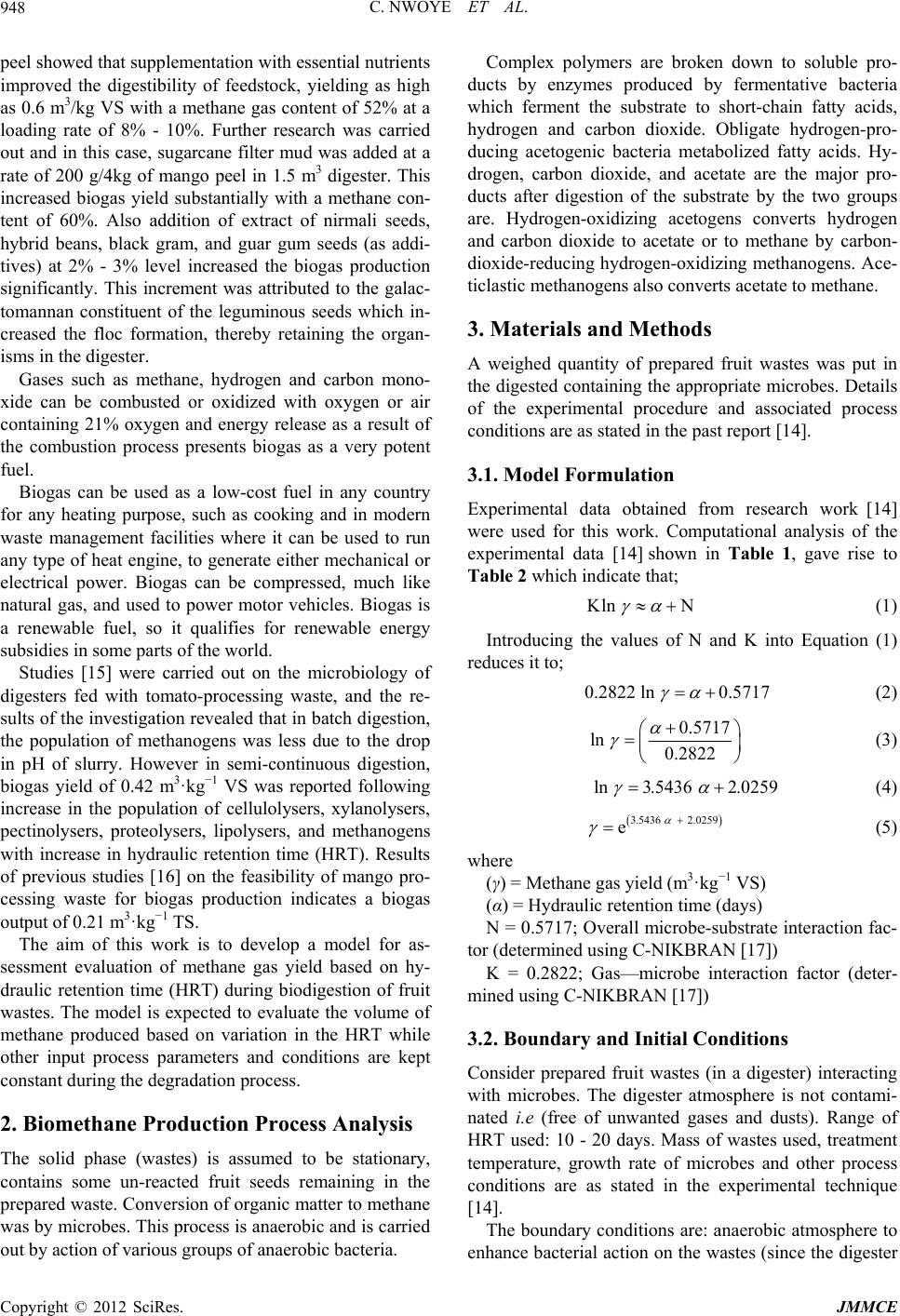
C. NWOYE ET AL.
948
peel showed that supplementation with essential nutrients
improved the digestibility of feedstock, yielding as high
as 0.6 m3/kg VS with a methane gas content of 52% at a
loading rate of 8% - 10%. Further research was carried
out and in this case, sugarcane filter mud was added at a
rate of 200 g/4kg of mango peel in 1.5 m3 digester. This
increased biogas yield substantially with a methane con-
tent of 60%. Also addition of extract of nirmali seeds,
hybrid beans, black gram, and guar gum seeds (as addi-
tives) at 2% - 3% level increased the biogas production
significantly. This increment was attributed to the galac-
tomannan constituent of the leguminous seeds which in-
creased the floc formation, thereby retaining the organ-
isms in the digester.
Gases such as methane, hydrogen and carbon mono-
xide can be combusted or oxidized with oxygen or air
containing 21% oxygen and energy release as a result of
the combustion process presents biogas as a very potent
fuel.
Biogas can be used as a low-cost fuel in any country
for any heating purpose, such as cooking and in modern
waste management facilities where it can be used to run
any type of heat engine, to generate either mechanical or
electrical power. Biogas can be compressed, much like
natural gas, and used to power motor vehicles. Biogas is
a renewable fuel, so it qualifies for renewable energy
subsidies in some parts of the world.
Studies [15] were carried out on the microbiology of
digesters fed with tomato-processing waste, and the re-
sults of the investigation revealed that in batch digestion,
the population of methanogens was less due to the drop
in pH of slurry. However in semi-continuous digestion,
biogas yield of 0.42 m3·kg−1 VS was reported following
increase in the population of cellulolysers, xylanolysers,
pectinolysers, proteolysers, lipolysers, and methanogens
with increase in hydraulic retention time (HRT). Results
of previous studies [16] on the feasibility of mango pro-
cessing waste for biogas production indicates a biogas
output of 0.21 m3·kg−1 TS.
The aim of this work is to develop a model for as-
sessment evaluation of methane gas yield based on hy-
draulic retention time (HRT) during biodigestion of fruit
wastes. The model is expected to evaluate the volume of
methane produced based on variation in the HRT while
other input process parameters and conditions are kept
constant during the degradation process.
2. Biomethane Production Process Analysis
The solid phase (wastes) is assumed to be stationary,
contains some un-reacted fruit seeds remaining in the
prepared waste. Conversion of organic matter to methane
was by microbes. This process is anaerobic and is carried
out by action of various groups of anaerobic bacteria.
Complex polymers are broken down to soluble pro-
ducts by enzymes produced by fermentative bacteria
which ferment the substrate to short-chain fatty acids,
hydrogen and carbon dioxide. Obligate hydrogen-pro-
ducing acetogenic bacteria metabolized fatty acids. Hy-
drogen, carbon dioxide, and acetate are the major pro-
ducts after digestion of the substrate by the two groups
are. Hydrogen-oxidizing acetogens converts hydrogen
and carbon dioxide to acetate or to methane by carbon-
dioxide-reducing hydrogen-oxidizing methanogens. Ace-
ticlastic methanogens also converts acetate to methane.
3. Materials and Methods
A weighed quantity of prepared fruit wastes was put in
the digested containing the appropriate microbes. Details
of the experimental procedure and associated process
conditions are as stated in the past report [14].
3.1. Model Formulation
Experimental data obtained from research work [14]
were used for this work. Computational analysis of the
experimental data [14] shown in Table 1, gave rise to
Table 2 which indicate that;
Kln N
(1)
Introducing the values of N and K into Equation (1)
reduces it to;
0.2822 ln 0.5717
(2)
0.5717
ln 0.2822
(3)
ln 3.5436 2.0259
(4)
3.5436 2.0259
e
(5)
where
(γ) = Methane gas yield (m3·kg−1 VS)
(α) = Hydraulic retention time (days)
N = 0.5717; Overall microbe-substrate interaction fac-
tor (determined using C-NIKBRAN [17])
K = 0.2822; Gas—microbe interaction factor (deter-
mined using C-NIKBRAN [17])
3.2. Boundary and Initial Conditions
Consider prepared fruit wastes (in a digester) interacting
with microbes. The digester atmosphere is not contami-
nated i.e (free of unwanted gases and dusts). Range of
HRT used: 10 - 20 days. Mass of wastes used, treatment
temperature, growth rate of microbes and other process
conditions are as stated in the experimental technique
[14].
The boundary conditions are: anaerobic atmosphere to
enhance bacterial action on the wastes (since the digester
Copyright © 2012 SciRes. JMMCE