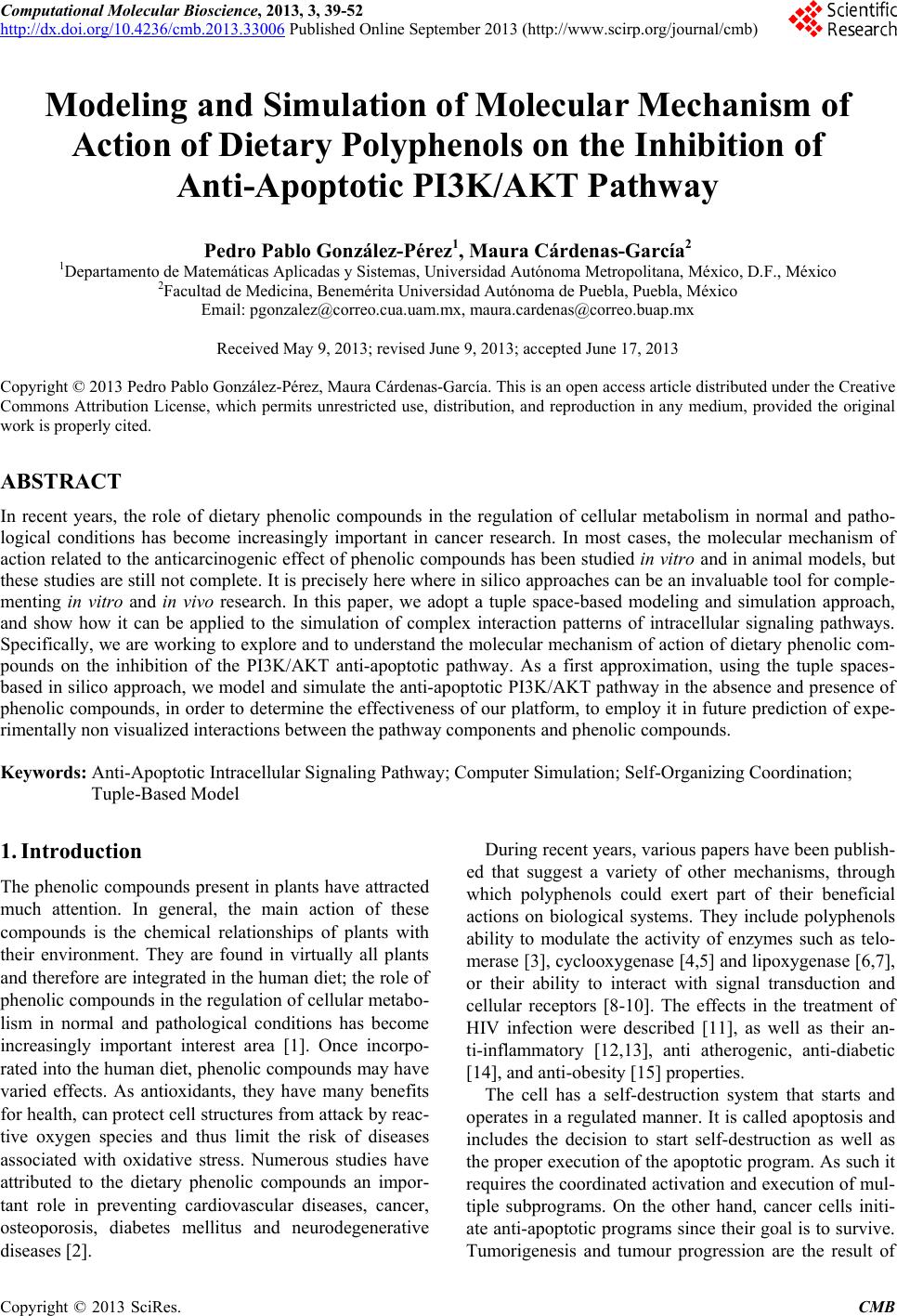 Computational Molecular Bioscience, 2013, 3, 39-52 http://dx.doi.org/10.4236/cmb.2013.33006 Published Online September 2013 (http://www.scirp.org/journal/cmb) Copyright © 2013 SciRes. CMB Modeling and Simulation of Molecular Mechanism of Action of Dietary Polyphenols on the Inhibition of Anti-Apoptotic PI3K/AKT Pathway Pedro Pablo González-Pérez1, Maura Cárdenas-García2 1Departamento de Matemáticas Aplicadas y Sistemas, Universidad Autónoma Metropolitana, México, D.F., México 2Facultad de Medicina, Benemérita Universidad Autónoma de Puebla, Puebla, México Email: pgonzalez@correo.cua.uam.mx, maura.cardenas@correo.buap.mx Received May 9, 2013; revised June 9, 2013; accepted June 17, 2013 Copyright © 2013 Pedro Pablo González-Pérez, Maura Cárdenas-García. This is an open access article distributed under the Creative Commons Attribution License, which permits unrestricted use, distribution, and reproduction in any medium, provided the original work is properly cited. ABSTRACT In recent years, the role of dietary phenolic compounds in the regulation of cellular metabolism in normal and patho- logical conditions has become increasingly important in cancer research. In most cases, the molecular mechanism of action related to the anticarcinogenic effect of phenolic compounds has been studied in vitro and in animal models , but these studies are still not co mplete. It is precisely here where in silico approaches can be an invaluable too l for co mple- menting in vitro and in vivo research. In this paper, we adopt a tuple space-based modeling and simulation approach, and show how it can be applied to the simulation of complex interaction patterns of intracellular signaling pathways. Specifically, we are working to explore and to understand the molecular mechanism of action of dietary phenolic com- pounds on the inhibition of the PI3K/AKT anti-apoptotic pathway. As a first approximation, using the tuple spaces- based in silico approach, we model and simulate the anti -apoptotic PI3K/AKT pathway in the absence and presence of phenolic compounds, in order to determine the effectiveness of our platform, to employ it in future prediction of expe- rimentally non visualized interactions between the pathway components and phe n olic compounds. Keywords: Anti-Apoptotic Intracellular Signaling Pathway; Computer Simulatio n; Self-Organizing Coordination; Tuple-Based Model 1. Introduction The phenolic compounds present in plants have attracted much attention. In general, the main action of these compounds is the chemical relationships of plants with their environment. They are found in virtually all plants and therefore are integrated in the human diet; the role of phenolic compounds in the regulation of cellular metabo- lism in normal and pathological conditions has become increasingly important interest area [1]. Once incorpo- rated into the human diet, phenolic compounds may have varied effects. As antioxidants, they have many benefits for health, can protect cell structures from attack by reac- tive oxygen species and thus limit the risk of diseases associated with oxidative stress. Numerous studies have attributed to the dietary phenolic compounds an impor- tant role in preventing cardiovascular diseases, cancer, osteoporosis, diabetes mellitus and neurodegenerative diseases [2]. During recent years, various papers have been publish- ed that suggest a variety of other mechanisms, through which polyphenols could exert part of their beneficial actions on biological systems. They include polyphenols ability to modulate the activity of enzymes such as telo- merase [3], cyclooxygenase [4,5] and lipoxygenase [6,7], or their ability to interact with signal transduction and cellular receptors [8-10]. The effects in the treatment of HIV infection were described [11], as well as their an- ti-inflammatory [12,13], anti atherogenic, anti-diabetic [14], and anti-obesity [15] properties. The cell has a self-destruction system that starts and operates in a regulated manner. It is called apoptosis and includes the decision to start self-destruction as well as the proper execution of the apoptotic program. As such it requires the coordinated activation and execution of mul- tiple subprograms. On the other hand, cancer cells initi- ate anti-apoptotic programs since th eir goal is to survive. Tumorigenesis and tumour progression are the result of
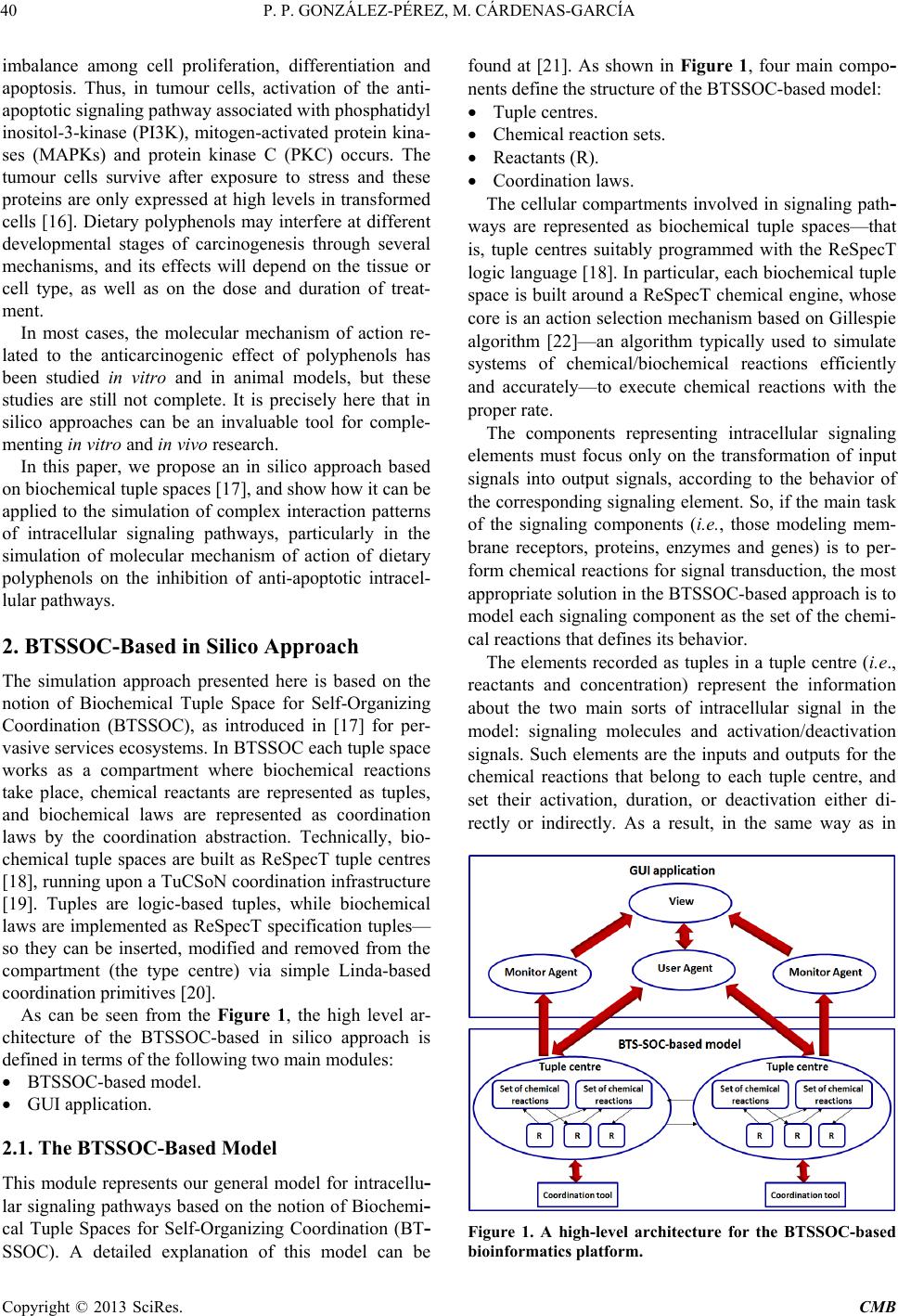 P. P. GONZÁLEZ-PÉREZ, M. CÁRDENAS-GARCÍA Copyright © 2013 SciRes. CMB imbalance among cell proliferation, differentiation and apoptosis. Thus, in tumour cells, activation of the anti- apoptotic signaling pathway associated with p hosphatidyl inositol-3-kinase (PI3K), mitogen-activated protein kina- ses (MAPKs) and protein kinase C (PKC) occurs. The tumour cells survive after exposure to stress and these proteins are only expressed at high levels in transformed cells [16]. Dietary polyphenols may interfere at different developmental stages of carcinogenesis through several mechanisms, and its effects will depend on the tissue or cell type, as well as on the dose and duration of treat- ment. In most cases, the molecular mechanism of action re- lated to the anticarcinogenic effect of polyphenols has been studied in vitro and in animal models, but these studies are still not complete. It is precisely here that in silico approaches can be an invaluable tool for comple- menting in vitro and in vivo research. In this paper, we propose an in silico approach based on biochemical tuple spaces [17], and show how it can be applied to the simulation of complex interaction patterns of intracellular signaling pathways, particularly in the si mul ation of molecular mechanism of action of dietary polyphenols on the inhibition of anti-apoptotic intracel- lular pathways. 2. BTSSOC-Based in Silico Approach The simulation approach presented here is based on the notion of Biochemical Tuple Space for Self-Organizing Coordination (BTSSOC), as introduced in [17] for per- vasive services ecosystems. In BTSSOC each tuple space works as a compartment where biochemical reactions take place, chemical reactants are represented as tuples, and biochemical laws are represented as coordination laws by the coordination abstraction. Technically, bio- chemical tuple spaces are built as ReSpecT tuple centres [18], running upon a TuCSoN c oordination infra structure [19]. Tuples are logic-based tuples, while biochemical laws are implemented as ReSpecT specification tuples— so they can be inserted, modified and removed from the compartment (the type centre) via simple Linda-based coordina tion primitives [20]. As can be seen from the Figure 1, the high level ar- chitecture of the BTSSOC-based in silico approach is defined in terms of the following two main modules: • BTSSOC-based model. • GUI application. 2.1. The BTSSOC-Based Model This module represents our general model for intracellu- lar signaling pathways based on the notion of Biochemi- cal Tuple Spaces for Self-Organizing Coordination (BT- SSOC). A detailed explanation of this model can be found at [21]. As shown in Figure 1, four main compo- nents define the structure of the BTSSOC-based model: • Tuple centres. • Chemical reaction sets. • Reactants (R). • Coordination laws. The cellular compartments involved in signaling path- ways are represented as biochemical tuple spaces—that is, tuple centres suitably programmed with the ReSpecT logic language [18]. In particular, each biochemical tuple space is built around a ReSpecT chemical engine, whose core is an action selection mechanism based on Gillespie algorithm [22]—an algorithm typically used to simulate systems of chemical/biochemical reactions efficiently and accurately—to execute chemical reactions with the proper rate. The components representing intracellular signaling elements must focus only on the transformation of input signals into output signals, according to the behavior of the corresponding signaling element. So, if the main ta sk of the signaling components (i.e., those modeling mem- brane receptors, proteins, enzymes and genes) is to per- form chemical reactions for signal transduction, the most appropriate solution in the BTSSOC-bas ed approach is to model each signaling component as the set of the chemi- cal reactions that defines its behavior. The elements recorded as tuples in a tuple centre (i.e., reactants and concentration) represent the information about the two main sorts of intracellular signal in the model: signaling molecules and activation/deactivation signals. Such elements are the inputs and outputs for the chemical reactions that belong to each tuple centre, and set their activation, duration, or deactivation either di- rectly or indirectly. As a result, in the same way as in Figure 1. A high-level architecture for the BTSSOC-based bioinformatics platform.
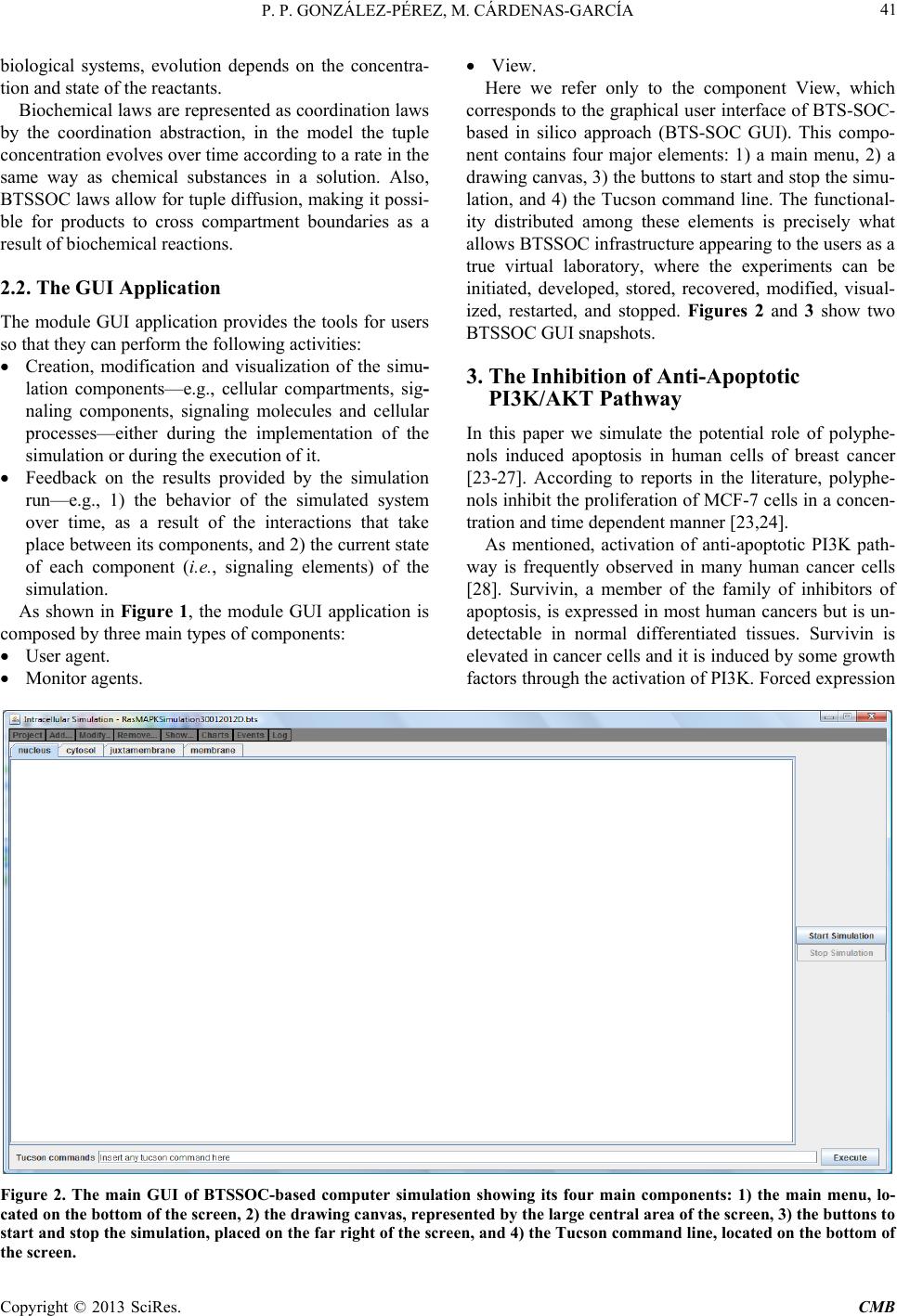 P. P. GONZÁLEZ-PÉREZ, M. CÁRDENAS-GARCÍA Copyright © 2013 SciRes. CMB 41 biological systems, evolution depends on the concentra- tion and state of the reactants. Biochemical laws are represented as coordination laws by the coordination abstraction, in the model the tuple concentration evolves over time according to a rate in the same way as chemical substances in a solution. Also, BTSSOC laws allow for tuple diffu sion, making it possi- ble for products to cross compartment boundaries as a result of bi ochemical reactions. 2.2. The GUI Application The module GUI application provides the tools for users so that they can perform the following activities: • Creation, modification and visualization of the simu- lation components—e.g., cellular compartments, sig- naling components, signaling molecules and cellular processes—either during the implementation of the simulation or during the execution of it. • Feedback on the results provided by the simulation run—e.g., 1) the behavior of the simulated system over time, as a result of the interactions that take place between its components, and 2) the current state of each component (i.e., signaling elements) of the simulation. As shown in Figure 1, the module GUI application is composed by three main types of components: • User agent. • Monitor agents. • View. Here we refer only to the component View, which corresponds to the graphical user interface of BTS-SOC- based in silico approach (BTS-SOC GUI). This compo- nent contains four major elements: 1) a main menu, 2) a drawing canvas, 3) the buttons to start and stop the simu- lation, and 4) the Tucson command line. The functional- ity distributed among these elements is precisely what allows BTSSOC infrastructure appearing to the users as a true virtual laboratory, where the experiments can be initiated, developed, stored, recovered, modified, visual- ized, restarted, and stopped. Figures 2 and 3 show two BTSSOC GUI snapshots. 3. The Inhibition of Anti-Apoptotic PI3K/AKT Path way In this paper we simulate the potential role of polyphe- nols induced apoptosis in human cells of breast cancer [23-27]. According to reports in the literature, polyphe- nols inhibit the prolif eration of MCF-7 cells in a concen- tration and time dependent manner [23,24]. As mentioned, activation of anti-apoptotic PI3K path- way is frequently observed in many human cancer cells [28]. Survivin, a member of the family of inhibitors of apoptosis, is expressed in most human cancers but is un- detectable in normal differentiated tissues. Survivin is elevated in cancer cells and it is induced by some growth factors through the activation of PI3K. Forced expression Figure 2. The main GUI of BTSSOC-based computer simulation showing its four main components: 1) the main menu, lo- cated on the bottom of the scr een, 2) the drawing canvas, represented by the large central area of the screen, 3) the buttons to start and stop the simulation, placed on the far right of the screen, and 4) the Tucson command line, located on the bottom of the screen.
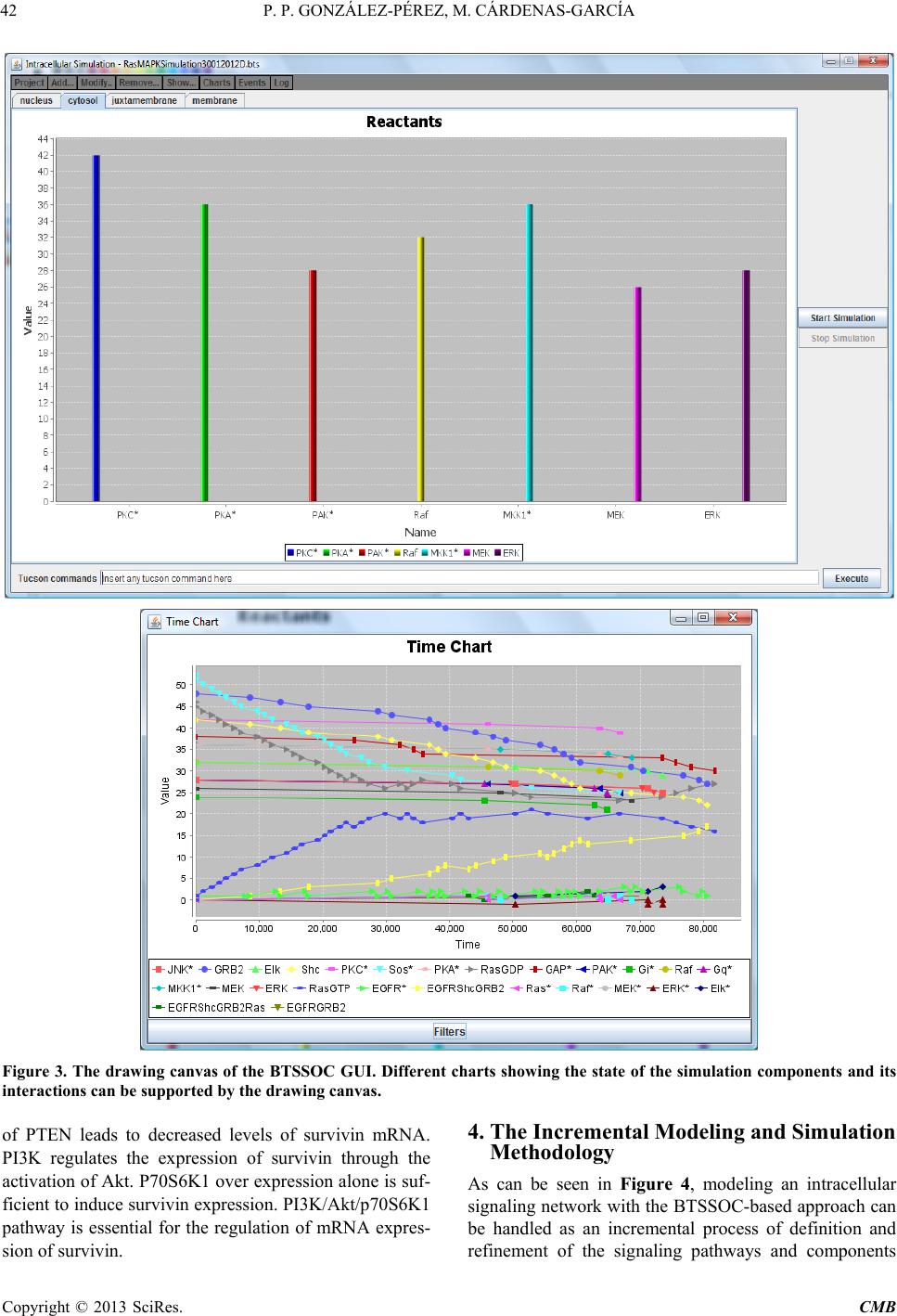 P. P. GONZÁLEZ-PÉREZ, M. CÁRDENAS-GARCÍA Copyright © 2013 SciRes. CMB Figure 3. The drawing canvas of the BTSSOC GUI. Different charts showing the state of the simulation components and its interac ti on s can be supported by the dr aw ing canvas. of PTEN leads to decreased levels of survivin mRNA. PI3K regulates the expression of survivin through the activation of Akt. P70S6K1 over expression alone is suf- ficient to induce survivin expression. PI3K/Akt/p70S6K1 pathway is essential for the regulation of mRNA expres- sion of survivin. 4. The Incremental Modeling and Simulation Methodology As can be seen in Figure 4, modeling an intracellular signaling network with the BTSSOC-based a pproach can be handled as an incremental process of definition and refinement of the signaling pathways and components
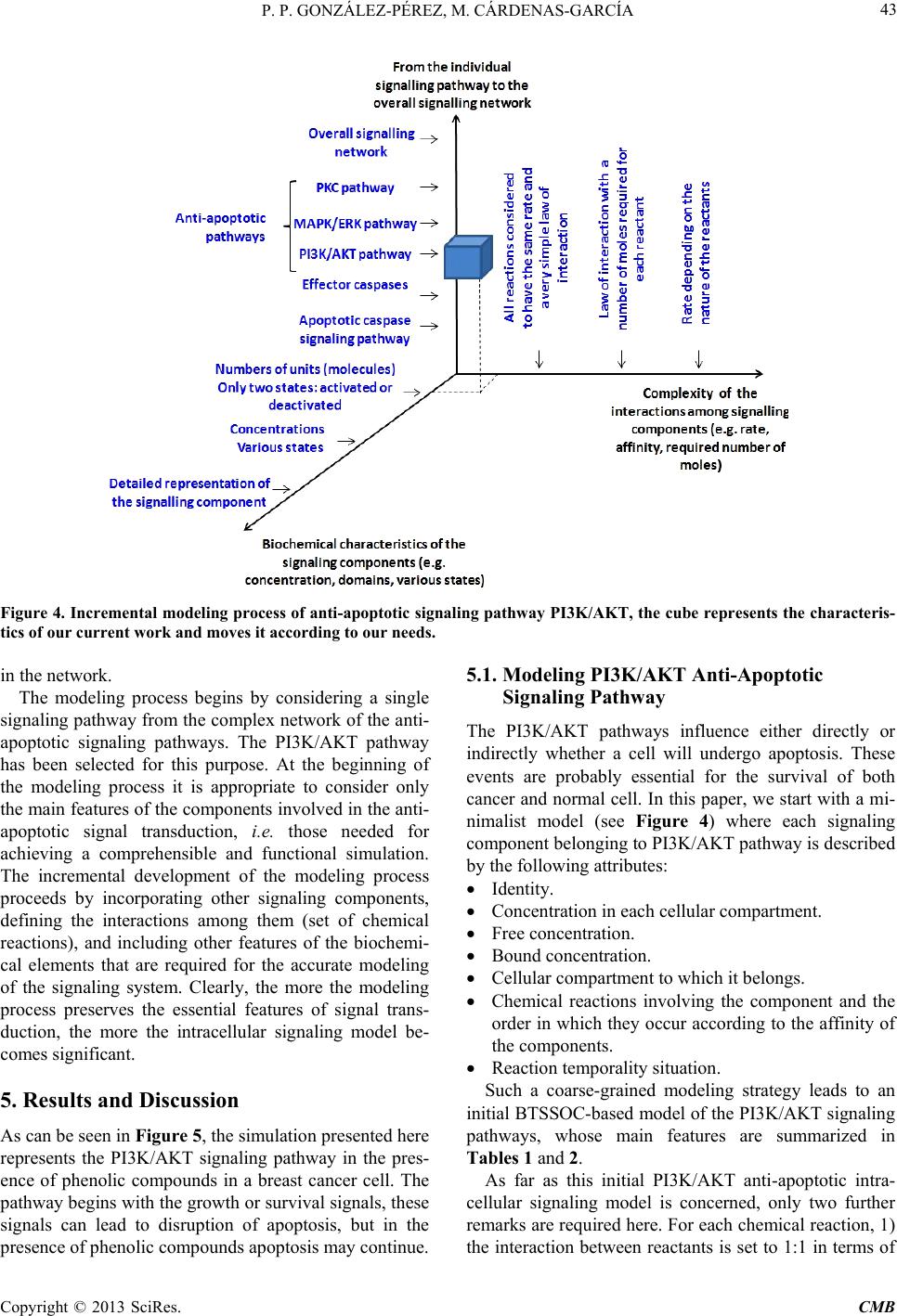 P. P. GONZÁLEZ-PÉREZ, M. CÁRDENAS-GARCÍA Copyright © 2013 SciRes. CMB 43 Figure 4. Incremental modeling process of anti-apoptotic signaling pathway PI3K/AKT, the cube represents the characteris- tics of our current work and moves it according to our needs. in the network. The modeling process begins by considering a single signaling pathway from the complex network of the anti- apoptotic signaling pathways. The PI3K/AKT pathway has been selected for this purpose. At the beginning of the modeling process it is appropriate to consider only the main features of the components involved in the anti- apoptotic signal transduction, i.e. those needed for achieving a comprehensible and functional simulation. The incremental development of the modeling process proceeds by incorporating other signaling components, defining the interactions among them (set of chemical reactions), and including other features of the biochemi- cal elements that are required for the accurate modeling of the signaling system. Clearly, the more the modeling process preserves the essential features of signal trans- duction, the more the intracellular signaling model be- comes significant. 5. Results and Discussion As can be seen in Figure 5, the simulation presented here represents the PI3K/AKT signaling pathway in the pres- ence of phenolic compounds in a breast cancer cell. The pathway begins with the grow th or survival signals, these signals can lead to disruption of apoptosis, but in the presence o f ph e nolic compounds apoptosis may continue. 5.1. Modeling PI3K/AKT Anti-Apoptotic Signaling Pathway The PI3K/AKT pathways influence either directly or indirectly whether a cell will undergo apoptosis. These events are probably essential for the survival of both cancer and normal cell. In this paper, we start with a mi- nimalist model (see Figure 4) where each signaling component belonging to PI3K/AKT pathway is described by the following attributes: • Identity. • Concentration in each cellular compartment. • Free concentration. • Bound concentration. • Cellular compartment to which it belongs. • Chemical reactions involving the component and the order in which they occur according to the affinity of the components. • Reaction temporality situation. Such a coarse-grained modeling strategy leads to an initial BTSSOC-based model of the PI3K/AKT signaling pathways, whose main features are summarized in Tables 1 and 2. As far as this initial PI3K/AKT anti-apoptotic intra- cellular signaling model is concerned, only two further remarks are required here. For each chemical reaction, 1) the interaction between reactants is set to 1:1 in terms of
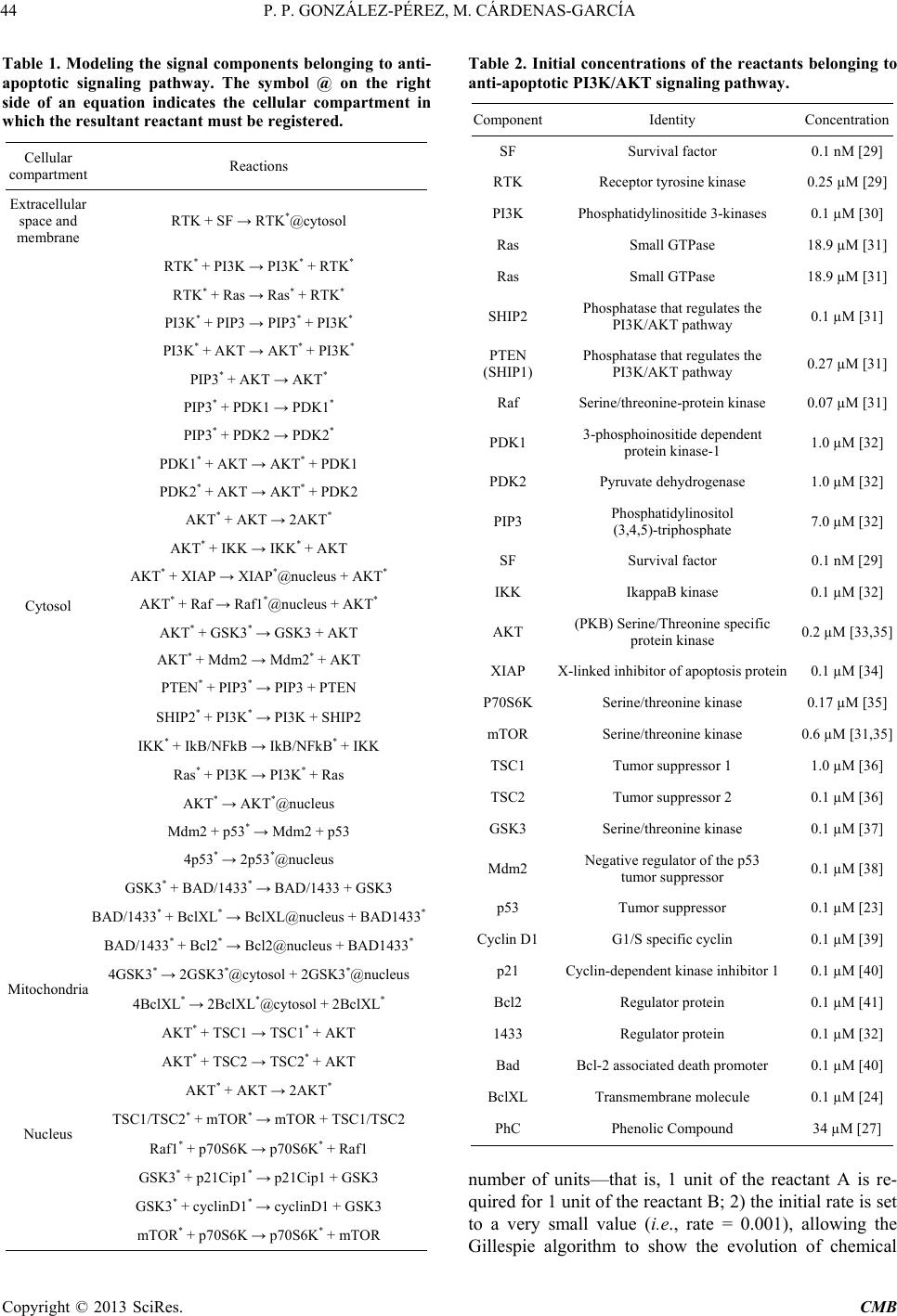 P. P. GONZÁLEZ-PÉREZ, M. CÁRDENAS-GARCÍA Copyright © 2013 SciRes. CMB Table 1. Modeling the signal components belonging to anti- apoptotic signaling pathway. The symbol @ on the right side of an equation indicates the cellular compartment in which the resultant reactant must be registered. Cellular compartment Reactions Extracellular space and membrane RTK + SF → RTK*@cytosol Cytosol RTK* + PI3K → PI3K* + RTK* RTK* + Ras → Ras* + RTK * PI3K* + PIP3 → PIP3* + PI3K* PI3K* + AKT → AKT* + PI3K* PIP3* + AKT → AKT* PIP3* + PDK1 → PDK1* PIP3* + PDK2 → PDK2* PDK1* + AKT → AKT* + PDK1 PDK2* + AKT → AKT* + PDK2 AKT* + AKT → 2AKT* AKT* + IKK → IKK* + AKT AKT* + XIAP → XIAP*@nucleus + AKT* AKT* + Raf → Raf1*@nucleus + AKT* AKT* + GSK3* → GSK3 + AKT AKT* + Mdm2 → Mdm2* + AKT PTEN* + PIP3* → PIP3 + PTEN SHIP2* + PI3K* → PI3K + SHIP2 IKK* + IkB/NFkB → IkB/NFkB* + IKK Ras* + PI3K → PI3K* + Ras AKT* → AKT*@nucleus Mdm2 + p53* → Mdm2 + p53 4p53* → 2p53*@nucleus GSK3* + BAD/1433* → BAD/1433 + GSK3 BAD/1433* + BclXL* → BclXL@nucleus + BAD1433* BAD/1433* + Bcl2* → Bcl2@nucleus + BAD1433* Mitochondria 4GSK3* → 2GSK3*@cytoso l + 2GSK3*@nucleus 4BclXL* → 2BclXL*@cyt osol + 2BclXL* Nucleus AKT* + TSC1 → TSC1* + AKT AKT* + TSC2 → TSC2* + AKT AKT* + AKT → 2AKT* TSC1/TSC2* + mTOR* → mTOR + TSC1/TSC2 Raf1* + p70S6K → p70S6K* + Raf1 GSK3* + p21Cip1* → p21Cip1 + GSK3 GSK3* + cyclinD1* → cyclinD1 + GSK3 mTOR* + p70S6K → p70S6K* + mTOR Table 2. Initial concentrations of the reactants belonging to anti-apoptotic PI3K/AKT signaling pathway. Component Identity Concentration SF Survival factor 0.1 nM [29] RTK Receptor tyrosine kinase 0.25 µM [29] PI3K Phosphatidylinositide 3-kinases 0.1 µM [30] Ras Small GTPase 18.9 µM [31] Ras Small GTPase 18.9 µM [31] SHIP2 Phosphatase that regulates the PI3K/AKT pathway 0.1 µM [31] PTEN (SHIP1) Phosphatase that regulates the PI3K/AKT pathway 0.27 µM [31] Raf Serine/threonine-protein kinase 0.07 µM [31] PDK1 3-phosphoinositide dependent protein kinase-1 1.0 µM [32] PDK2 Pyruvate dehydrogenase 1.0 µM [32] PIP3 Phosphatidylinositol (3,4,5)-triphosphate 7.0 µM [32] SF Survival factor 0.1 nM [29] IKK IkappaB kinase 0.1 µM [32] AKT (PKB) Serine/Threonine specific protein kinase 0.2 µM [33,35] XIAP X-linked in hibitor of apoptosis protein 0.1 µM [34] P70S6K Serine/threonine kinase 0.17 µM [35] mTOR Serine/threonine kinase 0.6 µM [31,35] TSC1 Tumor suppressor 1 1.0 µM [36] TSC2 Tumor suppressor 2 0.1 µM [36] GSK3 Serine/threonine kinase 0.1 µM [37] Mdm2 Negative regulator of the p53 tumor suppressor 0.1 µM [38] p53 Tumor suppressor 0.1 µM [23] Cyclin D1 G1/S specific cyclin 0.1 µM [39] p21 Cyclin-dependent kinase inhibitor 1 0.1 µM [40] Bcl2 Regulator protein 0.1 µM [41] 1433 Regulator protein 0.1 µM [32] Bad Bcl-2 associated death promoter 0.1 µM [40] BclXL Transmembran e molecule 0.1 µM [24] PhC Phenolic Compound 34 µ M [27] number of units—that is, 1 unit of the reactant A is re- quired for 1 unit of the reactant B; 2) the initial rate is set to a very small value (i.e., rate = 0.001), allowing the Gillespie algorithm to show the evolution of chemical
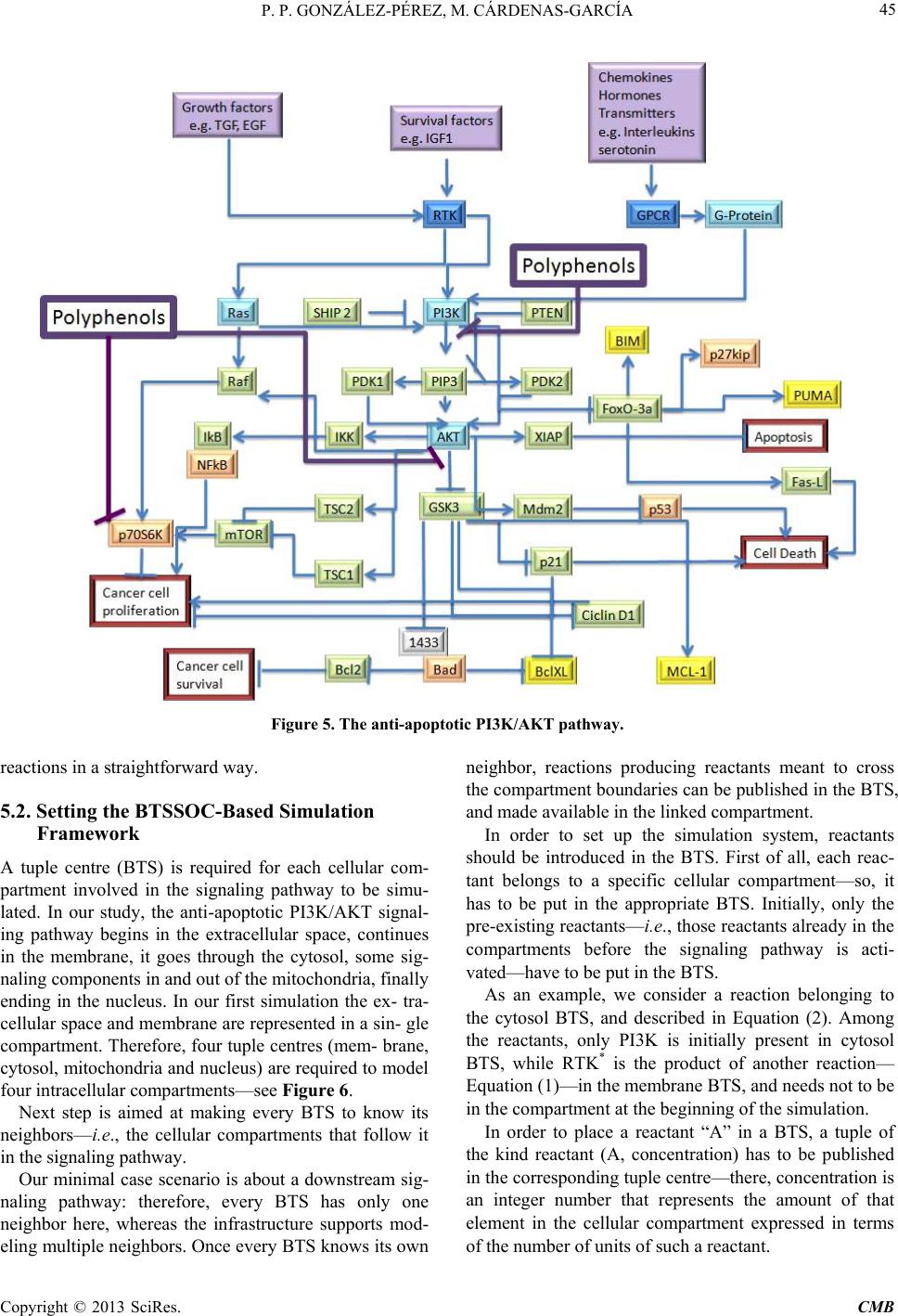 P. P. GONZÁLEZ-PÉREZ, M. CÁRDENAS-GARCÍA Copyright © 2013 SciRes. CMB 45 Figure 5. The anti-apoptotic PI3K/AKT pathway. reactions in a straightforward way. 5.2. Setting the BTSSOC-Based Simulation Framework A tuple centre (BTS) is required for each cellular com- partment involved in the signaling pathway to be simu- lated. In our study, the anti-apoptotic PI3K/AKT signal- ing pathway begins in the extracellular space, continues in the membrane, it goes through the cytosol, some sig- naling components in and out of the mitochondria, finally ending in the nucleus. In our first simulation the ex- tra- cellular space and membrane are represented in a sin- gle compartment. Therefore, four tuple centres (mem- brane, cytosol, mitochondria and nucleus) are required to model four intracellular compartment s—see F igure 6. Next step is aimed at making every BTS to know its neighbors—i. e., the cellular compartments that follow it in the signaling pathway. Our minimal case scenario is about a downstream sig- naling pathway: therefore, every BTS has only one neighbor here, whereas the infrastructure supports mod- eling multiple neighbors. Once every BTS knows its own neighbor, reactions producing reactants meant to cross the compartment boundaries can be published in the BTS, and made available in the linked compartment. In order to set up the simulation system, reactants should be introduced in the BTS. First of all, each reac- tant belongs to a specific cellular compartment—so, it has to be put in the appropriate BTS. Initially, only the pre-existing reactants—i.e., those reactants already in the compartments before the signaling pathway is acti- vated—have to be put in the BTS. As an example, we consider a reaction belonging to the cytosol BTS, and described in Equation (2). Among the reactants, only PI3K is initially present in cytosol BTS, while RTK* is the product of another reaction— Equation (1)—in the membrane BTS, and needs not to be in the compartment at the beginning of the simulation. In order to place a reactant “A” in a BTS, a tuple of the kind reactant (A, concentration) has to be published in the corresponding tuple centre—there, concentration is an integer number that represents the amount of that element in the cellular compartment expressed in terms of the number of units of such a reactant.
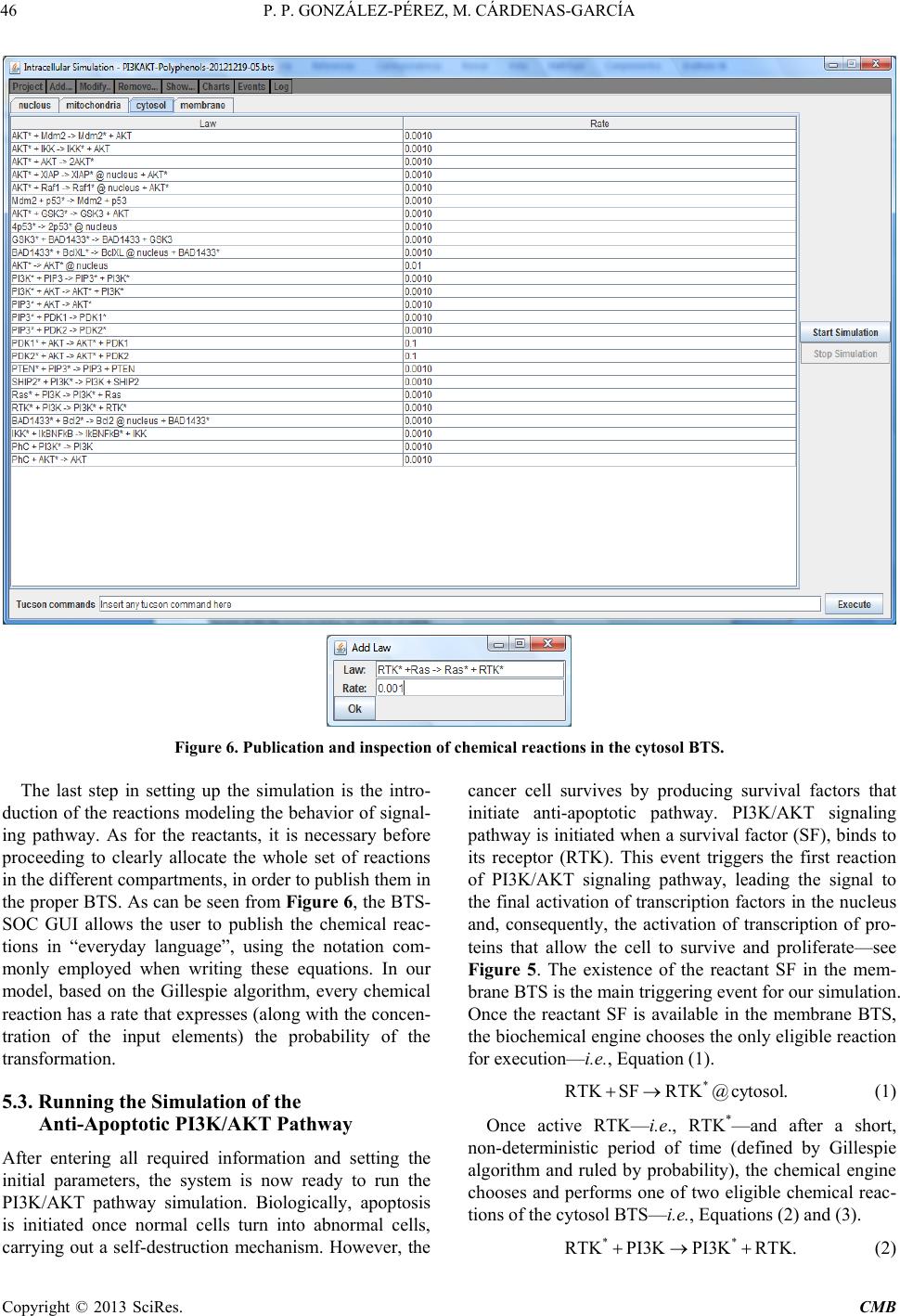 P. P. GONZÁLEZ-PÉREZ, M. CÁRDENAS-GARCÍA Copyright © 2013 SciRes. CMB Figure 6. Publication and inspection of chemical reactions in the cytosol BTS. The last step in setting up the simulation is the intro- duction of the reactions modeling the behavior of signal- ing pathway. As for the reactants, it is necessary before proceeding to clearly allocate the whole set of reactions in the different compartments, in order to publish them in the proper BTS. As can be seen from Figure 6, the BTS- SOC GUI allows the user to publish the chemical reac- tions in “everyday language”, using the notation com- monly employed when writing these equations. In our model, based on the Gillespie algorithm, every chemical reaction has a rate that expresses (along with the concen- tration of the input elements) the probability of the transformation. 5.3. Running the Simulation of the Anti-Apoptotic PI3K/AKT Pathway After entering all required information and setting the initial parameters, the system is now ready to run the PI3K/AKT pathway simulation. Biologically, apoptosis is initiated once normal cells turn into abnormal cells, carrying out a self-destruction mechanism. However, the cancer cell survives by producing survival factors that initiate anti-apoptotic pathway. PI3K/AKT signaling pathway is initiated when a survival factor (SF), binds to its receptor (RTK). This event triggers the first reaction of PI3K/AKT signaling pathway, leading the signal to the final activation of transcription factors in the nucleus and, consequently, the activation of transcription of pro- teins that allow the cell to survive and proliferate—see Figure 5. The existence of the reactant SF in the mem- brane BTS is the main triggering event for our simulation. Once the reactant SF is available in the membrane BTS, the biochemical engine chooses the only eligible reaction for execution—i.e., Equation (1). (1) Once active RTK—i.e., RTK*—and after a short, non-deterministic period of time (defined by Gillespie algorithm and ruled by probability), the chemical engine chooses and performs one of two eligible chemical reac- tions of the cytosol BTS—i.e., Equations (2) and (3). RTKPI3KPI3K RTK.+→+ (2)
 P. P. GONZÁLEZ-PÉREZ, M. CÁRDENAS-GARCÍA Copyright © 2013 SciRes. CMB 47 RTKRasRasRTK .+→ + (3) Which one among the two reactions is picked for exe- cution by the biochemical engine—given both the iden- tical initial rates and the interaction 1:1 between reactants —depends on the free concentration for each reagent involved in the reaction. The production of PI3K* and, consequently, the in- cremental availability of th is one in the cytoso l BTS lead PIP3 a ctivation and thus the amplification of the original signal, as shown in Equation (4) to Equation (10). * ** PI3KPIP3PIP3PI3K .+→ + (4 ) * ** PI3KAKTAKTPI3K .+→ + (5) PIP3AKTAKT .+→ (6) (7) PIP3PDK2PDK2.+→ (8) (9 ) (10) Figure 7 shows the phase of the simulation where the activation of AKT (AKT*) have taken place. AKT acti- vation allows the sequential activation of a series of re- agents and hence cell survival and proliferation. AKT target proteins can be classified into three distinct groups: anti-apoptotic proteins, anti-p53 and proteins promoting cell proliferation. In the PI3K/AKT simulation the phos- phorylation of these target proteins is represented in Eq- uation (11) to Equation (17). AKT IKKIKK AKT.+→ + (11) AKTXIAPXIAP@nucleusAKT .+→+ (12) AKTRaf1Raf1@nucleusAKT .+→+ (13) ** AKTGSK3GSK3AKT.+ →+ (14) AKTMdm2Mdm2 AKT. (15) AKTTSC1TSC1 AKT.+→ + (16) (17) Figures 8 and 9 show the variation in levels of reac- tants and products during signal transduction to achieve the activation of many downstream effector proteins— e.g., IKK, XIAP, Raf1 and Mdm2. Thus, the signal transduction goes ahead until reaching the activation of transcription factors in the nucleus BTS. As mentioned above, in each execution cycle the ac- tion selection mechanism—through the Gillespie algo- rithm—determines: 1) how long to wait before becomes active again, and 2) which reaction execute among all eligible chemical reactions. Figure 7. Execution of the reactions involved in the first segment of the simulation: from SF binding to AKT activation. At the bottom of the graph can be seen all the reactants implicated in these reactions. The symbol “*” refers to active/phos- pho- rylated state of the reactant.
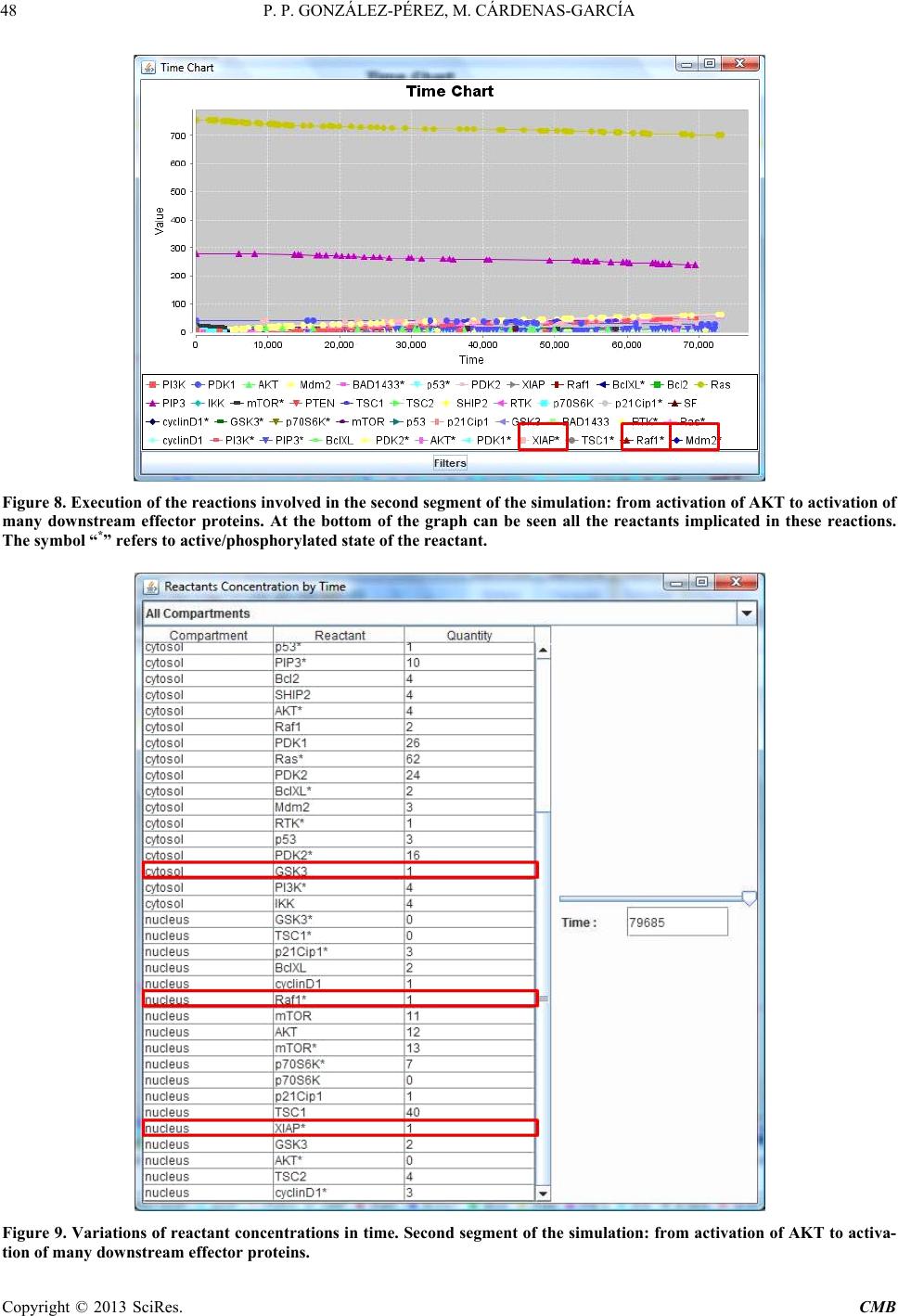 P. P. GONZÁLEZ-PÉREZ, M. CÁRDENAS-GARCÍA Copyright © 2013 SciRes. CMB Figure 8. Execution of the reactions involved in the second segment of the simulation: from activation of AKT to activation of many downstream effector proteins. At the bottom of the graph can be seen all the reactants implicated in these reactions. The symbol “*” refers to active/phosphorylated state of the reactant. Figure 9. Variations of reactant concentrations in time. Second segment of the simulation: from activation of AKT to activa- tion of many downstream effector proteins.
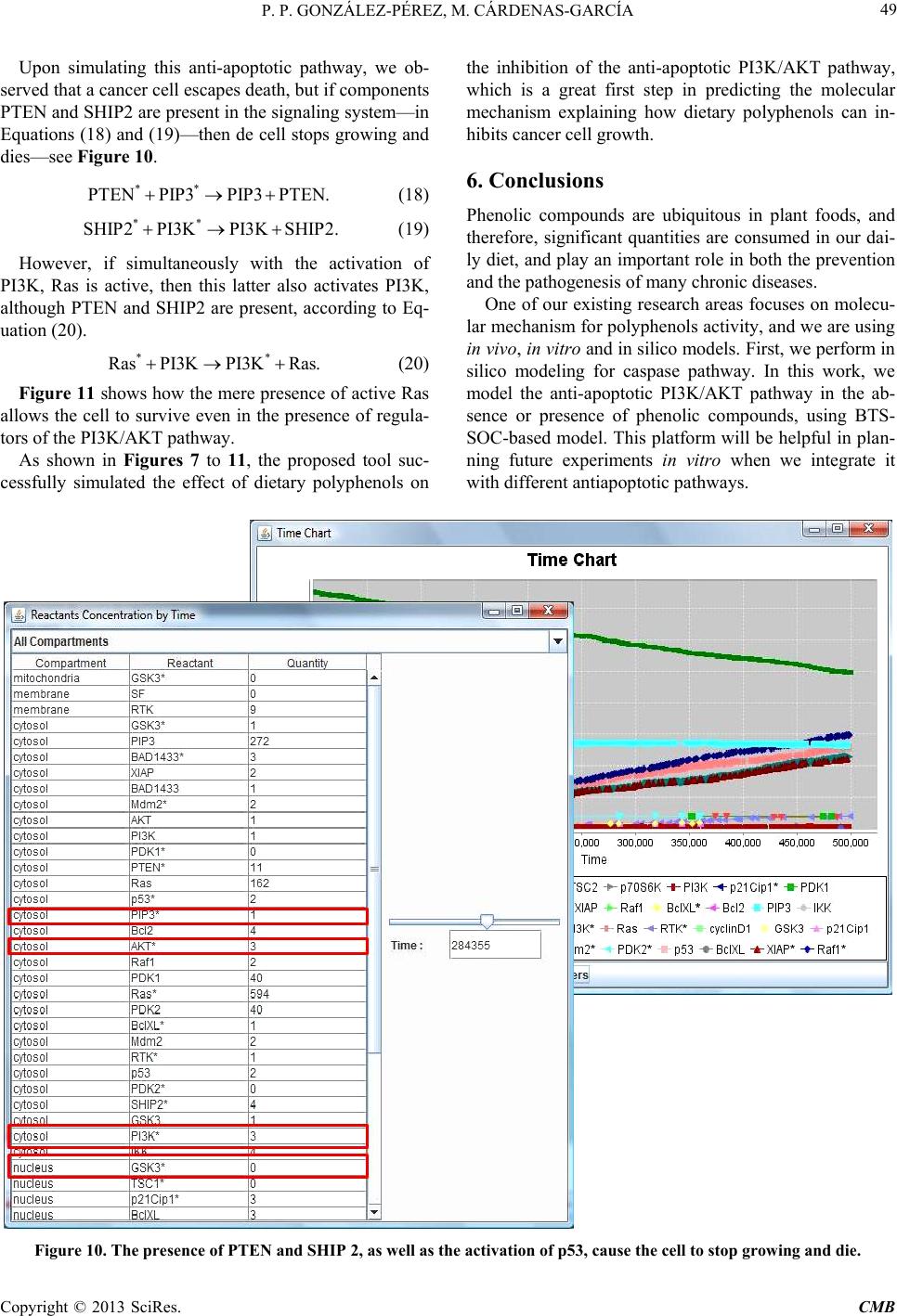 P. P. GONZÁLEZ-PÉREZ, M. CÁRDENAS-GARCÍA Copyright © 2013 SciRes. CMB 49 Upon simulating this anti-apoptotic pathway, we ob- served that a cancer cell escapes death, but if components PTEN and SHIP2 are present in the signaling system—in Equations (18 ) and (19)—then de cell stops growing and dies—see Figure 10. ** PTENPIP3PIP3 PTEN.+ →+ (18) ** SHIP2PI3KPI3K SHIP2.+ →+ (19) However, if simultaneously with the activation of PI3K, Ras is active, then this latter also activates PI3K, although PTEN and SHIP2 are present, according to Eq- uation (20). Ras PI3KPI3KRas.+→ + (2 0) Figure 11 shows how the mere presence of active Ras allows the cell to survive even in the presence of regula- tors of the PI3 K/ A KT pat hway. As shown in Figures 7 to 11, the proposed tool suc- cessfully simulated the effect of dietary polyphenols on the inhibition of the anti-apoptotic PI3K/AKT pathway, which is a great first step in predicting the molecular mechanism explaining how dietary polyphenols can in- hibits cancer cell growth. 6. Conclusions Phenolic compounds are ubiquitous in plant foods, and therefore, significant quantities are consumed in our dai- ly diet, and play an important role in both the prevention and the pathogenesis of many chronic diseases. One of our existing research areas focuses on molecu- lar mechanism for po lyphenols activity, and we are using in vivo, in vitro and in silico models. First, we perform in silico modeling for caspase pathway. In this work, we model the anti-apoptotic PI3K/AKT pathway in the ab- sence or presence of phenolic compounds, using BTS- SOC-based model. This platform will be helpful in plan- ning future experiments in vitro when we integrate it with different antiapoptotic pathways. Figure 10. The presence of PTEN and SHIP 2, as well as the activation of p53, cause the cell to stop growing and die.
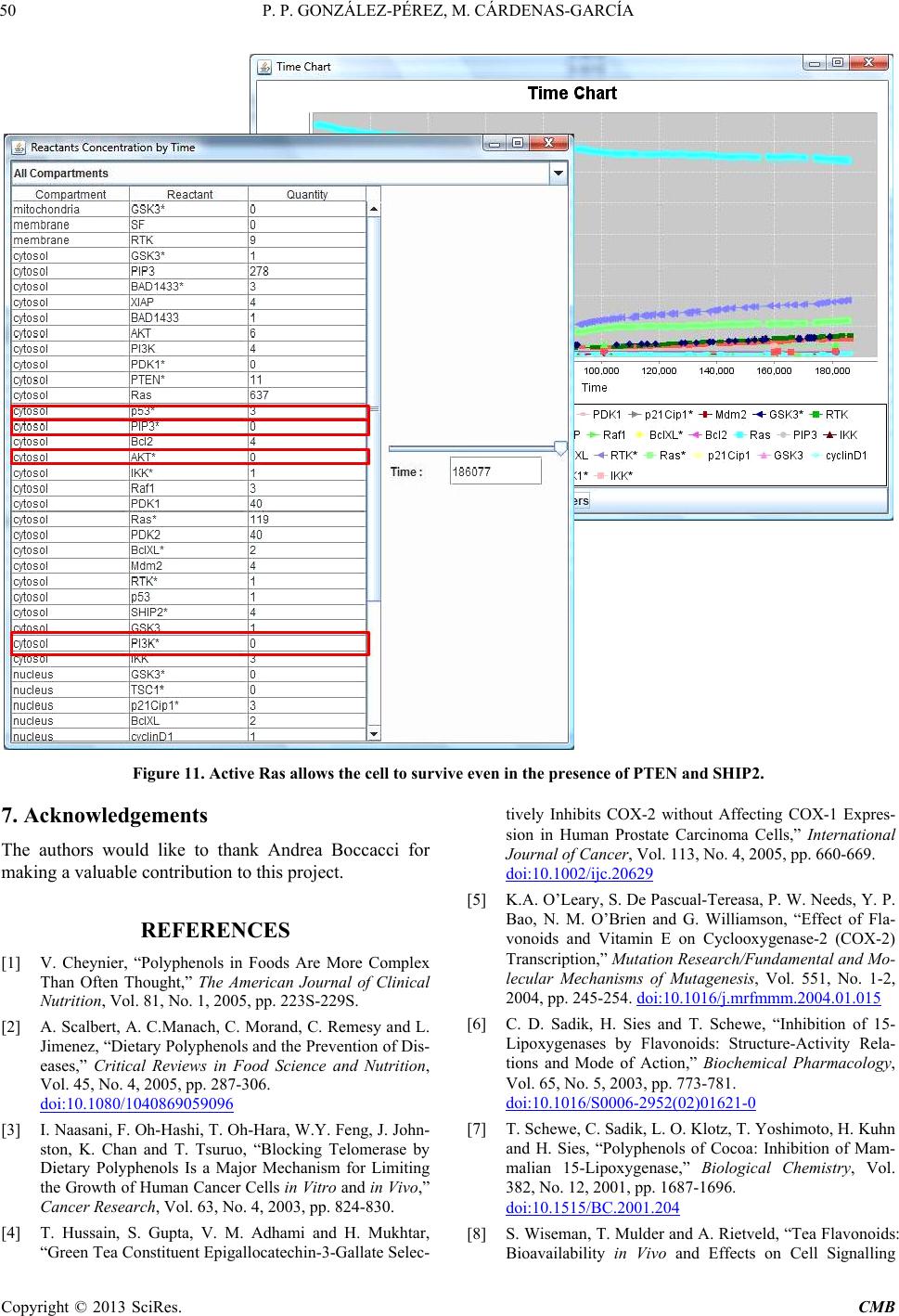 P. P. GONZÁLEZ-PÉREZ, M. CÁRDENAS-GARCÍA Copyright © 2013 SciRes. CMB Figure 11. Active Ras allows the cell to survive even in the presence of PTEN and SHIP2. 7. Acknowledgements The authors would like to thank Andrea Boccacci for making a valuable contribution to this project. REFERENCES [1] V. Cheynier, “Polyphenols in Foods Are More Complex Than Often Thought,” The American Journal of Clinical Nutrition, Vol. 81, No. 1, 2005, pp. 223S-229S. [2] A. Scalbert, A. C.Manach, C. Morand, C. Remesy and L. Jimenez, “Dietary Polyphenols and the Prevention of Di s- eases,” Critical Reviews in Food Science and Nutrition, Vol. 45, No. 4, 2005, pp. 287-306. doi:10.1080/1040869059096 [3] I. Naasani, F. Oh-Hashi, T. Oh-Hara, W.Y. Feng, J. John- ston, K. Chan and T. Tsuruo, “Blocking Telomerase by Dietary Polyphenols Is a Major Mechanism for Limiting the Growth of Human Cancer Cells in Vitro and in Vivo,” Cancer Research, Vol. 63, No. 4, 2003, pp. 824-830. [4] T. Hussain, S. Gupta, V. M. Adhami and H. Mukhtar, “Green Tea Constituent Epigallocatechin-3-Gallate Selec- tively Inhibits COX-2 without Affecting COX-1 Expres- sion in Human Prostate Carcinoma Cells,” International Journal of Cancer, Vol. 113, No. 4, 2005, pp. 660-669. doi:10.1002/ijc.20629 [5] K.A. O’Leary, S. De Pascual-Tereasa, P. W. Needs, Y. P. Bao, N. M. O’Brien and G. Williamson, “Effect of Fla- vonoids and Vitamin E on Cyclooxygenase-2 (COX-2) Transcription,” Mut ation Research/Fundamental and Mo- lecular Mechanisms of Mutagenesis, Vol. 551, No. 1-2, 2004, pp. 245-254. doi:10.1016/j.mrfmmm.2004.01.015 [6] C. D. Sadik, H. Sies and T. Schewe, “Inhibition of 15- Lipoxygenases by Flavonoids: Structure-Activity Rela- tions and Mode of Action ,” Biochemical Pharmacology, Vol. 65, No. 5, 2003, pp. 773-781. doi:10.1016/S0006-2952(02)01621-0 [7] T. Schewe, C. Sadi k, L. O. Klotz, T. Yoshimoto, H. Kuhn and H. Sies, “Polyphenols of Cocoa: Inhibition of Ma m- malian 15-Lipoxygenase,” Biological Chemistry, Vol. 382, No. 12, 2001, pp. 1687-1696. doi:10.1515/BC.2001.204 [8] S. Wiseman, T. Mulder and A. Rietveld, “Tea Flavonoids: Bioavailability in Vivo and Effects on Cell Signalling
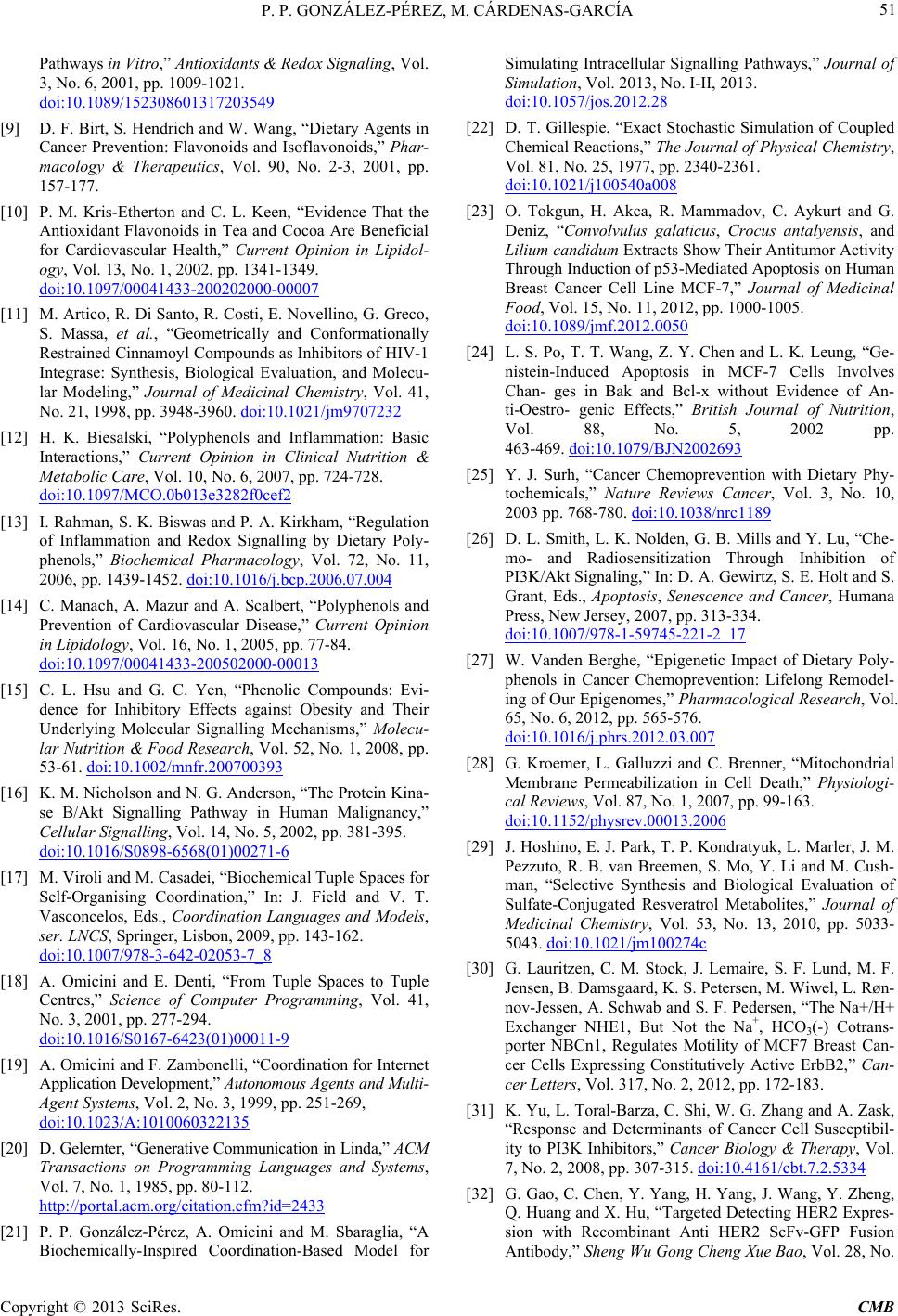 P. P. GONZÁLEZ-PÉREZ, M. CÁRDENAS-GARCÍA Copyright © 2013 SciRes. CMB 51 Pathways in Vitro,” Antioxidants & Redox Signaling, Vol. 3, No. 6, 2001, pp. 1009-1021. doi:10.1089/152308601317203549 [9] D. F. Birt, S. Hendrich and W. Wang, “Dietary Agents in Cancer Prevention: Flavonoids and Isoflavonoids,” Phar- macology & Therapeutics, Vol. 90, No. 2-3, 2001, pp. 157-177. [10] P. M. Kris-Etherton and C. L. Keen, “Evidence That the Antioxidant Flavonoids in Tea and Cocoa Are Beneficial for Cardiovascular Health,” Current Opinion in Lipidol- ogy, Vol. 13, No. 1, 2002, pp. 1341-1349. doi:10.1097/00041433-200202000-00007 [11] M. Artico, R. Di Sant o, R. Costi, E. Novellino, G. Greco, S. Massa, et al., “Geometrically and Conformationally Restrained Cinnamoyl Compounds as Inhibitors of HIV-1 Integrase: Synthesis, Biological Evaluation, and Molecu- lar Modeling,” Journal of Medicinal Chemistry, Vol. 41, No. 21, 1998, pp. 3948-3960. doi:10.1021/jm9707232 [12] H. K. Biesalski, “Polyphenols and Inflammation: Basic Interactions,” Current Opinion in Clinical Nutrition & Metabolic Care, Vol. 10, No. 6, 2007, pp. 724-728. doi:10.1097/MCO.0b013e3282f0cef2 [13] I. Rahman, S. K. Biswas and P. A. Kirkham, “Regulation of Inflammation and Redox Signalling by Dietary Poly- phenols,” Biochemical Pharmacology, Vol. 72, No. 11, 2006, pp. 1439-1452. doi:10.1016/j.bcp.2006.07.004 [14] C. Manach, A. Mazur and A. Scalbert, “Polyphenols and Prevention of Cardiovascular Disease,” Current Opinion in Lipidology, Vol . 16, No. 1, 2005, pp. 77-84. doi:10.1097/00041433-200502000-00013 [15] C. L. Hsu and G. C. Yen, “Phenolic Compounds: Evi- dence for Inhibitory Effects against Obesity and Their Underlying Molecular Signalling Mechanisms,” Molecu- lar Nutrition & Food Research, Vol. 52, No. 1, 2008, pp. 53-61. doi:10.1002/mnfr.200700393 [16] K. M. Nicholson and N. G. Anderson, “The Protein Kina- se B/Akt Signalling Pathway in Human Malignancy,” Cellular Signalling, Vol. 14, No. 5, 2002, pp. 381-395. doi:10.1016/S0898-6568(01)00271-6 [17] M. Viroli and M. Casadei, “Biochemical Tuple Spaces for Self-Organising Coordination,” In: J. Field and V. T. Vasconcelos, Eds., Coordination Languages and Models, ser. LNCS, Springer, Lisbon, 2009, pp. 143-162. doi:10.1007/978-3-642-02053-7_8 [18] A. Omicini and E. Denti, “From Tuple Spaces to Tuple Centres,” Science of Computer Programming, Vol. 41, No. 3, 2001, pp. 277-294. doi:10.1016/S0167-6423(01)00011-9 [19] A. Omicini and F. Zambonell i, “Coordination for Internet Application Deve lopment,” Autonomous Agents and Multi- Agent Systems, Vol. 2, No. 3, 1999, pp. 251-269, doi:10.1023/A:1010060322135 [20] D. Gelernter, “Generative Communication in Linda,” ACM Transactions on Programming Languages and Systems, Vol. 7, No. 1, 1985, pp. 80-112. http://portal.acm.org/citation.cfm?id=2433 [21] P. P. González-Pérez, A. Omicini and M. Sbaraglia, “A Biochemically-Inspired Coordination-Based Model for Simulating Intracellular Signalling Pathways,” Journal of Simulation, Vol. 2013, No. I-II, 2013. doi:10.1057/jos.2012.28 [22] D. T. Gillespie, “Exact Stochastic Simulation of Coupled Chemical Reactions,” The Journal of Physical Chemistry, Vol. 81, No. 25, 1977, pp. 2340-2361. doi:10.1021/j100540a008 [23] O. Tokgun, H. Akca, R. Mammadov, C. Aykurt and G. Deniz, “Convolvulus galaticus, Crocus antalyensis, and Lilium candidum Extracts Show Their Antitumor Activity Through Induction of p53-Mediated Apoptosis on Human Breast Cancer Cell Line MCF-7,” Journal of Medicinal Food, Vol. 15, No. 11, 2012, pp. 1000-1005. doi:10.1089/jmf.2012.0050 [24] L. S. Po, T. T. Wang, Z. Y. Chen and L. K. Leung, “Ge- nistein-Induced Apoptosis in MCF-7 Cells Involves Chan- ges in Bak and Bcl-x without Evidence of An- ti-Oestro- genic Effects,” British Journal of Nutrition, Vol. 88, No. 5, 2002 pp. 463-469. doi:10.1079/BJN2002693 [25] Y. J. Surh, “Cancer Chemoprevention with Dietary Phy- tochemicals,” Nature Reviews Cancer, Vol. 3, No. 10, 2003 pp. 768-780. doi:10.1038/nrc1189 [26] D. L. Smith, L. K. Nolden, G. B. Mills and Y. Lu, “Che- mo- and Radiosensitization Through Inhibition of PI3K/Akt Signaling,” In: D. A. Gewirtz, S. E. Holt and S. Grant, Eds., Apoptosis, Senescence and Cancer, Humana Press, New Jersey , 2007, pp. 313-334. doi:10.1007/978-1-59745-221-2_17 [27] W. Vanden Berghe, “Epigenetic Impact of Dietary Poly- phenols in Cancer Chemoprevention: Lifelong Remodel- ing of Our Epigenomes,” Pharmacological Research, Vol. 65, No. 6, 2012, pp. 565-576. doi:10.1016/j.phrs.2012.03.007 [28] G. Kroemer, L. Galluzzi and C. Brenner, “Mitochondrial Membrane Permeabilization in Cell Death,” Physiologi- cal Reviews, Vol. 87, No. 1, 2007, pp. 99-163. doi:10.1152/physrev.00013.2006 [29] J. Hoshino, E. J. Park, T. P. Kondratyuk, L. Marler, J. M. Pezzuto, R. B. van Breemen, S. Mo, Y. Li and M. Cush- man, “Selective Synthesis and Biological Evaluation of Sulfate-Conjugated Resveratrol Metabolites,” Journal of Medicinal Chemistry, Vol. 53, No. 13, 2010, pp. 5033- 5043. doi:10.1021/jm100274c [30] G. Lauritzen, C. M. Stock, J. Lemaire, S. F. Lund, M. F. Jensen, B. Damsgaard, K. S. Petersen, M. Wiwel, L. Røn- nov-Jessen, A. Schwab and S. F. Pedersen, “The Na+/H+ Exchanger NHE1, But Not the Na+, HCO3(-) Cotrans- porter NBCn1, Regulates Motility of MCF7 Breast Can- cer Cells Expressing Constitutively Active ErbB2,” Can- cer Letters, Vol. 317, No. 2, 2012, pp. 172-183. [31] K. Yu, L. Toral-Barza, C. Shi, W. G. Zhang and A. Zask, “Response and Determinants of Cancer Cell Susceptibil- ity to PI3K Inhibitors,” Cancer Biology & Therapy, Vol. 7, No. 2, 2008, pp. 307-315. doi:10.4161/cbt.7.2.5334 [32] G. Gao, C. Chen, Y. Yang, H. Yang, J. Wang, Y. Zheng, Q. Huang and X. Hu, “Targeted Detecting HER2 Expres- sion with Recombinant Anti HER2 ScFv-GFP Fusion Antibody,” She ng Wu Gong Cheng Xue Bao, Vol. 28 , N o.
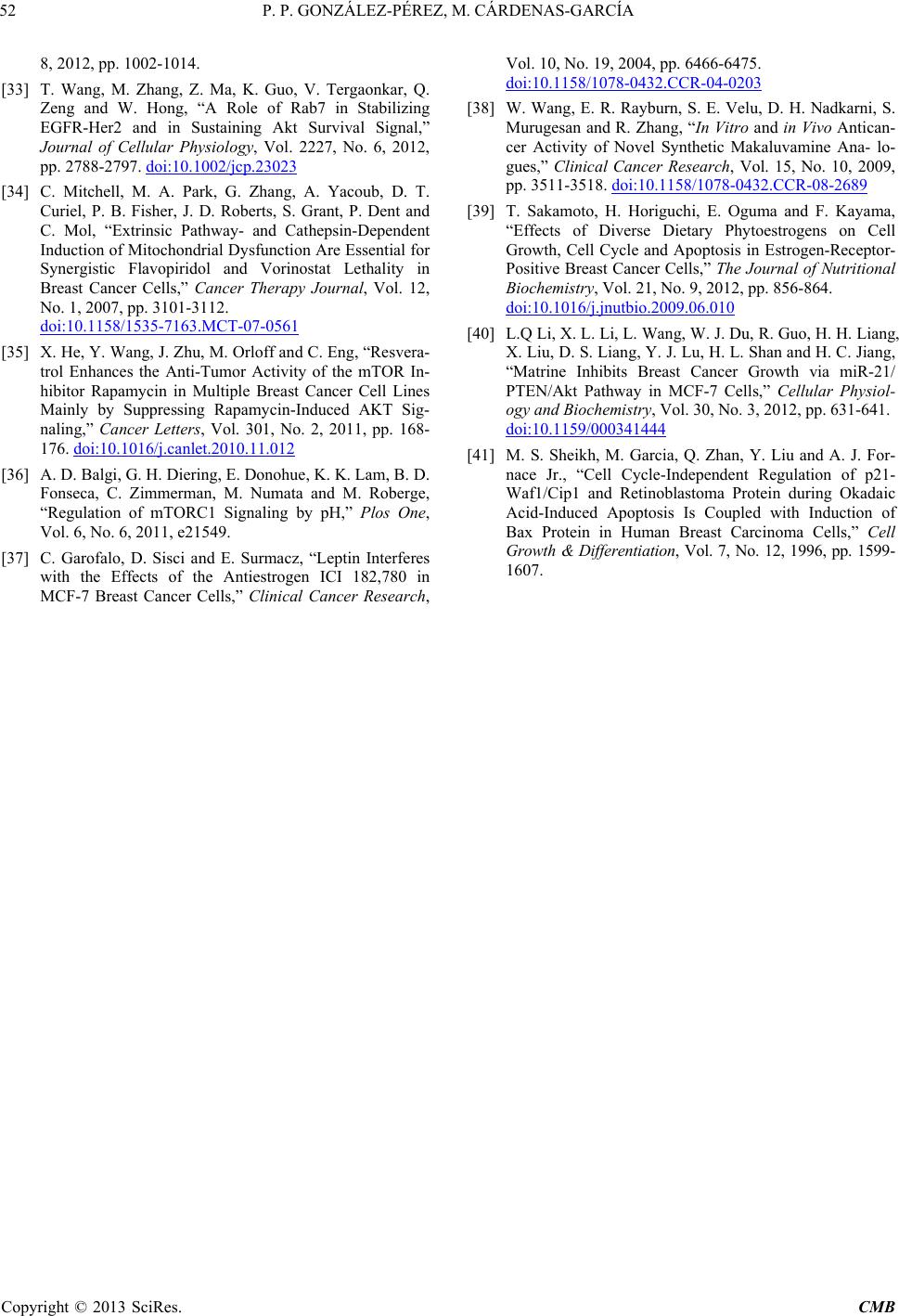 P. P. GONZÁLEZ-PÉREZ, M. CÁRDENAS-GARCÍA Copyright © 2013 SciRes. CMB 8, 2012, pp. 1002-1014. [33] T. Wang, M. Zhang, Z. Ma, K. Guo, V. Tergaonkar, Q. Zeng and W. Hong, “A Role of Rab7 in Stabilizing EGFR-Her2 and in Sustaining Akt Survival Signal,” Journal of Cellular Physiology, Vol. 2227, No. 6, 2012, pp. 2788-2797. doi:10.1002/jcp.23023 [34] C. Mitchell, M. A. Park, G. Zhang, A. Yacoub, D. T. Curiel, P. B. Fisher, J. D. Roberts, S. Grant, P. Dent and C. Mol, “Extrinsic Pathway- and Cathepsin-Dependent Induction of Mitochondrial Dysfunction Are Essential for Synergistic Flavopiridol and Vorinostat Lethality in Breast Cancer Cells,” Cancer Therapy Journal, Vol. 12, No. 1, 2007, pp. 3101-3112. doi:10.1158/1535-7163.MCT-07-0561 [35] X. He, Y. Wang, J. Zhu, M. Orloff and C. Eng, “Resvera- trol Enhances the Anti-Tumor Activity of the mTOR In- hibitor Rapamycin in Multiple Breast Cancer Cell Lines Mainly by Suppressing Rapamycin-Induced AKT Sig- naling,” Cancer Letters, Vol. 301, No. 2, 2011, pp. 168- 176. doi:10.1016/j.canlet.2010.11.012 [36] A. D. Balgi, G. H. Diering, E. Donohue, K. K. Lam, B. D. Fonseca, C. Zimmerman, M. Numata and M. Roberge, “Regulation of mTORC1 Signaling by pH,” Plos One, Vol. 6, No. 6, 2011, e21549. [37] C. Garofalo, D. Sisci and E. Surmacz, “Leptin Interferes with the Effects of the Antiestrogen ICI 182,780 in MCF-7 Breast Cancer Cells,” Clinical Cancer Research, Vol. 10, No. 19, 2004, pp. 6466-6475. doi:10.1158/1078-0432.CCR-04-0203 [38] W. Wang, E. R. Rayburn, S. E. Velu, D. H. Nadkarni, S. Murugesan and R. Zhang, “In Vitro and in Vivo Antican- cer Activity of Novel Synthetic Makaluvamine Ana- lo- gues,” Clinical Cancer Research, Vol. 15, No. 10, 2009, pp. 3511-3518. doi:10.1158/1078-0432.CCR-08-2689 [39] T. Sakamoto, H. Horiguchi, E. Oguma and F. Kayama, “Effects of Diverse Dietary Phytoestrogens on Cell Growth, Cell Cycle and Apoptosis in Estrogen-Receptor- Positive Breast Cancer Cells,” The Journal of Nutritional Biochemistry, V ol. 21, No. 9, 2012, pp. 856-864. doi:10.1016/j.jnutbio.2009.06.010 [40] L.Q Li, X. L. Li, L. Wang, W. J. Du, R. Guo, H. H. Liang, X. Liu, D. S. Liang, Y. J. Lu, H. L. Shan and H. C. Jiang, “Matrine Inhibits Breast Cancer Growth via miR-21/ PTEN/Akt Pathway in MCF-7 Cells,” Cellular Physiol- ogy and Biochemistry, Vol. 30, No. 3, 2012, pp. 631-641. doi:10.1159/000341444 [41] M. S. Sheikh, M. Garcia, Q. Zhan, Y. Liu and A. J. For- nace Jr., “Cell Cycle-Independent Regulation of p21- Waf1/Cip1 and Retinoblastoma Protein during Okadaic Acid-Induced Apoptosis Is Coupled with Induction of Bax Protein in Human Breast Carcinoma Cells,” Cell Growth & Differentiation, Vol. 7, No. 12, 1996, pp. 1599- 1607.
|