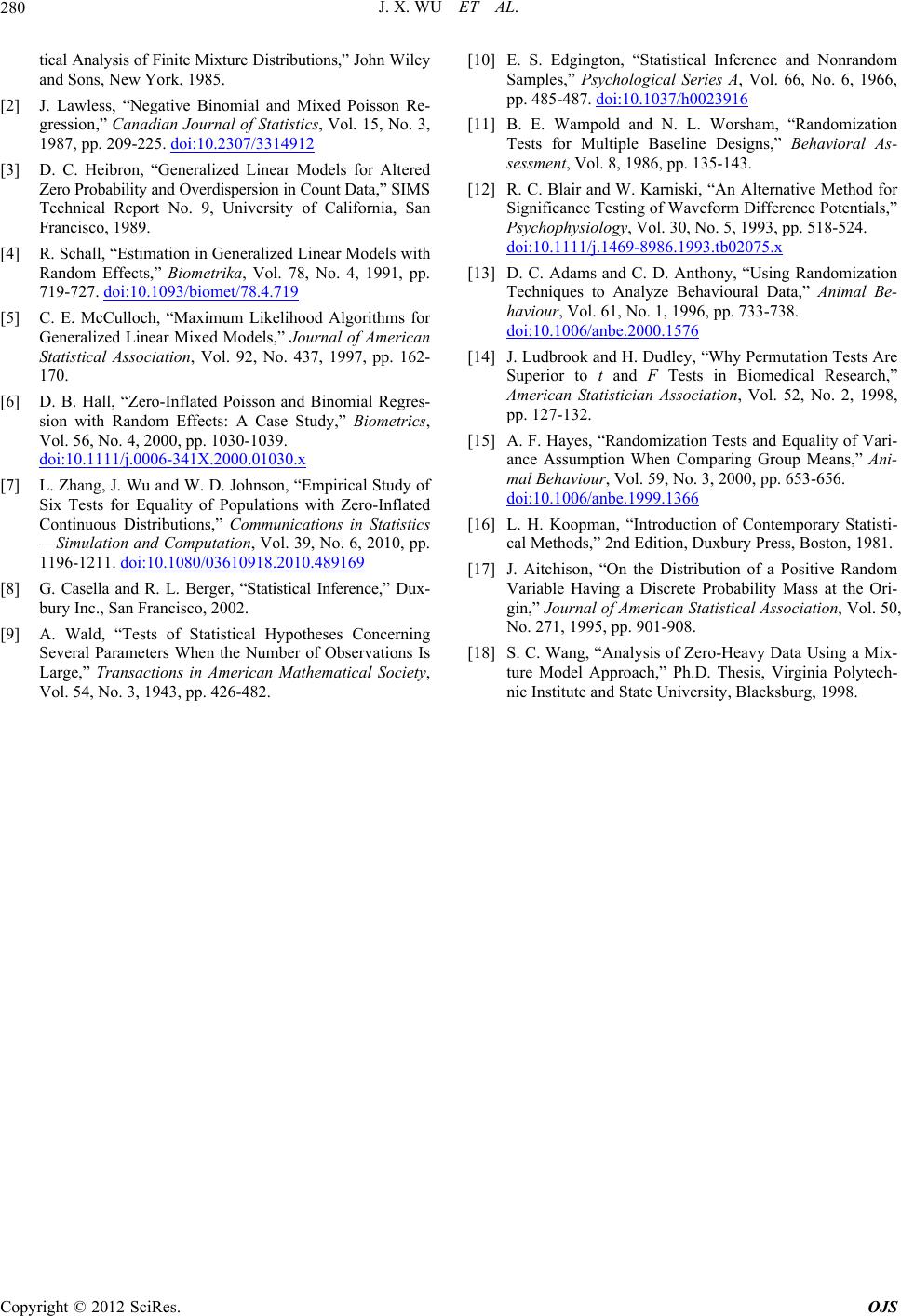
J. X. WU ET AL.
Copyright © 2012 SciRes. OJS
280
tical Analysis of Finite Mixture Distributions,” John Wiley
and Sons, New York, 1985.
[2] J. Lawless, “Negative Binomial and Mixed Poisson Re-
gression,” Canadian Journal of Statistics, Vol. 15, No. 3,
1987, pp. 209-225. doi:10.2307/3314912
[3] D. C. Heibron, “Generalized Linear Models for Altered
Zero Probability and Overdispersion in Count Data,” SIMS
Technical Report No. 9, University of California, San
Francisco, 1989.
[4] R. Schall, “Estimation in Generalized Linear Models with
Random Effects,” Biometrika, Vol. 78, No. 4, 1991, pp.
719-727. doi:10.1093/biomet/78.4.719
[5] C. E. McCulloch, “Maximum Likelihood Algorithms for
Generalized Linear Mixed Models,” Journal of American
Statistical Association, Vol. 92, No. 437, 1997, pp. 162-
170.
[6] D. B. Hall, “Zero-Inflated Poisson and Binomial Regres-
sion with Random Effects: A Case Study,” Biometrics,
Vol. 56, No. 4, 2000, pp. 1030-1039.
doi:10.1111/j.0006-341X.2000.01030.x
[7] L. Zhang, J. Wu and W. D. Johnson, “Empirical Study of
Six Tests for Equality of Populations with Zero-Inflated
Continuous Distributions,” Communications in Statistics
—Simulation and Computation, Vol. 39, No. 6, 2010, pp.
1196-1211. doi:10.1080/03610918.2010.489169
[8] G. Casella and R. L. Berger, “Statistical Inference,” Dux-
bury Inc., San Francisco, 2002.
[9] A. Wald, “Tests of Statistical Hypotheses Concerning
Several Parameters When the Number of Observations Is
Large,” Transactions in American Mathematical Society,
Vol. 54, No. 3, 1943, pp. 426-482.
[10] E. S. Edgington, “Statistical Inference and Nonrandom
Samples,” Psychological Series A, Vol. 66, No. 6, 1966,
pp. 485-487. doi:10.1037/h0023916
[11] B. E. Wampold and N. L. Worsham, “Randomization
Tests for Multiple Baseline Designs,” Behavioral As-
sessment, Vol. 8, 1986, pp. 135-143.
[12] R. C. Blair and W. Karniski, “An Alternative Method for
Significance Testing of Waveform Difference Potentials,”
Psychophysiology, Vol. 30, No. 5, 1993, pp. 518-524.
doi:10.1111/j.1469-8986.1993.tb02075.x
[13] D. C. Adams and C. D. Anthony, “Using Randomization
Techniques to Analyze Behavioural Data,” Animal Be-
haviour, Vol. 61, No. 1, 1996, pp. 733-738.
doi:10.1006/anbe.2000.1576
[14] J. Ludbrook and H. Dudley, “Why Permutation Tests Are
Superior to t and F Tests in Biomedical Research,”
American Statistician Association, Vol. 52, No. 2, 1998,
pp. 127-132.
[15] A. F. Hayes, “Randomization Tests and Equality of Vari-
ance Assumption When Comparing Group Means,” Ani-
mal Behaviour, Vol. 59, No. 3, 2000, pp. 653-656.
doi:10.1006/anbe.1999.1366
[16] L. H. Koopman, “Introduction of Contemporary Statisti-
cal Methods,” 2nd Edition, Duxbury Press, Boston, 1981.
[17] J. Aitchison, “On the Distribution of a Positive Random
Variable Having a Discrete Probability Mass at the Ori-
gin,” Journal of American Statistical Association, Vol. 50,
No. 271, 1995, pp. 901-908.
[18] S. C. Wang, “Analysis of Zero-Heavy Data Using a Mix-
ture Model Approach,” Ph.D. Thesis, Virginia Polytech-
nic Institute and State University, Blacksburg, 1998.