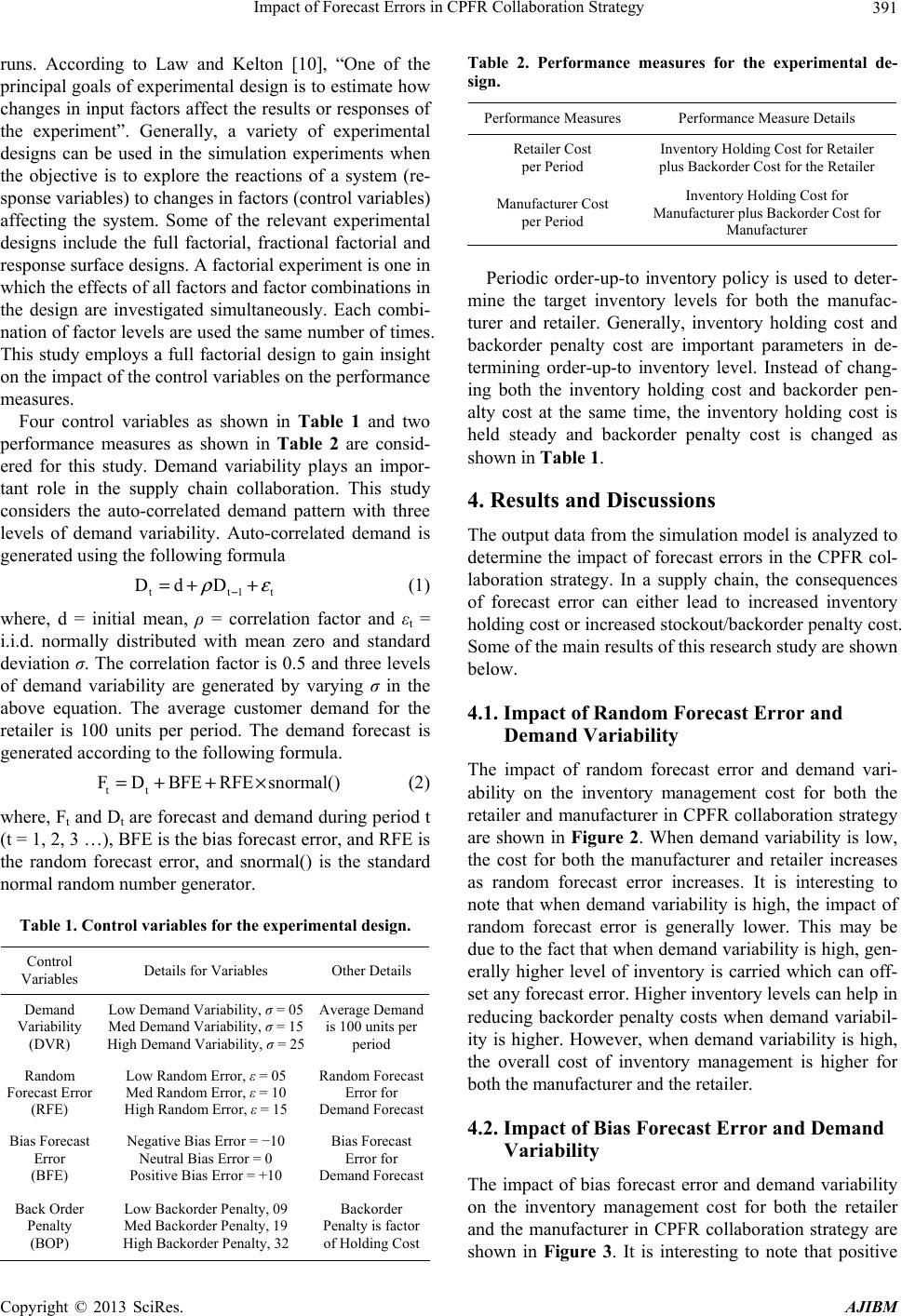
Impact of Forecast Errors in CPFR Collaboration Strategy 391
runs. According to Law and Kelton [10], “One of the
principal goals of experimental design is to estimate how
changes in input factors affect the results or responses of
the experiment”. Generally, a variety of experimental
designs can be used in the simulation experiments when
the objective is to explore the reactions of a system (re-
sponse variables) to changes in factors (control variables)
affecting the system. Some of the relevant experimental
designs include the full factorial, fractional factorial and
response surface designs. A factorial experiment is one in
which the effects of all factors and factor combinations in
the design are investigated simultaneously. Each combi-
nation of factor levels are used the same number of times.
This study employs a full factorial design to gain insight
on the impact of the control variables on the performance
measures.
Four control variables as shown in Table 1 and two
performance measures as shown in Table 2 are consid-
ered for this study. Demand variability plays an impor-
tant role in the supply chain collaboration. This study
considers the auto-correlated demand pattern with three
levels of demand variability. Auto-correlated demand is
generated using the following formula
tt1
Dd Dt
ε
−
=++ (1)
where, d = initial mean, ρ = correlation factor and εt =
i.i.d. normally distributed with mean zero and standard
deviation σ. The correlation factor is 0.5 and three levels
of demand variability are generated by varying σ in the
above equation. The average customer demand for the
retailer is 100 units per period. The demand forecast is
generated according to the following formula.
tt
FDBFERFEsnorml(a)=++ × (2)
where, Ft and Dt are forecast and demand during period t
(t = 1, 2, 3 …), BFE is the bias forecast error, and RFE is
the random forecast error, and snormal() is the standard
normal random number generator.
Table 1. Control variables for the experimental design.
Control
Variables Details for Variables Other Details
Demand
Variability
(DVR)
Low Demand Variability, σ = 05
Med Demand Variability, σ = 15
High Demand Variability, σ = 25
Average Demand
is 100 units per
period
Random
Forecast Error
(RFE)
Low Random Error, ε = 05
Med Random Error, ε = 10
High Random Error, ε = 15
Random Forecast
Error for
Demand Forecast
Bias Forecast
Error
(BFE)
Negative Bias Error = −10
Neutral Bias Error = 0
Positive Bias Error = +10
Bias Forecast
Error for
Demand Forecast
Back Order
Penalty
(BOP)
Low Backorder Penalty, 09
Med Backorder Penalty, 19
High Backorder Penalty, 32
Backorder
Penalty is factor
of Holding Cost
Table 2. Performance measures for the experimental de-
sign.
Performance Measures Performance Measure Details
Retailer Cost
per Period
Inventory Holding Cost for Retailer
plus Backorder Cost for the Retailer
Manufacturer Cost
per Period
Inventory Holding Cost for
Manufacturer plus Backorder Cost for
Manufacturer
Periodic order-up-to inventory policy is used to deter-
mine the target inventory levels for both the manufac-
turer and retailer. Generally, inventory holding cost and
backorder penalty cost are important parameters in de-
termining order-up-to inventory level. Instead of chang-
ing both the inventory holding cost and backorder pen-
alty cost at the same time, the inventory holding cost is
held steady and backorder penalty cost is changed as
shown in Table 1.
4. Results and Discussions
The output data from the simulation model is analyzed to
determine the impact of forecast errors in the CPFR col-
laboration strategy. In a supply chain, the consequences
of forecast error can either lead to increased inventory
holding cost or increased stockout/backorder penalty cost.
Some of the main results of this research study are shown
below.
4.1. Impact of Random Forecast Error and
Demand Variability
The impact of random forecast error and demand vari-
ability on the inventory management cost for both the
retailer and manufacturer in CPFR collaboration strategy
are shown in Figure 2. When demand variability is low,
the cost for both the manufacturer and retailer increases
as random forecast error increases. It is interesting to
note that when demand variability is high, the impact of
random forecast error is generally lower. This may be
due to the fact that when demand variability is high, gen-
erally higher level of inventory is carried which can off-
set any forecast error. Higher inventory levels can help in
reducing backorder penalty costs when demand variabil-
ity is higher. However, when demand variability is high,
the overall cost of inventory management is higher for
both the manufacturer and the retailer.
4.2. Impact of Bias Forecast Error and Demand
Variability
The impact of bias forecast error and demand variability
on the inventory management cost for both the retailer
and the manufacturer in CPFR collaboration strategy are
shown in Figure 3. It is interesting to note that positive
Copyright © 2013 SciRes. AJIBM