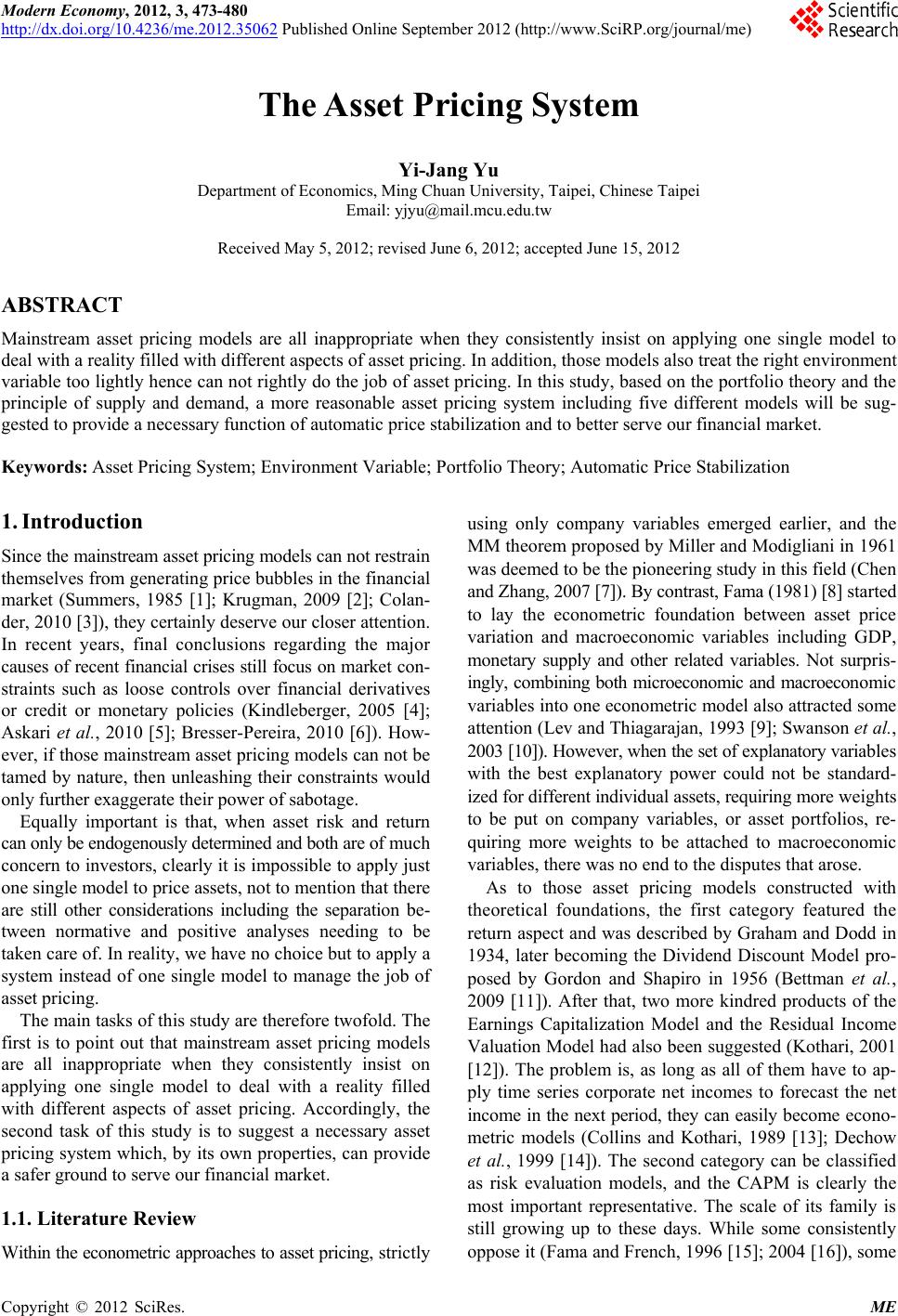 Modern Economy, 2012, 3, 473-480 http://dx.doi.org/10.4236/me.2012.35062 Published Online September 2012 (http://www.SciRP.org/journal/me) The Asset Pricing System Yi-Jang Yu Department of Economics, Ming Chuan University, Taipei, Chinese Taipei Email: yjyu@mail.mcu.edu.tw Received May 5, 2012; revised June 6, 2012; accepted June 15, 2012 ABSTRACT Mainstream asset pricing models are all inappropriate when they consistently insist on applying one single model to deal with a reality filled with different aspects of asset pricing. In addition, those models also treat the right environment variable too lightly hence can not rightly do the job of asset pricing. In this study, based on the portfolio theory and the principle of supply and demand, a more reasonable asset pricing system including five different models will be sug- gested to provide a necessary function of automatic price stabilization and to better serve our financial market. Keywords: Asset Pricing System; Environment Variable; Portfolio Theory; Automatic Price Stabilization 1. Introduction Since the mainstream asset pricing models can not restrain themselves from generating price bubbles in the financial market (Summers, 1985 [1]; Krugman, 2009 [2]; Colan- der, 2010 [3]), they certainly deserve our closer attention. In recent years, final conclusions regarding the major causes of recent financial crises still focus on market con- straints such as loose controls over financial derivatives or credit or monetary policies (Kindleberger, 2005 [4]; Askari et al., 2010 [5]; Bresser-Pereira, 2010 [6]). How- ever, if those mainstream asset pricing models can not be tamed by nature, then unleashing their constraints would only further exaggerate their power of sabotage. Equally important is that, when asset risk and return can only be endogenously determined and both are of much concern to investors, clearly it is impossible to apply just one single model to price assets, not to mention that there are still other considerations including the separation be- tween normative and positive analyses needing to be taken care of. In reality, we have no choice but to apply a system instead of one single model to manage the job of asset pricing. The main tasks of this study are therefore twofold. The first is to point out that mainstream asset pricing models are all inappropriate when they consistently insist on applying one single model to deal with a reality filled with different aspects of asset pricing. Accordingly, the second task of this study is to suggest a necessary asset pricing system which, by its own properties, can provide a safer ground to serve our financial market. 1.1. Literature Review Within the econometric approaches to asset pricing, strictly using only company variables emerged earlier, and the MM theorem proposed by Miller and Modigliani in 1961 was deemed to be the pioneering study in this field (Chen and Zhang, 2007 [7]). By contrast, Fama (1981) [8] started to lay the econometric foundation between asset price variation and macroeconomic variables including GDP, monetary supply and other related variables. Not surpris- ingly, combining both microeconomic and macroeconomic variables into one econometric model also attracted some attention (Lev and Thiagarajan, 1993 [9]; Swanson et al., 2003 [10]). However, when the set of explanatory variables with the best explanatory power could not be standard- ized for different individual assets, requiring more weights to be put on company variables, or asset portfolios, re- quiring more weights to be attached to macroeconomic variables, there was no end to the disputes that arose. As to those asset pricing models constructed with theoretical foundations, the first category featured the return aspect and was described by Graham and Dodd in 1934, later becoming the Dividend Discount Model pro- posed by Gordon and Shapiro in 1956 (Bettman et al., 2009 [11]). After that, two more kindred products of the Earnings Capitalization Model and the Residual Income Valuation Model had also been suggested (Kothari, 2001 [12]). The problem is, as long as all of them have to ap- ply time series corporate net incomes to forecast the net income in the next period, they can easily become econo- metric models (Collins and Kothari, 1989 [13]; Dechow et al., 1999 [14]). The second category can be classified as risk evaluation models, and the CAPM is clearly the most important representative. The scale of its family is still growing up to these days. While some consistently oppose it (Fama and French, 1996 [15]; 2004 [16]), some C opyright © 2012 SciRes. ME
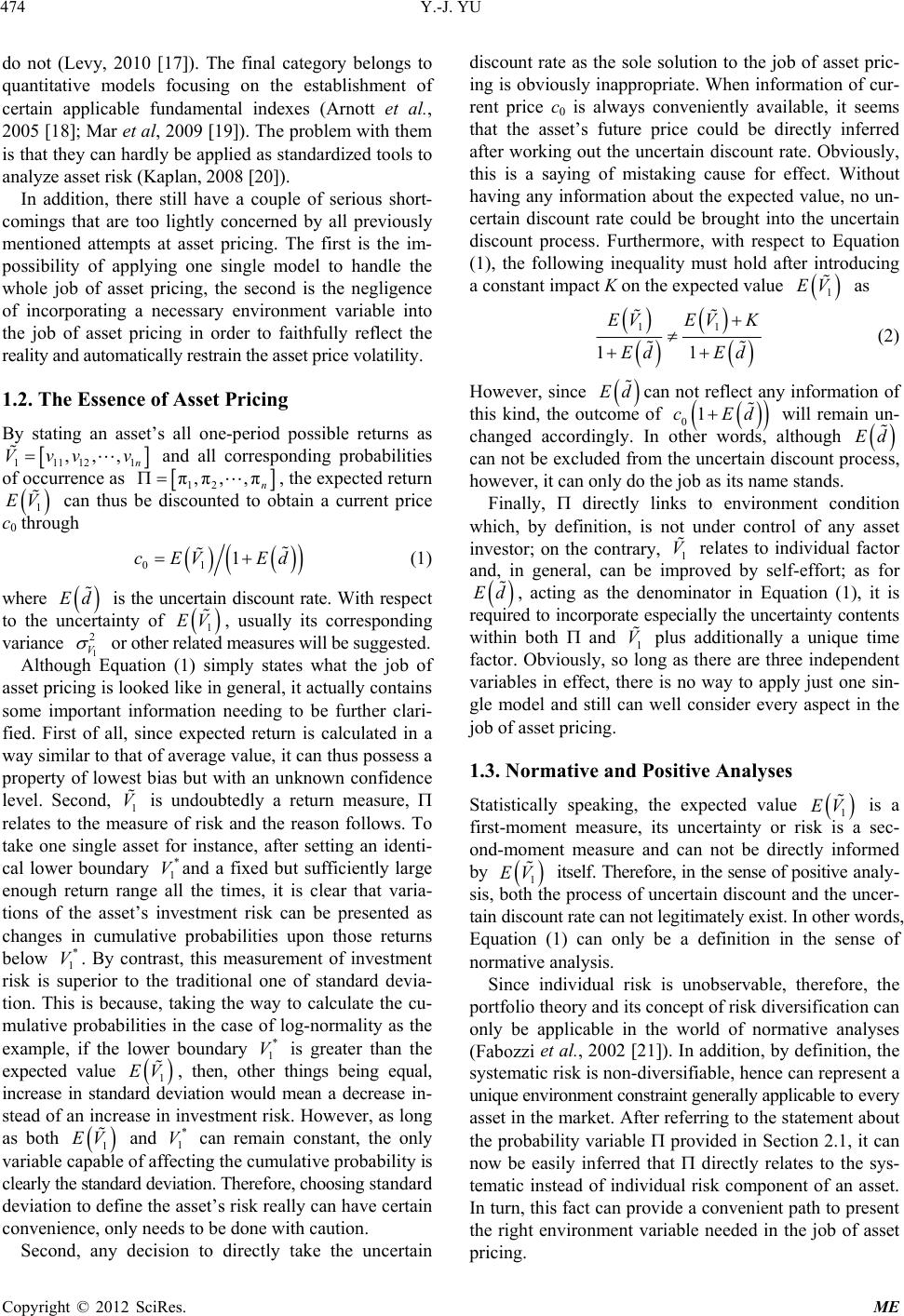 Y.-J. YU 474 do not (Levy, 2010 [17]). The final category belongs to quantitative models focusing on the establishment of certain applicable fundamental indexes (Arnott et al., 2005 [18]; Mar et al, 2009 [19]). The problem with them is that they can hardly be applied as standardized tools to analyze asset risk (Kaplan, 2008 [20]). In addition, there still have a couple of serious short- comings that are too lightly concerned by all previously mentioned attempts at asset pricing. The first is the im- possibility of applying one single model to handle the whole job of asset pricing, the second is the negligence of incorporating a necessary environment variable into the job of asset pricing in order to faithfully reflect the reality and automatically restrain the asset price volatility. 1.2. The Essence of Asset Pricing By stating an asset’s all one-period possible returns as 11 Vv 112 and all corresponding probabilities of occurrence as 1n ,,,v v 12 n, the expected return can thus be discounted to obtain a current price c0 through π,π,,π 1 EV 01 cE 1V Ed Ed EV (1) where is the uncertain discount rate. With respect to the uncertainty of 1, usually its corresponding variance 1 2 V or other related measures will be suggested. Although Equation (1) simply states what the job of asset pricing is looked like in general, it actually contains some important information needing to be further clari- fied. First of all, since expected return is calculated in a way similar to that of average value, it can thus possess a property of lowest bias but with an unknown confidence level. Second, 1 is undoubtedly a return measure, relates to the measure of risk and the reason follows. To take one single asset for instance, after setting an identi- cal lower boundary 1and a fixed but sufficiently large enough return range all the times, it is clear that varia- tions of the asset’s investment risk can be presented as changes in cumulative probabilities upon those returns below . By contrast, this measurement of investment risk is superior to the traditional one of standard devia- tion. This is because, taking the way to calculate the cu- mulative probabilities in the case of log-normality as the example, if the lower boundary 1 is greater than the expected value , then, other things being equal, increase in standard deviation would mean a decrease in- stead of an increase in investment risk. However, as long as both 1 and 1 V can remain constant, the only variable capable of affecting the cumulative probability is clearly the standard deviation. Therefore, choosing standard deviation to define the asset’s risk really can have certain convenience, only needs to be done with caution. V * V * V EV * 1 EV * 1 V 1 EV Second, any decision to directly take the uncertain discount rate as the sole solution to the job of asset pric- ing is obviously inappropriate. When information of cur- rent price c0 is always conveniently available, it seems that the asset’s future price could be directly inferred after working out the uncertain discount rate. Obviously, this is a saying of mistaking cause for effect. Without having any information about the expected value, no un- certain discount rate could be brought into the uncertain discount process. Furthermore, with respect to Equation (1), the following inequality must hold after introducing a constant impact K on the expected value as 11 11 EVEV K Ed Ed (2) However, since Ed 1cEd can not reflect any information of this kind, the outcome of 0 will remain un- changed accordingly. In other words, although Ed V can not be excluded from the uncertain discount process, however, it can only do the job as its name stands. Finally, directly links to environment condition which, by definition, is not under control of any asset investor; on the contrary, 1 relates to individual factor and, in general, can be improved by self-effort; as for Ed V , acting as the denominator in Equation (1), it is required to incorporate especially the uncertainty contents within both and 1 plus additionally a unique time factor. Obviously, so long as there are three independent variables in effect, there is no way to apply just one sin- gle model and still can well consider every aspect in the job of asset pricing. 1.3. Normative and Positive Analyses Statistically speaking, the expected value EV 1 is a first-moment measure, its uncertainty or risk is a sec- ond-moment measure and can not be directly informed by EV 1 itself. Therefore, in the sense of positive analy- sis, both the process of uncertain discount and the uncer- tain discount rate can not legitimately exist. In other words, Equation (1) can only be a definition in the sense of normative analysis. Since individual risk is unobservable, therefore, the portfolio theory and its concept of risk diversification can only be applicable in the world of normative analyses (Fabozzi et al., 2002 [21]). In addition, by definition, the systematic risk is non-diversifiable, hence can represent a unique environment constraint generally applicable to every asset in the market. After referring to the statement about the probability variable provided in Section 2.1, it can now be easily inferred that directly relates to the sys- tematic instead of individual risk component of an asset. In turn, this fact can provide a convenient path to present the right environment variable needed in the job of asset pricing. Copyright © 2012 SciRes. ME
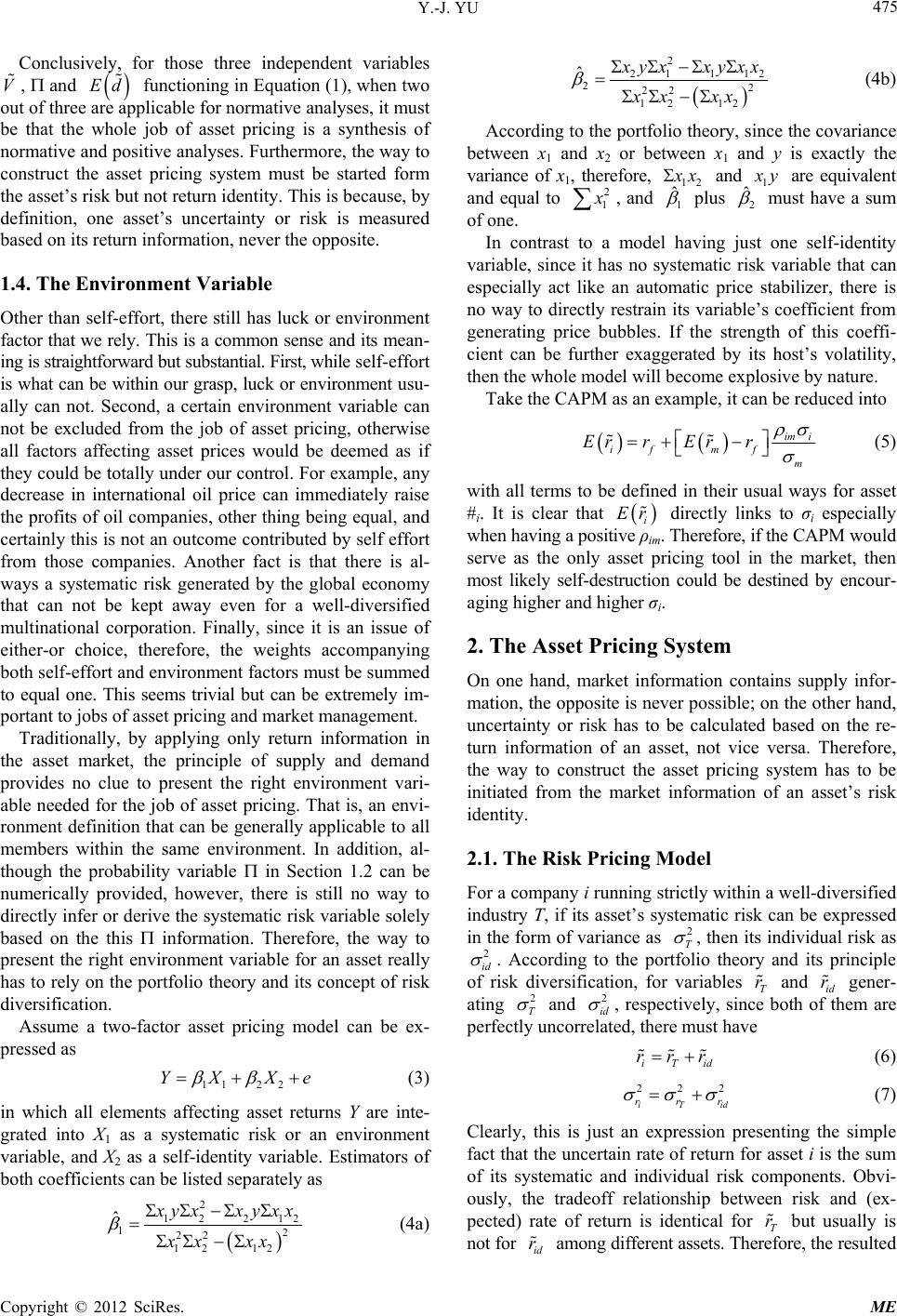 Y.-J. YU 475 Conclusively, for those three independent variables , and functioning in Equation (1), when two out of three are applicable for normative analyses, it must be that the whole job of asset pricing is a synthesis of normative and positive analyses. Furthermore, the way to construct the asset pricing system must be started form the asset’s risk but not return identity. This is because, by definition, one asset’s uncertainty or risk is measured based on its return information, never the opposite. V Ed 112 2 Xe 1.4. The Environment Variable Other than self-effort, there still has luck or environment factor that we rely. This is a common sense and its mean- ing is straightforward but substantial. First, while self-effort is what can be within our grasp, luck or environment usu- ally can not. Second, a certain environment variable can not be excluded from the job of asset pricing, otherwise all factors affecting asset prices would be deemed as if they could be totally under our control. For example, any decrease in international oil price can immediately raise the profits of oil companies, other thing being equal, and certainly this is not an outcome contributed by self effort from those companies. Another fact is that there is al- ways a systematic risk generated by the global economy that can not be kept away even for a well-diversified multinational corporation. Finally, since it is an issue of either-or choice, therefore, the weights accompanying both self-effort and environment factors must be summed to equal one. This seems trivial but can be extremely im- portant to jobs of asset pricing and market management. Traditionally, by applying only return information in the asset market, the principle of supply and demand provides no clue to present the right environment vari- able needed for the job of asset pricing. That is, an envi- ronment definition that can be generally applicable to all members within the same environment. In addition, al- though the probability variable in Section 1.2 can be numerically provided, however, there is still no way to directly infer or derive the systematic risk variable solely based on the this information. Therefore, the way to present the right environment variable for an asset really has to rely on the portfolio theory and its concept of risk diversification. Assume a two-factor asset pricing model can be ex- pressed as YX (3) in which all elements affecting asset returns Y are inte- grated into X1 as a systematic risk or an environment variable, and X2 as a self-identity variable. Estimators of both coefficients can be listed separately as 2 12 122 12 ˆ212 2 12 yx xx xyxx xx (4a) 2 21 112 22 22 12 12 ˆ yx xyxx xx xx 1 (4b) According to the portfolio theory, since the covariance between x1 and x2 or between x1 and y is exactly the variance of x1, therefore, 2 x and 1 y 2 1 are equivalent and equal to 1 ˆ2 ˆ , and plus must have a sum of one. In contrast to a model having just one self-identity variable, since it has no systematic risk variable that can especially act like an automatic price stabilizer, there is no way to directly restrain its variable’s coefficient from generating price bubbles. If the strength of this coeffi- cient can be further exaggerated by its host’s volatility, then the whole model will become explosive by nature. Take the CAPM as an example, it can be reduced into im i if mf m Er rErr (5) with all terms to be defined in their usual ways for asset #i. It is clear that Er 2 i directly links to σi especially when having a positive ρim. Therefore, if the CAPM would serve as the only asset pricing tool in the market, then most likely self-destruction could be destined by encour- aging higher and higher σi. 2. The Asset Pricing System On one hand, market information contains supply infor- mation, the opposite is never possible; on the other hand, uncertainty or risk has to be calculated based on the re- turn information of an asset, not vice versa. Therefore, the way to construct the asset pricing system has to be initiated from the market information of an asset’s risk identity. 2.1. The Risk Pricing Model For a company i running strictly within a well-diversified industry T, if its asset’s systematic risk can be expressed in the form of variance as T , then its individual risk as id 2 . According to the portfolio theory and its principle of risk diversification, for variables T and id r gener- ating T r 2 and id 2 , respectively, since both of them are perfectly uncorrelated, there must have iTid rrr 222 iTid rrr (6) r id r (7) Clearly, this is just an expression presenting the simple fact that the uncertain rate of return for asset i is the sum of its systematic and individual risk components. Obvi- ously, the tradeoff relationship between risk and (ex- pected) rate of return is identical for T but usually is not for among different assets. Therefore, the resulted Copyright © 2012 SciRes. ME
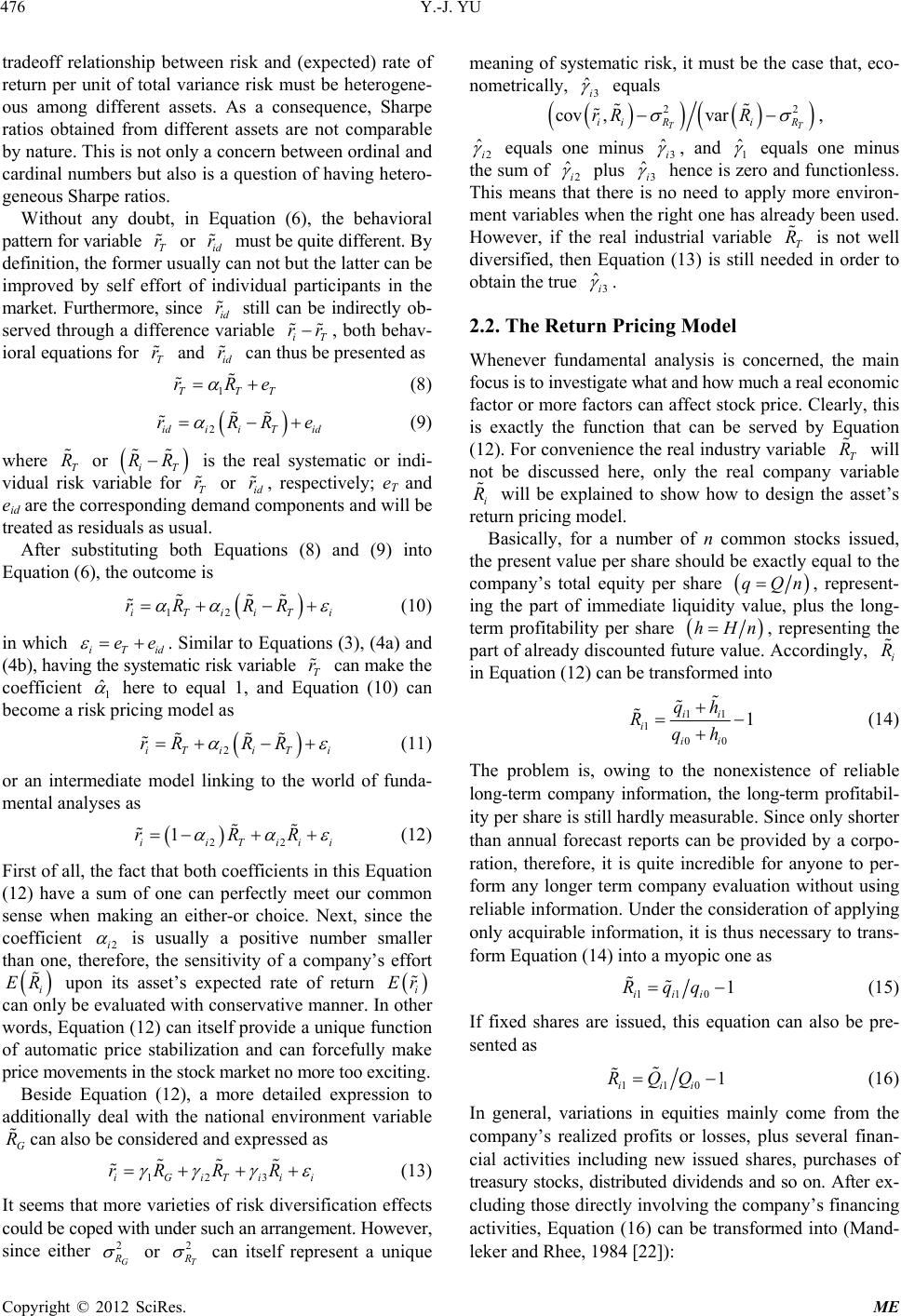 Y.-J. YU 476 tradeoff relationship between risk and (expected) rate of return per unit of total variance risk must be heterogene- ous among different assets. As a consequence, Sharpe ratios obtained from different assets are not comparable by nature. This is not only a concern between ordinal and cardinal numbers but also is a question of having hetero- geneous Sharpe ratios. Without any doubt, in Equation (6), the behavioral pattern for variable T or id r must be quite different. By definition, the former usually can not but the latter can be improved by self effort of individual participants in the market. Furthermore, since id still can be indirectly ob- served through a difference variable iT , both behav- ioral equations for and can thus be presented as r r rr T r 1TTT rRe i Tid rRRe RR r r id r (8) 2id i (9) where T or iT is the real systematic or indi- vidual risk variable for T or id, respectively; eT and eid are the corresponding demand components and will be treated as residuals as usual. R After substituting both Equations (8) and (9) into Equation (6), the outcome is iiT RR 12iT rR i ee (10) in which iTid r ˆ . Similar to Equations (3), (4a) and (4b), having the systematic risk variable T can make the coefficient 1 here to equal 1, and Equation (10) can become a risk pricing model as iT i RR 2iTi rR 22Tii R (11) or an intermediate model linking to the world of funda- mental analyses as 1 ii rR i (12) First of all, the fact that both coefficients in this Equation (12) have a sum of one can perfectly meet our common sense when making an either-or choice. Next, since the coefficient 2i is usually a positive number smaller than one, therefore, the sensitivity of a company’s effort i upon its asset’s expected rate of return ER Er G R 3iTii R R i can only be evaluated with conservative manner. In other words, Equation (12) can itself provide a unique function of automatic price stabilization and can forcefully make price movements in the stock market no more too exciting. Beside Equation (12), a more detailed expression to additionally deal with the national environment variable can also be considered and expressed as 12iG rR i 2 G (13) It seems that more varieties of risk diversification effects could be coped with under such an arrangement. However, since either or 2 T can itself represent a unique meaning of systematic risk, it must be the case that, eco- nometrically, 3 ˆi equals 22 cov ,var TT ii Ri R rR R ˆ , 2i equals one minus 3 ˆi , and 1 ˆ equals one minus the sum of 2i ˆ plus 3i ˆ hence is zero and functionless. This means that there is no need to apply more environ- ment variables when the right one has already been used. However, if the real industrial variable T is not well diversified, then Equation (13) is still needed in order to obtain the true R 3 ˆi . 2.2. The Return Pricing Model Whenever fundamental analysis is concerned, the main focus is to investigate what and how much a real economic factor or more factors can affect stock price. Clearly, this is exactly the function that can be served by Equation (12). For convenience the real industry variable T will not be discussed here, only the real company varia i R will be explained to show how to design the asset’s return pricing model. R ble Basically, for a number of n common stocks issued, the present value per share should be exactly equal to the company’s total equity per share qQn, represent- ing the part of immediate liquidity value, plus the long- term profitability per share hHn i R , representing the part of already discounted future value. Accordingly, in Equation (12) can be transformed into 11 1 00 1 ii i ii qh Rqh (14) The problem is, owing to the nonexistence of reliable long-term company information, the long-term profitabil- ity per share is still hardly measurable. Since only shorter than annual forecast reports can be provided by a corpo- ration, therefore, it is quite incredible for anyone to per- form any longer term company evaluation without using reliable information. Under the consideration of applying only acquirable information, it is thus necessary to trans- form Equation (14) into a myopic one as 110 1 iii Rqq (15) If fixed shares are issued, this equation can also be pre- sented as 110 1 iii RQQ (16) In general, variations in equities mainly come from the company’s realized profits or losses, plus several finan- cial activities including new issued shares, purchases of treasury stocks, distributed dividends and so on. After ex- cluding those directly involving the company’s financing activities, Equation (16) can be transformed into (Mand- leker and Rhee, 1984 [22]): Copyright © 2012 SciRes. ME
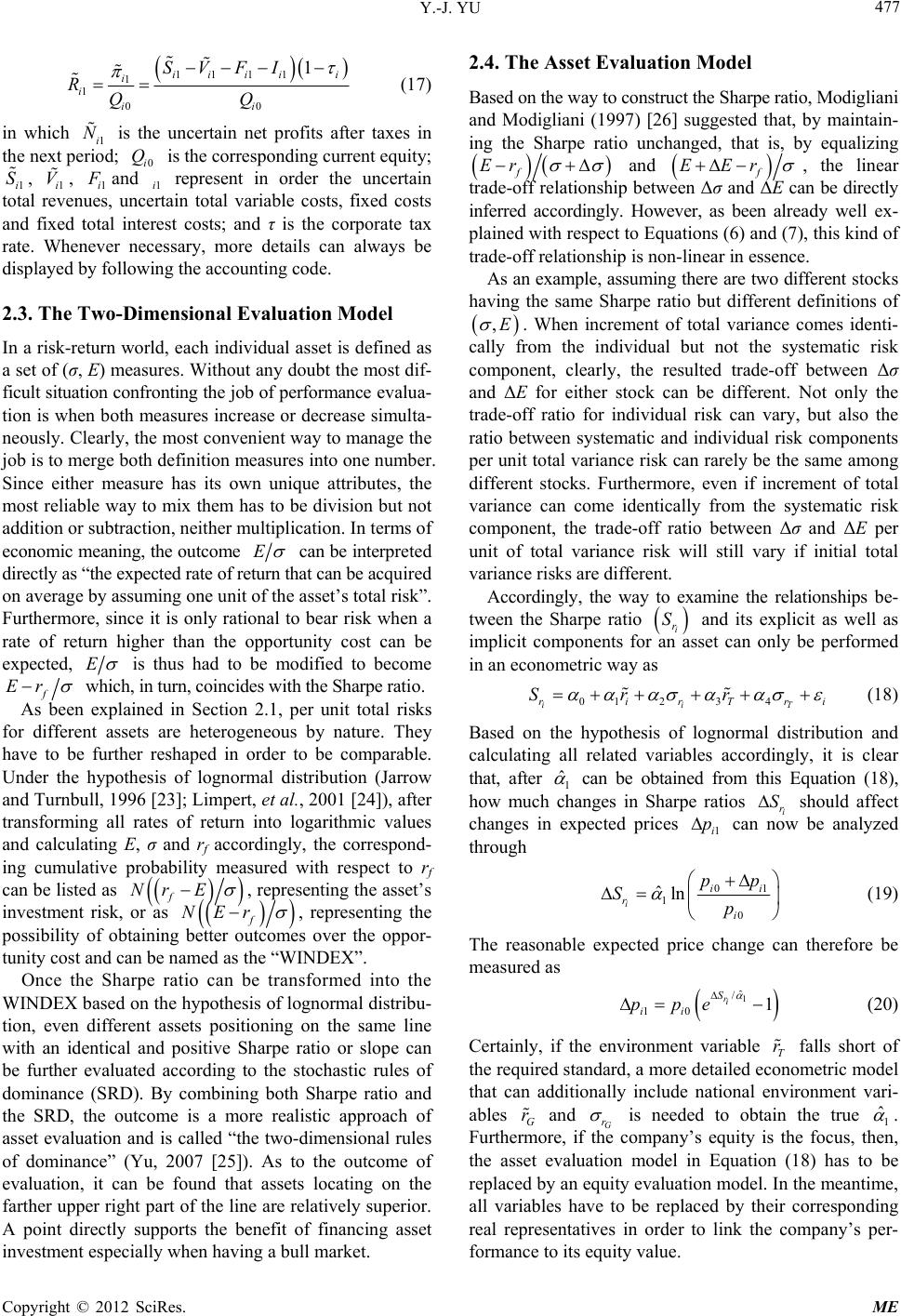 Y.-J. YU 477 11 1 1 ii i i ii SV F RQQ 11 00 1 iii I N 0 Q S (17) in which 1i is the uncertain net profits after taxes in the next period; i is the corresponding current equity; 1i, 1i, 1i V and 1i represent in order the uncertain total revenues, uncertain total variable costs, fixed costs and fixed total interest costs; and τ is the corporate tax rate. Whenever necessary, more details can always be displayed by following the accounting code. 2.3. The Two-Dimensional Evaluation Model In a risk-return world, each individual asset is defined as a set of (σ, E) measures. Without any doubt the most dif- ficult situation confronting the job of performance evalua- tion is when both measures increase or decrease simulta- neously. Clearly, the most convenient way to manage the job is to merge both definition measures into one number. Since either measure has its own unique attributes, the most reliable way to mix them has to be division but not addition or subtraction, neither multiplication. In terms of economic meaning, the outcome E can be interpreted directly as “the expected rate of return that can be acquired on average by assuming one unit of the asset’s total risk”. Furthermore, since it is only rational to bear risk when a rate of return higher than the opportunity cost can be expected, E is thus had to be modified to become f Er which, in turn, coincides with the Sharpe ratio. As been explained in Section 2.1, per unit total risks for different assets are heterogeneous by nature. They have to be further reshaped in order to be comparable. Under the hypothesis of lognormal distribution (Jarrow and Turnbull, 1996 [23]; Limpert, et al., 2001 [24]), after transforming all rates of return into logarithmic values and calculating E, σ and rf accordingly, the correspond- ing cumulative probability measured with respect to rf can be listed as Nr E f , representing the asset’s investment risk, or as NEr f , representing the possibility of obtaining better outcomes over the oppor- tunity cost and can be named as the “WINDEX”. Once the Sharpe ratio can be transformed into the WINDEX based on the hypothesis of lognormal distribu- tion, even different assets positioning on the same line with an identical and positive Sharpe ratio or slope can be further evaluated according to the stochastic rules of dominance (SRD). By combining both Sharpe ratio and the SRD, the outcome is a more realistic approach of asset evaluation and is called “the two-dimensional rules of dominance” (Yu, 2007 [25]). As to the outcome of evaluation, it can be found that assets locating on the farther upper right part of the line are relatively superior. A point directly supports the benefit of financing asset investment especially when having a bull market. 2.4. The Asset Evaluation Model Based on the way to construct the Sharpe ratio, Modigliani and Modigliani (1997) [26] suggested that, by maintain- ing the Sharpe ratio unchanged, that is, by equalizing f f Er EEr and , the linear trade-off relationship between Δσ and ΔE can be directly inferred accordingly. However, as been already well ex- plained with respect to Equations (6) and (7), this kind of trade-off relationship is non-linear in essence. As an example, assuming there are two different stocks having the same Sharpe ratio but different definitions of ,E . When increment of total variance comes identi- cally from the individual but not the systematic risk component, clearly, the resulted trade-off between Δσ and ΔE for either stock can be different. Not only the trade-off ratio for individual risk can vary, but also the ratio between systematic and individual risk components per unit total variance risk can rarely be the same among different stocks. Furthermore, even if increment of total variance can come identically from the systematic risk component, the trade-off ratio between Δσ and ΔE per unit of total variance risk will still vary if initial total variance risks are different. Accordingly, the way to examine the relationships be- tween the Sharpe ratio S 01 234 iiT rirTri Sr r i r and its explicit as well as implicit components for an asset can only be performed in an econometric way as ˆ (18) Based on the hypothesis of lognormal distribution and calculating all related variables accordingly, it is clear that, after 1 can be obtained from this Equation (18), how much changes in Sharpe ratios i r should affect changes in expected prices can now be analyzed through S 1i p 01 1 0 ˆln i ii r i pp Sp 1 ˆ / 10 1 r i S ii ppe (19) The reasonable expected price change can therefore be measured as r r (20) Certainly, if the environment variable T falls short of the required standard, a more detailed econometric model that can additionally include national environment vari- ables G and G r is needed to obtain the true 1 ˆ . Furthermore, if the company’s equity is the focus, then, the asset evaluation model in Equation (18) has to be replaced by an equity evaluation model. In the meantime, all variables have to be replaced by their corresponding real representatives in order to link the company’s per- formance to its equity value. Copyright © 2012 SciRes. ME
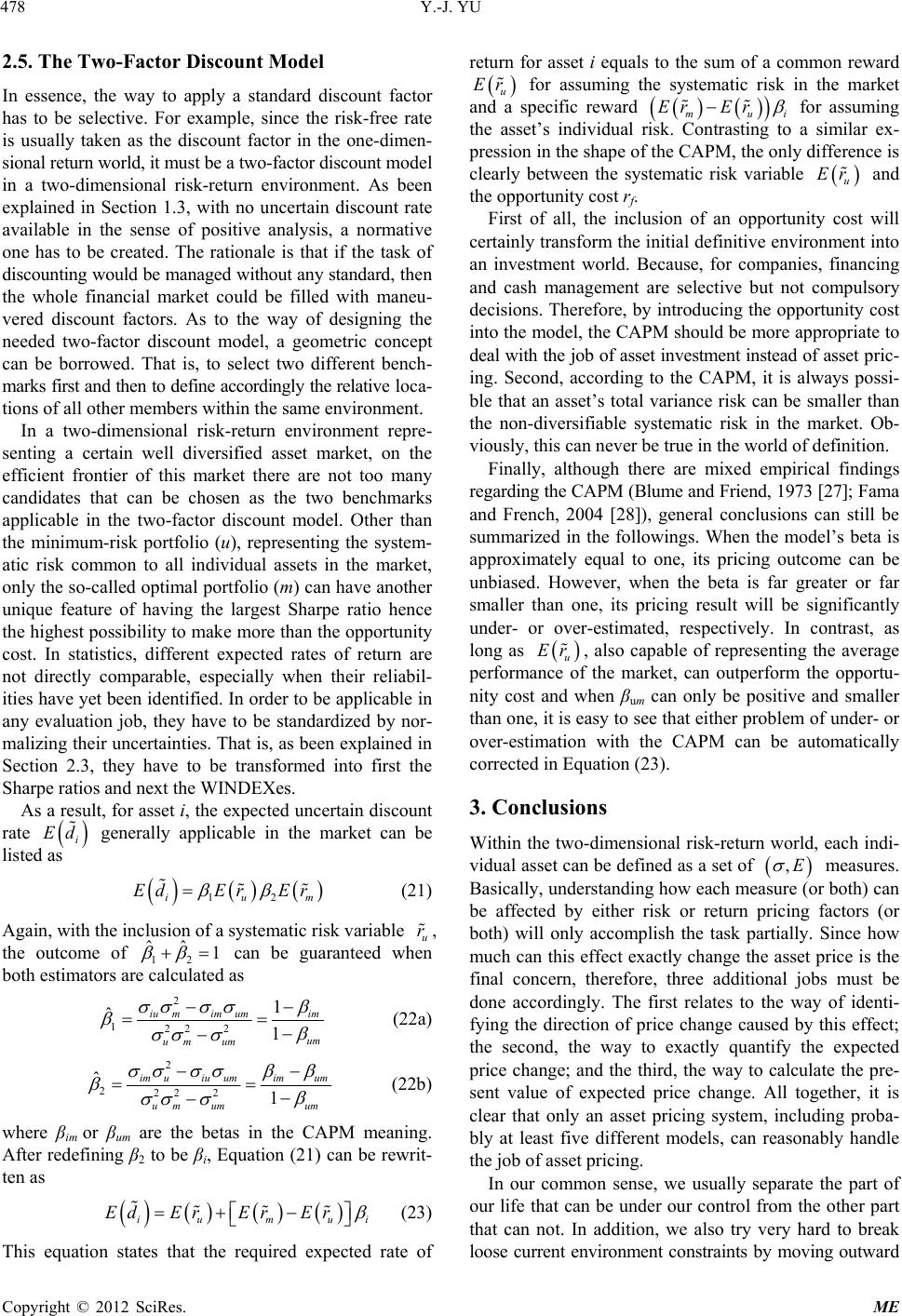 Y.-J. YU 478 2.5. The Two-Factor Discount Model In essence, the way to apply a standard discount factor has to be selective. For example, since the risk-free rate is usually taken as the discount factor in the one-dimen- sional return world, it must be a two-factor discount model in a two-dimensional risk-return environment. As been explained in Section 1.3, with no uncertain discount rate available in the sense of positive analysis, a normative one has to be created. The rationale is that if the task of discounting would be managed without any standard, then the whole financial market could be filled with maneu- vered discount factors. As to the way of designing the needed two-factor discount model, a geometric concept can be borrowed. That is, to select two different bench- marks first and then to define accordingly the relative loca- tions of all other members within the same environment. In a two-dimensional risk-return environment repre- senting a certain well diversified asset market, on the efficient frontier of this market there are not too many candidates that can be chosen as the two benchmarks applicable in the two-factor discount model. Other than the minimum-risk portfolio (u), representing the system- atic risk common to all individual assets in the market, only the so-called optimal portfolio (m) can have another unique feature of having the largest Sharpe ratio hence the highest possibility to make more than the opportunity cost. In statistics, different expected rates of return are not directly comparable, especially when their reliabil- ities have yet been identified. In order to be applicable in any evaluation job, they have to be standardized by nor- malizing their uncertainties. That is, as been explained in Section 2.3, they have to be transformed into first the Sharpe ratios and next the WINDEXes. As a result, for asset i, the expected uncertain discount rate generally applicable in the market can be listed as i Ed 12ium Er Er th ˆ 1 Ed (21) Again, with the inclusion of a systematic risk variable u r , e outcome of 12 ˆ can be guaranteed when both estimators are calculated as 2 122 ˆiu mim um 2 1 1 um im um um (22a) 2 222 2 ˆim uiu um um um1 im um um mui Er (22b) where βim or βum are the betas in the CAPM meaning. After redefining β2 to be βi, Equation (21) can be rewrit- ten as iu Ed ErEr (23) This equation states that the required expected rate of return for asset i equals to the sum of a common reward Er u for assuming the systematic risk in the market and a specific reward mui Er Er for assuming the asset’s individual risk. Contrasting to a similar ex- pression in the shape of the CAPM, the only difference is clearly between the systematic risk variable u Er and the opportunity cost rf. First of all, the inclusion of an opportunity cost will certainly transform the initial definitive environment into an investment world. Because, for companies, financing and cash management are selective but not compulsory decisions. Therefore, by introducing the opportunity cost into the model, the CAPM should be more appropriate to deal with the job of asset investment instead of asset pric- ing. Second, according to the CAPM, it is always possi- ble that an asset’s total variance risk can be smaller than the non-diversifiable systematic risk in the market. Ob- viously, this can never be true in the world of definition. Finally, although there are mixed empirical findings regarding the CAPM (Blume and Friend, 1973 [27]; Fama and French, 2004 [28]), general conclusions can still be summarized in the followings. When the model’s beta is approximately equal to one, its pricing outcome can be unbiased. However, when the beta is far greater or far smaller than one, its pricing result will be significantly under- or over-estimated, respectively. In contrast, as long as Er ,E u, also capable of representing the average performance of the market, can outperform the opportu- nity cost and when βum can only be positive and smaller than one, it is easy to see that either problem of under- or over-estimation with the CAPM can be automatically corrected in Equation (23). 3. Conclusions Within the two-dimensional risk-return world, each indi- vidual asset can be defined as a set of measures. Basically, understanding how each measure (or both) can be affected by either risk or return pricing factors (or both) will only accomplish the task partially. Since how much can this effect exactly change the asset price is the final concern, therefore, three additional jobs must be done accordingly. The first relates to the way of identi- fying the direction of price change caused by this effect; the second, the way to exactly quantify the expected price change; and the third, the way to calculate the pre- sent value of expected price change. All together, it is clear that only an asset pricing system, including proba- bly at least five different models, can reasonably handle the job of asset pricing. In our common sense, we usually separate the part of our life that can be under our control from the other part that can not. In addition, we also try very hard to break loose current environment constraints by moving outward Copyright © 2012 SciRes. ME
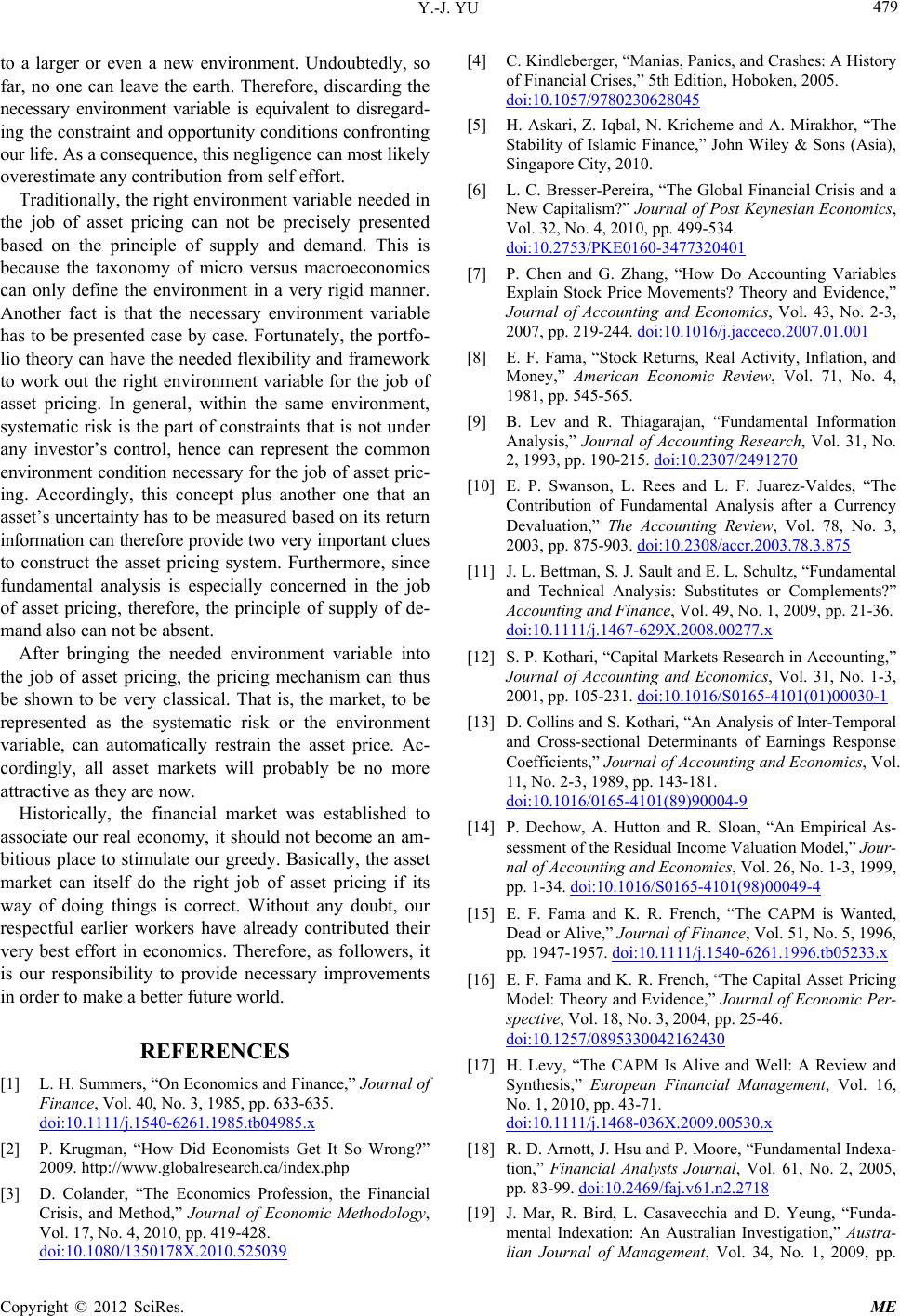 Y.-J. YU 479 to a larger or even a new environment. Undoubtedly, so far, no one can leave the earth. Therefore, discarding the necessary environment variable is equivalent to disregard- ing the constraint and opportunity conditions confronting our life. As a consequence, this negligence can most likely overestimate any contribution from self effort. Traditionally, the right environment variable needed in the job of asset pricing can not be precisely presented based on the principle of supply and demand. This is because the taxonomy of micro versus macroeconomics can only define the environment in a very rigid manner. Another fact is that the necessary environment variable has to be presented case by case. Fortunately, the portfo- lio theory can have the needed flexibility and framework to work out the right environment variable for the job of asset pricing. In general, within the same environment, systematic risk is the part of constraints that is not under any investor’s control, hence can represent the common environment condition necessary for the job of asset pric- ing. Accordingly, this concept plus another one that an asset’s uncertainty has to be measured based on its return information can therefore provide two very important clues to construct the asset pricing system. Furthermore, since fundamental analysis is especially concerned in the job of asset pricing, therefore, the principle of supply of de- mand also can not be absent. After bringing the needed environment variable into the job of asset pricing, the pricing mechanism can thus be shown to be very classical. That is, the market, to be represented as the systematic risk or the environment variable, can automatically restrain the asset price. Ac- cordingly, all asset markets will probably be no more attractive as they are now. Historically, the financial market was established to associate our real economy, it should not become an am- bitious place to stimulate our greedy. Basically, the asset market can itself do the right job of asset pricing if its way of doing things is correct. Without any doubt, our respectful earlier workers have already contributed their very best effort in economics. Therefore, as followers, it is our responsibility to provide necessary improvements in order to make a better future world. REFERENCES [1] L. H. Summers, “On Economics and Finance,” Journal of Finance, Vol. 40, No. 3, 1985, pp. 633-635. doi:10.1111/j.1540-6261.1985.tb04985.x [2] P. Krugman, “How Did Economists Get It So Wrong?” 2009. http://www.globalresearch.ca/index.php [3] D. Colander, “The Economics Profession, the Financial Crisis, and Method,” Journal of Economic Methodology, Vol. 17, No. 4, 2010, pp. 419-428. doi:10.1080/1350178X.2010.525039 [4] C. Kindleberger, “Manias, Panics, and Crashes: A History of Financial Crises,” 5th Edition, Hoboken, 2005. doi:10.1057/9780230628045 [5] H. Askari, Z. Iqbal, N. Kricheme and A. Mirakhor, “The Stability of Islamic Finance,” John Wiley & Sons (Asia), Singapore City, 2010. [6] L. C. Bresser-Pereira, “The Global Financial Crisis and a New Capitalism?” Journal of Post Keynesian Economics, Vol. 32, No. 4, 2010, pp. 499-534. doi:10.2753/PKE0160-3477320401 [7] P. Chen and G. Zhang, “How Do Accounting Variables Explain Stock Price Movements? Theory and Evidence,” Journal of Accounting and Economics, Vol. 43, No. 2-3, 2007, pp. 219-244. doi:10.1016/j.jacceco.2007.01.001 [8] E. F. Fama, “Stock Returns, Real Activity, Inflation, and Money,” American Economic Review, Vol. 71, No. 4, 1981, pp. 545-565. [9] B. Lev and R. Thiagarajan, “Fundamental Information Analysis,” Journal of Accounting Research, Vol. 31, No. 2, 1993, pp. 190-215. doi:10.2307/2491270 [10] E. P. Swanson, L. Rees and L. F. Juarez-Valdes, “The Contribution of Fundamental Analysis after a Currency Devaluation,” The Accounting Review, Vol. 78, No. 3, 2003, pp. 875-903. doi:10.2308/accr.2003.78.3.875 [11] J. L. Bettman, S. J. Sault and E. L. Schultz, “Fundamental and Technical Analysis: Substitutes or Complements?” Accounting and Finance, Vol. 49, No. 1, 2009, pp. 21-36. doi:10.1111/j.1467-629X.2008.00277.x [12] S. P. Kothari, “Capital Markets Research in Accounting,” Journal of Accounting and Economics, Vol. 31, No. 1-3, 2001, pp. 105-231. doi:10.1016/S0165-4101(01)00030-1 [13] D. Collins and S. Kothari, “An Analysis of Inter-Temporal and Cross-sectional Determinants of Earnings Response Coefficients,” Journal of Accounting and Economics, Vol. 11, No. 2-3, 1989, pp. 143-181. doi:10.1016/0165-4101(89)90004-9 [14] P. Dechow, A. Hutton and R. Sloan, “An Empirical As- sessment of the Residual Income Valuation Model,” Jour- nal of Accounting and Economics, Vol. 26, No. 1-3, 1999, pp. 1-34. doi:10.1016/S0165-4101(98)00049-4 [15] E. F. Fama and K. R. French, “The CAPM is Wanted, Dead or Alive,” Journal of Finance, Vol. 51, No. 5, 1996, pp. 1947-1957. doi:10.1111/j.1540-6261.1996.tb05233.x [16] E. F. Fama and K. R. French, “The Capital Asset Pricing Model: Theory and Evidence,” Journal of Economic Per- spective, Vol. 18, No. 3, 2004, pp. 25-46. doi:10.1257/0895330042162430 [17] H. Levy, “The CAPM Is Alive and Well: A Review and Synthesis,” European Financial Management, Vol. 16, No. 1, 2010, pp. 43-71. doi:10.1111/j.1468-036X.2009.00530.x [18] R. D. Arnott, J. Hsu and P. Moore, “Fundamental Indexa- tion,” Financial Analysts Journal, Vol. 61, No. 2, 2005, pp. 83-99. doi:10.2469/faj.v61.n2.2718 [19] J. Mar, R. Bird, L. Casavecchia and D. Yeung, “Funda- mental Indexation: An Australian Investigation,” Austra- lian Journal of Management, Vol. 34, No. 1, 2009, pp. Copyright © 2012 SciRes. ME
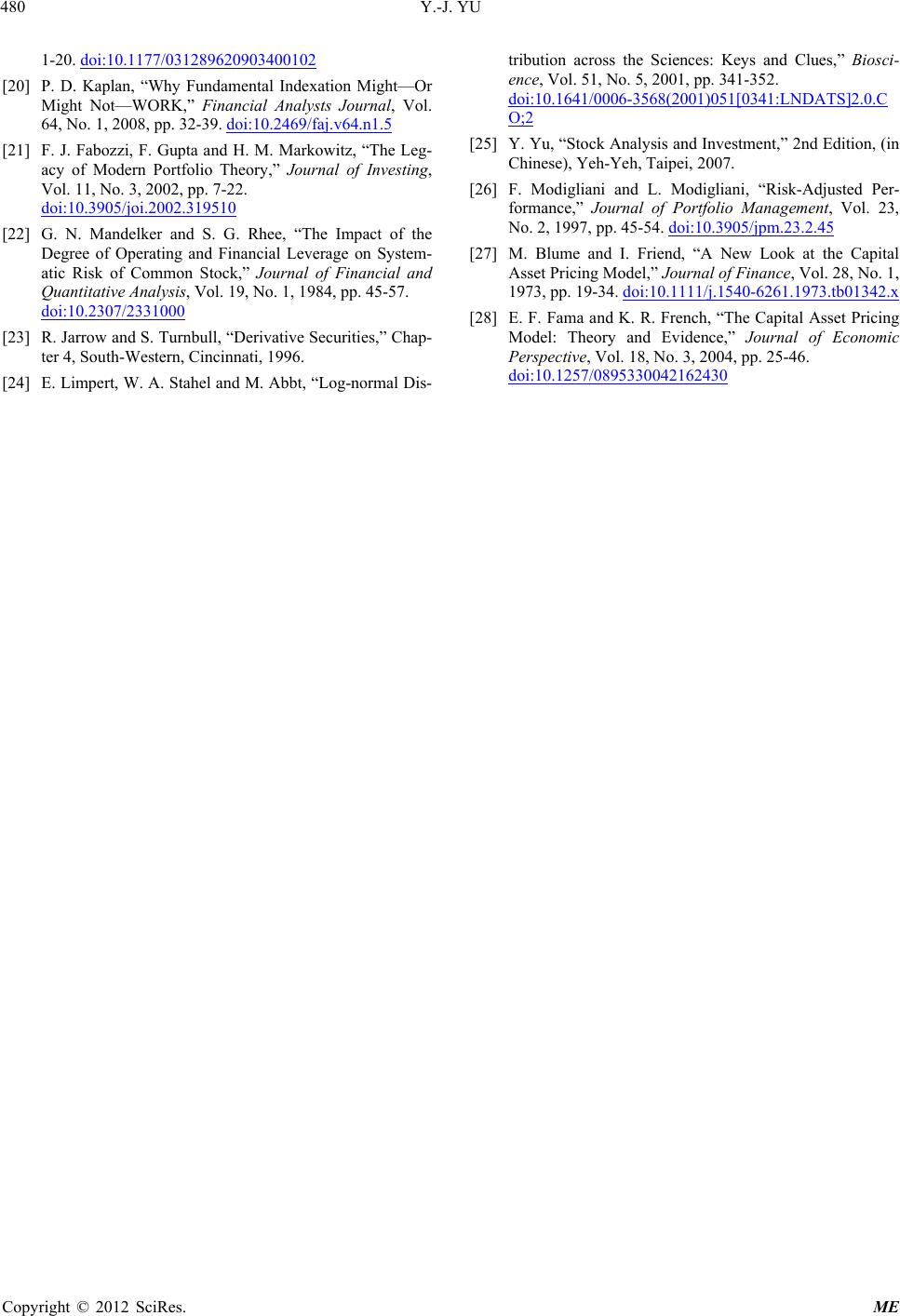 Y.-J. YU Copyright © 2012 SciRes. ME 480 1-20. doi:10.1177/031289620903400102 [20] P. D. Kaplan, “Why Fundamental Indexation Might—Or Might Not—WORK,” Financial Analysts Journal, Vol. 64, No. 1, 2008, pp. 32-39. doi:10.2469/faj.v64.n1.5 [21] F. J. Fabozzi, F. Gupta and H. M. Markowitz, “The Leg- acy of Modern Portfolio Theory,” Journal of Investing, Vol. 11, No. 3, 2002, pp. 7-22. doi:10.3905/joi.2002.319510 [22] G. N. Mandelker and S. G. Rhee, “The Impact of the Degree of Operating and Financial Leverage on System- atic Risk of Common Stock,” Journal of Financial and Quantitative Analysis, Vol. 19, No. 1, 1984, pp. 45-57. doi:10.2307/2331000 [23] R. Jarrow and S. Turnbull, “Derivative Securities,” Chap- ter 4, South-Western, Cincinnati, 1996. [24] E. Limpert, W. A. Stahel and M. Abbt, “Log-normal Dis- tribution across the Sciences: Keys and Clues,” Biosci- ence, Vol. 51, No. 5, 2001, pp. 341-352. doi:10.1641/0006-3568(2001)051[0341:LNDATS]2.0.C O;2 [25] Y. Yu, “Stock Analysis and Investment,” 2nd Edition, (in Chinese), Yeh-Yeh, Taipei, 2007. [26] F. Modigliani and L. Modigliani, “Risk-Adjusted Per- formance,” Journal of Portfolio Management, Vol. 23, No. 2, 1997, pp. 45-54. doi:10.3905/jpm.23.2.45 [27] M. Blume and I. Friend, “A New Look at the Capital Asset Pricing Model,” Journal of Finance, Vol. 28, No. 1, 1973, pp. 19-34. doi:10.1111/j.1540-6261.1973.tb01342.x [28] E. F. Fama and K. R. French, “The Capital Asset Pricing Model: Theory and Evidence,” Journal of Economic Perspective, Vol. 18, No. 3, 2004, pp. 25-46. doi:10.1257/0895330042162430
|