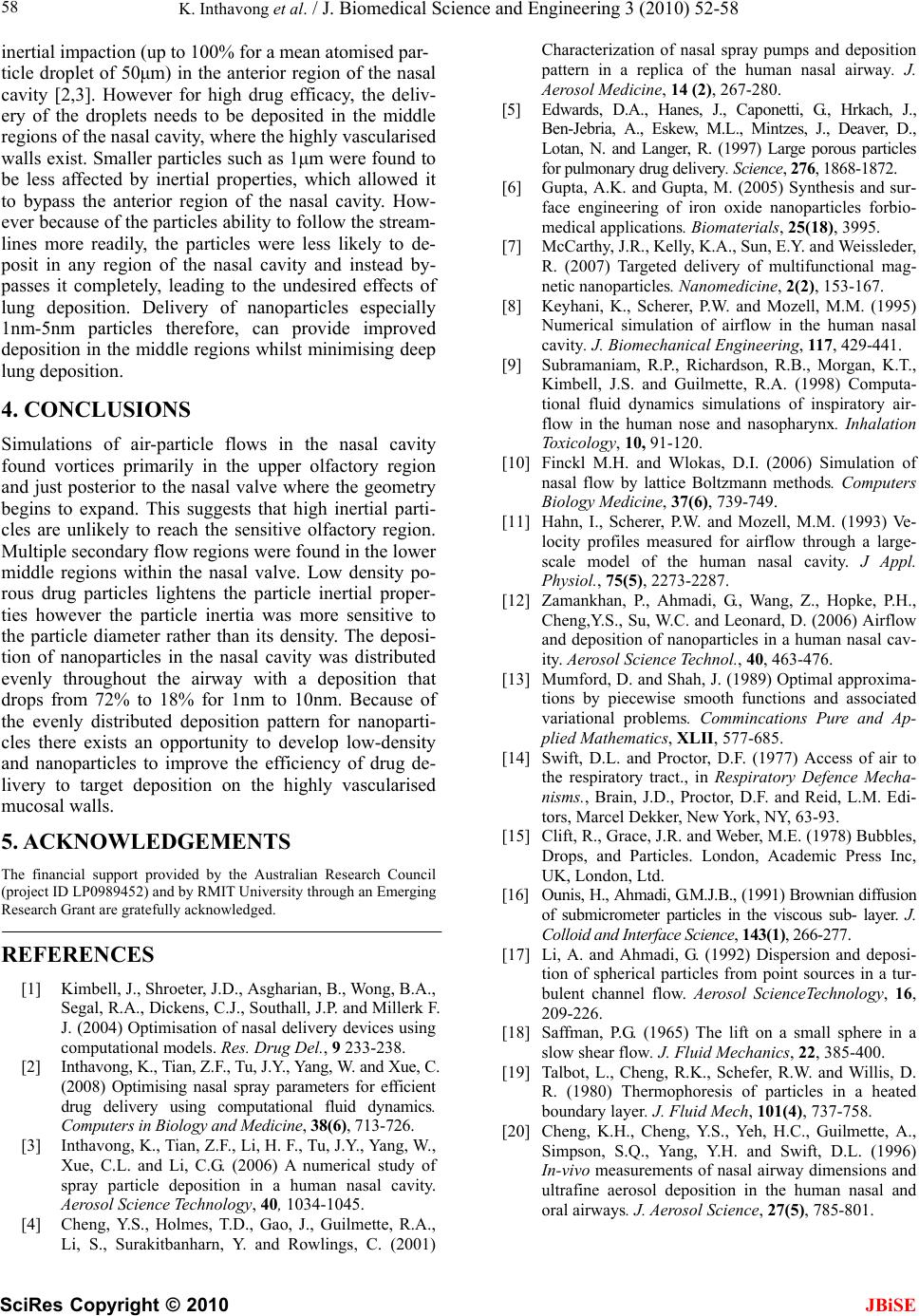
K. Inthavong et al. / J. Biomedical Science and Engineering 3 (2010) 52-58
SciRes Copyright © 2010 JBiSE
58
inertial impaction (up to 100% for a mean atomised par-
ticle droplet of 50μm) in the anterior region of the nasal
cavity [2,3]. However for high drug efficacy, the deliv-
ery of the droplets needs to be deposited in the middle
regions of the nasal cavity, where the highly vascularised
walls exist. Smaller particles such as 1μm were found to
be less affected by inertial properties, which allowed it
to bypass the anterior region of the nasal cavity. How-
ever because of the particles ability to follow the stream-
lines more readily, the particles were less likely to de-
posit in any region of the nasal cavity and instead by-
passes it completely, leading to the undesired effects of
lung deposition. Delivery of nanoparticles especially
1nm-5nm particles therefore, can provide improved
deposition in the middle regions whilst minimising deep
lung deposition.
4. CONCLUSIONS
Simulations of air-particle flows in the nasal cavity
found vortices primarily in the upper olfactory region
and just posterior to the nasal valve where the geometry
begins to expand. This suggests that high inertial parti-
cles are unlikely to reach the sensitive olfactory region.
Multiple secondary flow regions were found in the lower
middle regions within the nasal valve. Low density po-
rous drug particles lightens the particle inertial proper-
ties however the particle inertia was more sensitive to
the particle diameter rather than its density. The deposi-
tion of nanoparticles in the nasal cavity was distributed
evenly throughout the airway with a deposition that
drops from 72% to 18% for 1nm to 10nm. Because of
the evenly distributed deposition pattern for nanoparti-
cles there exists an opportunity to develop low-density
and nanoparticles to improve the efficiency of drug de-
livery to target deposition on the highly vascularised
mucosal walls.
5. ACKNOWLEDGEMENTS
The financial support provided by the Australian Research Council
(project ID LP0989452) and by RMIT University through an Emerging
Research Grant are gratefully acknowledged.
REFERENCES
[1] Kimbell, J., Shroeter, J.D., Asgharian, B., Wong, B.A.,
Segal, R.A., Dickens, C.J., Southall, J.P. and Millerk F.
J. (2004) Optimisation of nasal delivery devices using
computational models. Res. Drug Del., 9 233-238.
[2] Inthavong, K., Tian, Z.F., Tu, J.Y., Yang, W. and Xue, C.
(2008) Optimising nasal spray parameters for efficient
drug delivery using computational fluid dynamics.
Computers in Biology and Medicine, 38(6), 713-726.
[3] Inthavong, K., Tian, Z.F., Li, H. F., Tu, J.Y., Yang, W.,
Xue, C.L. and Li, C.G. (2006) A numerical study of
spray particle deposition in a human nasal cavity.
Aerosol Science Technology, 40, 1034-1045.
[4] Cheng, Y.S., Holmes, T.D., Gao, J., Guilmette, R.A.,
Li, S., Surakitbanharn, Y. and Rowlings, C. (2001)
Characterization of nasal spray pumps and deposition
pattern in a replica of the human nasal airway. J.
Aerosol Medicine, 14 (2), 267-280.
[5] Edwards, D.A., Hanes, J., Caponetti, G., Hrkach, J.,
Ben-Jebria, A., Eskew, M.L., Mintzes, J., Deaver, D.,
Lotan, N. and Langer, R. (1997) Large porous particles
for pulmonary drug delivery. Science, 276, 1868-1872.
[6] Gupta, A.K. and Gupta, M. (2005) Synthesis and sur-
face engineering of iron oxide nanoparticles forbio-
medical applications. Biomaterials, 25(18), 3995.
[7] McCarthy, J.R., Kelly, K.A., Sun, E.Y. and Weissleder,
R. (2007) Targeted delivery of multifunctional mag-
netic nanoparticles. Nanomedicine, 2(2), 153-167.
[8] Keyhani, K., Scherer, P.W. and Mozell, M.M. (1995)
Numerical simulation of airflow in the human nasal
cavity. J. Biomechanical Engineering, 117, 429-441.
[9] Subramaniam, R.P., Richardson, R.B., Morgan, K.T.,
Kimbell, J.S. and Guilmette, R.A. (1998) Computa-
tional fluid dynamics simulations of inspiratory air-
flow in the human nose and nasopharynx. Inhalation
Toxicology, 10, 91-120.
[10] Finckl M.H. and Wlokas, D.I. (2006) Simulation of
nasal flow by lattice Boltzmann methods. Computers
Biology Medicine, 37(6), 739-749.
[11] Hahn, I., Scherer, P.W. and Mozell, M.M. (1993) Ve-
locity profiles measured for airflow through a large-
scale model of the human nasal cavity. J Appl.
Physiol., 75(5), 2273-2287.
[12] Zamankhan, P., Ahmadi, G., Wang, Z., Hopke, P.H.,
Cheng,Y.S., Su, W.C. and Leonard, D. (2006) Airflow
and deposition of nanoparticles in a human nasal cav-
ity. Aerosol Science Technol., 40, 463-476.
[13] Mumford, D. and Shah, J. (1989) Optimal approxima-
tions by piecewise smooth functions and associated
variational problems. Commincations Pure and Ap-
plied Mathematics, XLII, 577-685.
[14] Swift, D.L. and Proctor, D.F. (1977) Access of air to
the respiratory tract., in Respiratory Defence Mecha-
nisms., Brain, J.D., Proctor, D.F. and Reid, L.M. Edi-
tors, Marcel Dekker, New York, NY, 63-93.
[15] Clift, R., Grace, J.R. and Weber, M.E. (1978) Bubbles,
Drops, and Particles. London, Academic Press Inc,
UK, London, Ltd.
[16] Ounis, H., Ahmadi, G.M.J.B., (1991) Brownian diffusion
of submicrometer particles in the viscous sub- layer. J.
Colloid and Interface Science, 143(1), 266-277.
[17] Li, A. and Ahmadi, G. (1992) Dispersion and deposi-
tion of spherical particles from point sources in a tur-
bulent channel flow. Aerosol ScienceTechnology, 16,
209-226.
[18] Saffman, P.G. (1965) The lift on a small sphere in a
slow shear flow. J. Fluid Mechanics, 22, 385-400.
[19] Talbot, L., Cheng, R.K., Schefer, R.W. and Willis, D.
R. (1980) Thermophoresis of particles in a heated
boundary layer. J. Fluid Mech, 101(4), 737-758.
[20] Cheng, K.H., Cheng, Y.S., Yeh, H.C., Guilmette, A.,
Simpson, S.Q., Yang, Y.H. and Swift, D.L. (1996)
In-vivo measurements of nasal airway dimensions and
ultrafine aerosol deposition in the human nasal and
oral airways. J. Aerosol Science, 27(5), 785-801.