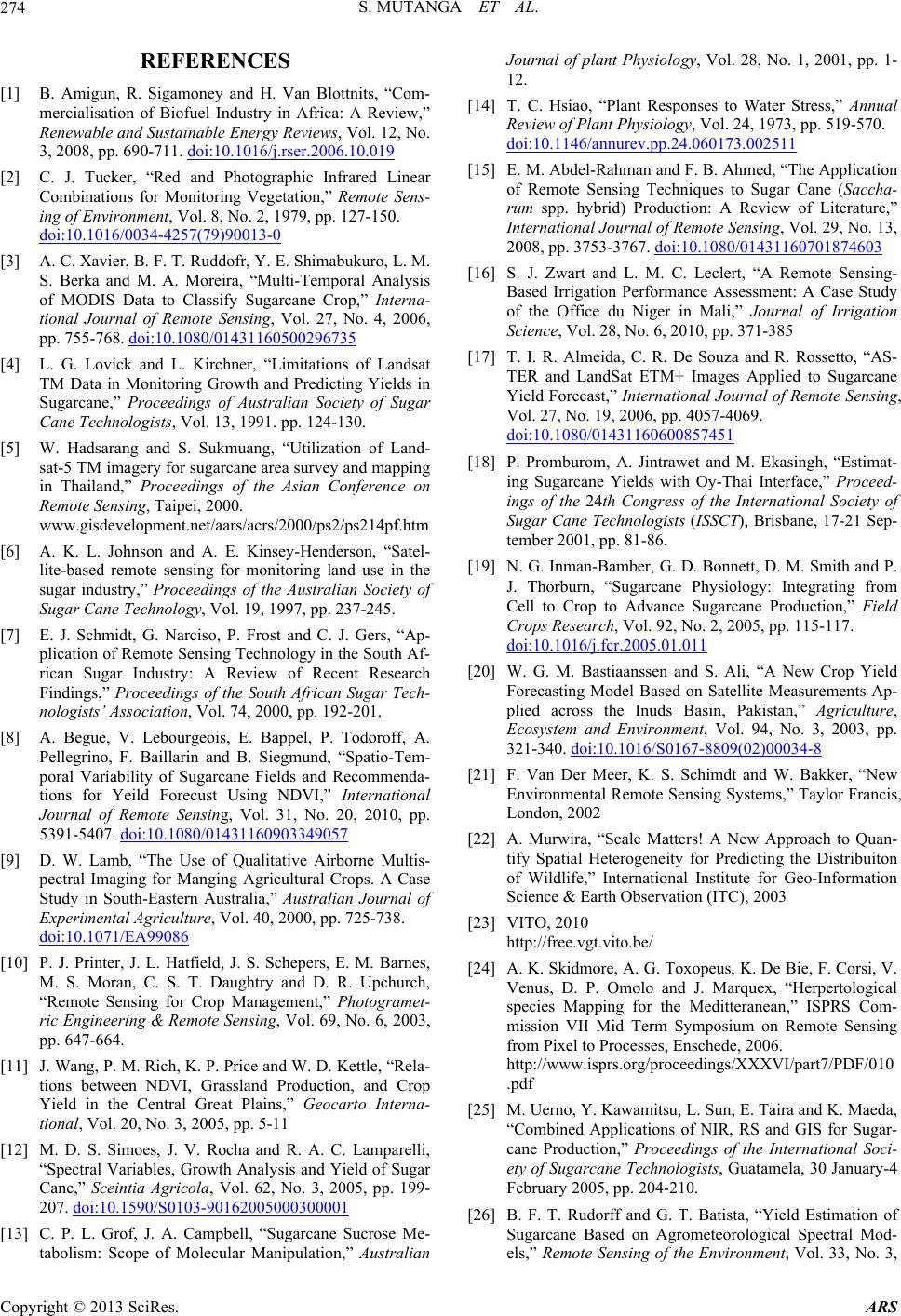
S. MUTANGA ET AL.
274
REFERENCES
[1] B. Amigun, R. Sigamoney and H. Van Blottnits, “Com-
mercialisation of Biofuel Industry in Africa: A Review,”
Renewable and Sustainable Energy Reviews, Vol. 12, No.
3, 2008, pp. 690-711. doi:10.1016/j.rser.2006.10.019
[2] C. J. Tucker, “Red and Photographic Infrared Linear
Combinations for Monitoring Vegetation,” Remote Sens-
ing of Environment, Vol. 8, No. 2, 1979, pp. 127-150.
doi:10.1016/0034-4257(79)90013-0
[3] A. C. Xavier, B. F. T. Ruddofr, Y. E. Shimabukuro, L. M.
S. Berka and M. A. Moreira, “Multi-Temporal Analysis
of MODIS Data to Classify Sugarcane Crop,” Interna-
tional Journal of Remote Sensing, Vol. 27, No. 4, 2006,
pp. 755-768. doi:10.1080/01431160500296735
[4] L. G. Lovick and L. Kirchner, “Limitations of Landsat
TM Data in Monitoring Growth and Predicting Yields in
Sugarcane,” Proceedings of Australian Society of Sugar
Cane Technologists, Vol. 13, 1991. pp. 124-130.
[5] W. Hadsarang and S. Sukmuang, “Utilization of Land-
sat-5 TM imagery for sugarcane area survey and mapping
in Thailand,” Proceedings of the Asian Conference on
Remote Sensing, Taipei, 2000.
www.gisdevelopment.net/aars/acrs/2000/ps2/ps214pf.htm
[6] A. K. L. Johnson and A. E. Kinsey-Henderson, “Satel-
lite-based remote sensing for monitoring land use in the
sugar industry,” Proceedings of the Australian Society of
Sugar Cane Technology, Vol. 19, 1997, pp. 237-245.
[7] E. J. Schmidt, G. Narciso, P. Frost and C. J. Gers, “Ap-
plication of Remote Sensing Technology in the South Af-
rican Sugar Industry: A Review of Recent Research
Findings,” Proceedings of the South African Sugar Tech-
nologists’ Association, Vol. 74, 2000, pp. 192-201.
[8] A. Begue, V. Lebourgeois, E. Bappel, P. Todoroff, A.
Pellegrino, F. Baillarin and B. Siegmund, “Spatio-Tem-
poral Variability of Sugarcane Fields and Recommenda-
tions for Yeild Forecust Using NDVI,” International
Journal of Remote Sensing, Vol. 31, No. 20, 2010, pp.
5391-5407. doi:10.1080/01431160903349057
[9] D. W. Lamb, “The Use of Qualitative Airborne Multis-
pectral Imaging for Manging Agricultural Crops. A Case
Study in South-Eastern Australia,” Australian Journal of
Experimental Agriculture, Vol. 40, 2000, pp. 725-738.
doi:10.1071/EA99086
[10] P. J. Printer, J. L. Hatfield, J. S. Schepers, E. M. Barnes,
M. S. Moran, C. S. T. Daughtry and D. R. Upchurch,
“Remote Sensing for Crop Management,” Photogramet-
ric Engineering & Remote Sensing, Vol. 69, No. 6, 2003,
pp. 647-664.
[11] J. Wang, P. M. Rich, K. P. Pric e and W. D. Ket tle, “Rela -
tions between NDVI, Grassland Production, and Crop
Yield in the Central Great Plains,” Geocarto Interna-
tional, Vol. 20, No. 3, 2005, pp. 5-11
[12] M. D. S. Simoes, J. V. Rocha and R. A. C. Lamparelli,
“Spectral Variables, Growth Analysis and Yield of Sugar
Cane,” Sceintia Agricola, Vol. 62, No. 3, 2005, pp. 199-
207. doi:10.1590/S0103-90162005000300001
[13] C. P. L. Grof, J. A. Campbell, “Sugarcane Sucrose Me-
tabolism: Scope of Molecular Manipulation,” Australian
Journal of plant Physiology, Vol. 28, No. 1, 2001, pp. 1-
12.
[14] T. C. Hsiao, “Plant Responses to Water Stress,” Annual
Review of Plant Physiology, Vol. 24, 1973, pp. 519-570.
doi:10.1146/annurev.pp.24.060173.002511
[15] E. M. Abdel-Rahman and F. B. Ahmed, “The Application
of Remote Sensing Techniques to Sugar Cane (Saccha-
rum spp. hybrid) Production: A Review of Literature,”
International Journal of Remote Sensing, Vol. 29, No. 13,
2008, pp. 3753-3767. doi:10.1080/01431160701874603
[16] S. J. Zwart and L. M. C. Leclert, “A Remote Sensing-
Based Irrigation Performance Assessment: A Case Study
of the Office du Niger in Mali,” Journal of Irrigation
Science, Vol. 28, No. 6, 2010, pp. 371-385
[17] T. I. R. Almeida, C. R. De Souza and R. Rossetto, “AS-
TER and LandSat ETM+ Images Applied to Sugarcane
Yield Forecast,” International Journal of Remote Sensing,
Vol. 27, No. 19, 2006, pp. 4057-4069.
doi:10.1080/01431160600857451
[18] P. Promburom, A. Jintrawet and M. Ekasingh, “Estimat-
ing Sugarcane Yields with Oy-Thai Interface,” Proceed-
ings of the 24th Congress of the International Society of
Sugar Cane Technologists (ISSCT), Brisbane, 17-21 Sep-
tember 2001, pp. 81-86.
[19] N. G. Inman-Bamber, G. D. Bonnett, D. M. Smith and P.
J. Thorburn, “Sugarcane Physiology: Integrating from
Cell to Crop to Advance Sugarcane Production,” Field
Crops Research, Vol. 92, No. 2, 2005, pp. 115-117.
doi:10.1016/j.fcr.2005.01.011
[20] W. G. M. Bastiaanssen and S. Ali, “A New Crop Yield
Forecasting Model Based on Satellite Measurements Ap-
plied across the Inuds Basin, Pakistan,” Agriculture,
Ecosystem and Environment, Vol. 94, No. 3, 2003, pp.
321-340. doi:10.1016/S0167-8809(02)00034-8
[21] F. Van Der Meer, K. S. Schimdt and W. Bakker, “New
Environmental Remote Sensing Systems,” Taylor Francis,
London, 2002
[22] A. Murwira, “Scale Matters! A New Approach to Quan-
tify Spatial Heterogeneity for Predicting the Distribuiton
of Wildlife,” International Institute for Geo-Information
Science & Earth Observation (ITC), 2003
[23] VITO, 2010
http://free.vgt.vito.be/
[24] A. K. Skidmore, A. G. Toxopeus, K. De Bie, F. Corsi, V.
Venus, D. P. Omolo and J. Marquex, “Herpertological
species Mapping for the Meditteranean,” ISPRS Com-
mission VII Mid Term Symposium on Remote Sensing
from Pixel to Processes, Enschede, 2006.
http://www.isprs.org/proceedings/XXXVI/part7/PDF/010
.pdf
[25] M. Uerno, Y. Kawamitsu, L. Sun, E. Taira and K. Maeda,
“Combined Applications of NIR, RS and GIS for Sugar-
cane Production,” Proceedings of the International Soci-
ety of Sugarcane Technologists, Guatamela, 30 January-4
February 2005, pp. 204-210.
[26] B. F. T. Rudorff and G. T. Batista, “Yield Estimation of
Sugarcane Based on Agrometeorological Spectral Mod-
els,” Remote Sensing of the Environment, Vol. 33, No. 3,
Copyright © 2013 SciRes. ARS