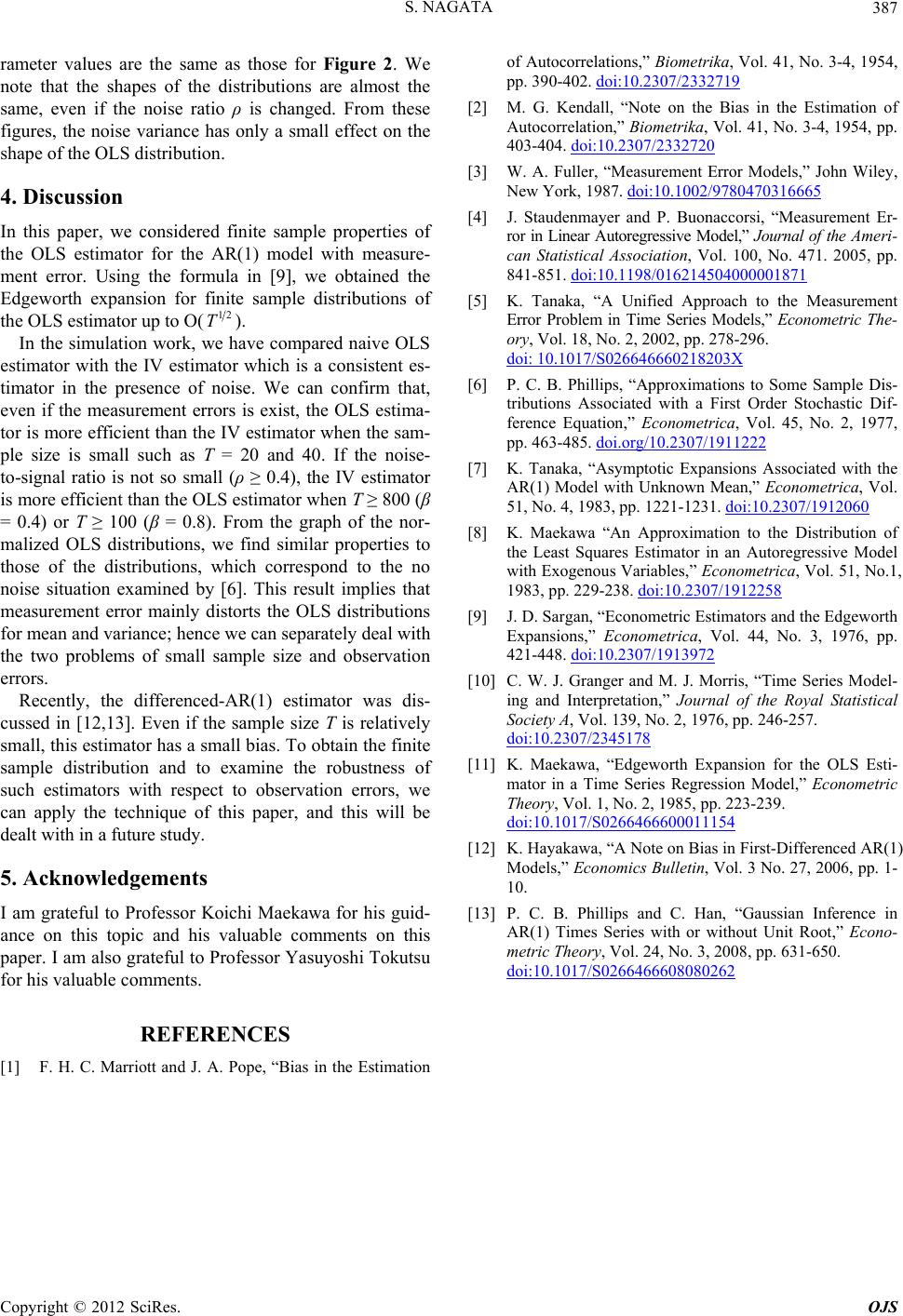
S. NAGATA 387
rameter values are the same as those for Figure 2. We
note that the shapes of the distributions are almost the
same, even if the noise ratio ρ is changed. From these
figures, the noise variance has only a small effect on the
shape of the OLS distribution.
4. Discussion
In this paper, we considered finite sample properties of
the OLS estimator for the AR(1) model with measure-
ment error. Using the formula in [9], we obtained the
Edgeworth expansion for finite sample distributions of
the OLS estimator up to O(12
T).
In the simulation work, we have compared naive OLS
estimator with the IV estimator which is a consistent es-
timator in the presence of noise. We can confirm that,
even if the measurement errors is exist, the OLS estima-
tor is more efficient than the IV estimator when the sam-
ple size is small such as T = 20 and 40. If the noise-
to-signal ratio is not so small (ρ ≥ 0.4), the IV estimator
is more efficient than the OLS estimator when T ≥ 800 (β
= 0.4) or T ≥ 100 (β = 0.8). From the graph of the nor-
malized OLS distributions, we find similar properties to
those of the distributions, which correspond to the no
noise situation examined by [6]. This result implies that
measurement error mainly distorts the OLS distributions
for mean and variance; hence we can separately deal with
the two problems of small sample size and observation
errors.
Recently, the differenced-AR(1) estimator was dis-
cussed in [12,13]. Even if the sample size T is relatively
small, this estimator has a small bias. To obtain the finite
sample distribution and to examine the robustness of
such estimators with respect to observation errors, we
can apply the technique of this paper, and this will be
dealt with in a future study.
5. Acknowledgements
I am grateful to Professor Koichi Maekawa for his guid-
ance on this topic and his valuable comments on this
paper. I am also grateful to Professor Yasuyoshi Tokutsu
for his valuable comments.
REFERENCES
[1] F. H. C. Marriott and J. A. Pope, “Bias in the Estimation
of Autocorrelations,” Biometrika, Vol. 41, No. 3-4, 1954,
pp. 390-402. doi:10.2307/2332719
[2] M. G. Kendall, “Note on the Bias in the Estimation of
Autocorrelation,” Biometrika, Vol. 41, No. 3-4, 1954, pp.
403-404. doi:10.2307/2332720
[3] W. A. Fuller, “Measurement Error Models,” John Wiley,
New York, 1987. doi:10.1002/9780470316665
[4] J. Staudenmayer and P. Buonaccorsi, “Measurement Er-
ror in Linear Autoregressive Model,” Journal of the Ameri-
can Statistical Association, Vol. 100, No. 471. 2005, pp.
841-851. doi:10.1198/016214504000001871
[5] K. Tanaka, “A Unified Approach to the Measurement
Error Problem in Time Series Models,” Econometric The-
ory, Vol. 18, No. 2, 2002, pp. 278-296.
doi: 10.1017/S026646660218203X
[6] P. C. B. Phillips, “Approximations to Some Sample Dis-
tributions Associated with a First Order Stochastic Dif-
ference Equation,” Econometrica, Vol. 45, No. 2, 1977,
pp. 463-485. doi.org/10.2307/1911222
[7] K. Tanaka, “Asymptotic Expansions Associated with the
AR(1) Model with Unknown Mean,” Econometrica, Vol.
51, No. 4, 1983, pp. 1221-1231. doi:10.2307/1912060
[8] K. Maekawa “An Approximation to the Distribution of
the Least Squares Estimator in an Autoregressive Model
with Exogenous Variables,” Econometrica, Vol. 51, No.1,
1983, pp. 229-238. doi:10.2307/1912258
[9] J. D. Sargan, “Econometric Estimators and the Edgeworth
Expansions,” Econometrica, Vol. 44, No. 3, 1976, pp.
421-448. doi:10.2307/1913972
[10] C. W. J. Granger and M. J. Morris, “Time Series Model-
ing and Interpretation,” Journal of the Royal Statistical
Society A, Vol. 139, No. 2, 1976, pp. 246-257.
doi:10.2307/2345178
[11] K. Maekawa, “Edgeworth Expansion for the OLS Esti-
mator in a Time Series Regression Model,” Econometric
Theory, Vol. 1, No. 2, 1985, pp. 223-239.
doi:10.1017/S0266466600011154
[12] K. Hayakawa, “A Note on Bias in First-Differenced AR(1)
Models,” Economics Bulletin, Vol. 3 No. 27, 2006, pp. 1-
10.
[13] P. C. B. Phillips and C. Han, “Gaussian Inference in
AR(1) Times Series with or without Unit Root,” Econo-
metric Theory, Vol. 24, No. 3, 2008, pp. 631-650.
doi:10.1017/S0266466608080262
Copyright © 2012 SciRes. OJS