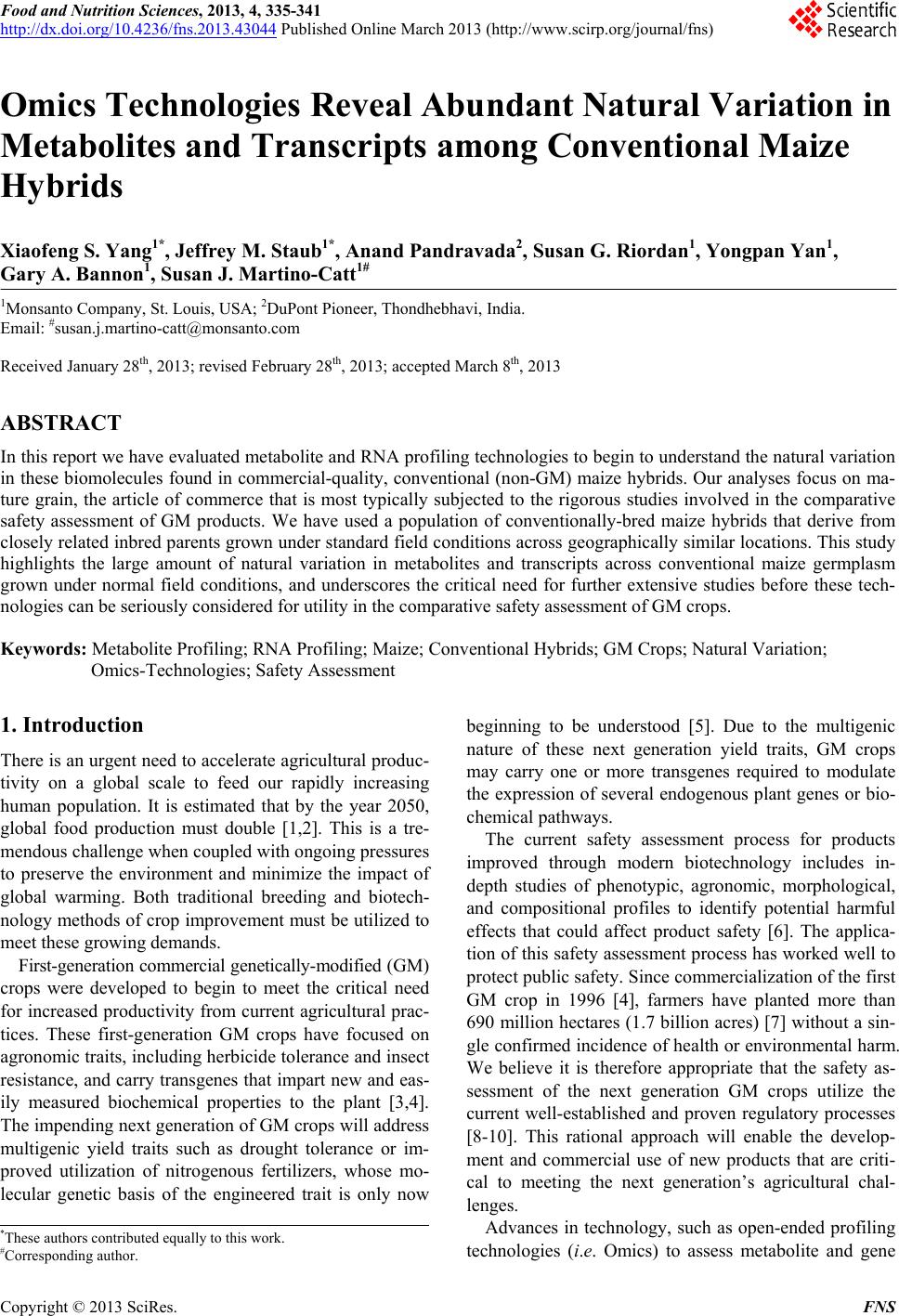 Food and Nutrition Sciences, 2013, 4, 335-341 http://dx.doi.org/10.4236/fns.2013.43044 Published Online March 2013 (http://www.scirp.org/journal/fns) Omics Technologies Reveal Abundant Natural Variation in Metabolites and Transcripts among Conventional Maize Hybrids Xiaofeng S. Yang1*, Jeffrey M. Staub1*, Anand Pandravada2, Susan G. Riordan1, Yongpan Yan1, Gary A. Bannon1, Susan J. Martino-Catt1# 1Monsanto Company, St. Louis, USA; 2DuPont Pioneer, Thondhebhavi, India. Email: #susan.j.martino-catt@monsanto.com Received January 28th, 2013; revised February 28th, 2013; accepted March 8th, 2013 ABSTRACT In this report we have evaluated metabolite and RNA profiling technologies to begin to und erstand the natural variation in these biomolecules found in commercial-quality, conventional (non-GM) maize hybrids. Our analyses focus on ma- ture grain, the article of commerce that is most typically subjected to the rigorous studies involved in the comparative safety assessment of GM products. We have used a population of conventionally-bred maize hybrids that derive from closely related inbred parents grown under standard field conditions across g eographically similar locati ons. This study highlights the large amount of natural variation in metabolites and transcripts across conventional maize germplasm grown under normal field conditions, and underscores the critical need for further extensive studies before these tech- nologies can be seriously considered for utility in the comparative safety assessment of GM crops. Keywords: Metabolite Profiling; RNA Profiling; Maize; Conventional Hybrids; GM Crops; Natural Variation; Omics-Technologies; Safety Assessment 1. Introduction There is an urgent need to accelerate agricultural produc- tivity on a global scale to feed our rapidly increasing human population. It is estimated that by the year 2050, global food production must double [1,2]. This is a tre- mendous challenge when coupled with ongoing pressures to preserve the environment and minimize the impact of global warming. Both traditional breeding and biotech- nology methods of crop improvement must be utilized to meet these growing demands. First-generation commercial g enetically-mod ified ( GM) crops were developed to begin to meet the critical need for increased productivity from current agricultural prac- tices. These first-generation GM crops have focused on agronomic traits, including herbicide tolerance and insect resistance, and carry transgenes that impart new and eas- ily measured biochemical properties to the plant [3,4]. The impending next generation of GM crops will address multigenic yield traits such as drought tolerance or im- proved utilization of nitrogenous fertilizers, whose mo- lecular genetic basis of the engineered trait is only now beginning to be understood [5]. Due to the multigenic nature of these next generation yield traits, GM crops may carry one or more transgenes required to modulate the expression of several endogenous plant genes or bio- chemical pathways. The current safety assessment process for products improved through modern biotechnology includes in- depth studies of phenotypic, agronomic, morphological, and compositional profiles to identify potential harmful effects that could affect product safety [6]. The applica- tion of this safety assessment pro cess has worked well to protect public safety. Since commercialization of the first GM crop in 1996 [4], farmers have planted more than 690 million h ectares (1.7 billion acres) [7] without a sin- gle confirmed incidence of health or environmental harm. We believe it is therefore appropriate that the safety as- sessment of the next generation GM crops utilize the current well-established and proven regulatory processes [8-10]. This rational approach will enable the develop- ment and commercial use of new products that are criti- cal to meeting the next generation’s agricultural chal- lenges. Advances in technology, such as open-ended profiling technologies (i.e. Omics) to assess metabolite and gene *These authors contributed equally to this work. #Corresponding author. Copyright © 2013 SciRes. FNS
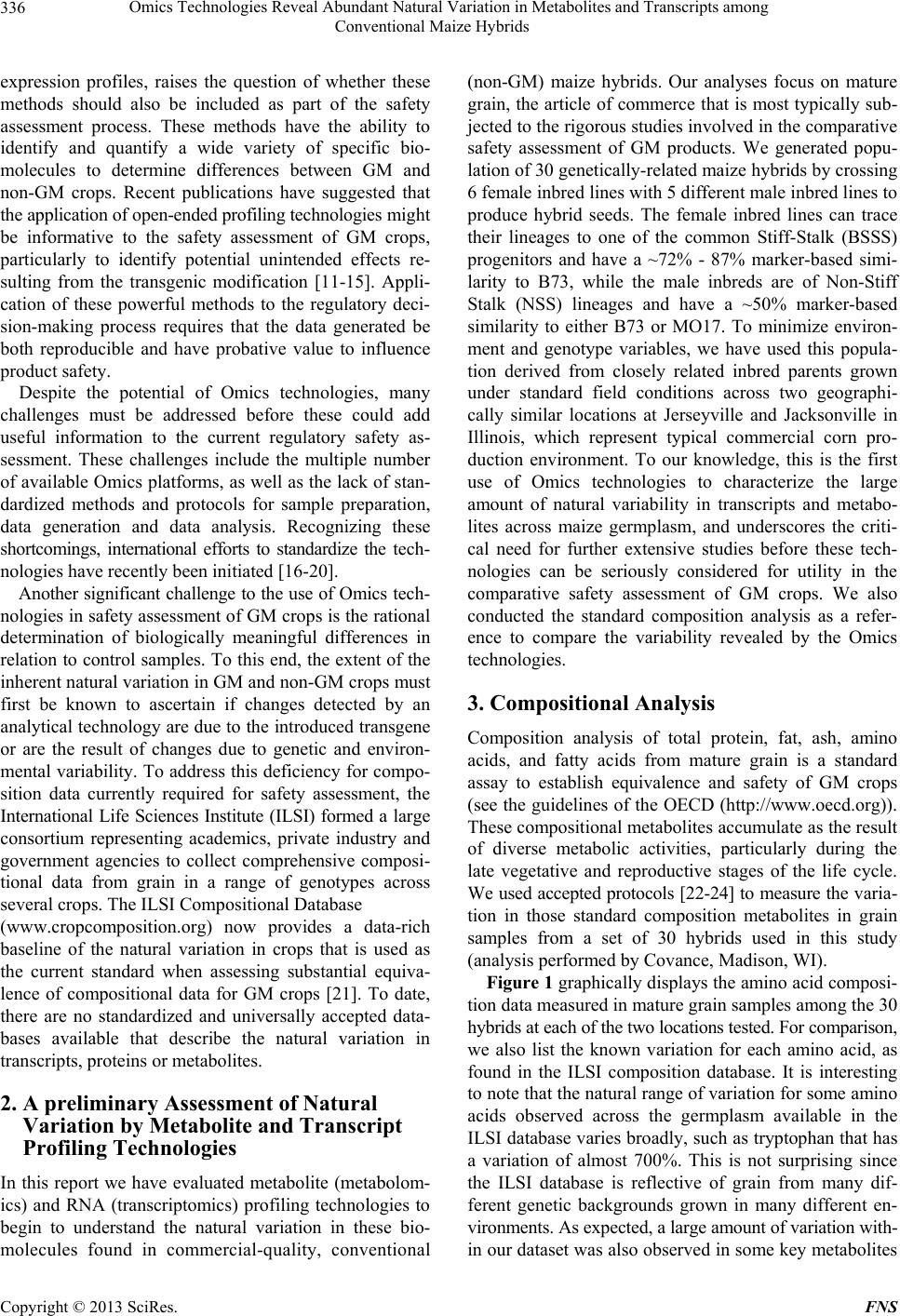 Omics Technologies Reveal Abundant Natural Variation in Metabolites and Transcripts among Conventional Maize Hybrids 336 expression profiles, raises the question of whether these methods should also be included as part of the safety assessment process. These methods have the ability to identify and quantify a wide variety of specific bio- molecules to determine differences between GM and non-GM crops. Recent publications have suggested that the application of open-ended profiling technologies mig ht be informative to the safety assessment of GM crops, particularly to identify potential unintended effects re- sulting from the transgenic modification [11-15]. Appli- cation of these powerful methods to the regulatory deci- sion-making process requires that the data generated be both reproducible and have probative value to influence product safety. Despite the potential of Omics technologies, many challenges must be addressed before these could add useful information to the current regulatory safety as- sessment. These challenges include the multiple number of available Omics platforms, as well as the lack of stan- dardized methods and protocols for sample preparation, data generation and data analysis. Recognizing these shortcomings, international efforts to standardize the tech- nologies have recently been initiated [16-20]. Another significant challenge to the use of Omics tec h- nologies in safety assessment of GM crops is the rational determination of biologically meaningful differences in relation to control samples. To this end, the extent of the inherent natural variation in GM and non-GM crops must first be known to ascertain if changes detected by an analytical technology are due to the introduced transgene or are the result of changes due to genetic and environ- mental variability. To address th is deficiency for compo- sition data currently required for safety assessment, the International Life Sciences Institute (ILSI) formed a lar ge consortium representing academics, private industry and government agencies to collect comprehensive composi- tional data from grain in a range of genotypes across several crops. The ILSI Compositional Database (www.cropcomposition.org) now provides a data-rich baseline of the natural variation in crops that is used as the current standard when assessing substantial equiva- lence of compositional data for GM crops [21]. To date, there are no standardized and universally accepted data- bases available that describe the natural variation in transcripts, proteins or metabolites. 2. A preliminary Assessment of Natural Variation by Metabolite and Transcript Profiling Technologies In this report we have evaluated metabolite (metabolom- ics) and RNA (transcriptomics) profiling technologies to begin to understand the natural variation in these bio- molecules found in commercial-quality, conventional (non-GM) maize hybrids. Our analyses focus on mature grain, the article of commerce that is most typically sub- jected to the rigorous studies involved in the comparative safety assessment of GM products. We generated popu- lation of 30 genetically-related maize hybrids by crossing 6 female inbred lines with 5 different male inbred lines to produce hybrid seeds. The female inbred lines can trace their lineages to one of the common Stiff-Stalk (BSSS) progenitors and have a ~72% - 87% marker-based simi- larity to B73, while the male inbreds are of Non-Stiff Stalk (NSS) lineages and have a ~50% marker-based similarity to either B73 or MO17. To minimize environ- ment and genotype variables, we have used this popula- tion derived from closely related inbred parents grown under standard field conditions across two geographi- cally similar locations at Jerseyville and Jacksonville in Illinois, which represent typical commercial corn pro- duction environment. To our knowledge, this is the first use of Omics technologies to characterize the large amount of natural variability in transcripts and metabo- lites across maize germplasm, and underscores the criti- cal need for further extensive studies before these tech- nologies can be seriously considered for utility in the comparative safety assessment of GM crops. We also conducted the standard composition analysis as a refer- ence to compare the variability revealed by the Omics technologies. 3. Compositional Analysis Composition analysis of total protein, fat, ash, amino acids, and fatty acids from mature grain is a standard assay to establish equivalence and safety of GM crops (see the guidelines of the OECD (http://www.oecd.org)). These compositional metabolites accumulate as the result of diverse metabolic activities, particularly during the late vegetative and reproductive stages of the life cycle. We used accepted protocols [22-24] to mea sure the var ia- tion in those standard composition metabolites in grain samples from a set of 30 hybrids used in this study (analysis performed by Covance, Madison, WI). Figure 1 graphically displays the amino acid composi- tion data measured in mature grain samples among the 30 hybrids at each of the two locations tested. For comparison, we also list the known variation for each amino acid, as found in the ILSI composition database. It is interesting to note that the natural range of variation for some amino acids observed across the germplasm available in the ILSI database varies broadly, such as tryptophan that has a variation of almost 700%. This is not surprising since the ILSI database is reflective of grain from many dif- ferent genetic backgrounds grown in many different en- vironments. As expected, a large amount of variation wit h- in our dataset was also observed in some key metabolites Copyright © 2013 SciRes. FNS
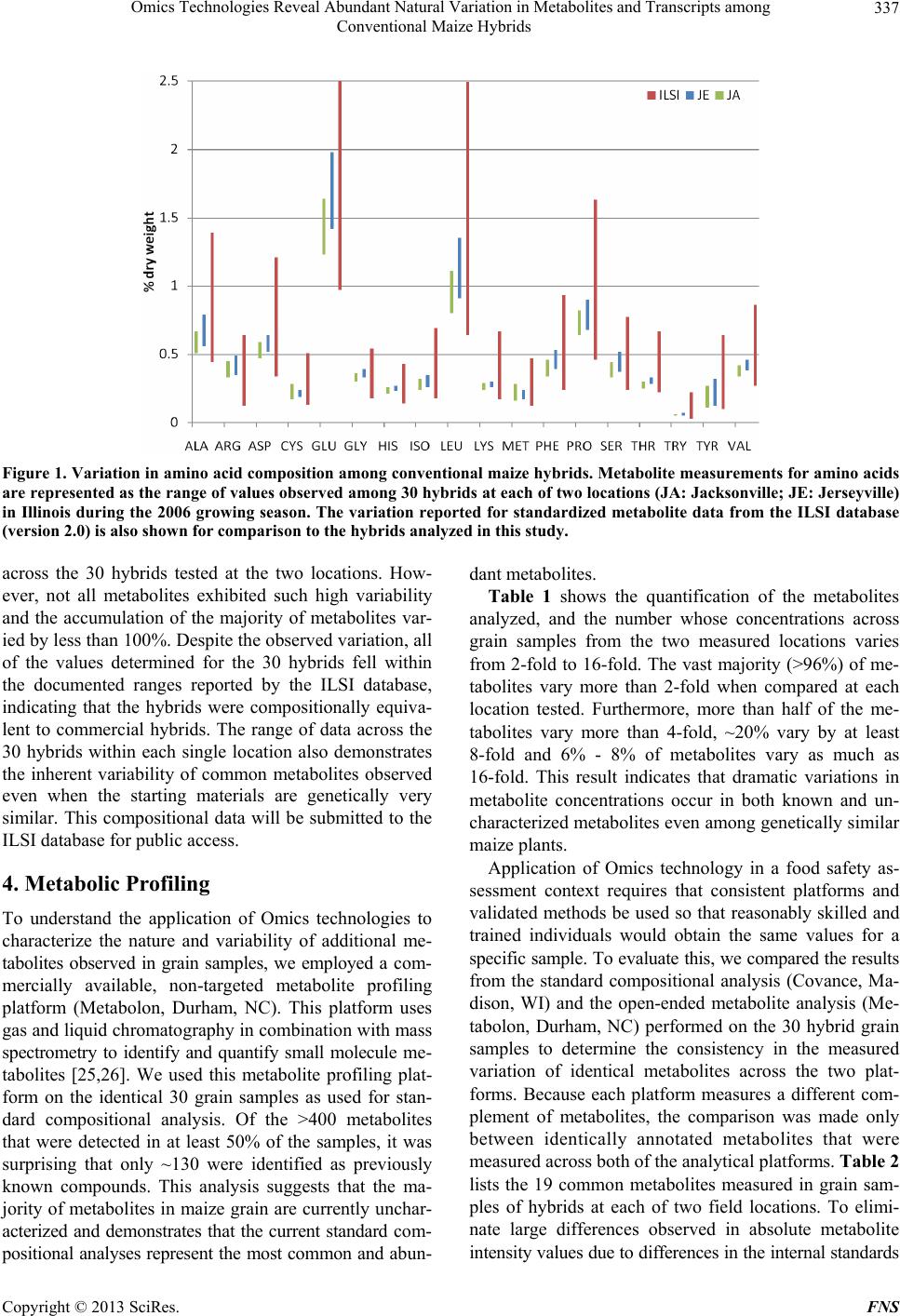 Omics Technologies Reveal Abundant Natural Variation in Metabolites and Transcripts among Conventional Maize Hybrids Copyright © 2013 SciRes. FNS 337 Figure 1. Variation in amino acid composition among conventional maize hybrids. Metabolite measurements for amino acids are represented as the range of values observed among 30 hybrids at each of two locations (JA: Jacksonville; JE: Jerseyville) in Illinois during the 2006 growing season. The variation reported for standardized metabolite data from the ILSI database (version 2.0) is also shown for comparison to the hybrids analyzed in this study . across the 30 hybrids tested at the two locations. How- ever, not all metabolites exhibited such high variability and the accumulation of the majority of metabolites var- ied by less than 100%. Despite th e observed v ariation, all of the values determined for the 30 hybrids fell within the documented ranges reported by the ILSI database, indicating that the hybrids were compositionally equiva- lent to commercial hybrids. The range of data across the 30 hybrids within each single location also demonstrates the inherent variability of common metabolites observed even when the starting materials are genetically very similar. This compositional data will be submitted to the ILSI database for public access. 4. Metabolic Profiling To understand the application of Omics technologies to characterize the nature and variability of additional me- tabolites observed in grain samples, we employed a com- mercially available, non-targeted metabolite profiling platform (Metabolon, Durham, NC). This platform uses gas and liquid chro matography in combinatio n with mass spectrometry to identify and quantify small molecule me- tabolites [25,26]. We used this metabolite profiling plat- form on the identical 30 grain samples as used for stan- dard compositional analysis. Of the >400 metabolites that were detected in at least 50% of the samples, it was surprising that only ~130 were identified as previously known compounds. This analysis suggests that the ma- jority of metabolites in maize grain are currently u nchar- acterized and demonstrates that the current standard com- positional analyses represent the most common and abun- dant metabolites. Table 1 shows the quantification of the metabolites analyzed, and the number whose concentrations across grain samples from the two measured locations varies from 2-fold to 16-fold. The vast majority (>96%) of me- tabolites vary more than 2-fold when compared at each location tested. Furthermore, more than half of the me- tabolites vary more than 4-fold, ~20% vary by at least 8-fold and 6% - 8% of metabolites vary as much as 16-fold. This result indicates that dramatic variations in metabolite concentrations occur in both known and un- characterized metabolites even among genetically similar maize plants. Application of Omics technology in a food safety as- sessment context requires that consistent platforms and validated methods be used so that reasonably skilled and trained individuals would obtain the same values for a specific sample. To evaluate this, we compared the results from the standard compositional analysis (Covance, Ma- dison, WI) and the open-ended metabolite analysis (Me- tabolon, Durham, NC) performed on the 30 hybrid grain samples to determine the consistency in the measured variation of identical metabolites across the two plat- forms. Because each platform measures a different com- plement of metabolites, the comparison was made only between identically annotated metabolites that were measured across both of the analytical platforms. Table 2 lists the 19 common metabolites measured in grain sam- ples of hybrids at each of two field locations. To elimi- nate large differences observed in absolute metabolite intensity va lues due to differen ces in the in tern al stand ards
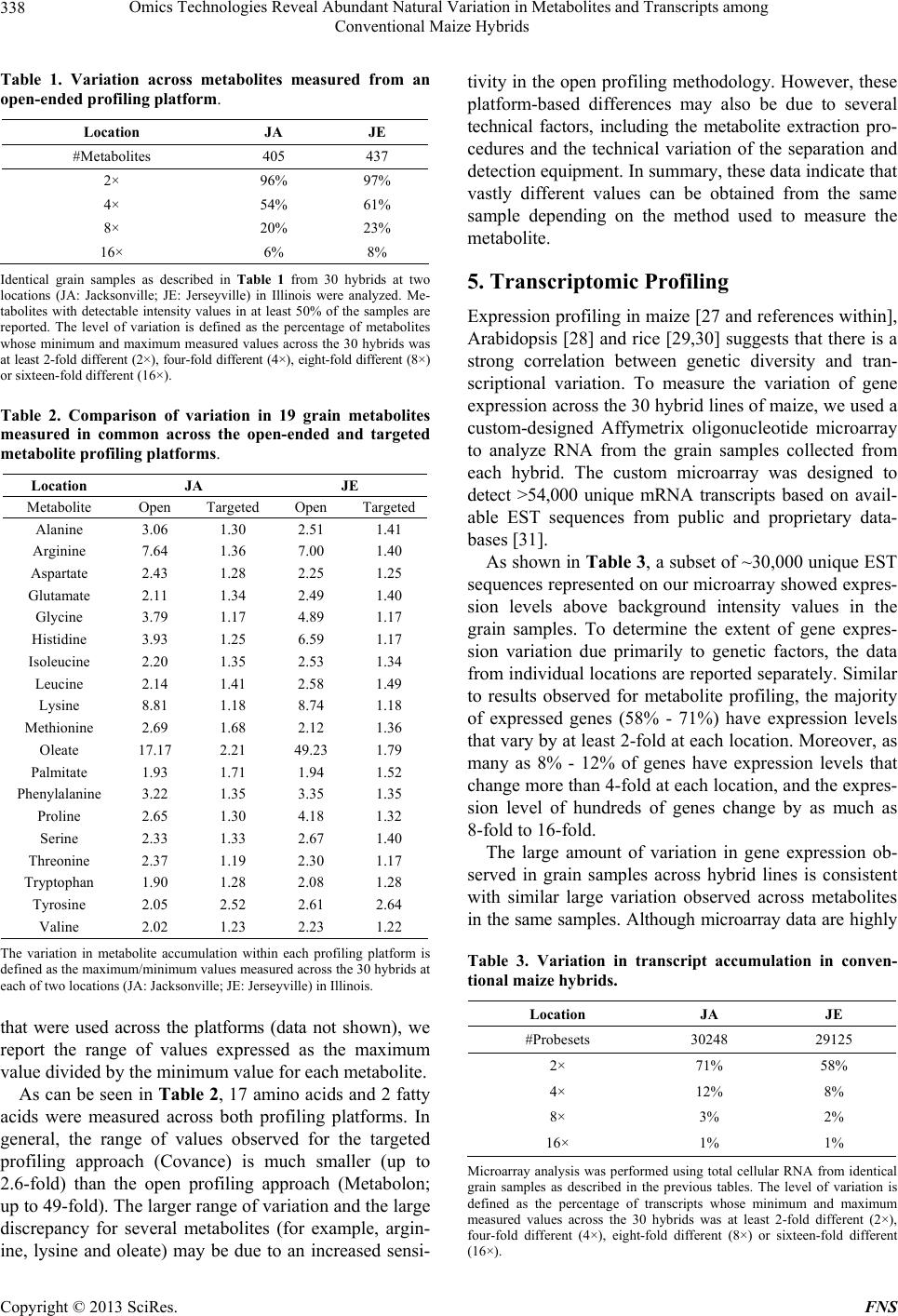 Omics Technologies Reveal Abundant Natural Variation in Metabolites and Transcripts among Conventional Maize Hybrids 338 Table 1. Variation across metabolites measured from an open-ended profiling platform. Location JA JE #Metabolites 405 437 2× 96% 97% 4× 54% 61% 8× 20% 23% 16× 6% 8% Identical grain samples as described in Table 1 from 30 hybrids at two locations (JA: Jacksonville; JE: Jerseyville) in Illinois were analyzed. Me- tabolites with detectable intensity values in at least 50% of the samples are reported. The level of variation is defined as the percentage of metabolites whose minimum and maximum measured values across the 30 hybrids was at least 2-fold different (2×), four-fold different (4×), eight-fold different (8×) or sixteen-fold differ ent (16×). Table 2. Comparison of variation in 19 grain metabolites measured in common across the open-ended and targeted metabolite profiling platforms. Location JA JE Metabolite Open Targeted Open Targeted Alanine 3.06 1.30 2.51 1.41 Arginine 7.64 1.36 7.00 1.40 Aspartate 2.43 1.28 2.25 1.25 Glutamate 2.11 1.34 2.49 1.40 Glycine 3.79 1.17 4.89 1.17 Histidine 3.93 1.25 6.59 1.17 Isoleucine 2.20 1.35 2.53 1.34 Leucine 2.14 1.41 2.58 1.49 Lysine 8.81 1.18 8.74 1.18 Methionine 2.69 1.68 2.12 1.36 Oleate 17.17 2.21 49.23 1.79 Palmitate 1.93 1.71 1.94 1.52 Phenylalanine 3.22 1.35 3.35 1.35 Proline 2.65 1.30 4.18 1.32 Serine 2.33 1.33 2.67 1.40 Threonine 2.37 1.19 2.30 1.17 Tryptophan 1.90 1.28 2.08 1.28 Tyrosine 2.05 2.52 2.61 2.64 Valine 2.02 1.23 2.23 1.22 The variation in metabolite accumulation within each profiling platform is defined as the maximum/minimum values measured across the 30 hybrids at each of two locations (JA: Jacksonville; JE: Jerseyville) in Illinois. that were used across the platforms (data not shown), we report the range of values expressed as the maximum value divided by the minimum value for each metabolite. As can be seen in Table 2 , 17 amino acids and 2 fatty acids were measured across both profiling platforms. In general, the range of values observed for the targeted profiling approach (Covance) is much smaller (up to 2.6-fold) than the open profiling approach (Metabolon; up to 49-fold). The larger range of variation and the large discrepancy for several metabolites (for example, argin- ine, lysine and oleate) may be due to an increased sensi- tivity in the open prof iling methodology. However, these platform-based differences may also be due to several technical factors, including the metabolite extraction pro- cedures and the technical variation of the separation and detection equipment. In summary, these data indicate that vastly different values can be obtained from the same sample depending on the method used to measure the metabolite. 5. Transcriptomic Profiling Expression profiling in maize [27 and references within], Arabidopsis [28] and rice [29,30] suggests that there is a strong correlation between genetic diversity and tran- scriptional variation. To measure the variation of gene expression across the 30 hybrid lines of maize, we used a custom-designed Affymetrix oligonucleotide microarray to analyze RNA from the grain samples collected from each hybrid. The custom microarray was designed to detect >54,000 unique mRNA transcripts based on avail- able EST sequences from public and proprietary data- bases [31] . As shown in Tab le 3, a su bset of ~30,000 unique EST sequences represented on our microarray showed expres- sion levels above background intensity values in the grain samples. To determine the extent of gene expres- sion variation due primarily to genetic factors, the data from individual locations are reported separately. Similar to results observed for metabolite profiling, the majority of expressed genes (58% - 71%) have expression levels that vary by at least 2-fold at each location. Moreover, as many as 8% - 12% of genes have expression levels that change more than 4-fold at each location, and the expr es- sion level of hundreds of genes change by as much as 8-fold to 16-fold. The large amount of variation in gene expression ob- served in grain samples across hybrid lines is consistent with similar large variation observed across metabolites in the same samples. Although microarray data are hig hly Table 3. Variation in transcript accumulation in conven- tional maize hybrids. Location JA JE #Probesets 30248 29125 2× 71% 58% 4× 12% 8% 8× 3% 2% 16× 1% 1% Microarray analysis was performed using total cellular RNA from identical grain samples as described in the previous tables. The level of variation is defined as the percentage of transcripts whose minimum and maximum measured values across the 30 hybrids was at least 2-fold different (2×), four-fold different (4×), eight-fold different (8×) or sixteen-fold different (16×). Copyright © 2013 SciRes. FNS
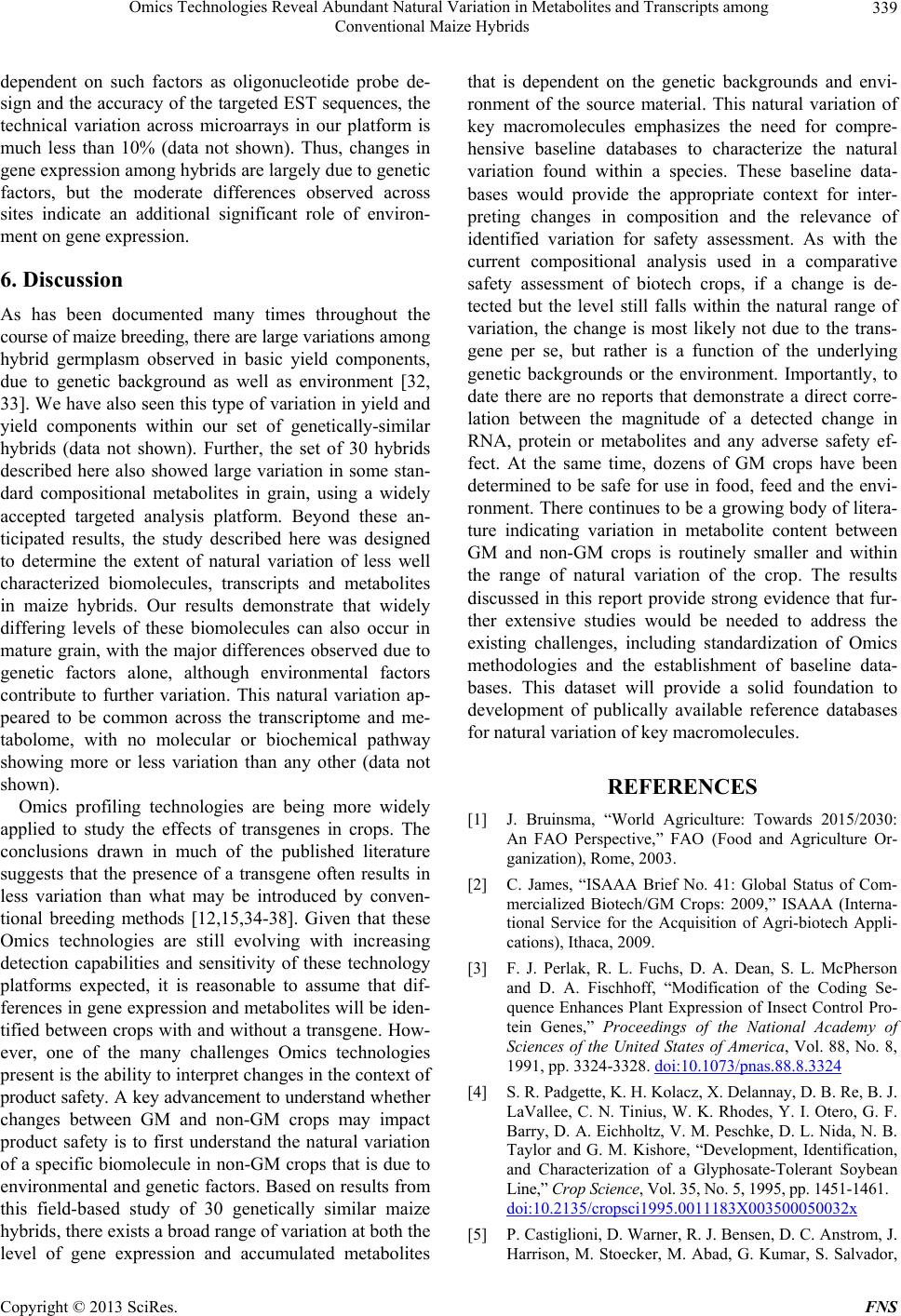 Omics Technologies Reveal Abundant Natural Variation in Metabolites and Transcripts among Conventional Maize Hybrids 339 dependent on such factors as oligonucleotide probe de- sign and the accuracy of the targeted EST sequences, the technical variation across microarrays in our platform is much less than 10% (data not shown). Thus, changes in gene expression among hybrids are largely due to genetic factors, but the moderate differences observed across sites indicate an additional significant role of environ- ment on gene expression. 6. Discussion As has been documented many times throughout the course of maize breeding, there are large var iations among hybrid germplasm observed in basic yield components, due to genetic background as well as environment [32, 33]. We have also seen this type of variation in yield and yield components within our set of genetically-similar hybrids (data not shown). Further, the set of 30 hybrids described here also showed large variation in some stan- dard compositional metabolites in grain, using a widely accepted targeted analysis platform. Beyond these an- ticipated results, the study described here was designed to determine the extent of natural variation of less well characterized biomolecules, transcripts and metabolites in maize hybrids. Our results demonstrate that widely differing levels of these biomolecules can also occur in mature grain, with the major differences observed due to genetic factors alone, although environmental factors contribute to further variation. This natural variation ap- peared to be common across the transcriptome and me- tabolome, with no molecular or biochemical pathway showing more or less variation than any other (data not shown). Omics profiling technologies are being more widely applied to study the effects of transgenes in crops. The conclusions drawn in much of the published literature suggests that the presence of a transgene often results in less variation than what may be introduced by conven- tional breeding methods [12,15,34-38]. Given that these Omics technologies are still evolving with increasing detection capabilities and sensitivity of these technology platforms expected, it is reasonable to assume that dif- ferences in gene expression and metabolites will b e iden- tified between crops with and without a transgene. How- ever, one of the many challenges Omics technologies present is the ab ility to in terpret chan ges in the contex t of product safety. A key advancement to understand wh eth er changes between GM and non-GM crops may impact product safety is to first understand the natural variation of a specific biomolecule in non-GM crops that is due to environmental and genetic factors. Based on results from this field-based study of 30 genetically similar maize hybrids, there exists a broad range of variation at both the level of gene expression and accumulated metabolites that is dependent on the genetic backgrounds and envi- ronment of the source material. This natural variation of key macromolecules emphasizes the need for compre- hensive baseline databases to characterize the natural variation found within a species. These baseline data- bases would provide the appropriate context for inter- preting changes in composition and the relevance of identified variation for safety assessment. As with the current compositional analysis used in a comparative safety assessment of biotech crops, if a change is de- tected but the level still falls within the natural range of variation, the change is most likely not due to the trans- gene per se, but rather is a function of the underlying genetic backgrounds or the environment. Importantly, to date there are no reports that demonstrate a direct corre- lation between the magnitude of a detected change in RNA, protein or metabolites and any adverse safety ef- fect. At the same time, dozens of GM crops have been determined to be safe for use in food, feed and the envi- ronment. There continues to be a growing body of litera- ture indicating variation in metabolite content between GM and non-GM crops is routinely smaller and within the range of natural variation of the crop. The results discussed in this report provide strong evidence that fur- ther extensive studies would be needed to address the existing challenges, including standardization of Omics methodologies and the establishment of baseline data- bases. This dataset will provide a solid foundation to development of publically available reference databases for natural variation of key macromolecules. REFERENCES [1] J. Bruinsma, “World Agriculture: Towards 2015/2030: An FAO Perspective,” FAO (Food and Agriculture Or- ganization), Rome, 2003. [2] C. James, “ISAAA Brief No. 41: Global Status of Com- mercialized Biotech/GM Crops: 2009,” ISAAA (Interna- tional Service for the Acquisition of Agri-biotech Appli- cations), Ithaca, 2009. [3] F. J. Perlak, R. L. Fuchs, D. A. Dean, S. L. McPherson and D. A. Fischhoff, “Modification of the Coding Se- quence Enhances Plant Expression of Insect Control Pro- tein Genes,” Proceedings of the National Academy of Sciences of the United States of America, Vol. 88, No. 8, 1991, pp. 3324-3328. doi:10.1073/pnas.88.8.3324 [4] S. R. Padgette, K. H. Kolacz, X. Delannay, D. B. Re, B. J. LaVallee, C. N. Tinius, W. K. Rhodes, Y. I. Otero, G. F. Barry, D. A. Eichholtz, V. M. Peschke, D. L. Nida, N. B. Taylor and G. M. Kishore, “Development, Identification, and Characterization of a Glyphosate-Tolerant Soybean Line,” Crop Science, Vol. 35, No. 5, 1995, pp. 1451-1461. doi:10.2135/cropsci1995.0011183X003500050032x [5] P. Castiglioni, D. Warner, R. J. Bensen, D. C. Anstrom, J. Harrison, M. Stoecker, M. Abad, G. Kumar, S. Salvador, Copyright © 2013 SciRes. FNS
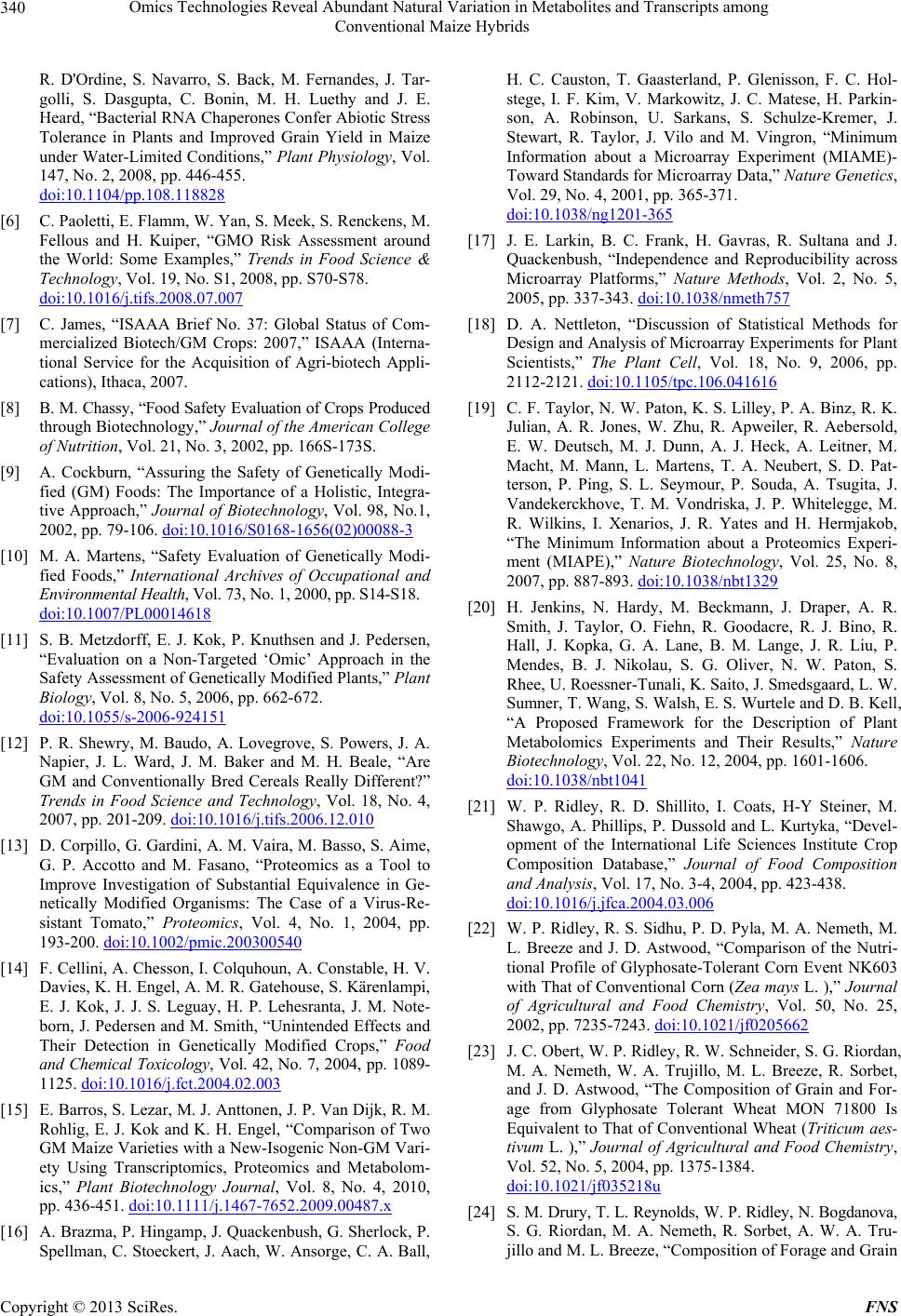 Omics Technologies Reveal Abundant Natural Variation in Metabolites and Transcripts among Conventional Maize Hybrids 340 R. D'Ordine, S. Navarro, S. Back, M. Fernandes, J. Tar- golli, S. Dasgupta, C. Bonin, M. H. Luethy and J. E. Heard, “Bacterial RNA Chaperones Confer Abiotic Stress Tolerance in Plants and Improved Grain Yield in Maize under Water-Limited Conditions,” Plant Physiology, Vol. 147, No. 2, 2008, pp. 446-455. doi:10.1104/pp.108.118828 [6] C. Paoletti , E. Flamm, W. Yan, S. Meek, S. Renckens, M. Fellous and H. Kuiper, “GMO Risk Assessment around the World: Some Examples,” Trends in Food Science & Technology, Vol. 19, No. S1, 2008, pp. S70-S78. doi:10.1016/j.tifs.2008.07.007 [7] C. James, “ISAAA Brief No. 37: Global Status of Com- mercialized Biotech/GM Crops: 2007,” ISAAA (Interna- tional Service for the Acquisition of Agri-biotech Appli- cations), Ithaca, 2007. [8] B. M. Chassy, “Food Safety Evaluation of Crop s Produced through Biotechnology,” Journal of the American College of Nutrition, Vol. 21, No. 3, 2002, pp. 166S-173S. [9] A. Cockburn, “Assuring the Safety of Genetically Modi- fied (GM) Foods: The Importance of a Holistic, Integra- tive Approach,” Journal of Biotechnology, Vol. 98, No.1, 2002, pp. 79-106. doi:10.1016/S0168-1656(02)00088-3 [10] M. A. Martens, “Safety Evaluation of Genetically Modi- fied Foods,” International Archives of Occupational and Environmental Health, Vol. 73, No. 1, 2000, pp. S14-S18. doi:10.1007/PL00014618 [11] S. B. Metzdorff, E. J. Kok, P. Knuthsen and J. Pedersen, “Evaluation on a Non-Targeted ‘Omic’ Approach in the Safety Assessment of Genetically Modified Plants,” Plant Biology, Vol. 8, No. 5, 2006, pp. 662-672. doi:10.1055/s-2006-924151 [12] P. R. Shewry, M. Baudo, A. Lovegrove, S. Powers, J. A. Napier, J. L. Ward, J. M. Baker and M. H. Beale, “Are GM and Conventionally Bred Cereals Really Different?” Trends in Food Science and Technology, Vol. 18, No. 4, 2007, pp. 201-209. doi:10.1016/j.tifs.2006.12.010 [13] D. Corpillo, G. Gardini, A. M. Vaira, M. Basso, S. Aime, G. P. Accotto and M. Fasano, “Proteomics as a Tool to Improve Investigation of Substantial Equivalence in Ge- netically Modified Organisms: The Case of a Virus-Re- sistant Tomato,” Proteomics, Vol. 4, No. 1, 2004, pp. 193-200. doi:10.1002/pmic.200300540 [14] F. Cellini, A. Chesson, I. Colquhoun, A. Constable, H. V. Davies, K. H. Engel, A. M. R. Gatehouse, S. Kärenlampi, E. J. Kok, J. J. S. Leguay, H. P. Lehesranta, J. M. Note- born, J. Pedersen and M. Smith, “Unintended Effects and Their Detection in Genetically Modified Crops,” Food and Chemical Toxicology, Vol. 42, No. 7, 2004, pp. 1089- 1125. doi:10.1016/j.fct.2004.02.003 [15] E. Barros, S. Lezar, M. J. Anttonen, J. P. Van Dijk, R. M. Rohlig, E. J. Kok and K. H. Engel, “Comparison of Two GM Maize Varieties with a New-Isogenic Non-GM Vari- ety Using Transcriptomics, Proteomics and Metabolom- ics,” Plant Biotechnology Journal, Vol. 8, No. 4, 2010, pp. 436-451. doi:10.1111/j.1467-7652.2009.00487.x [16] A. Brazma, P. Hingamp, J. Quackenbush, G. Sherlock, P. Spellman, C. Stoeckert, J. Aach, W. Ansorge, C. A. Ball, H. C. Causton, T. Gaasterland, P. Glenisson, F. C. Hol- stege, I. F. Kim, V. Markowitz, J. C. Matese, H. Parkin- son, A. Robinson, U. Sarkans, S. Schulze-Kremer, J. Stewart, R. Taylor, J. Vilo and M. Vingron, “Minimum Information about a Microarray Experiment (MIAME)- Toward Standards for Microarray Data,” Nature G enetics, Vol. 29, No. 4, 2001, pp. 365-371. doi:10.1038/ng1201-365 [17] J. E. Larkin, B. C. Frank, H. Gavras, R. Sultana and J. Quackenbush, “Independence and Reproducibility across Microarray Platforms,” Nature Methods, Vol. 2, No. 5, 2005, pp. 337-343. doi:10.1038/nmeth757 [18] D. A. Nettleton, “Discussion of Statistical Methods for Design and Analysis of Microarray Experiments for Plant Scientists,” The Plant Cell, Vol. 18, No. 9, 2006, pp. 2112-2121. doi:10.1105/tpc.106.041616 [19] C. F. Taylor, N. W. Paton, K. S. Lilley, P. A. Binz, R. K. Julian, A. R. Jones, W. Zhu, R. Apweiler, R. Aebersold, E. W. Deutsch, M. J. Dunn, A. J. Heck, A. Leitner, M. Macht, M. Mann, L. Martens, T. A. Neubert, S. D. Pat- terson, P. Ping, S. L. Seymour, P. Souda, A. Tsugita, J. Vandekerckhove, T. M. Vondriska, J. P. Whitelegge, M. R. Wilkins, I. Xenarios, J. R. Yates and H. Hermjakob, “The Minimum Information about a Proteomics Experi- ment (MIAPE),” Nature Biotechnology, Vol. 25, No. 8, 2007, pp. 887-893. doi:10.1038/nbt1329 [20] H. Jenkins, N. Hardy, M. Beckmann, J. Draper, A. R. Smith, J. Taylor, O. Fiehn, R. Goodacre, R. J. Bino, R. Hall, J. Kopka, G. A. Lane, B. M. Lange, J. R. Liu, P. Mendes, B. J. Nikolau, S. G. Oliver, N. W. Paton, S. Rhee, U. Roessner-Tunali, K. Saito, J. Smedsgaard, L. W. Sumner, T. Wang, S. Wal sh, E. S. Wurtel e and D. B. Kell , “A Proposed Framework for the Description of Plant Metabolomics Experiments and Their Results,” Nature Biotechnology, Vol. 22, No. 12, 2004, pp. 1601-1606. doi:10.1038/nbt1041 [21] W. P. Ridley, R. D. Shillito, I. Coats, H-Y Steiner, M. Shawgo, A. Phillips, P. Dussold and L. Kurtyka, “Devel- opment of the International Life Sciences Institute Crop Composition Database,” Journal of Food Composition and Analysis, Vol. 17, No. 3-4, 2004, pp. 423-438. doi:10.1016/j.jfca.2004.03.006 [22] W. P. Ridley, R. S. Sidhu, P. D. Pyla, M. A. Nemeth, M. L. Breeze and J. D. Astwood, “Comparison of the Nutri- tional Profile of Glyphosate-Tolerant Corn Event NK603 with That of Conventional Corn (Zea mays L. ),” Journal of Agricultural and Food Chemistry, Vol. 50, No. 25, 2002, pp. 7235-7243. doi:10.1021/jf0205662 [23] J. C. Obert, W. P. Ridley, R. W. Schneider, S. G. Riordan, M. A. Nemeth, W. A. Trujillo, M. L. Breeze, R. Sorbet, and J. D. Astwood, “The Composition of Grain and For- age from Glyphosate Tolerant Wheat MON 71800 Is Equivalent to That of Conventional Wheat (Triticum aes- tivum L. ),” Journal of Agricultural and Food Chemistry, Vol. 52, No. 5, 2004, pp. 1375-1384. doi:10.1021/jf035218u [24] S. M. Drury, T. L. Reynolds, W. P. Ridley, N. Bo gdanova, S. G. Riordan, M. A. Nemeth, R. Sorbet, A. W. A. Tru- jillo and M. L. Breeze, “Composition of Forage and Grain Copyright © 2013 SciRes. FNS
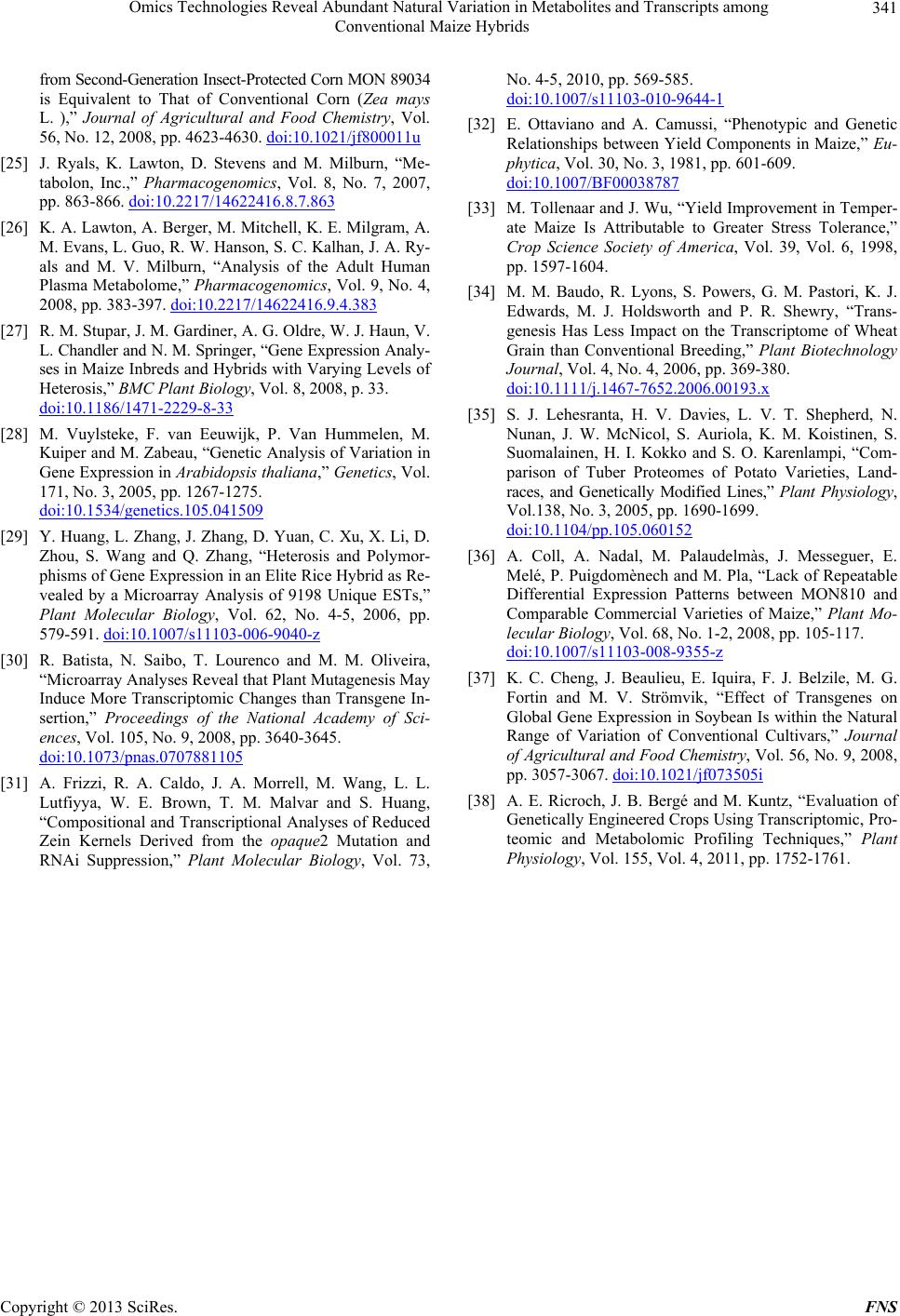 Omics Technologies Reveal Abundant Natural Variation in Metabolites and Transcripts among Conventional Maize Hybrids Copyright © 2013 SciRes. FNS 341 from Second-Generation Insect-Protected Corn MON 89034 is Equivalent to That of Conventional Corn (Zea mays L. ),” Journal of Agricultural and Food Chemistry, Vol. 56, No. 12, 2008, pp. 4623-4630. doi:10.1021/jf800011u [25] J. Ryals, K. Lawton, D. Stevens and M. Milburn, “Me- tabolon, Inc.,” Pharmacogenomics, Vol. 8, No. 7, 2007, pp. 863-866. doi:10.2217/14622416.8.7.863 [26] K. A. Lawton, A. Berger, M. Mitchell , K. E. Milgram, A. M. Evans, L. Guo, R. W. Hanson, S. C. Kalhan, J. A. Ry- als and M. V. Milburn, “Analysis of the Adult Human Plasma Metabolome,” Pharmacogenomics, Vol. 9, No. 4, 2008, pp. 383-397. doi:10.2217/14622416.9.4.383 [27] R. M. Stupar, J. M. Gardiner, A. G. Oldre, W. J. Haun, V. L. Chandler and N. M. Springer, “Gene Expression Analy - ses in Maize Inbreds and Hybrids with Varying Levels of Heterosis,” BMC Plant Biology, Vol. 8, 2008, p. 33. doi:10.1186/1471-2229-8-33 [28] M. Vuylsteke, F. van Eeuwijk, P. Van Hummelen, M. Kuiper and M. Zabeau, “Genetic Analysis of Variation in Gene Expression in Arabidopsis thaliana,” Genetics, Vol. 171, No. 3, 2005, pp. 1267-1275. doi:10.1534/genetics.105.041509 [29] Y. Huang, L. Zhang, J. Zhang, D. Yuan, C. Xu, X. Li, D. Zhou, S. Wang and Q. Zhang, “Heterosis and Polymor- phisms of Gene Expression in an Elite Rice Hybrid as Re- vealed by a Microarray Analysis of 9198 Unique ESTs,” Plant Molecular Biology, Vol. 62, No. 4-5, 2006, pp. 579-591. doi:10.1007/s11103-006-9040-z [30] R. Batista, N. Saibo, T. Lourenco and M. M. Oliveira, “Microarray Analyses Reveal that Plant Mutagenesis May Induce More Transcriptomic Changes than Transgene In- sertion,” Proceedings of the National Academy of Sci- ences, Vol. 105, No. 9, 2008, pp. 3640-3645. doi:10.1073/pnas.0707881105 [31] A. Frizzi, R. A. Caldo, J. A. Morrell, M. Wang, L. L. Lutfiyya, W. E. Brown, T. M. Malvar and S. Huang, “Compositional and Transcriptional Analyses of Reduced Zein Kernels Derived from the opaque2 Mutation and RNAi Suppression,” Plant Molecular Biology, Vol. 73, No. 4-5, 2010, pp. 569-585. doi:10.1007/s11103-010-9644-1 [32] E. Ottaviano and A. Camussi, “Phenotypic and Genetic Relationships between Yield Components in Maize,” Eu- phytica, Vol. 30, No. 3, 1981, pp. 601-609. doi:10.1007/BF00038787 [33] M. Tollenaar and J. Wu, “Yield Improvement in Temper- ate Maize Is Attributable to Greater Stress Tolerance,” Crop Science Society of America, Vol. 39, Vol. 6, 1998, pp. 1597-1604. [34] M. M. Baudo, R. Lyons, S. Powers, G. M. Pastori, K. J. Edwards, M. J. Holdsworth and P. R. Shewry, “Trans- genesis Has Less Impact on the Transcriptome of Wheat Grain than Conventional Breeding,” Plant Biotechnology Journal, Vol. 4, No. 4, 2006, pp. 369-380. doi:10.1111/j.1467-7652.2006.00193.x [35] S. J. Lehesranta, H. V. Davies, L. V. T. Shepherd, N. Nunan, J. W. McNicol, S. Auriola, K. M. Koistinen, S. Suomalainen, H. I. Kokko and S. O. Karenlampi, “Com- parison of Tuber Proteomes of Potato Varieties, Land- races, and Genetically Modified Lines,” Plant Physiology, Vol.138, No. 3, 2005, pp. 1690-1699. doi:10.1104/pp.105.060152 [36] A. Coll, A. Nadal, M. Palaudelmàs, J. Messeguer, E. Melé, P. Puigdomènech and M. Pla, “Lack of Repeatable Differential Expression Patterns between MON810 and Comparable Commercial Varieties of Maize,” Plant Mo- lecular Biology, Vol. 68, No. 1-2, 2008, pp. 105-117. doi:10.1007/s11103-008-9355-z [37] K. C. Cheng, J. Beaulieu, E. Iquira, F. J. Belzile, M. G. Fortin and M. V. Strömvik, “Effect of Transgenes on Global Gene Expression in Soybean Is within the Natural Range of Variation of Conventional Cultivars,” Journal of Agricultural and Food Che mistry, Vol. 56, No. 9, 2008, pp. 3057-3067. doi:10.1021/jf073505i [38] A. E. Ricroch, J. B. Bergé and M. Kuntz, “Evaluation of Genetically Engineered Crops Using Transcriptomic, Pro- teomic and Metabolomic Profiling Techniques,” Plant Physiology, Vol. 155, Vol. 4, 2011, pp. 1752-1761.
|